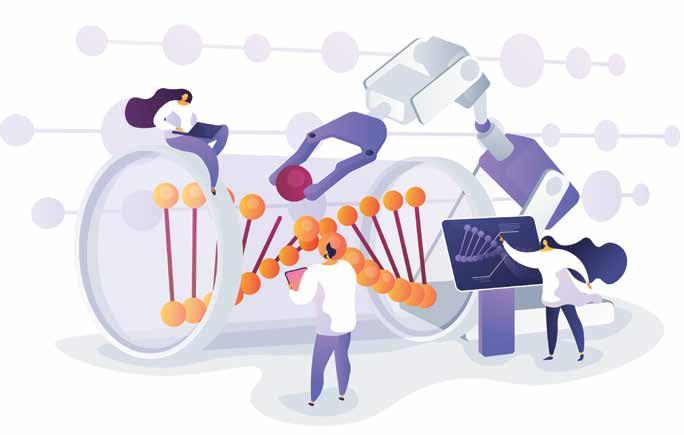
6 minute read
Design and Analysis of Cancer Clinical Trials for Personalised Medicine
Cancer clinical trials for personalised medicine should be appropriately designed and analysed reflecting the various factors. Biomarkers play a key role in the development of personalised medicine. In this article, we review the design and analysis of two phase II cancer clinical trials of personalised medicine, one with a predictive biomarker and the other with a prognostic biomarker. We discuss statistical testing method and its sample size calculation method for each of the trials.
Sin-Ho Jung, Department of Biostatistics and Bioinformatics Duke University School of Medicine
Advertisement
Personalised medicine for caner is a treatment module tailored to individual patients based on various factors including biomarkers. Different types of biomarkers are measured from the tumour, blood or urine using molecular, biochemical, physiological, anatomical, or imaging method before treatment or during the course of treatment. Observed biomarkers can be used for the selection of personalised treatment for cancer patients. For example, predictive biomarkers are used to predict the response to a specific treatment and prognostic biomarkers are used to measure the aggressiveness of a disease for patients with no or a non-targeted treatment.
These biomarkers can be used to select a treatment for cancer patients. However, biomarkers should be validated before being used to select a treatment in clinical trials. If a biomarker has not been validated through a clinical trial yet, it can be used as a stratification factor of a randomised clinical trial. In such a case, the biomarker can be validated through the trial.
The methods required for design and analysis of a clinical trial with a biomarker-guided personalised medicine can be different depending on the type of the biomarker. In this sense, it is impossible to present a unified design and analysis method for clinical trials of various type of personalised medicines. Various design issues of randomised clinical trials with biomarkers has been widely discussed by Freidlin et al. (2010).
In this article, we discuss design and analysis methods for two cancer clinical
trials of personalised medicine, one with a predictive biomarker and the other with a prognostic biomarker. We use a time to event endpoint in this paper, but the same concept can be used for any kind of endpoints including a dichotomous one, such as tumour response. Readers may refer to Jung (2018) for the details of statistical methods that are discussed in this article.
Methods
A Phase II Trial with a Predictive Biomarker
A predictive biomarker provides information on the likelihood of response to a specific chemotherapy. For example, Sun et al. (2011)observed that non-small cell lung cancer (NSCLC) tumours expressing high thymidylate synthase (TS) levels were resistant to pemetrexed in a preclinical study, but it was not validated by a clinical study yet. A phase II trial (Sun et al. 2015) was conducted to investigate whether TS expression was a predictive biomarker for pemetrexed+cisplatin (PC) or not in patients with nonsquamous NSCLC. In this trial, gemcitabine+cisplatin (GC) was used as a non-targeted control treatment. PC was expected to be more efficacious than GC in TS-negative group, while the two regimens would be similarly efficacious in TS-positive group. To test the hypothesis that TS is a predictive biomarker of PC, patients were randomised between GC and PC arms stratified by TS-positivity. This trial was designed with overall response (OR) as the primary endpoint and progression-free survival (PFS) as one of secondary endpoints. In this article, we assume that the study was designed using PFS as the primary endpoint. For the final analysis of PFS, the hypothesis was tested on the interaction term between treatment indicator (taking 0 for PC and 1 for GC) and TS-positivity using a Cox(1972) proportional hazards model with covariates of a treatment indicator, the TS-positivity, and their interaction. If we want to test if GC is really untargeted therapy or not as a preliminary analysis, we will test if TS-positivity effect is significantly different from 0.
