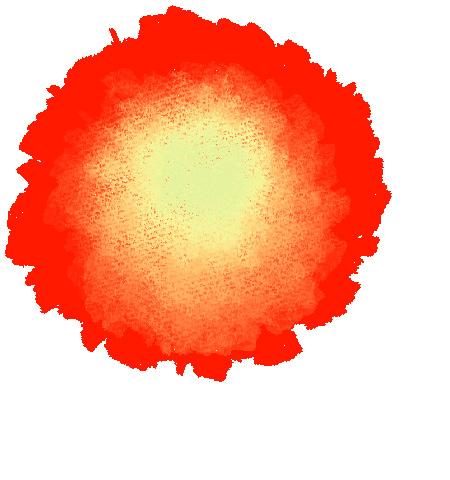
5 minute read
Mature Students We Are All
Who are mature students?
I would like to propose that we all are, and that if you are not, you should be. In a world of CPD and SMART goals; there is a lingering feeling we could just be one restructure from redundancy. Therefore, surely it is as important as ever to ensure not just that we are learning, but that we have evidence of it, for any prospective employer?
Advertisement
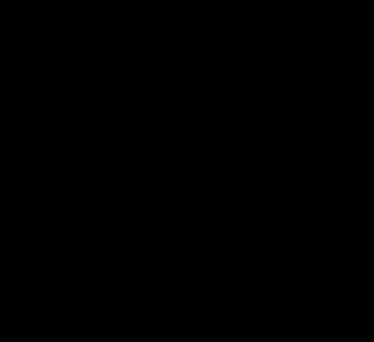
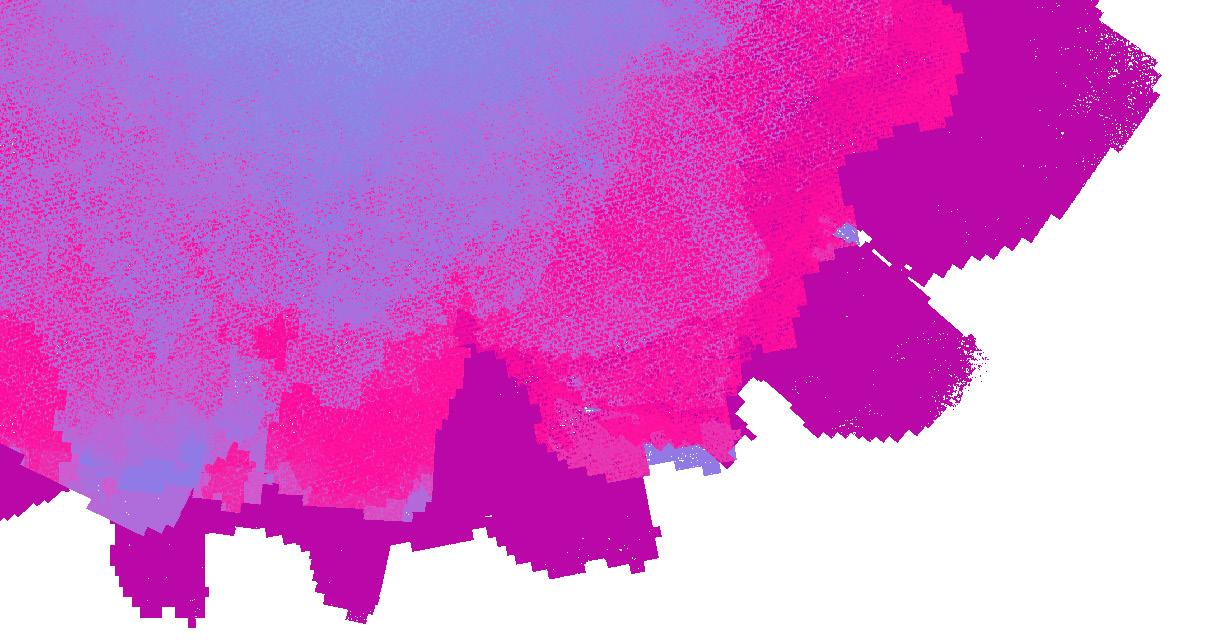
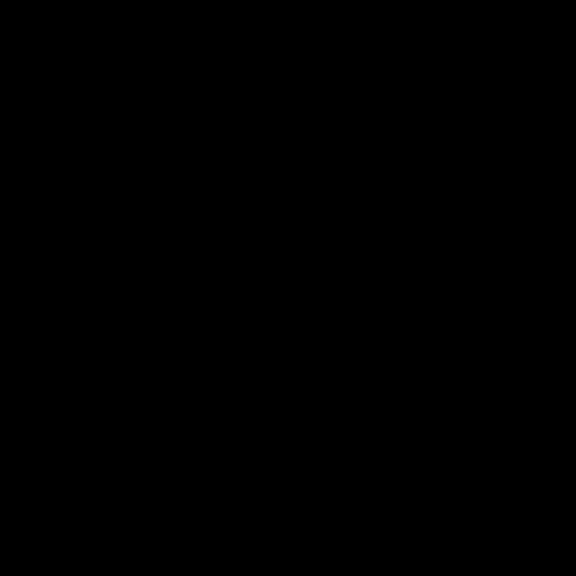
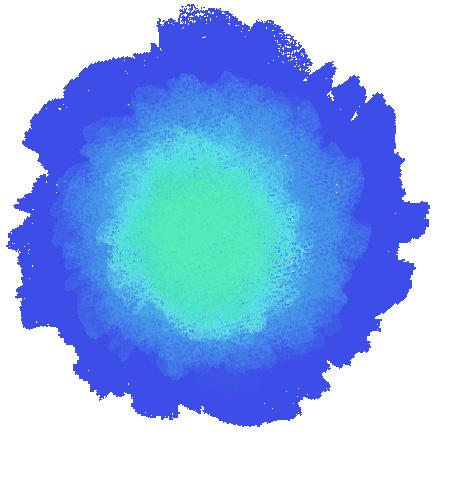
The days of a job for life are gone, we’re seeing GPs balloting to take strike action, with nurses and teachers already on the picket line. These are people who, when starting their training, believed their vocation guaranteed a career for life, in which they would be respected; and we might argue more importantly, appropriately renumerated. It would appear they now feel differently, and I wonder how many of them are noting their numerable transferable skills, for employment in another industry?
For those in full-time employment who believe their school days are a distant memory, I believe they are missing the bigger picture. I remember being excited about the Spice Girls launching a fifth T.V. channel and a day when nobody had mobile phones. Surely if the world we live in has changed so greatly, why wouldn’t the way of work? It may be possible if in your sixties, with the guarantee of retirement, to stick it out in the same role for your remaining work life, making plans to take up golf or sign up for that bread-making class. It may not be in the work environment but with a newfound amount of leisure time, surely this is a return to education, albeit one with no dusty used textbooks or detentions?
