
26 minute read
Artificial intelligence in diagnosing lung
from Issue 39
CRITICAL REVIEW Artificial intelligence in diagnosing lung cancer: Applications and future developments
Bachelor of Health Sciences (Honours), Class of 2024, McMaster University Correspondence: phungj3@mcmaster.ca
Advertisement
ABSTRACT Computer-aided diagnosis (CAD) systems lie at the intersection of medicine and computer science. Over the last couple of decades, consistent research and technological advances have resulted in a steady improvement of CAD systems that are capable of assisting in the detection and diagnosis of various diseases. However, several limitations prevent CAD from being implemented in clinical practice. The primary purpose of this review is to provide a general overview of CAD systems in the context of lung cancer, as well as assess the critical challenges that CAD must overcome. Such challenges include data privacy and sharing laws, radiologist workflow integration, and the lack of a standardized performance evaluation. Thus, coordination between radiologists, researchers, and medical institutions will play a pivotal role in shaping the future development of CAD systems in healthcare.
CONTEXT The applications of artificial intelligence (AI) in the field of medicine have made much headway in recent years, especially with regards to detection and diagnosis of diseases using computer-aided diagnosis (CAD) systems. AI utilizes computers to simulate human intelligent processes, including learning, reasoning, and thinking.1 In particular, convolutional neural networks (CNNs), a subset of AI, can automatically extract image features after training on labelled samples.1,2 Given the availability of large datasets and increased computing power, CNN-based CAD systems have produced promising results for many tasks, including image classification, correct image detection, and segmentation, proving themselves capable of replacing current CAD systems based on manual input.3
Although there are variations in how CAD systems function, most undergo five general steps: acquisition, preprocessing (increasing the precision and accuracy of algorithms), segmentation (separating the study region from other organs and tissues in radiographic images), nodule detection (marking the location of pulmonary nodules in the image), and elimination of false positives.4 CAD systems are further divided into computer-aided detection (CADe) and computer-aided diagnosis (CADx).3 This distinction is critical as their functions differ: CADe systems detect potential lesions and reveal abnormalities in medical images, whereas CADx systems primarily serve to characterize, classify, and distinguish lesions.5
Although the purpose of CAD is to assist radiologists in the detection of lung nodules, CAD has not been implemented in routine clinical practice, with research efforts still focused primarily on improving performance.4 Currently, computerized tomography (CT) scans are the most common imaging modality for radiomics analyses, especially for diagnosing lung cancer, due to their high spatial resolution, cost-effectiveness, wide availability, and noninvasiveness.5,6 CNNs have been successfully developed to detect pulmonary nodules and lesion segmentation through training on publicly available databases and testing on various datasets such as the Lung Image Database Consortium and Image Database Resource Initiative (LIDC-IDRI).7,8
EVALUATING EFFECTIVENESS OF CAD CAD can potentially reduce radiologists’ workloads and enhance their performances. Reading time is significantly shorter with CAD used as a concurrent reader compared to when the reading is done after interpretation by a radiologist.4 Moreover, CAD has been shown to improve the performance of experienced and inexperienced radiologists.9 Kligerman et al. determined that CAD can improve a radiologist’s ability to accurately detect lung nodules that were initially missed, while Sahiner et al. further demonstrated that it can help detect small size nodules under five millimetres, which are easily overlooked by inspection alone.10,11 As such, CAD consistently improves performance among thoracic radiologists and can play a critical role in the detection of lung nodules.
Despite the clear impact of CAD on lung nodule detection, its performance varies significantly depending on the study. Generally, a research study’s CAD system can be evaluated through several metrics including accuracy, precision, sensitivity, specificity, true positive rate, and false positive rate.2 Although the establishment of databases like the LIDCIDRI has provided a large repository of training images that have steadily improved sensitivity and specificity, CAD systems remain inconsistent depending on the method and training dataset.12 For example, one model trained on the LIDC-IDRI dataset was a multi-view CNN, a 3D model that encodes for richer spatial information. Its error rate was 5.41%, and the sensitivity and specificity rates were 90.49% and 99.91%, respectively.12 Conversely, another study conducted by Nishio et al. utilized the deep CNN method on a clinical dataset; their highest reported accuracy score was 68.0%.13 Overall, the CAD effectiveness varies depending on the method and training dataset used.
Much of the existing literature is focused on CADe systems, involving the detection of nodules or lesions. Zhang et al. noted in their appraisal that there have been many reviews about CADe systems, but few regarding CADx systems.5 CADe and CADx are often regarded separately, with most research focused on individually optimizing either system. 14
1. Cong L, Feng W, Yao Z, Zhou X, Xiao W. Deep learning model as a new trend in computer-aided diagnosis of tumor pathology for lung cancer. J Cancer. 2020;11(12):3615-22. Available from: doi:10.7150/jca.43268. 2. Gao J, Jiang Q, Zhou B, Chen D. Convolutional neural networks for computer-aided detection or diagnosis in medical image analysis: An overview. Math Biosci Eng. 2019;16(6):6536-61. Available from: doi:10.3934/mbe.2019326. 3. Lee SM, Seo JB, Yun J, Cho YH, Vogel-Claussen J, Mark L, et al. Deep learning applications in chest radiography and computed tomography. J Thorac Imaging. 2019;34(2):75-85. Available from: doi:10.1097/RTI.0000000000000387. 4. Al Mohammad B, Brennan PC, Mello-Thoms C. A review of lung cancer screening and the role of computer-aided detection. Clin Radiol. 2017;72(7):433-42. Available from: doi:10.1016/j.crad.2017.01.002. 5. Zhang G, Yang Z, Gong L, Jiang S, Wang L, Cao X, et al. An appraisal of nodule diagnosis for lung cancer in CT images. J Med Syst. 2019;43(7):181. Available from: doi:10.1007/s10916-019-1327-0. 6. Chen B, Zhang R, Gan Y, Yang L, Li W. Development and clinical application of radiomics in lung cancer. Radiat Oncol. 2017;12(1):154. Available from: doi:10.1186/s13014-017-0885-x. 7. Benzaquen J, Boutros J, Marquette C, Delingette H, Hofman P. Lung cancer screening, towards a multidimensional approach: Why and how? Cancers (Basel). 