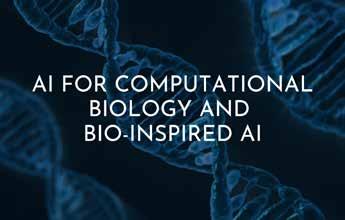
6 minute read
AI for computational biology and bio-inspired AI
Research activity of Axis 3 during the period 2019-2021
Research topics
Axis 3 aims at quantifying biological structures in space and time from new-generation data at all scales (molecules, cells, tissues, organs) in order to understand and model biological phenomena. Data are heterogeneous, massive, high-dimensional, and embed complex correlations. Novel AI technologies are required to characterize normal and pathological biological functions and to understand the adaptation of organisms to their changing environment (computational biology). Conversely, insights from cognition offer exciting perspectives to propose new bio-inspired AI algorithms and architectures (bio-inspired AI).
3IA Chairs
A total of 8 researchers have contributed to Axis 3: • 4 Chairs since Oct. 2019 (L. Blanc-Féraud, F. Cazals, P. Reynaud-Bouret, E. Van Obberghen-Schilling) • 2 Chairs since Sept. 1, 2021 (P. Barbry, B. Miramond) • 1 International Chair (D. Wales) • 1 Affiliate Chair (D. Rouquié) since 2020
International chair - since 2019
David Wales - University of Cambridge
Solution landscapes for Machine Learning
We explore machine learning landscapes in the cost function parameter space, which isanalogous to the potential energy surface of a molecule as a function of atomic coordinates. Ongoing advances in methodology developed in chemical physics, can therefore be immediately applied to ML solution landscapes. Our objectives are to use these tools to design improved predictions, and apply them to problems in molecular science and health care. In particular, we seek improved machine learning tools for clinician diagnostic support, to provide earlier detection of the deteriorating (and improving) patient. Specific applications include prediction of readmission to intensive care, which represent a failure in down-transfer to the ward, and are often associated with patient mortality.
Chairs 2019
Laure Blanc-Féraud - CNRS
Imaging for biology Recent advances in microscope technology provide outstanding images that allow biologists to address fundamental questions. This project aims at developing new AI methods and algorithms for (i) novel acquisition setups for super resolution imaging, and (ii) extraction of valuable quantitative information from these large heterogeneous datasets.
Frédéric Cazals - Inria
AIMS: Artificial intelligence for molecular studies
By learning essential features of proteins and their complexes, we shall deliver biologically relevant information for large molecular systems on biologically relevant time scales, leveraging our understanding of biological functions at the atomic level, and providing key inputs for protein design and engineering, and protein interaction networks.
Patricia Reynaud-Bouret - CNRS
MEL: Modeling and estimating learning
We are defining new probabilistic models and new estimation methods to understand the deformation of functional connectivity during learning in in vivo experiments.
Ellen Van Obberghen-Schilling - Inserm
AI-powered analysis of the tumor microenvironment
Our project will integrate tissue imaging modalities and artificial intelligence-based analysis tools for a deeper understanding and control of cancer, targeting tumor microenvironment and on the role of the extracellular matrix (ECM) in carcinoma progression, spread and response to therapy.
Chairs 2020
Pascal Barbry - CNRS
Human Lung Atlas
The project elaborates on state-of-the-art approaches in genomics and cell biology to describe complex biological samples at the single-cell resolution. Multidimensional biological experiments result in large scale descriptions of DNA, RNA and protein expressions that can be integrated in time and space. The project aims at: (1) developing novel data-mining approaches based on machine learning and AI; (2) apply them to the study of the normal and pathological lung, in the context of serious threats that touch this organ (COVID-19, asthma, cystic fibrosis, cancer,...).
Benoît Miramond - Université Côte d'Azur
Bio inspired AI from neurosciences to embedded autonomous devices
The research project seeks to draw on the structure and function of the biological brain to develop more energy-efficient AI methods and algorithms. The scientific approach ranges from neural dynamics to the emerging cognitive properties of these networks and ultimately to the design of embedded neuromorphic electronic circuits. The project will focus on building bridges between the NeuroMod neuroscience institute and the 3IA Cote d'Azur institute.
Affiliate Chair 2020
David Rouquié - Université Côte d'Azur | BAYER
Human health chemical risk assessments
The main focus of my research activity as affiliate chair at 3IA Côte d’Azur is on the emerging theme of chemical safety by design. This theme derives from all the on-going initiatives to improve the characterization of the risk of chemicals to humans by using more data from non-animal technologies and goes far beyond. Indeed, we are living a paradigm shift in the way bioactive small molecules are discovered but also de-risked. Instead of relying on numerous, long, costly cycles of trials and errors, thanks to the advance in systems biology and state-of-the-art machine learning algorithms it is possible to proactively drive de novo chemical design with high probability to induce specific biological responses. For the first time, we have shown as proof of concept that a learning procedure can automatically design molecules that have a high probability to induce a desired transcriptomic profile in cell lines. In my position of affiliate chair at 3IA Côte d’Azur, this approach will be further developed by building the pillars necessary to guide chemical design toward optimized safety profiles while maintaining the desired biological effect of the compounds.
Actions and highlights
• Single cell: Characterization of the individual cells of lungs and airways contributes to research on the
SARS-CoV-2 virus and the Human Cell Atlas. New methods are proposed to analyze the structure of
RNAs at single-cell resolution and to obtain the spatial transcriptomic data needed to detail the mutational landscape in tumors (Chair: P. Barbry). • Molecular studies: The essential features of proteins discovered by statistical analysis or by combining sequence-based and structural data analyses can reveal biological functions at the atomic level. This contributes to a better understanding of interfaces of proteins within complexes and their dynamics, and provides key information about protein design and engineering (Chair: F. Cazals). Theoretical results have also been obtained for the characterization of the energy landscape used to support the clinician’s early diagnosis using electronic patient health data (Chair: D. Wales). Also, chemical-safe molecules with the desired biological responses are designed from transcriptomic and cell-painting data using GAN (Chair: D. Rouquié, Bayer). • Super-resolution microscopy and extracellular matrix: This research exploits the recent results obtained in sparse optimization and combines them with the relevant acquisition protocols to obtain 2D and 3D super-resolution optical microscopy images of living cells, allowing the study of fine structures in and outside the cells (Chair: L. Blanc-Féraud). Also, structural biomarkers extracted from ECM network images in vitro of the tumor microenvironment have provided useful information for the study of tumor development, prognosis and response to treatment, in addition to standard cell analyses (Chair: E. Van
Obberghen-Schilling). • Bio-inspired AI: This topic is studied both from a behavioral and neuronal point of view. It states complex theoretical questions using spike sorting, simulation of Hawkes processes, mean-field limits or reconstruction of functional connectivity. This last method has been proven to be able to follow the mnesic trace and investigate where a memory is encoded (Chair: P. Bouret). Furthermore, the development of a spike neural network architecture or a self-organizing neural architecture inspired by the brain leads to low-power classification algorithms (Chair: B. Miramond). Realizations are embedded in autonomous devices, such as the VS23 Soyuz space flight or in smart glasses.