July 19, 2023
The authors, Ning et al, have proposed what they refer to as a “broad class” of “so called” CoxAalen transformation models which have elements of both multiplicative and additive covariate effects on the baseline hazard function contained within the transformation. Originally, the Cox proporiotnal hazards regression model and the Aalen additive model were separate, but over the years, an attempt to incorporate the models has been studied, hence the Cox-Aalen model. In terms of the transformation models, the authors refer speciically to Zeng and Lin’s model (2006) to avoid confusion which they used a non-parametric maximum likelihood estimator in presence of right censoring and developed a system of equations for jumps of the baseline cumulative hazard function at exact failure times through an EM algorithm. Their approach though assumed the covariate effects were multiplicative. In addition, though EM-type algorithms existed for finding the NPMLE of semiparamtric transformation models, Elashoff ad Ryan (2004) propsed an expectation-solving (ES) algorithm to handle missing data for general estimating equations.
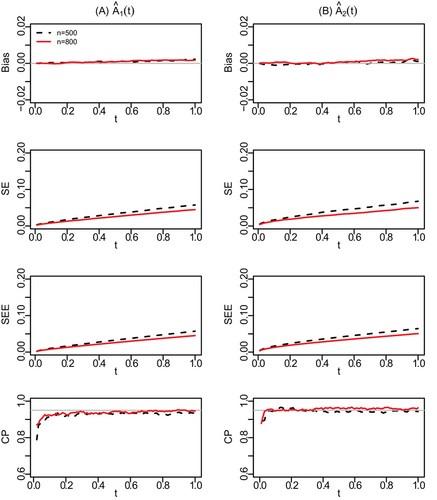
The proposed method by the authors incorporates both the Cox-Aalen method and the transformation method with the ES algorithm, which is a like a block Newton-Gauss-Seidel algorithm which they refer to as the semi-paramtric Cox-Aalen transformation models. They stated that their proposed class of semi-paramtric models are very flexible and contain many models used often in survival anlaysis. Using their ES algorithm, they essentially estimated parameters by treating parameters as missing. This algorithm iterated between an E-step where the complete data are replaced by their expectatons and and S-step where the expected values are then substituted into the complete data estimating equations, and these equations are then solved. They had said this algorithm avoided solving a large high dimensional array of parameters and the betas are obtained from the Newton-Raphson method.
According to their simulations, their method performed well and also on in a real dataset. In their discussion, they do not suggest any issues with their model performance but only suggest future research should incorporate model misspecificiation. In review of this method, the authors have definitely combined a Cox-Aalen type method for multiplicative and also for additive covariate effect and also employed EM type algorithms for estimation of parameters to avoid high dimesionality. What the authors had not discussed were the computational issues of their method, whether or not their method is available in a function, and under what situations to best use their method. Essentially, no guidance for users was provided which makes thinking of the use of this method difficult in applications.
Written by, Usha GovindarajuluKeywords: survival, Cox, Aalen, transformation, NPMLE, EM algorithm
References
Elashoff, M. & Ryan, L. (2004) An EM algorithm for estimating equations Journal of Computational and Graphical Statistics, 13(1), 48– 65.
Ning X, Pan Y, Sun Y, and Gilbert PB (2023). “A semi-parametric Cox-Aalen transformation model with censored data” Biometrics, https://doi.org/10.1111/biom.13895
Zeng, D. & Lin, D. (2006) Efficient estimation of semiparametric transformation models for counting processes. Biometrika, 93(3), 627– 640.
https://onlinelibrary.wiley.com/cms/asset/74d8f918-3a1e-4f99-8c9b-e7a71dc640cd/biom13895fig-0001-m.jpg