2019;11(2):212. Available from: doi:10.3390/cancers11020212. 8. Armato SG 3rd, McLennan G, Bidaut L, McNittGray MF, Meyer CR, Reeves AP, et al. The Lung Image Database Consortium (LIDC) and Image Database Resource Initiative (IDRI): A completed reference database of lung nodules on CT scans. Med Phys. 2011;38(2):915-31. Available from: doi:10.1118/1.3528204. 9. Marten K, Seyfarth T, Auer F, Wiener E, Grillhösl A, Obenauer S, et al. Computer-assisted detection of pulmonary nodules: Performance evaluation of an expert knowledge-based detection system in consensus reading with experienced and inexperienced chest radiologists. Eur Radiol. 2004;14(10):193038. Available from: doi:10.1007/s00330-0042389-y. 10. Kligerman S, Cai L, White CS. The effect of computer-aided detection on radiologist performance in the detection of lung cancers previously missed on a chest radiograph. J Thorac Imaging. 2013;28(4):244-52. Available from: doi:10.1097/ RTI.0b013e31826c29ec. 11. Sahiner B, Chan HP, Hadjiiski LM, Cascade PN, Kazerooni EA, Chughtai AR, et al. Effect of CAD on radiologists' detection of lung nodules on thoracic CT scans: Analysis of an observer performance study by nodule size. Acad Radiol. 2009;16(12):1518-30. Available from: doi:10.1016/j.acra.2009.08.006. 12. Kang G, Liu K, Hou B, Zhang N. 3D multi-view convolutional neural networks for lung nodule classification. PLoS One. 2017;12(11):e0188290. Available from: doi:10.1371/journal.pone.0188290. 13. Nishio M, Sugiyama O, Yakami M, Ueno S, Kubo T, Kuroda T, et al. Computer-aided diagnosis of lung nodule classification between benign nodule, primary lung cancer, and metastatic lung cancer at different image size using deep convolutional neural network with transfer learning. PLoS One. 2018;13(7):e0200721. Available from: doi:10.1371/journal.pone.0200721. 14. Ozdemir O, Russell RL, Berlin AA. A 3D probabilistic deep learning system for detection and diagnosis of lung cancer using low-dose CT scans. IEEE Trans Med Imaging. 2020;39(5):1419-29. Available from: doi:10.1109/TMI.2019.2947595. 15. Liu B, Chi W, Li X, Li P, Liang W, Liu H, et al. Evolving the pulmonary nodules diagnosis from classical approaches to deep learning-aided decision support: Three decades' development course and future prospect. J Cancer Res Clin Oncol. 2020;146(1):153-85. Available from: doi:10.1007/s00432-019-03098-5. 16. Abouelmehdi K, Beni-Hssane A, Khaloufi H, Saadi M. Big data security and privacy in healthcare: A review. Procedia Comput Sci. 2017;113(1):73-80. Available from: doi:10.1016/j.procs.2017.08.292. 17. Bossuyt PM, Reitsma JB, Bruns DE, Gatsonis CA, Glasziou PP, Irwig L, et al. STARD 2015: An updated list of essential items for reporting diagnostic accuracy studies. BMJ. 2015;351(1):h5527. Available from: doi:10.1136/bmj.h5527. 18. Savitha G, Jidesh P. Advances in Intelligent Systems and Computing. Singapore: Springer; 2019. 11-23 p. 19. Ather S, Kadir T, Gleeson F. Artificial intelligence and radiomics in pulmonary nodule management: Current status and future applications. Clin Radiol. 2020;75(1):13-9. Available from: doi:10.1016/j. crad.2019.04.017. 20. Yanase J, Triantaphyllou E. The seven key challenges for the future of computer-aided diagnosis in medicine. Int J Med Inform. 2019;129(1):413-22. Available from: doi:10.1016/j.ijmedinf.2019.06.017. However, a study by Ozdemir et al. suggests that coupling CADe and CADx systems can result in improved performance.14 Using an open-source dataset, CADe and CADx systems were developed simultaneously, allowing for an optimized system which can reduce the false positive rate and serve as an end-to-end automated diagnostic tool for lung cancer.14
ETHICAL AND PRACTICAL LIMITATIONS Several limitations currently plague the field of CAD and hinder its development. Data scarcity is one such challenge, as properly and reliably training a CAD system is resource-intensive. Labeling tools must be made available to radiologists to create high-quality datasets, and although databases are expanding, a higher volume of data is required to improve functionality.15 There are several work-arounds, such as transfer learning —a machine learning method that involves pre-training on a large dataset, followed by fine-tuning on another dataset— that has been demonstrated to show improved accuracy despite the lack of labeled images.13 Supervised learning could also be utilized on a small portion of the dataset to train networks, followed by unsupervised learning that classifies the remaining unlabeled data.15 However, the primary concern pertains to legal and ethical issues surrounding data privacy.
Numerous data confidentiality laws govern the use of patient images in academic settings, and medical institutions may face fines if personal health information is compromised.3 Despite the need for extensive high-quality images to train CNNs, healthcare organizations may be deterred from contributing to a shared learning dataset as the possibility of mishandling medical images and facing litigation is not worth the risk. Nevertheless, data security in healthcare continues to improve, and new privacy-preserving models such as deidentification and anonymization are crucial to safeguarding and managing patient records.16 Once the security of privacyenhancing technologies is strengthened, the risks associated with uploading and sharing patient data can be drastically reduced, and de-identified patient data can be used for research with the approval of a research ethics board. Thus, a vast number of medical facilities may be incentivized to collaborate and contribute to a shared training dataset for CAD systems.
Another challenge that must be addressed is the diagnostic accuracy of CAD. The performance of CAD systems is steadily improving, but still varies with the study or method. The lack of a standardized performance assessment for CAD systems adds to the problem. Although at least one measure of accuracy is recommended by the Standards for Reporting of Diagnostic Accuracy Studies, the specific measures are not clearly stated and measures reported in published articles remain inconsistent depending on the application of the CAD system (e.g. segmentation or classification).17 By establishing a well-defined standard such that the most important metrics are consistently reported in the literature, comparing different CAD systems becomes easier and more reliable, which would provide a direction for future researchers to build on past studies. In particular, Gao et al. highlighted eight common evaluation metrics that can be used to form a standard, including accuracy, precision, sensitivity, specificity, and true and false positive rates.2 CAD systems that perform well against other systems can then be replicated; for example, the optimal deep CNN method that yields high results across several metrics can be further investigated by researchers on alternate datasets.18 Thus, developing a standardized evaluation of various CAD systems is necessary to unify research efforts.
