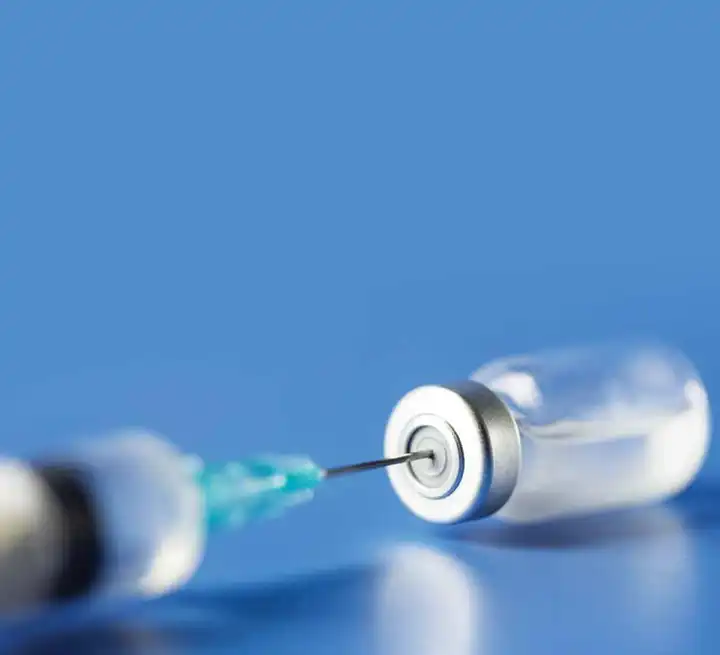
12 minute read
Crucial Decision-making in Drug
Crucial Decision-making in Drug Development for COVID-19 Modelling and simulation guides
As COVID-19 shutters schools and businesses, stresses hospital systems, and threatens patients’ lives, modelling and simulation is guiding crucial decision-making on the frontlines in the development of drugs and vaccines to battle this pandemic. It is being used to track viral outbreaks, show how preventive measures are helping flatten the curve, determine appropriate doses for on-market drugs being tested off-label against COVID-19, and compare the effectiveness of rapidly emerging therapeutics. Now, it is also helping to select the best vaccines for deployment against the disease.
Advertisement
Thomas Kerbusch, Chief Growth Officer, Certara M odelling and simulation has been growing rapidly in impact and is now actively used by biopharmaceutical companies worldwide for drug development and regulatory agencies for review and approval. It aims to integrate information from diverse data sources to help decrease uncertainty and lower failure rates, and develop information that cannot or would not be generated experimentally. Almost all novel drugs use these technologies for drug development.
Medical researchers worldwide are employing modelling and simulation to determine which on-market drugs, drug combinations and vaccines may be safe and effective against SARS-CoV-2, the virus responsible for COVID-19.
Treating Different Disease States
COVID-19 involves three disease stages. When a person first gets infected, the virus is actively replicating. At that stage, the goal is to inhibit replication, so viral replication inhibitors are used to supplement the person’s own immune response. Repurposed antivirals, which are already approved and on-market for another indication, are currently being employed to treat this initial disease state. But new, more tailored drugs are being investigated too.
In the second stage of the disease, the viral replication results in respiratory epithelial cell death and local pulmonary inflammation. At this second stage, a person may start to experience significant respiratory problems, such as Acute Respiratory Distress Syndrome (ARDS), requiring supplemental oxygen and potentially a ventilator.
In the final stage, there is a systemic overreaction of their immune system, known as a “cytokine storm.” This overreaction weakens the body’s physical barriers, causing the virus to go deeper into the tissue, starting to affect organs like the lungs and brain and impact the blood clotting system.
COVID-19 is difficult to manage, because the effectiveness of viral inhibition treatment is highly dependent on timing of infection and initial viral load. Sophisticated computer models of antiviral drug exposure, including combinations, and its effect on individual virological response have been built. Disease progression is also incorporated into these models to ensure that the right drug is administered with the right dose and right time.