For PFS, the sample size will be calculated by specifying: 1. The expected hazard rates of PC for
TS-positive and TS-negative groups, and that for GCC for the whole patient population (Note that GC is expected to have the same PFS distribution between TS-positive and TS-negative groups.) 2. TC-positivity 3. Accrual rate and additional followup period
4. Alpha and statistical power If one wants to calculate the sample size for the primary endpoint OR, we need to specify response rates, instead of hazard rates of the two treatments in #1, of the two treatment arms for TS-positive and TS-negative patients. And, in the final data analysis, the Cox regression model will be replaced by a logistic regression model.
A Phase II Trial with a Prognostic Biomarker
A prognostic biomarker provides information on the aggressiveness of a disease for patients regardless of a specific treatment. We review a trial with an imaging prognostic biomarker. Chemotherapy ABVD (Doxorubicin, Bleomycin, Vinblastine, Dacarbazine) had been a standard regimen for patients with non-bulky stage I and II Hodgkin lymphoma. In a previous study (Straus et al. 2011) on 6 cycles of ABVD, each patient had an FDG-PET (fluorodeoxyglucose positron-emissiontomography) imaging after 2 cycles of ABVD, and the patients with a negative PET image and those with a positive PET image were found to have a 3-year PFS of 86 per cent and 52 per cent, respectively, with an estimated hazard ratio(HR) of HR0=4.3.
In a new single-arm phase II trial (Strauss et al. 2018), the patients with a negative PET image after 2 cycles of ABVD were treated by additional 4 cycles of ABVD as in the previous study, whereas those with a positive PET image after 2 cycles of ABVD were treated by 4 cycles of a more aggressive chemotherapy BEACOPP (bleomycin, etoposide, doxorubicin hydrochloride, cyclophosphamide, vincristine, procarbazine, prednisone) plus radiation therapy (RT).In this trial, investigators wanted to show that, by treating PET-positive patients with the more aggressive therapy BEACOPP plus RT, their PFS would become closer to that of PET-negative patients who were treated by the standard chemotherapy ABVD. The HR between PET-positive
group and PET-negative group would be as high as HR0=4.3 if both patient groups are treated by ABVD, while it was expected be able to lower it to HR1=2 by treating PET-positive patients with BEACOPP plus RT.
If the estimated HR from the resulting data was shown to be significantly smaller than HR0=4.3 by the noninferiority log-rank statistic (Jung et al. 2005), then we would conclude that 4 cycles of BEACOPP plus RT is more efficacious than 4 cycles of ABVD for PET-positive patients. The required sample size for the study was calculated using the formula proposed by Jung and Chow (2012) by specifying: 1. The expected hazard rates of PFS for 6 cycles ABVD for PET-positive patients and for PET-negative patients, and that for 2 cycles of ABVD and RT plus 4 cycles of BEACOPP for PET-positive patients 2. PET-positivity 3. Expected accrual rate and additional follow-up period 4. Alpha and statistical power
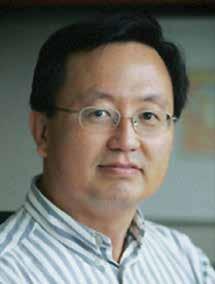
Discussions
The sample sizes and statistical power for the example trials discussed above depend on the prevalence of the biomarker positivity. So, if the observed prevalence is very different from that assumed at the sample size calculation, then the calculated sample size may be underpowered. To address this issue, we may check the observed prevalence before closing patient accrual and recalculate the sample size based on the observed prevalence.
Although we demonstrated the two trials based on the endpoint of PFS, the design and analysis concepts discussed in this article can be used for any other types of endpoints
References are available at www.pharmafocusasia.com
AUTHOR BIO
Sin-Ho Jung has been collaborating on cancer studies for 30 years in the cooperative cancer trial group setting as well as within an NCI-designated cancer center setting. Jung’s recent methodological researches include design and analysis methods for clinical trials, high throughput projects, and health big data.