Statistical issues in survival analysis (Causal mediation additive hazards)
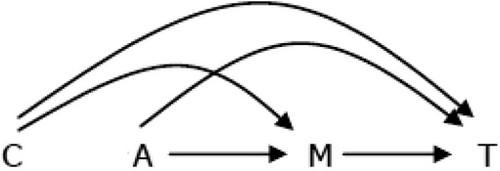
February 12, 2025
The authors focused on causal mediation analysis in survival analysis, more specifically with additive hazards modeling with an exposure by mediator interaction term. The causal mediation framework had come about over time from the mediation area and then was also proposed for survival analysis where one of the first was by Lange and Hansen (2011). They suggested measuring the natural direct and indirect effects based on the hazard difference scale. Their estimation method worked under the Aalen additive hazards model, which they said had advantages over the Cox model in both causal interpretation and mathematical consistency. Since then, many other authors proposed methods in this area, but so far, none of the studies considered an exposure-mediator interaction effect when the additive hazards model was used. The authors extended work from Vansteelandt et al (2019) and Aalen et al (2020) to accommodate this interaction under additive hazards modeling.
Mediation analysis has also been shown in other survival models. VandereWeele (2011) presented identification results for causal survival effect under the Cox model and the accelerate failure time model, where his work even allowed for an exposure-mediator interaction on the survival outcome. Ongoing work has been conducted and there has even been work on survival analysis with multiple mediators by multiple authors. One issue is that the prior research in this area typically assumed that the mediator and confounders were precisely measured. It has been well known that measurement error can distort statistical inference. Though methods have been proposed to handle measurement error in survival analysis, some progress has been made in causal mediation by several other authors including VanderWeele (2015). An adjustment method by Cessie et al (2012) and VanderWeele, Valeri, and Ogburn (2012) was developed solely for the linear and logistic models but could not eliminate bias in the setup by Aalen. The authors have compared this method to their proposed method for measurement error correction.
In their setup they make an assumption that there is not unmeasured confounding of the various relationship between mediator and exposure or outcome. They showed their formulas for direct and indirect effects estimation with the incorporation of the exposure by meditator interaction. For their measurement error correction, they allowed covariate effects to be piecewise constant. They then re-estimated the direct and indirect effects with their corrections. They also showed adjusted for measurement error in the mediator and the confounder as well. They also proposed a measurement error correction method for the parameter estimation under the additive hazards
model and the mediator regression model. They do not consider the Aalen additive hazards model since it lacks measurement error correction. They then proposed to estimate the causal effects.
They ran simulation studies in the absence and presence of exposure-mediator interaction in order to evaluate the performance of the direct effect and the indirect effect and the total effect when measurement error exists in the mediator and confounders. They found the corrected method had a much smaller vias compared to the naïve and adjusted methods in estimating direct and indirect effects. In a real dataset analysis, they found that measurement error attenuates the direct effect estimation while it has a negligible impact on inference of the indirect effect. The authors noted that in this study they assumed the mediator was normally distributed. Their future work would use misclassified discrete covariates or nonnormal continuous covariates. They may also consider multiple mediators.
Written by,
Usha Govindarajulu
Keywords: causal mediation, additive hazards, interaction, survival analysis
References:
Aalen, O. O., M. J. Stensrud, V. Didelez, R. Daniel, K. Røysland, and S. Strohmaier 2020 “Time-Dependent Mediators in Survival Analysis: Modeling Direct and Indirect Effects With the Additive Hazards Model.” Biometrical Journal 62: 532–549.
Lange, T., and J. V. Hansen. 2011. “Direct and Indirect Effects in a Survival Context.” Epidemiology 22: 575–581.
le Cessie, S., J. Debeij, F. R. Rosendaal, S. C. Cannegieter, and J. P. Vandenbroucke 2012 “Quantification of Bias in Direct Effects Estimates Due to Different Types of Measurement Error in the Mediator.” Epidemiology 23: 551–560.
VanderWeele, T. J. 2011. “Causal Mediation Analysis With Survival Data.” Epidemiology 22: 582–585.
VanderWeele, T. J. 2015. Explanation in Causal Inference: Methods for Mediation and Interaction New York: Oxford University Press.
VanderWeele, T. J., L. Valeri, and E. L. Ogburn 2012. “The Role of Measurement Error and Misclassification in Mediation Analysis: Mediation and Measurement Error.” Epidemiology 23: 561–564.
Vansteelandt, S., M. Linder, S. Vandenberghe, J. Steen, and J. Madsen. 2019. “Mediation Analysis of Time-To-Event Endpoints Accounting for Repeatedly Measured Mediators Subject to Time-Varying Confounding.” Statistics in Medicine 38: 4828–4840.
Yan, Y and Shen L (2025) “Mediation Analysis With Exposure-Mediator Interaction and Covariate Measurement Error Under the Additive Hazards Model” Biometrical Journal. https://doi.org/10.1002/bimj.70035
https://onlinelibrary.wiley.com/cms/asset/94f9da65-2244-4e3a-8919-8ca899bcd938/bimj70035fig-0001-m.png