Finally, coordination between radiologists and researchers is needed to integrate CAD into clinical practice. Despite its potential to improve radiologist performance, CAD systems remain absent in clinical settings as they fail to integrate with radiologists’ workflow.19 As a result, CAD systems are likely to be regarded as low priority compared to their clinical tasks.2,19 Nevertheless, combining the knowledge of radiologists and computer analysis can enhance CAD system performance.5 Radiologists have a strong understanding of the diagnostic process and can identify the strengths and areas of improvement
of current CAD systems. The implementation of a feedback system would offer insight and guidance to academic researchers developing practical CAD systems.20
CONCLUSION Artificial intelligence has the potential to improve patient outcomes by detecting, classifying, and diagnosing pulmonary nodules. Although substantial progress in CAD research has been made, there are still significant barriers to widespread clinical implementation. Current research suggests that developing CADe and CADx simultaneously can optimize performance. High-quality databases are required to train CAD systems, while privacy and security are crucial to ethically and legally share patient data for research. Furthermore, the lack of a standardized performance assessment tool has persistently made comparison between published literature difficult. Successful collaboration between professionals in the field of medicine and computer science is necessary to improve the effectiveness of CAD systems and, by extension, patient care.
REVIEWED BY: DR. DAVID KOFF
Dr. David Koff is a Professor of radiology and Chair of the Department of Radiology at McMaster University. He leads research projects on radiation risk, the validation of technology, and applications of artificial intelligence to medical imaging. He is currently the Chair of Canada Safe Imaging (CSI), an initiative he launched to promote radiation safety in Canada.
EDITED BY: NICK TELLER & TAAHA HASSAN
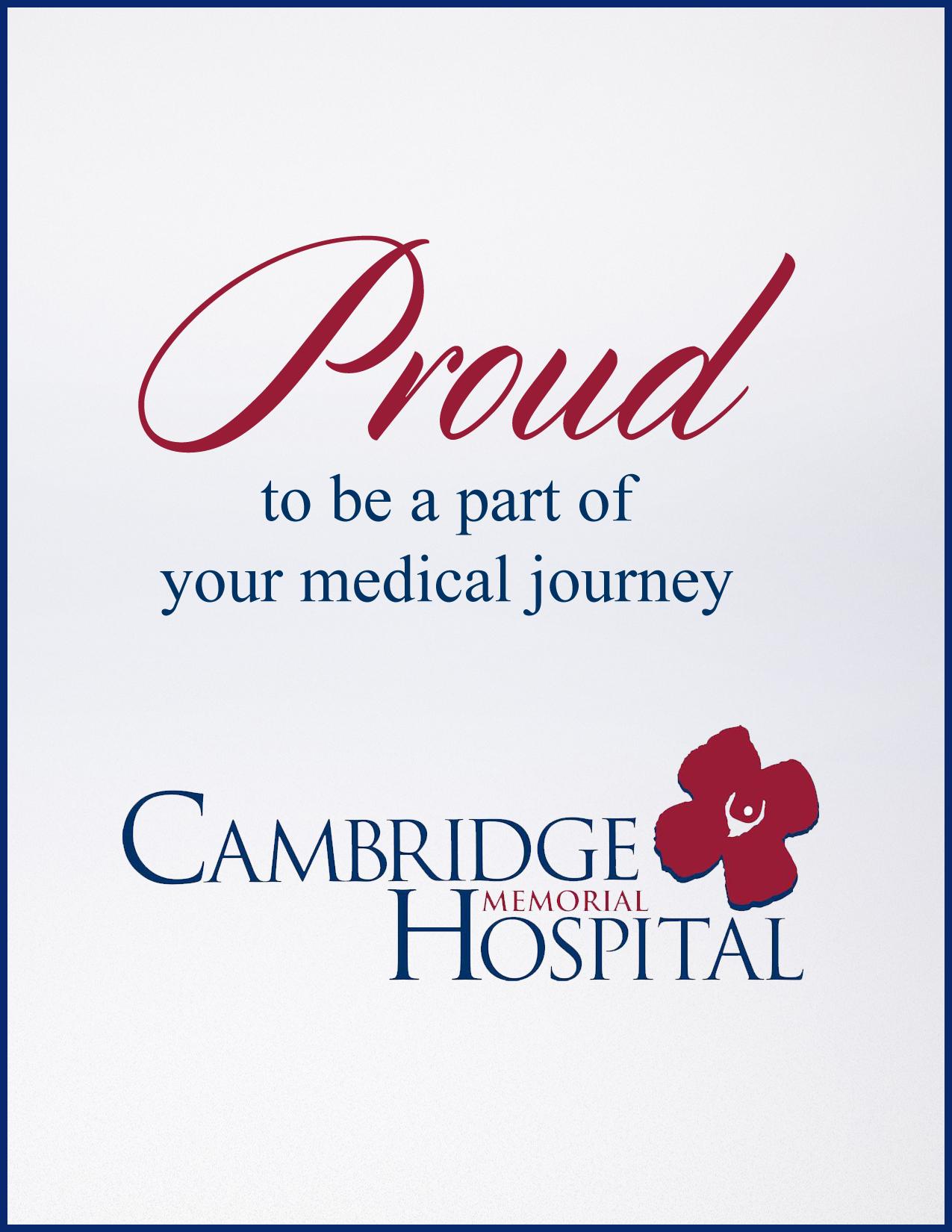
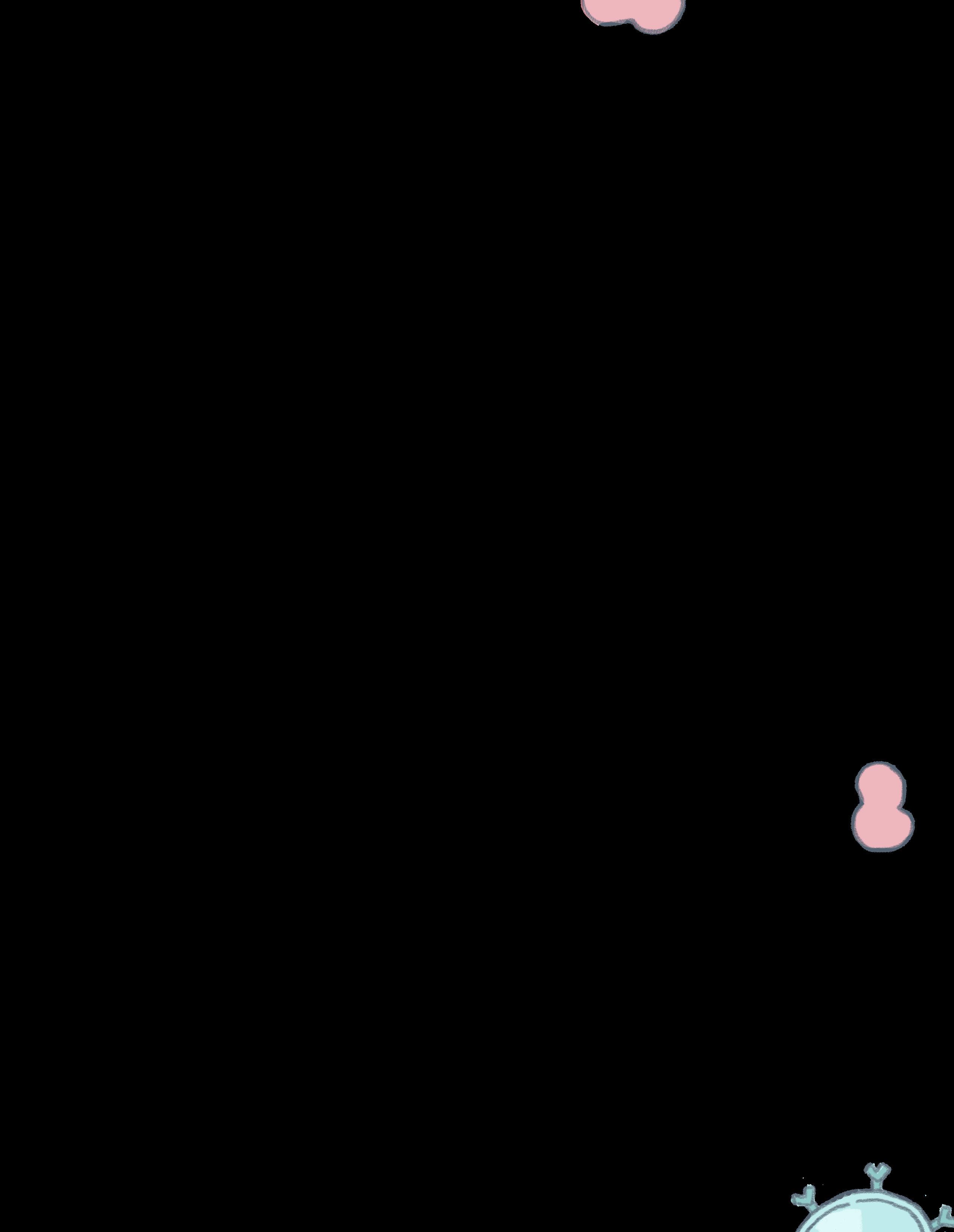
CRITICAL REVIEW
CARs on the road: Who gets a seat?
KATHERINE TAPLIN1 & PREETAMA BADYAL1
1Bachelor of Health Sciences (Honours) Class of 2024, McMaster University
ARTIST BEVERLY NG