Developing the Best Vaccines
The traditional approach to vaccine development is to first test the candidate vaccine in a small number of people in a Phase 1 trial to see if it is safe. Then, it is tested on a larger group of people in Phase 2 to see if it is safe and generates an immune response. After that, it goes into a large Phase 3 trial to see if it actually works in preventing subjects from getting the disease.
While safety is extremely important, it is not the only important factor. If the dose level (vaccine strength and adjuvant selection and dose) and regimen (number of vaccine inoculations) are optimised based solely on safety considerations, a vaccine may be developed that's not sufficiently efficacious.
By using model-based approaches to predict expected safety and efficacy, optimal vaccine candidates can be identified the first time and accelerate the development process.
On July 6, the World Health Organization reported that there were 149 candidate vaccines under evaluation for COVID-19. Several of them entered large-scale clinical trials in July. It is anticipated that more than one COVID-19 vaccine will be selected. The number of doses needed is so large that production limitations will effectively require there to be several safe and effective vaccines.
Accelerating Development with Advanced Quantitative Approaches
Model-based meta-analysis (MBMA) approaches can be used to extrapolate from the correlate of protection as measured in preclinical assays and predict what the clinical immune response is likely to be. Summary clinical trial data can then be employed to compare efficacy between different repurposed drugs. It will also soon be possible to compare different vaccines to determine which provide superior efficacy. MBMA is ideally suited to make these comparisons across a wide range of differently designed clinical trials, patient populations and countries.
Another cutting-edge approach involves adapting a quantitative systems pharmacology (QSP) model for immunological response to COVID-19 applications. This unique QSP immunology model was originally developed to determine unwanted immunogenicity by predicting whether an administration of a specific monoclonal antibody is expected to trigger unwanted immunological responses defined by an antibody-antibody reaction. However, this QSP model is now being repurposed to predict the desired immunological response of a vaccine. Mediated by the
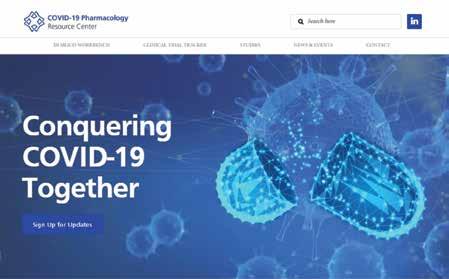
Photo of the COVID-19 Pharmacology Resource Center at www.covidpharmacology.com
Photo of the compound screening dashboard in the COVID-19 Pharmacology Resource Center. The compound screening dashboard has a heatmap where you can select compounds of interest and then the heatmap shows which ones rate high and low on proof of hope for COVID-19, based on key metrics such as Cmax/EC50 and Cminss/EC50.
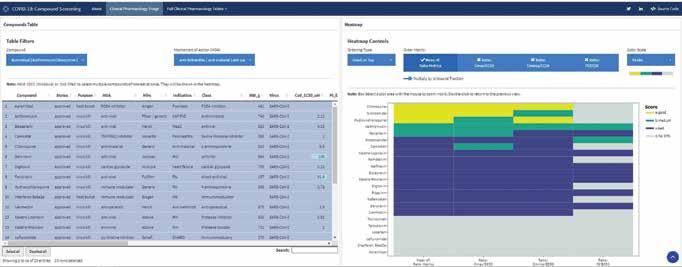
same immune system components, the QSP model can predict if a vaccine will provide sufficient and lasting immune protection. This allows researchers to predict, based on the vaccine’s antigen profile, what level of immune response it will generate, and not just the extent but also the duration of the immune response.
This type of modelling and simulation also helps to determine whether people will need to be inoculated once or twice, require initial boosting, or if the immune response will persist or wear off.
Improving Clinical Trials
There were 1,676 COVID-19 clinical trials underway globally as of July 6, according to Cytel’s Global Coronavirus COVID-19 Clinical Trial Tracker. They are all competing for resources – patients, staff or funding – leading to concerns that some of the studies will be underpowered and unable to produce meaningful, statistically significant results.
Modelling and simulation has been used to support more than a dozen COVID-19 programs, with trial design, dose optimisation, and stage-gate decisions. Larger studies have also been created in which many smaller trials can be included. This ensures that the same high level of rigorous study conduct is applied across all trials and their data can be pooled, making their results much more powerful from an interpretation perspective.
