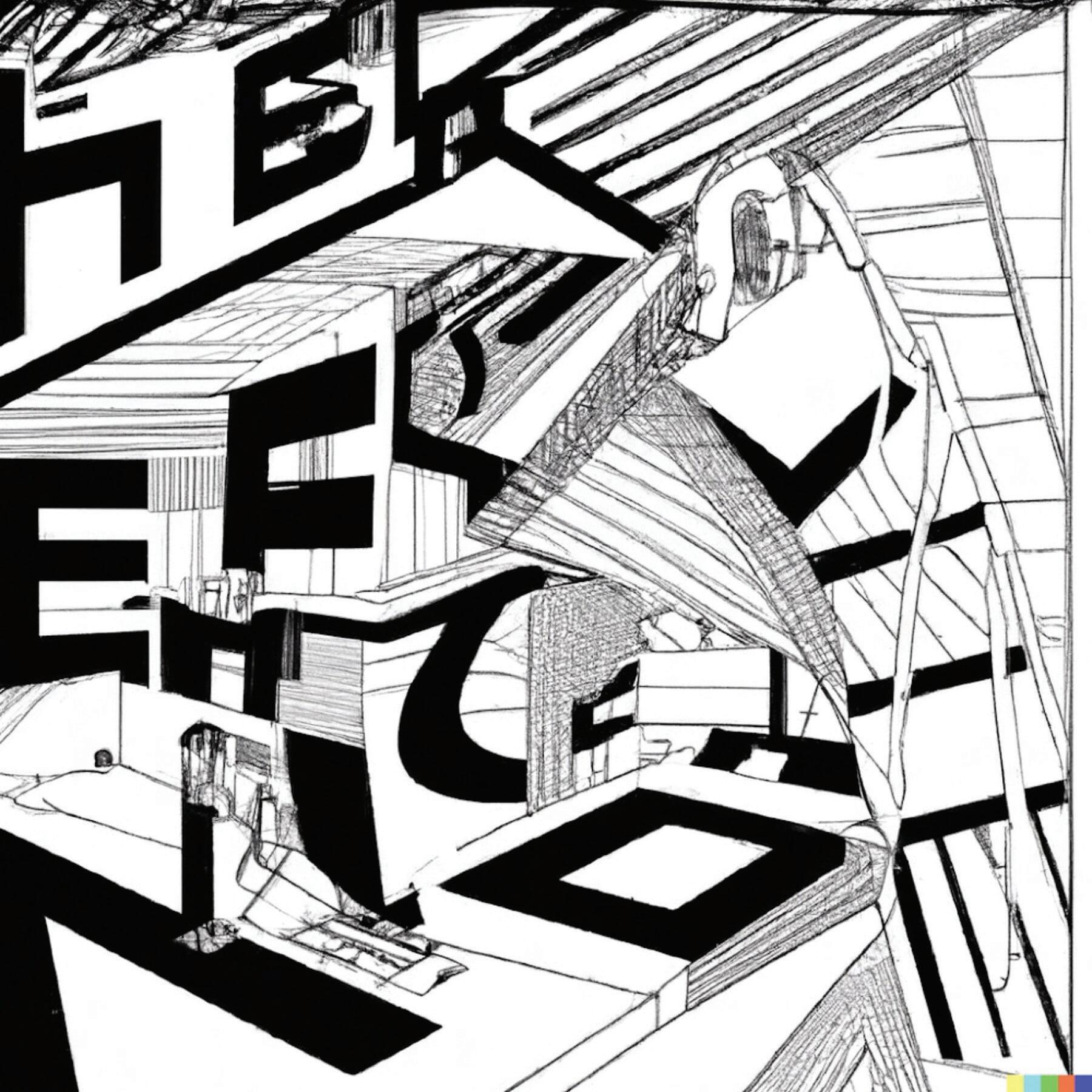
11 minute read
FILLING IN THE
FILLING IN THE ______:
How AI is changing the way we see writing
Advertisement
“You are about to begin reading Italo Calvino’s new novel, If on a Winter’s Night a Traveler. Relax. Concentrate. Dispel every other thought. Let the world around you fade. Best to close the door; the TV is always on in the next room. Tell the others right away, ‘No, I don’t want to watch TV!’ Raise your voice—they won’t hear you otherwise—‘I’m reading! I don’t want to be disturbed!’ Maybe they haven’t heard you, with all that racket; speak louder, yell: ‘I’m beginning chapter one of Italo Calvino’s new novel!’ Or if you prefer, don’t say anything; just hope that they’ll leave you alone.”