ABSTRACT Chimeric antigen receptor (CAR) T-cell therapy is a form of immunotherapy that has shown potential for inducing complete remission in relapsed/refractory hematopoietic cancers. Depending on the patient’s therapeutic needs, CAR T-cells may be constructed using different cytoplasmic and extracellular domains to alter their affinity, persistence, and proliferation. While the therapy holds promise, the conventional use of CAR T-cell therapy is limited by the occurrence of side effects, substantial rates of relapse, and manufacturing logistics. The objective of this review is to discuss the potential of CAR T-cell therapies, as well as patient characteristics which may influence its efficacy.
INTRODUCTION
Engineering T-cells became commonplace in the mid-2000s, with first generation chimeric antigen receptors (CARs) developed in 1993.¹ Currently approved CAR T-cell therapies are developed from the patient’s own T-cells.² These cells are engineered to express specific T-cell receptors targeted to an antigen on the surface of cancerous cells, allowing the immune system to identify previously unrecognizable immunomodulatory cancer cells.2,3 CAR T-cells most commonly use a single-chain variable fragment (scFv) on the CAR to bind to cancerous antigens.⁴ When this binding occurs, a signal is generated and transmitted through the CAR cytoplasmic domain, consisting of costimulatory and signalling domains, activating the CAR T-cell.² Once activated, the T-cells initiate cytotoxic functions which can yield cancer cell elimination.²
Currently, the United States Food and Drug Administration (FDA) has approved CAR T-cell therapies for the treatment of relapsed/refractory B-cell acute lymphoblastic leukemia (R/R B-ALL) and lymphomas in children and young adults.¹ Although this treatment has shown promising results, the procedure has been shown to cause adverse effects. The objective of this review is to present the benefits, challenges, and variations in success rates across demographics of patients receiving CAR T-cell therapies.
ADVANTAGES OF CAR T-CELL THERAPY
Inducing Complete Remission CAR T-cell therapies have shown great promise for treating relapsed/refractory hematopoietic cancers by inducing complete remission in patients for whom chemotherapy is no longer effective. There are two main CAR T-cell therapies approved by the FDA: Yescarta and Kymriah.⁵ The CAR T-cells used in these treatments target the CD19 antigen found on B-cell cancers like ALL and B-cell lymphomas. ⁶ In a clinical trial, Kymriah was administered to 93 patients with refractory B-cell lymphomas, with 52% of patients responding to the treatment and 40% achieving complete remission.⁷ In a clinical trial to test the efficacy of Yescarta, 101 patients with a type of refractory lymphoma received an infusion.8 The overall response rate after one year was 82%, out of which 58% achieved complete remission.8 These studies demonstrate the efficacy and potential for future CAR T-cell therapies.