QSP and physiologically-based pharmacokinetic (PBPK) modelling are being used to help select drugs for inclusion in trials based on their mechanism of action. QSP enables the drug’s mechanism of action to be figured out and allow researchers to determine whether it will be beneficial in the active treatment of COVID-19, either by inhibiting the virological response or modulating the immune response. PBPK modelling allows researchers to figure out whether the drug gets to the site of action.
One recent example of this is ivermectin. For any drug to be therapeutic, enough of the drug needs to get into the cell to be efficacious and still be safe. It was reported that ivermectin inhibits the replication of SARS-CoV-2 in cell culture. But how does this translate into safety and efficacy in humans? A PBPK model was used to study virtual patients and determine the relative potency levels of the drug in the body. It predicted that the right level of exposure of ivermectin will most likely not occur in cells to be efficacious without compromising safety.
Comparative Effectiveness to Identify Winner Vaccines
A COVID-19 Clinical Outcomes Database has been developed for drugs that show activity against SARS-CoV-2. It uses public clinical trial and observational study information. It already includes data on repurposed drugs, and it is being expanded daily with new trials and studies reading out. Vaccine trial data will also be added as they become available.
These clinical outcome databases include pooled information across trials, drugs, mechanisms of action and trial designs, and then MBMA is used to make inferences from all the data. This pooled MBMA approach allows trials to be combined that use different methodologies and patient populations. It enables a model to be built that includes patients at different stages of the disease.
From a modelling perspective, the Clinical Outcomes Database functions similarly to a registry trial. Instead of pooling dozens of trials, it pools hundreds of trials across all the relevant drugs. Different drugs are used in the same data pool and estimates are made of the impact of disease status, patient characteristics and trial designs on the outcome of treatments. Then by accounting for those differences in patients and
Image shows the CQ simulator visualisation from the COVID-19 Pharmacology Resource Center.
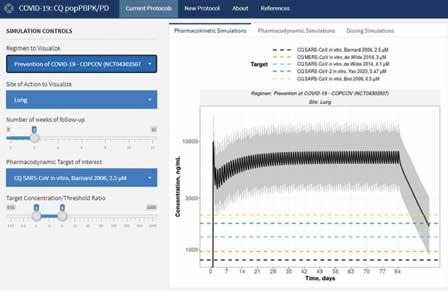
trial designs, it allows apples to apples comparisons to be made, resulting in the comparative effectiveness of different drug treatments.
The impact of a treatment on COVID-19 is based on the stage of disease progression. The power for detecting and estimating those effects is improved by pooling all that information.
Then, we can start asking critical questions: When is the right time to dose? What is the right dose? Which drugs work best on which patient population? Which trial design provides the best response in those populations?
Analogously for vaccines, there are questions around comparative effectiveness and making sure that we learn quickly which are the likely winners from the initial response trials.
Insights from this Clinical Outcomes Database will be provided in the COVID19 Pharmacology Resource Center. We developed the Center with support from the COVID-19 Therapeutics Accelerator to foster global collaboration, share best practices, and facilitate COVID-19 candidate drug development.
Shifting to Combination Therapies
COVID-19 is such a complex disease that combination therapy will likely be
Excellence in Antibody and ELISA Development
For more information please contact us:
service@biogenes.de
Preclinical Research
Clinical Phases I, II
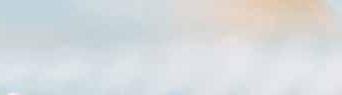
Clinical Phase III
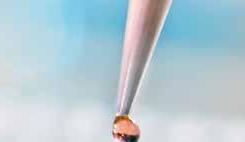
Approval (FDA, EMA)
Custom Antibodies
mAbs, pAbs and anti-idiotypic Antibodies
Generic 360-HCP ELISA Kits
for CHO cells and E.coli
Custom ELISAs
Host Cell Protein (HCP) Assays
Analytical Services
2D Western Blot, DIGE, IAC
Proactive project management Reliable Flexible Full con dentiality
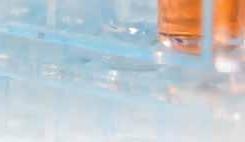


BioGenes GmbH · Berlin, Germany · ✆ +49 (0) 30 6576 2396 · www.biogenes.de
required to treat it. Our models allow us to look at the combined effects of different drugs and the impact of temporal administration. They allow us to suggest at which point in the disease progression one drug should be given, and when to switch to another, for optimal patient benefit, which can then be further evaluated in pre-clinical and clinical studies.
