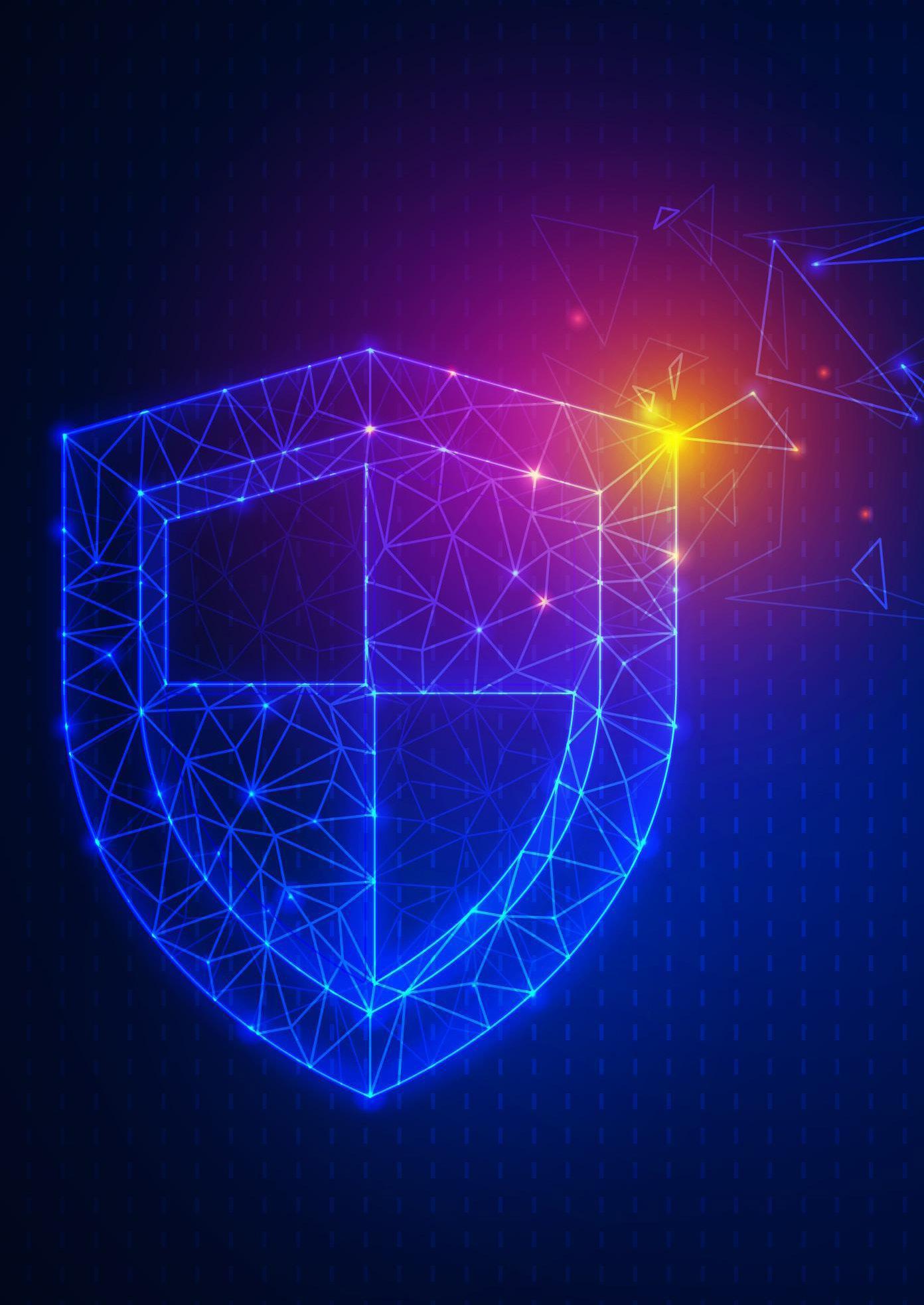
10 minute read
Convergence of cybersecurity and big data science
Prof Jan Eloff Johan Smit The Cybersecurity Framework of the NIST was developed in the USA with the aim of assisting companies to understand the scope of cybersecurity and to minimise risk exposure. It consists of five functions that explain the convergence benefits of cybersecurity and big data science: Identify, Protect, Detect, Respond and Recover.
The successful convergence of cybersecurity and big data science necessitates a clear understanding of big data, data science and cybersecurity. A global survey by the international Ponemon Institute, in conjunction with IBM, found that companies that leveraged the convergence of cybersecurity and big data science dramatically improved their overall cyber and information security posture.
Research conducted by the Cybersecurity and Big Data Science Research Group at the University of Pretoria examined the Cybersecurity Framework of the National Institute of Standards and Technology (NIST) to obtain an understanding of the convergence benefits of cybersecurity and big data science. This provided the foundation for several projects aimed at improving detection mechanisms by leveraging these convergence benefits.