Reducing Off-Tumor Toxicities One of the challenges in developing efficient cancer treatments is reducing toxicity to non-cancerous cells.9 Many chemotherapeutic drugs target highly active pathways involved in the cell cycle that are not specific to cancer cells, thereby killing healthy cells and worsening the patient’s prognosis.¹⁰ In comparison, CAR T-cell therapies offer a degree of specificity that is absent from conventional treatments. CAR T-cells can be constructed using various scFv domains to recognize a particular antigen on cancerous cells, such as CD19 found on B-cell cancers.¹1 Thus, CAR T-cell therapy has potential to significantly reduce off-tumor toxicities.
However, off-tumour toxicities are still commonplace in patients who receive CAR T-cell therapies, which requires additional treatments to manage associated adverse effects. One common example seen with CD19 CAR T-cell therapy is the ablation of B-cells, which renders the patient immunodeficient.12 This occurs because the CD19 antigen found on hematological cancers are also found on normal B-cells.6 These patients can be treated through periodic administration of intravenous immunoglobulins to replace the antibodies no longer being produced. Nevertheless, there are other off-tumor toxicities for which further research is needed to determine the mechanisms involved and the appropriate treatments.12
Variability of CAR T-Cells Depending on the therapeutic needs of patients, CAR T-cells can be altered at the scFv and the cytoplasmic domain to modulate affinity, persistence, and proliferation.4 Using mouse tumor models, Liu et al. observed that CAR T-cells constructed using lower affinity scFvs had fewer off-tumour responses, increasing their therapeutic index.13 Studies suggest that high affinity scFvs may hinder CAR T-cells’ ability to discriminate between cancer cells and healthy cells.13 CD19 CAR T-cells with higher affinity scFvs for their target antigen have greater anti-tumor activity.12
Additionally, in a study comparing the functionality and persistence of CAR T-cell cytoplasmic domains, Zhao et al. concluded that CAR T-cells with a CD28 cytoplasmic domain expand more efficiently than those with a 4-1BB domain, leading to more rapid cytotoxic effects.¹⁴,¹⁵ However, 4-1BB CAR T-cells demonstrate a higher persistence, generating long-term tumor immunity and reducing the risk of cancer relapse.¹³,¹⁶ These two instances highlight the complex interrelationships at play in cancer immunology, complicating the development of an efficacious CAR T-cell therapy.¹⁷
SETBACKS IN CAR T-CELL THERAPY
Cytokine Release Syndrome A primary adverse effect of CAR T-cell therapies is cytokine release syndrome (CRS), marked by elevated serum cytokine levels.¹⁵ Cytokines are small proteins released by immune cells acting as chemical messengers.¹⁸ Following their interactions with cancerous cells, activated CAR T-cells release pro-inflammatory cytokines.¹⁵, ¹⁹ Symptoms of CRS include fever, myalgia, hypotension, and hypoxia, with severe cases resulting in hemodynamic compromise, capillary leak, arrhythmias, renal failure, and various other complications.¹⁹ These life-threatening symptoms are also typically seen in early onset CRS, occurring within three days of CAR T-cell infusion.¹⁵ Studies done on Bcell malignancies have shown that factors such as a high bone marrow tumor burden and higher CAR T-cell dosages pose a greater risk for developing severe CRS through rapid CAR Tcell expansion.²⁰, ²¹
The incidence and severity of adverse effects can be greatly diminished with knowledge of CRS management and early intervention. A recent study suggests that lymphoma patients over 18 years of age display a lower incidence of severe CRS compared to a population under 18 years of age.²² In contrast, another study suggests the opposite pattern in patients with R/R ALL, where higher rates of CRS have been reported in the adult population.²³ These findings suggest a variance in the extent of CRS observed within different cancer types and a need for further research to clarify the relationship between age and CRS severity.