Regulatory and Deployment Strategies to Expand Access
A pharmacology to payer (P2P) model, which incorporates both epidemiological and health economic data, is being used to translate comparative effectiveness into real-world scenarios for COVID19 drugs and vaccines.
Deployment models can help to determine who should be treated first with the new vaccines. Do you start with healthcare workers on the frontlines? Then the elderly because they're affected the most, starting in elderly care homes where we're seeing the most flare-ups?
We can use epidemiological models to start describing the herd immunity that we need. Unfortunately, herd immunity is currently very low in most countries, running anywhere from 2 per cent to 4 per cent. Based on past experience, it will probably need to be approximately 60 per cent. Then, when the replication rate of the virus decreases, the infection risk goes down within the population.
Another consideration is how to navigate regulatory pathways to accelerate approval of COVID-19 therapeutics and vaccines. Modelling and simulation has been used to successfully fast-track drug approvals for orphan diseases and areas of high, unmet medical need, such as immuno-oncology. That expertise can be applied to COVID-19. Regulatory science and strategy expert teams that have successfully presented novel approaches, communicating the risk/ benefit profiles of new drugs, repurposed drugs and vaccines to regulators will have the requisite experience to meet that challenge.
Supporting Disrupted Trials and Programmes
COVID-19 is having a disruptive effect on clinical studies in other indications. Most trials for non-life-threatening conditions were put on hold or slowed down either because staff needed to provide clinical care for COVID-19 patients or the health risks of bringing patients in for follow-up appointments were too great.
Experienced regulatory teams can help their clients communicate the extent and impact of any trial disruptions to regulatory authorities. Then they work on mitigation solutions that help them get back on track. Modelling can also help disrupted trials recover their statistical power if patient attrition leaves them with incomplete data.
One of modelling’s strengths is that it looks across the population for the entire time course, whereas a classical statistical analysis looks at one time point and makes a comparison between two groups of patients. If a patient’s last visit data is missing because they dropped out of the study, we can make a reasonable prediction of what that last visit would have looked like based on their previous visits using a model.
Pharmacological models of drug response allow us to integrate data across time. We can borrow statistical strength across the time course to predict the
AUTHOR BIO
clinical endpoint. Therefore, we can still model the data even if data are missing.
Working Together
COVID-19 is a global problem that requires a global solution. Fortunately, modelling and simulation has already been adopted by global regulatory agencies, including the US Food and Drug Administration (FDA), European Medicines Authority (EMA), Japan’s Pharmaceuticals and Medical Devices Agency, China’s National Medical Products Administration, and the Australian Therapeutic Goods Agency.
The Asia-Pacific (APAC) pharmaceutical market is growing rapidly and by 2023, it will be larger than the European market. While Japan has the most established pharmaceutical industry, those in Greater China and South Korea are growing quickly, and their biopharmaceutical companies are now expanding beyond their domestic markets and eyeing international markets, using modern, innovative R&D pipelines. As a result, they have begun applying methodologies, such as modelling and simulation, which are required components in new drug applications to the FDA and EMA.
APAC’s expanding involvement in global drug development and increasing adoption of modelling and simulation, could not have come at a better time, because COVID-19 demands that we all work together with scientific and quantitative approaches to produce the best drugs and vaccines to protect us all against this disease.
References are available at www.pharmafocusasia.com
Thomas Kerbusch is Chief Growth Officer at Certara, overseeing executive client relationships and integrated software and service offerings in growth markets, including APAC. Kerbusch joined Certara in 2015, initially leading EU consulting services and later serving as Divisional President. Prior to that, he had a 15-year track record of successfully developing new drugs while leading and working with teams of researchers at Merck/ MSD, Schering-Plough, Organon and Pfizer.