If those old enough to be in full-time employment or retirement could be classed as mature students, then how is an 18-yearold a mature student I hear you ask? In their final teenage years, they have been studying for two-thirds of their life. If they make the decision to continue into Higher Education after securing the necessary grades, why would we not consider them experts in studying? Knowing how and what to revise to pass the exams they have sat. Plus, they have observed the generation before, who are only just reclaiming their life/work balance, and so wisely understand that jumping into full-time employment should be done with caution.
Interestingly the Higher Education Statistics Agency class undergraduate mature students as 21 years or older and young students as those under 21, so by their standards
Design: Connor Wharton we are either young or mature, there is no middle ground! However, eligibility for a SAAS student loan is based on the age of 25, although if an undergraduate has a dependent, or supported themselves from earnings or has lived with a partner, they are now a mature student. Why are we so keen to put a numerical label on mature students, what is the benefit of this? It does not guarantee any better understanding of an individual’s circumstances or from my own experience the ability of those that may be classed as mature to identify as so.
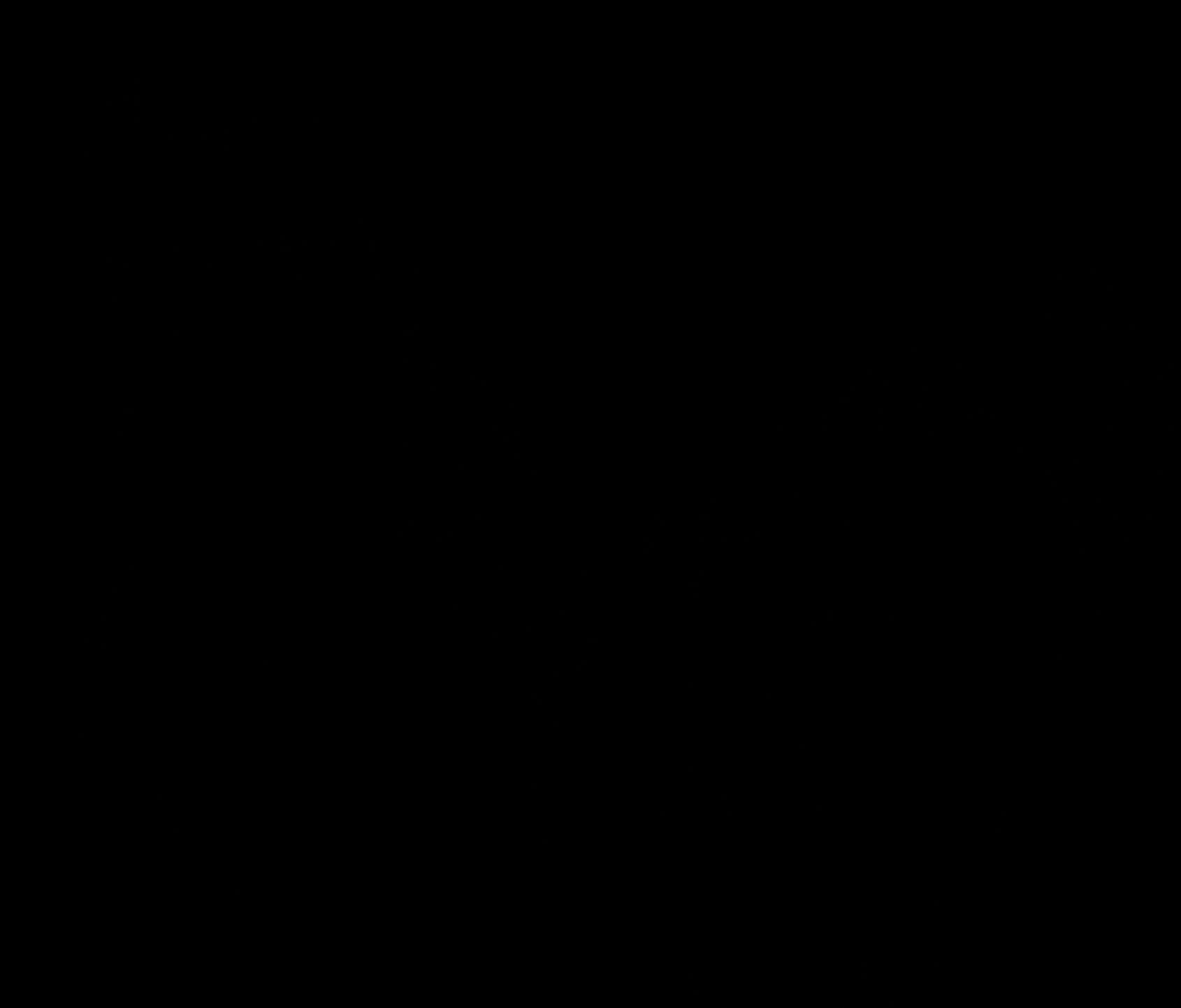
UCAS believe that ‘mature students’ are usually those entering tertiary education after a gap from secondary education, by including usually it supports my view that the term should be widened to be inclusive. I endorse this definition, as it moves away from age being the definer but instead to mindset, as anyone that is choosing to continue to learn is indeed a mature student.
The world is changing at an unprecedented rate, in both our personal and work lives. This is more than adaptation, as new skills are becoming prominent that didn’t exist a generation ago, to thrive and not just survive we need to keep our minds fresh and our CVs fresher, which is why I believe we should all be classed as mature students.
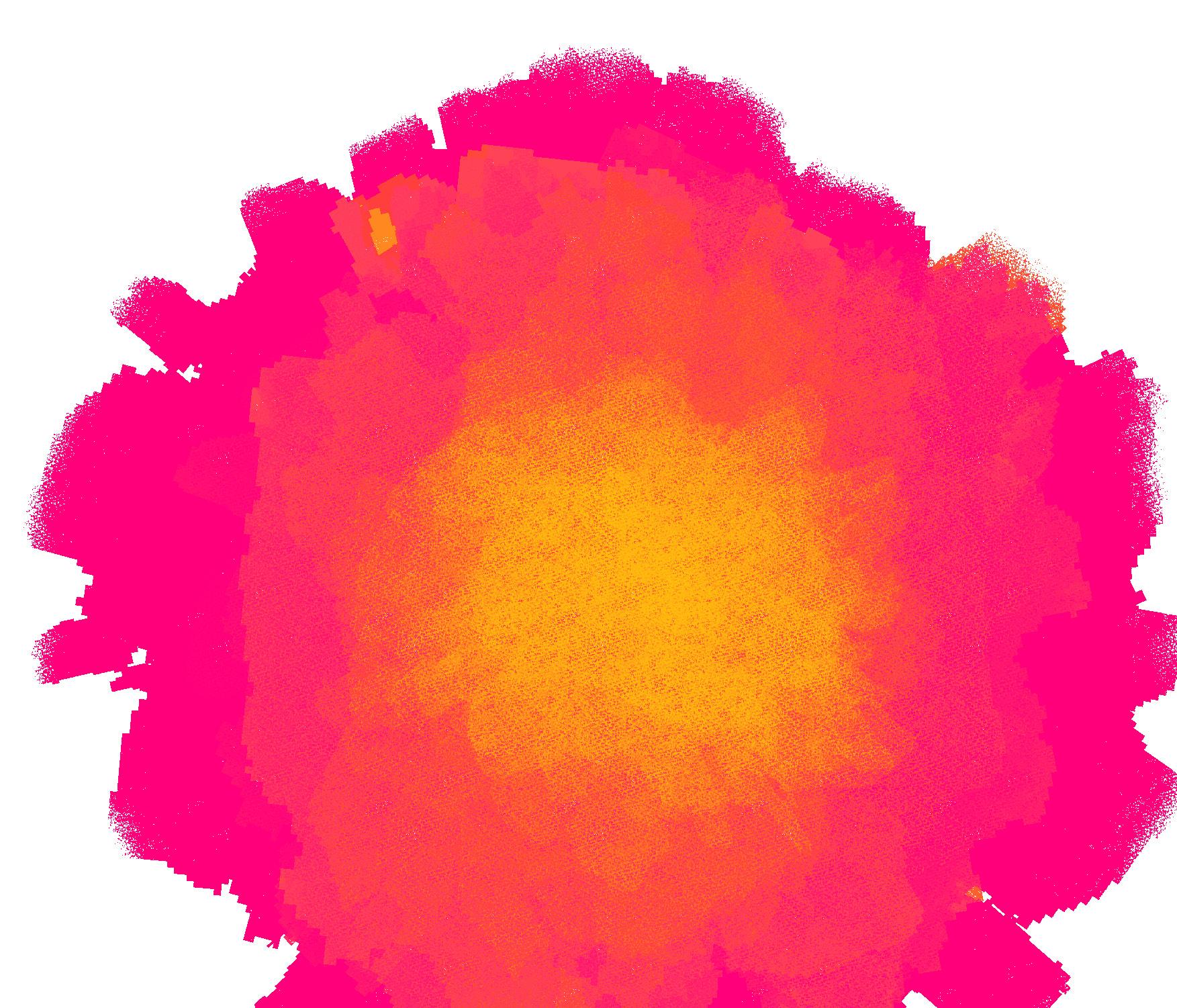