Immune Effector Cell-associated Neurotoxicity Syndrome Immune Effector Cell-Associated Neurotoxicity Syndrome (ICANS), a form of neurotoxicity, is the second most prominent adverse effect associated with CAR T-cell therapy.²⁴ ICANS presents as an encephalopathy, resulting in confusion, aphasia, cerebral edema, and motor weakness, potentially leading to comas, seizures, and death.⁸ The occurrence of ICANS associated with CD19 CAR T-cell therapy varies between 23-67% among patients with lymphoma, and between 40-62% in patients with leukemia.¹⁵ As CAR T-cell therapy is a relatively novel immunotherapy, the mechanism of ICANS development is currently not well understood.²⁵ However, there are two prevailing theories regarding its development. The first pertains to the disruption of the blood-brain barrier (BBB). Patients who develop ICANS have high levels of pro-inflammatory cytokines known to interact with endothelial cells of the BBB, which protects the brain from circulating toxins and pathogens.²⁶-²⁸ Increased cytokine levels may lead to BBB permeabilization, allowing cytokines to enter the brain.²⁷ The second theory is related to the expression of CD19 on brain cells. In a study by Parker et al., a population of healthy cells in the brain were found to express CD19.²⁹ Since these cells express the same target antigen as the cancerous cells, CAR T-cells may mistakenly target or damage them.²⁹
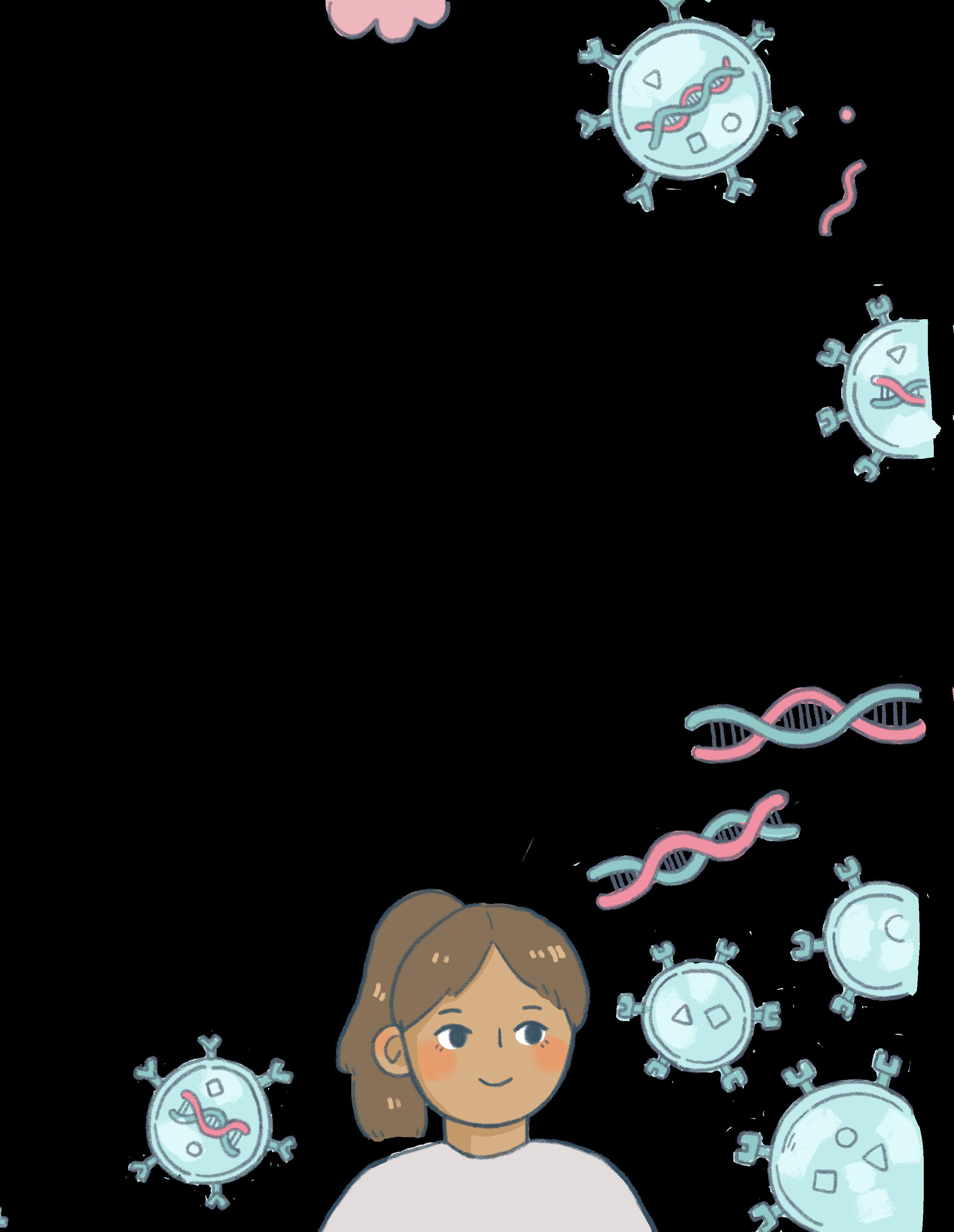
CAR T-cell designs and dosages may also influence the risk of developing ICANS. CAR T-cells with a CD28 cytoplasmic domain have greater proliferation, but lead to a greater incidence of ICANS.¹⁵,³¹ Additionally, higher doses of CAR T-cells have been associated with an increased risk of neurotoxicity.²⁷,³⁰ Further research on ICANS may help identify high-risk patients preemptively to allow for appropriate adjustments in CAR Tcell design and dosage.³¹
Treatment Logistics Financial costs, storage, handling, and timeline of treatment all hinder the widespread clinical administration of CAR T-cell therapy. There is a concern regarding the financial burden that the treatment imposes on patients.¹⁵ For patients with ALL, an infusion of Kymriah is over $200,000 USD more expensive than the average 100-day hematopoietic stem cell transplantation treatment (HSCT).³²,³³ Nonetheless, it is important to recognize that, unlike Kymriah treatments, autologous HSCT is not curative for ALL. In a 2014 trial conducted by Maude et al., 63% (n = 30) of patients treated with Kymriah remained in remission during a follow-up period of 24 months.³⁴ As a result of this cost barrier, this treatment is not financially accessible to the general population. This furthers an ongoing economic debate regarding the cost-effectiveness of CAR T-cell therapies, especially due to its novelty compared to conventional cancer treatments.³²
Moreover, the production of CAR T-cells is resource intensive, requiring genetic manipulation, quality control, and final cryopreservation of the expanded T-cell product prior to infusion.¹⁵ Additional equipment, training, and infrastructure are also needed for the administration and post-treatment monitoring of patients. Future efforts should address the implementation in impoverished communities and hospital systems with minimal funding.
CAR T-Cell Relapse There are two types of relapses that occur with CAR T-cell therapy. Negative CAR T-cell relapse occurs due to the loss of the target antigen on the tumor (e.g. CD19), allowing cancer cells to evade CAR T-cells.³⁵ Positive CAR T-cell relapse occurs due to poor persistence and proliferation of CAR T-cells despite the tumor expressing the target antigen.5 With further use of CAR T-cells, relapse has become an apparent obstacle, with up to 50% of patients relapsing within one year.³⁶
In a clinical study, CD19 CAR T-cells were administered to patients with R/R ALL, with 45% of those who achieved complete remission relapsing.³⁷ While positive relapse accounted for 6% of cases, negative relapse was 39%, making it a primary research focus.³⁷ In the case of ALL, potential treatment options include targeting the expression of CD22 on the cancer cells rather than CD19, and developing CAR T-cells with receptors able to target both CD19 and CD22. At this stage, CAR T-cell therapy can be considered effective for inducing complete remission in hematopoietic cancer patients. Given that CRS and ICANS are heightened in patients with higher disease burden and associated comorbidities, CAR T-cell therapy administration should consider the patient’s own treatment needs. Additional research is needed to better understand the mechanisms and management of multiple off-tumor toxicities. Reducing their incidence may be accomplished through alterations to individual CAR T-cell designs. Furthermore, there are a number of handling and economic challenges associated with CAR T-cell therapies. These therapies offer a promising future for those afflicted with R/R ALL and lymphomas, but its cost and patient safety concerns restrict its implementation in clinical settings.
