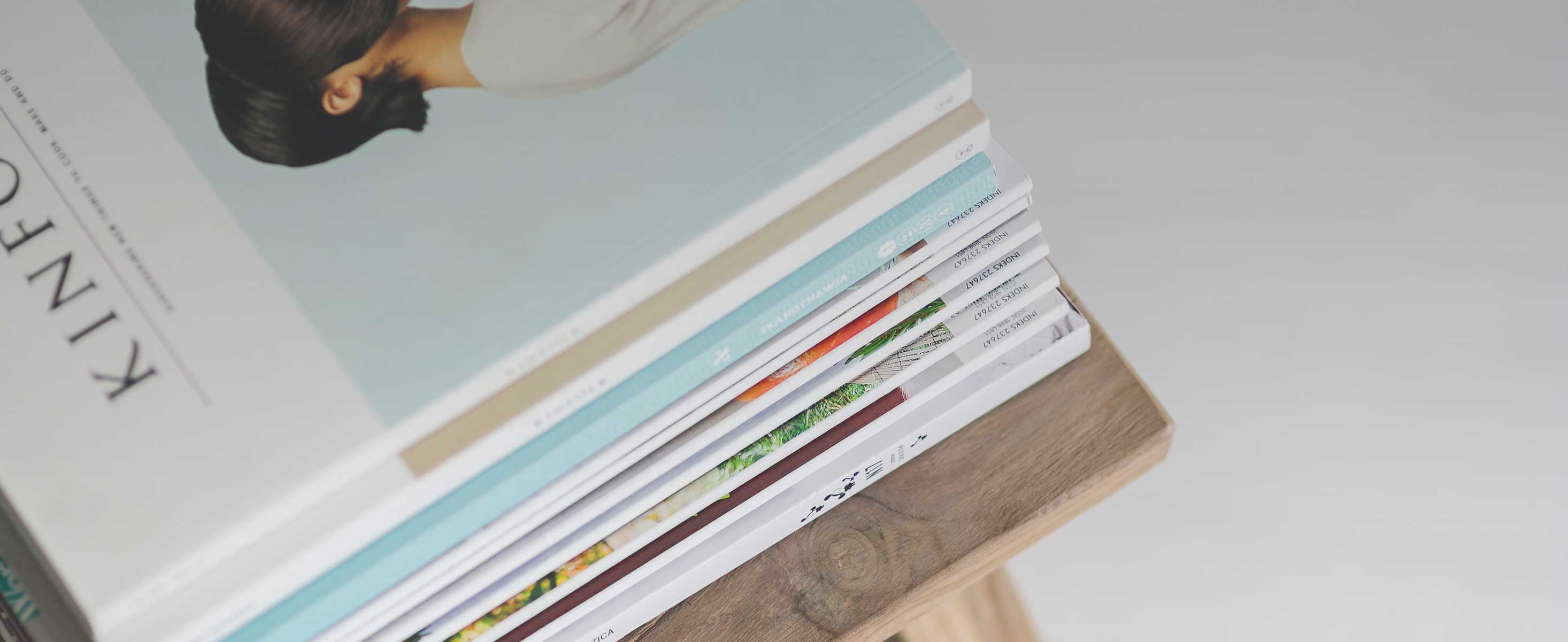
1 minute read
Fair AI Speech
New research at USC demonstrates how to train a popular language model to remove homophobic, anti-queer bias.
Modern text prediction isn’t perfect — but it can also be highly biased when predicting results related to marginalized communities.
A team of researchers from the USC Viterbi School of Engineering Information Sciences Institute and the USC Annenberg School for Communication and Journalism, led by Katy Felkner, a USC Viterbi PhD student in computer science and National Science Foundation Graduate Research Fellowship recipient, has developed a system to quantify and fix anti-queer bias in the artificial intelligence behind text prediction.
The project looks at detecting and reducing anti-queer bias in a large language model, or LLM — the “brain” behind the text prediction. LLM is an artificial intelligence
As a scholar and public intellectual with a doctorate from NYU, King has created multidisciplinary work in the fields of African American and African Diasporic cultural studies; performance studies; globalization and transnationalism studies; media and technology studies; music business, marketing and branding studies; and gender and queer studies.
An inaugural member of the Hip Hop Culture Council at the John F. Kennedy Center for the Performing Arts and the Black Genius Brain Trust, King serves on the editorial board of the Journal of Popular Music Studies. He brings a long history of publications as a scholar and a journalist, and extensive experience working with internationally known media outlets on series, podcasts and documentaries.
King acknowledges the sense of exuberance and commitment to excellence he felt from USC Thornton faculty, staff and that “completes” sentences by predicting the most likely string of words that follows a given prompt.
“Most LLMs are trained on huge amounts of data that’s crawled from the internet,” Felkner says. “They’re going to pick up every kind of social bias that you can imagine is out there on the web.”
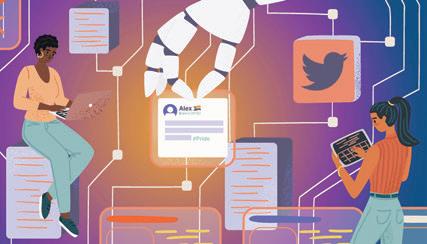
The project found that a popular LLM called BERT (Bidirectional Encoder Representations from Transformers) showed significant homophobic bias, which can then enforce users’ biases. To tackle this problem, Felkner gave BERT a tuneup by students. “Some things are very consistent about music transcendent music is still the goal,” he says. “And for any student at USC Thornton who’s focusing on making or researching music, my goal is to help them achieve that artistic and scholarly transcendence.”
TED B. KISSELL
feeding it tweets and news articles containing LGBTQ+ keywords from two separate databases of Felkner’s creation: QueerTwitter and QueerNews.
As the model was exposed to different perspectives and communities, it became more familiar with queer language and issues. After being trained with the new, more inclusive data, the model showed significantly less bias.
Long term, Felkner hopes the project can be used to train other LLMs, help researchers test the fairness of their natural language processing or even uncover completely new biases.
LILLIAN GOODWIN