DESCRIBING BIG DATA
The volume of data is one way to describe big data – and there is much more data today than ever before. On Twitter alone, over 500 million tweets are sent per day and mobile traffic is expected to grow from 11.5 exabytes per month in 2017 to 77 exabytes by 2022. However, big data is defined by more than just the volume of data. It is also described in terms of variety (whether the data is structured or unstructured) and velocity (the speed of data flow and how fast the data is created and moved).
One of the most important components of data science is machine learning. This is a field that spans disciplines such as computer science, statistics, mathematics, psychology and brain sciences. Combining machine learning with big data is a powerful development and forms
THE CYBERSECURITY FRAMEWORK
forensics
RECOVER
faster response prediction
RESPOND
the basis for the convergence of cybersecurity and big data science.
big data technologies big data analysis big data visualisation machine learning models automation user behaviour models
IDENTIFY
PROTECT
improve existing tools data protection prediction
DETECT
big data analysis big data visualisation attack detection
As the first function of the Cybersecurity Framework, Identify focuses on identifying risks and the security areas in the organisation that require priority focus. This is done by building an understanding and a baseline of the organisation and then working through the combined data. In the Identify function, the convergence of cybersecurity and big data science mainly focuses on big data techniques, including the benefit to be gained from using big data analysis and big data visualisation. Big data analysis can assist with creating correlations between various data sources, which can assist in digital forensic investigations. Data visualisation, on the other hand, can enable a better grasp on and a faster grasp of the data. Machine learning models can create a baseline for the organisation, and create user behaviour baselines as this has the potential to improve the discovery and detection of cybersecurity attacks and compromises. User behaviour baselines are valuable from a cybersecurity perspective since humans are often the weakest link in the cyber defence chain. Machine learning can also help define the characteristics of an existing system to identify or predict where a future attack can occur.
Protect
The Protect function refers to the safeguarding of computer networks and the information they contain. The focus is to limit cyber attacks and their impact, should an attack occur. Basic forms of protection include anti-virus programs and firewalls. However, these are reactive protection strategies, and more proactive strategies are required against newer, more advanced attacks. The convergence between cybersecurity and big data science can be leveraged to develop smarter firewalls that implement near real-time internet protocol (IP) updates to block or terminate malicious connections. State-of theart security and information event management technologies that employ big data and machine learning enable a user to view combined datasets, discover advanced attacks and predict where an attacker could try to infiltrate the cyber environment.
Detect
The Detect function focuses on detecting a cybersecurity event by monitoring and detecting anomalies, and detecting specific events. This is the function of the Cybersecurity Framework that creates the best opportunities to leverage advances in cybersecurity and big data science. One of the reasons for this is that detection is usually based on a quick understanding and interpretation of a huge amount of data, and quickly finding patterns and outliers in the data to assist with detecting anomalies. The convergence of cybersecurity and big data science can help improve the quality of cyber attack discovery. One way of implementing intrusion detection is through the use of an intrusion detection system that is enhanced with machine learning and a variety of machine learning algorithms. Regression algorithms can be used to predict what the next system call should be and to compare that result with what system call is really happening. In this way, anomalies can be detected, which could mean the detection of an attempted hack.
Respond
The Respond function refers to what needs to happen after a cybersecurity incident has been detected. In addition to defining the activities and actions that are required, this function is also concerned with limiting the impact of the potential incident. The Ponemon Institute found that having a tried-andtested response plan enables better attack prevention and reduces the cost of a breach. Adding intelligent automated responses through the convergence of cybersecurity and big data science can help manage constantly evolving attacks. Close to real-time decision making can be achieved with big data analysis. This can provide a fast response that will protect the organisation. Other decision-making options are to create streamlined processes to quarantine malware or to revoke IP access from attackers. Classifying an attack can also assist in improving response since this provides a guide to what could potentially be the next logical steps of the attack. Unsupervised learning in the form of selforganising maps have also been used to identify the attack class.
Recover
The Recover function refers to the plans, efforts and resources required to restore capabilities and services to a working state after being affected in a cyber attack. The intent is to have everything up and running as soon as possible through the proper planning and use of resources. One benefit is to employ unsupervised models to support forensic investigators when they determine what is normal and what is anomalous behaviour. These models allow the investigator to obtain a faster overview of what happened. A more formal approach was proposed by a research team supervised by Prof Hein Venter of the Department of Computer Science at the University of Pretoria (Karie, Kebande and Venter, 2019), who created a Deep Learning Cyber Forensics Framework to assist cyber investigators. This framework makes use of deep learning techniques to create a cyber forensic investigation engine, which assists in collecting, preserving, analysing and interpreting potential evidence.
RESEARCH TO IMPROVE DETECTION MECHANISMS
The Cybersecurity and Big Data Science Research Group has conducted several projects to improve detection mechanisms by leveraging the benefits of the convergence between cybersecurity and big data science.
References
Joubert, D. and Eloff, J.H.P., 2020. Cybersecurity – discovering insider threats in vehicle-tracking systems (in preparation for publication).
Karie, N., Kebande, V. and Venter, H., 2019. Diverging deep learning cognitive computing techniques into cyber forensics, Forensic Science International: Synergy 1(4).