Thus begins Italo Calvino’s novel If on a Winter’s Night a Traveler. It is a masterpiece of metafiction, a form of fiction that relies not on traditional forms of narration but on referring back to itself in a way that brings the reader into the story as a character. The passage above makes Calvino’s metafiction attempts unmistakably clear; the narrator speaks directly to the reader, who is attempting to read a book called If on a Winter’s Night a Traveler. There is no longer a separation between narration and reader.
I decided one day to embark on some creative experimentation of my own, taking some lessons from Calvino’s writing:
“You are already deep into the first chapter. You have not understood a thing. You feel uneasy. It is not because of the book, which so far seems interesting enough; nor is it that your concentration is flagging (if anything, it feels as if it has been heightened); it is something else entirely. You must put your finger on what it is: an uneasiness about yourself. About your being here, sitting in this room and reading these very words. You feel like someone who has lost his way and now does not know where he belongs or how he got here: he no longer knows what his own story is about or what story he belongs in.”
But what I meant by experimentation was not that I actually wrote this paragraph myself. I input the first paragraph of Calvino’s novel into an AI program colloquially called GPT-3, and the program generated this paragraph for me. It is written in the style of Calvino, but it is not Calvino—a quick Google search revealed that the previous paragraph is a completely unique combination of words.
Admittedly, there are subtle stylistic differences between the two passages. The first passage relies heavily on words such as “dispel” and “tell” to command the reader, while the second passage takes a more passive tone to describe the reader. But we have reached a level of AI sophistication where, if we have not previously read Calvino’s novel, it becomes difficult to discern that the second passage was not written by him. The differences between the passages do not point us to an answer—it could be that Calvino intended the stylistic change.
As I read the passage produced by GPT-3, I became aware that the writing was describing my own feelings before I was even aware of it. I was initially surprised that GPT-3 was able to so closely model Calvino’s style while also diverging slightly from it. However, as I read the passage again, thinking especially about the “uneasiness” that GPT-3 describes, I suddenly began to realize that I was experiencing an uneasiness—an uneasiness that only compounded after I realized that GPT-3 had predicted my own state of mind.
How is it, then, that an AI like GPT-3 could generate text that says so much? More than simply adopting Calvino’s writing style, the model produced something that describes the experience of reading. The passage it generates feels like more than just words strung together by a computer—its words take on a meaning of their own, produce some sort of sensation within us, and illuminate something about the world of which we have previously been unaware.
+++
In late 2015, a handful of Silicon Valley giants, including Elon Musk and Sam Altman, the former president of the start-up incubator Y Combinator, gathered together to found the organization OpenAI. The founding of the organization was a response to radical technological developments at the time—in particular, the creation of a neural network architecture called AlexNet that had achieved unprecedented accuracy in correctly classifying objects in images. The discovery spurred the use of similar neural network architectures in various other AI tasks, including language prediction. GPT-3, short for Generative Pre-trained Transformer 3, is the third-generation language prediction model created by OpenAI. Its goal is clear: to generate text based on any written prompt, and to do so in a convincingly human way.
The motivation behind GPT-3 and other language models reflects a consistent trend in associating language with intelligence. The Turing test, proposed by Alan Turing in 1950, determines a machine’s ability to demonstrate human intelligence by testing if a machine can convincingly engage in a conversation with a human being. Turing was not concerned with the question of whether a machine could actually think, believing that this was a meaningless question. Instead, a machine that could pass the Turing test would be capable of something like thinking. And this ‘something like thinking’ is none other than using language.
GPT-3 is one of many language models that has been built, whether implicitly or explicitly, on this particular line of thinking. The model is not something that simply generates text: more precisely, it uses complex statistical patterns to determine the most likely result. The underlying idea of GPT-3 is that language, which is the building block for ideas, can be understood and produced in a mechanistic way. To use GPT-3, it is first necessary to give it a prompt: a question, an excerpt from a book, or any other sort of text. Then, through an iterative series of calculations, the model determines the word that would be most likely to succeed the previous word. This process continues until it determines a suitable place to end.
This mechanistic conception of writing has literary roots. Jorge Luis Borges’s short story “The Library of Babel” imagines a universe in the form of a near-infinite library that is composed of interconnected, hexagonal rooms. Each room contains books of 410 pages consisting of every possible combination of letters. Everything that has ever been written and will be written can be found in the Library; in other words, it contains every possible combination of letters in every language. The vast majority of the books are filled with nonsense, but it would seem that the entire corpus of human knowledge is contained in the library, including the answers to all of life’s mysteries, the explanations of every natural phenomenon, and the correct predictions of every future event. The inhabitants of the Library spend their lives futilely searching for this knowledge. Because everything that can possibly be written already exists, the act of writing only involves a process in which letters are strung together.