Continuation of ‘A chilling revolution: The age of Cryo-EM!’ from Issue 99
Our journey of Protein Structure determination began with X-ray crystallography, following which we explored Cryo-EM. But as is with every technique, it too possessed shortcomings. As such, another journey began for structural biologists, resulting in a collaboration that utilised AI in the quest for structure determination. But before discussing this, let us look at a brief history of protein structure determination.
If one wanted to understand the structure of a miniature building, looking at Legos might be a good way to start. Anfinsen chose a similar path, postulating that a proteins structure could be determined purely by the sequence of amino acids that coded them. This dogma did have some firm grounding, as the chemical structures of these amino acids affected how a protein folded.
And yet it did not work with certain proteins; considerations weren’t made regarding other cellular processes that played a role in the folding process. Eventually, these missing gaps of understanding lead to the compilation of the protein folding problem. The issue drew from the fact that chemical structures that would come to be identified as proteins were ultimately made of atomic and sub-atomic particles , hence governed by the laws of thermodynamics, biochemistry and a bit of quantum physics.
However, the theoretical landscape was not the only thing that shaped structural biology.
With experimentation, the efforts of researchers had given rise to the powerful Cryo-EM and X-ray crystallography techniques. Moreover, these techniques had begun percolating in research labs, with the ever-growing number of results being entered into a central database - The Protein Data Bank. As such, structural biologists found themselves amidst a partially filled database with a growing theoretical understanding of the laws that enable chemical interactions which shape their field.