REVIEWED BY: DR. JONATHAN BRAMSON
Dr. Jonathan Bramson is a Professor in the Department of Pathology and Molecular Medicine as well as the Vice Dean of Research in the Faculty of Health Sciences at McMaster University. His research focuses on the development of immunological strategies that target cancer, using methods such as synthetic biology to direct T-cells against tumor targets.
EDITED BY: HANNAH SILVERMAN & JERRY DU
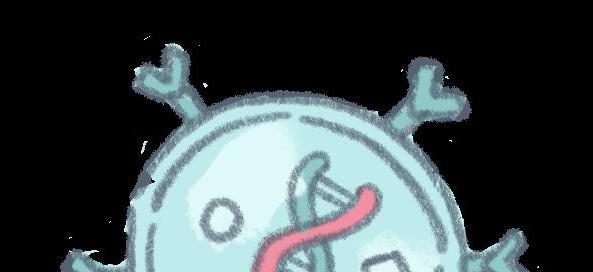
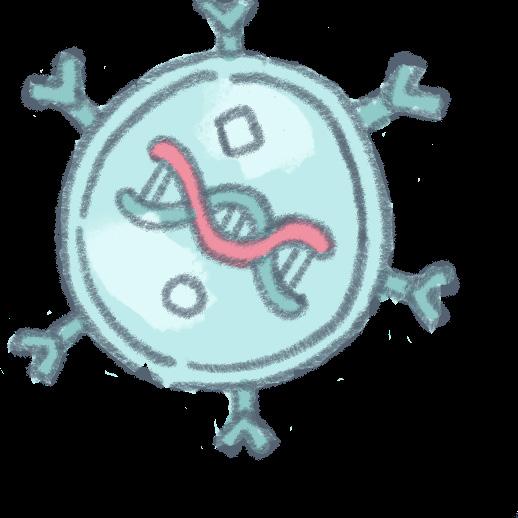
1. Styczynski, J. A brief history of CAR-T cells: From laboratory to the bedside. Acta Haematol Pol. 2020;51(1):2-5. Available from: doi:10.2478/ahp-2020-0002. 2. Mohanty R, Chowdhury CR, Arega S, Sen P, Ganguly P, Ganguly N. CAR T cell therapy: A new era for cancer treatment (Review). Oncol Rep. 2019;42(6):2183-95. Available from: doi:10.3892/ or.2019.7335. 3. Simon B, Harrer DC, Schuler-Thurner B, Schuler G, Uslu U. Arming T cells with a gp100-specific TCR and a CSPG4-specific CAR using combined DNA-and RNA-based receptor transfer. Cancers (Basel). 2019;11(5):2-13. Available from: doi:10.3390/cancers11050696. 4. Benmebarek M, Karches C, Cadilha BL, Lesch S, Endres S, Kobold S. Killing mechanisms of chimeric antigen receptor (CAR) T cells. Int J Mol Sci. 2019;20(1283):1-21. Available from: doi:10.3390/ ijms20061283. Virchow R, Rather LJ. Disease, life, and man. Stanford:Stanford University Press; 1958. 5. Guedan S, Calderon H, Posey AD Jr., Maus MV. Engineering and design of chimeric antigen receptors. Mol Ther Methods Clin Dev. 2019;12:145-52. Available from: doi:10.1016/j.omtm.2018.12.009. 6. Schuster SJ, Bishop MR, Tam CS, Waller EK, Borchmann P, McGuirk JP, et al. Tisagenlecleucel in adult relapsed or refractory diffuse large B-cell lymphoma. N Engl J Med. 2019;380(1):45-56. Available from: doi:10.1056/NEJMoa1804980. 7. Neelapu SS, Locke FL, Bartlett NL, Lekakis LJ, Miklos DB, Jacobson CA, et al. Axicabtagene ciloleucel CAR T cell therapy in refractory large B-cell lymphoma. N Engl J Med. 2017;377(26):2531-44. Available from: doi:10.1056/NEJMoa1707447. 8. Emens LA. Chemotherapy and tumor immunity: An unexpected collaboration. Front Biosci. 2008;13:249-57. Available from: doi:10.2741/2675. 9. Darzynkiewicz Z. Novel strategies of protecting non-cancer cells during chemotherapy: Are they ready for clinical testing? Oncotarget. 2011;2(3):107-8. Available from: doi:10.18632/ oncotarget.249. 10. Hay AK, Turtle CJ. Chimeric antigen receptor (CAR) T cells: Lessons learned from targeting of CD19 in B cell malignancies. Drugs. 2017;77(3):237-45. Available from: doi:10.1007/s40265-0170690-8. 11. Rafiq S, Hackett CS, Brentjens RJ. Engineering strategies to overcome the current roadblocks in CAR T-cell therapy. Nat Rev Clin Oncol. 2020;17(3):147-67. Available from: doi:10.1038/ s41571-019-0297-y. 12. Wang K, Wei G, Liu D. CD19: A biomarker for B cell development, lymphoma diagnosis and therapy. Exp Hematol Oncol. 2012;1(36):1-7. Available from: doi:10.1186/2162-3619-1-36. 13. Liu X, Jiang S, Fang C, Yang S, Olalere D, Pequignot EC, et al. Affinity-tuned ErbB2 or EGFR chimeric antigen receptor T cells exhibit an increased therapeutic index against tumors in mice. Cancer Res. 2015;75(17):3596-607. Available from: doi:10.1158/0008-5472.CAN-15-0159. 14. Zhao Z, Condomines M, Van der Stegen SJC, Gunset G, Plotkin J, Sadelain M. Structural design of engineered costimulation determines tumor rejection kinetics and persistence of CAR T cells. Cancer Cell. 2015;28(4):415-28. Available from: doi:10.1016/j.ccell.2015.09.004. 15. Santomasso B, Bachier C, Westin J, Rezvani K, Shpall EJ. The other side of CAR T-Cell therapy: Cytokine release syndrome, neurologic toxicity, and financial burden. American Society of Clinical Oncology Educational Book. 2019;39:433-44. Available from: doi:10.1200/EDBK_238691. 16. McLellan AD, Ali Hosseini Rad SM. Chimeric antigen receptor T cell persistence and memory cell formation. Immunol Cell Biol. 2019;97(7):664-74. Available from: doi:10.1111/imcb.12254. 17. Nie Y, Lu W, Chen D, Tu H, Guo Z, Zhou X, et al. Mechanisms underlying CD19-positive ALL relapse after anti-CD19 CAR T cell therapy and associated strategies. Biomark Res. 2020;8(18):1-17. Available from: doi:10.1186/s40364-020-00197-1. 18. Spear TT, Evavold BD, Baker BM, Nishimura MI. Understanding TCR affinity, antigen specificity, and cross-reactivity to improve TCR gene-modified T cells for cancer immunotherapy. Cancer Immunol Immunother. 