“The Library of Babel” hints at a future in which language models become the dominant form of writing. These models work by stringing together words in a mechanistic process governed by the laws of probability. What is striking about the Library is that it forces us to imagine that every possible combination of words already exists, and any text that is written is only the realization of some possibility. If we think of writing in this way, then what GPT-3 produces seems to be no different than what humans are doing: the process of writing may be different, but the end result, the text itself, shouldn’t be much different from a human-produced text. But such a conception of writing leaves out an important detail—the lived experience of humans that often goes into a particular work of writing.
Here is a question that has always bothered me about the Library, which seems particularly relevant in the context of GPT-3: what lies outside the Library? At first, this does not seem to be a particularly useful question—if the Library is infinite, then the obvious answer would be that there is nothing outside the Library. But this seemingly banal question becomes something puzzling and important. The Library contains every possible word in existence, including words we have not yet come to know, but these words don’t point to anything in the Library. The word “tree,” for instance, does not refer to an actual tree in the Library, and the Library contains all the textual knowledge of what a tree is without providing the knowledge of experiencing a tree.
GPT-3 behaves similarly to the inhabitants of the Library because it has no knowledge of what the words it produces actually signify. It can parse through nearly everything written by humans, but it is confined within this world of text that has no reference to the world outside of it. The round object that glows in the night sky is called a full moon. GPT-3 may be able to correctly predict the word “moon” in this sentence, but its existence strictly within the textual realm means that it has no way of knowing what the experience of seeing the moon is like.
This seems to pose some problems for the act of writing—if GPT-3 has no way of experiencing the world, how can GPT-3 ‘write’ in the way that Borges and Calvino did so flawlessly? It seems that while we can conceptualize both human writing and computer writing as a mechanistic stringing together of words, there is far more involved in the process. I often believe that there is something special to writing, writing as something that isn’t simply a combination of words, but something that arises from within the writer. When a human is writing, she is reflecting on her own ideas, thoughts, and experiences, and in that process gradually finds words to describe them. If told to write “a poem about the moon that evokes feelings of longing and solitude,” GPT-3 will churn out a poem that does precisely that. We can even ask GPT-3 to write in the style of a particular poet, and it will convincingly complete the task. But what GPT-3 can never do, which a human writer can, is actually experience the feelings of longing and solitude, the feeling that comes when one is sitting alone with one’s thoughts in the middle of the night and gazing at the moon. The poem is a result of this particular experience, and illuminates writing as a deeply human affair that cannot be emulated by artificial intelligence. The word ‘moon’ may exist within the Library, but the object it refers to lies firmly outside of it.
+++
In my brief experimentation with GPT-3, it seemed that what was most important to receiving high-quality output was crafting the right prompts. A prompt like “write an essay in which you analyze Calvino’s use of metafiction” produced something that vaguely resembled a five-paragraph essay with the straightforward style of an elementary school student. But when I entered the opening paragraph of Calvino’s novel, it was able to produce something convincingly Calvino-like with even a small stylistic change of its own. In artificial intelligence, this is a concept known as prompt engineering—the quality of output is directly correlated with the quality of the prompt given. The task of writing high quality prompts is not easy, and it emphasizes that language models are unable to write purely on their own. In a sense, they require a human to provide them with the experiential knowledge that they lack.
I can imagine a possible future where writing takes the shape of something collaborative between humans and AI. Writing, in its current human form, will not cease to exist, but there may be a growing proportion of writers who use models like GPT-3 to aid their own writing processes—no longer relying as heavily on the physical act of writing but on the artistic task of being able to write an elegant prompt. The focus may shift from writing to refining text: using the language model as a way to produce what the writer desires, a desire that still resides within us.
So how do we conceptualize writing in the context of AI? Perhaps there is no clear answer to this question. I will not lie—such experimentation with GPT-3 made me question my own abilities as a writer. But as I spent more time interacting with GPT-3 and exploring its capabilities, playing around with several prompts and watching the machine’s response quickly make its way across my screen, I could feel my initial unease dissipate. Several times, the machine’s output reflected an obvious lack of intelligence—digressions, repetition, poor word choice—but I also could not help but stare in silent stupefaction as it churned out lines of poetry in the manner of Shakespeare and metafiction in the style of Calvino. It drew out a curiosity in me that made me eager to play around more.
ERIC GUO B’23 is letting GPT-3 fill in the blanks.