Parallelly, the computational potential was on a logarithmic curve, with the understanding and capacity of computer programming blooming exponentially, utilising optimized programmes to receive complex outputs. Many tech companies had started expanding into the biological landscapes, with one such example being Google’s company DeepMind. Their own project saw them developing an algorithm for predicting protein structure using AI.
I have written about DeepMind and AlphaFold in Issue 92 of The Magdalen, but here I want to present a broad outline of the process of AI-based structure determination.
When a protein sequence for an unknown protein is obtained, it is compared with preexisting sequences to check for similarities, and hence their structure. For this article, let us assume that one such sample has a partial match with a pre-existing sequence.
The matched part is corrected and personalised – i.e. the unsimilar parts within the aligned sequence are marked for consideration during structure construction – upon a match for the unresolved protein. Once this is done, specific rules, including geometry and biochemical interactions, are used to draw a skeleton of the structure. Eventually, after multiple rounds of correction and optimisation, a part of the unresolved protein is readyconstructed in silico.
But along with this resolved part constructed from the aligned and matched sequence of the protein, the unmatched sequence is also given a construction attempt – but with a disclaimer known as the model confidence. But that is a topic perhaps for some other time. So is that it? Is the toolkit for structural biology complete?
Painstakingly, and patiently, structural biologists have picked apart the final protein structures one by one, and in doing so, pushed the development of the various techniques. But the journey of inventing new techniques does not end here; considering this series so far I am sure you know that. Perhaps, a new technique is slowly taking shape somewhere, awaiting to find its place in the world of structural biology!