2019;68(11):1881-9. Available from: doi:10.1007/s00262-019-02401-0. 19. Zhang JM, An J. Cytokines, inflammation, and pain. Int Anesthesiol Clin. 2007;45(2):27-37. Available from: doi:10.1097/AIA.0b013e318034194e. 20. Murthy H, Iqbal M, Chavez JC, Kharfan-Dabaja MA. Cytokine release syndrome: Current perspectives. Immunotargets Ther. 2019;8:43-52. Available from: doi:10.2147/ITT.S202015. 21. Hay KA, Hanafi L-A, Li D, Gust J, Liles WC, Wurfel MM, et al. Kinetics and biomarkers of severe cytokine release syndrome after CD19 chimeric antigen receptor-modified T-cell therapy. Blood. 2017;130(21):2295-306. Available from: doi:10.1182/blood-2017-06-793141. 22. Wang Z, Han W. Biomarkers of cytokine release syndrome and neurotoxicity related to CAR-T cell therapy. Biomark Res. 2018;6(4). Available from: doi:10.1186/s40364-018-0116-0. 23. Cao J, Wang H, Gao W, You J, Wu L, Wang Z. The incidence of cytokine release syndrome and neurotoxicity of CD19 chimeric antigen receptor–T cell therapy in the patient with acute lymphoblastic leukemia and lymphoma. Cytotherapy. 2020;22(4):214-26. Available from: doi:10.1016/j.jcyt.2020.01.015. 24. Sheth VS, Gauthier J. Taming the beast: CRS and ICANS after CAR T-cell therapy for ALL. Bone Marrow Transplant. 2020. Available from: doi:10.1038/s41409-020-01134-4. 25. Neelapu SS. Managing the toxicities of CAR T-cell therapy. Hematol Oncol. 2019;37(1):48-52. Available from: doi:10.1002/hon.2595. 26. Rice J, Nagle S, Randall J, Hinson HE. Chimeric antigen receptor T cell-related neurotoxicity: Mechanisms, clinical presentation, and approach to treatment. Curr Treat Options Neurol. 2019;21(8):40. Available from: doi:10.1007/s11940-019-0580-3. 27. Gust J, Hay KA, Hanafi LA, Li D, Myerson D, Gonzalez-Cuyar LF, et al. Endothelial activation and blood-brain barrier disruption in neurotoxicity after adoptive immunotherapy with CD19 CAR-T cells. Cancer Discov. 2017;7(12):1404-19. Available from: doi:10.1158/2159-8290.CD-17-0698. 28. Maude SL, Laetsch TW, Buechner J, Rives S, Boyer M, Bittencourt H, et al. Tisagenlecleucel in children and young adults with B-cell lymphoblastic leukemia. N Engl J Med. 2018;378(5):439-48. Available from: doi:10.1056/NEJMoa1709866. 29. Obermeier B, Daneman R, Ransohoff RM. Development, maintenance and disruption of the bloodbrain barrier. Nat Med. 2013;19(12):1584-96. Available from: doi:10.1038/nm.3407. 30. Parker KR, Migliorini D, Perkey E, Yost KE, Bhaduri A, Bagga P, et al. Single-cell analyses identify brain mural cells expressing CD19 as potential off-tumor targets for CAR-T immunotherapies. Cell. 2020;183(1):126-42. Available from: doi:10.1016/j.cell.2020.08.022. 31. Gust J, Taraseviciute A, Turtle CJ. Neurotoxicity associated with CD19-targeted CAR-T cell therapies. CNS Drugs. 2018;32(12):1091-101. Available from: doi:10.1007/s40263-018-0582-9. 32. Siegler EL, Kenderian SS. Neurotoxicity and cytokine release syndrome after chimeric antigen receptor T cell therapy: Insights into mechanisms and novel therapies. Front Immunol. 2020;11(1973):1-8. Available from: doi:10.3389/fimmu.2020.01973. 33. Hay A, Cheung M. CAR T-cells: costs, comparisons, and commentary. J Med Econ. 2019;22(7):61315. Available from: doi:10.1080/13696998.2019.1582059. 34. Maziarz RT, Guérin A, Gauthier G, Heroux J, Zhdanava M, Wu EQ, et al. Five-year direct costs of acute lymphoblastic leukemia pediatric patients undergoing allogeneic stem cell transplant. Int J Hematol Oncol. 2016;5(2):63-75. Available from: doi:10.2217/ijh-2016-0001. 35. Maude SL, Frey N, Shaw PA, Aplenc R, Barett DM, Bunin NJ, et al. Chimeric antigen receptor T cells for sustained remissions in leukemia. N Engl J Med. 2014;371:1507-17. Available from: doi:10.1056/NEJMoa1407222. 36. Xu X, Sun Q, Liang X, Chen Z, Zhang X, Zhou X, et al. Mechanisms of relapse after CD19 CAR T-Cell therapy for acute lymphoblastic leukemia and its prevention and treatment strategies. Front Immunol. 2019;10(2664):1-15. Available from: doi:10.3389/fimmu.2019.02664. 37. Ma S, Li X, Wang X, Cheng L, Li Z, Zhang C, et al. Current progress in CAR-T cell therapy for solid tumors. Int J Biol Sci. 2019;15(12):2548-60. Available from: doi:10.7150/ijbs.34213. 38. Gardner RA, Finney O, Annesley C, Brakke H, Summers C, Leger K, et al. Intent-to-treat leukemia remission by CD19 CAR T cells of defined formulation and dose in children and young adults. Blood. 2017;129(25):3322-31. Available from: doi:10.1182/blood-2017-02-769208.