Michael A. and Eloff J.H.P., 2020. Discovering “Insider IT Sabotage” based on human behaviour, Information and Computer Security. https://doi.org/10.1108/ICS-12-2019-0141 URL: https://www.emerald. com/insight/content/doi/10.1108/ICS-12-2019-0141/full/html.
National Institute of Standards and Technology. 2018. Framework for improving critical infrastructure cybersecurity, version 1.1. https://nvlpubs.nist.gov/nistpubs/CSWP/NIST.CSWP.04162018.pdf.
Ngejane, C.J., Sefara, J.T., Eloff, J.H.P. and Marivate, V., 2019. A digital forensic and machine learning approach for discovering behavioural patterns of online sexual predators (under revision for publication).
Ponemon Institute, 2019. Cost of a Data Breach Report 2019. https://www.ibm.com/security/databreach.
Van der Walt, E. and Eloff, J.H.P., 2018. Cybersecurity: Identity deception detection on social media platforms, Computers and Security 78. https://doi.org/10.1016/j.cose.2018.05.015.
Insider threats exploit system vulnerabilities from inside an organisation, such as employees who abuse their authorised access rights to cause harm to an organisation. The number and complexity of insider threats outpace currently available cybersecurity safeguards. In order to discover insider threats, a deep understanding of the application domain within the organisation, as well as processing big and fast-changing volumes of data, is required. In an attempt to minimise cybersecurity insider threats, rule-based approaches are used. The discovery of insider threats is an imprecise and complex problem, and these rulebased approaches only focus on known abuse scenarios. The sheer volume, velocity and variety of the big data generated by highly integrated systems today also make it impossible to discover abuse committed by insiders without using specialised tools. The advances made in big data and data science, such as anomaly detection algorithms, can be leveraged to develop specialised tools for the intelligent discovery of insider threats.
One project conducted to detect insider threats focused on disgruntled employees. These individuals utilise IT infrastructures such as email to execute malicious activities. The events leading up to the attack, triggered by the insider, are often found to be behavioural, rather than technical. One way of observing the behaviour of employees is to investigate their email communications. The problem is that, due to the high volume, velocity and complexity of emails, the risk of insider threats cannot be diminished with the rule-based approaches that are currently available to detect the harmful behaviour of employees. This project aimed to proactively detect fraudulent activities by leveraging the advantages of cybersecurity and big data science.
Another project focused on the use of anomaly detection algorithms to detect insider threats. Data was obtained from a motor vehicle tracking company with approximately 700 000 vehicle tracking devices that report to the company’s server infrastructure in near real-time. The data contained information such as location, and accelerometer and engine values. This resulted in large volumes of vehicle tracking data being obtained at a rate of between 1 000 and 4 000 data blocks per second. These volume and velocity characteristics of the real-life vehicle tracking big data were considered to be important aspects of the experimental work reported on in this project. Through machine learning models, the experimental results revealed that anomaly detection is a valid approach to detect insider threats. Identity deception is a major problem on social media platforms today. Think about cyber threats such as cyber bullying, cyber masquerading and the way sexual predators operate on the internet. In most threats of this kind, users are not honest about their identities. A project to investigate this phenomenon sought to develop solutions for the detection of fake identities created on social media platforms. Machine learning models developed for this project used attributes and features based on user account details. These attributes and features were extended with concepts borrowed from the field of psychology, such as the fact that humans lie about their age. Newly engineered features, such as gender derived from the profile image, were evaluated to grasp whether these features detect deception with greater accuracy. These machine learning results were applied to a model for the intelligent detection and interpretation of identity deception on social media platforms. This project shows that the cybersecurity threat of identity deception can potentially be minimised if the vulnerability in the current way of setting up user accounts on social media platforms can be re-engineered.
The detection of sexual predators on the internet
Social media chat logs can be used to analyse harmful behaviour as they can be used to detect harmful behaviour, such as paedophilia. This can make an important contribution to the cyber safety of children, preventing them from being exploited by online predators. The challenge is that digital forensic investigators are expected to collect evidence from chat logs, which is a daunting task because of the sheer volume and variety of data. A project to investigate this phenomenon suggested employing a Digital Forensic Process Model that is supported by machine learning methods to facilitate the automatic discovery of harmful conversations in chat logs. It also indicated how the tasks in a digital forensic investigation process can be organised to obtain usable machine learning results when investigating online predators.
Conclusion
There is a clear convergence between cybersecurity, big data and machine learning, and great value can be achieved from this convergence. Cybersecurity is the greatest beneficiary. Big data analysis and big data visualisation are used in various functions of cybersecurity to enable better use of and value extraction from the new big data datasets. These new datasets are a treasure for attackers, and cybersecurity principles can help protect them. Machine learning is used in a number of the listed functions of the Cybersecurity Framework, but the biggest benefit is achieved by improving the different types of detection mechanisms, which can range from insider threat detection to detecting sexual predators on the internet. However, these same techniques and tools are also available to the cyber attackers, who are improving their attacks to make use of new advancements.