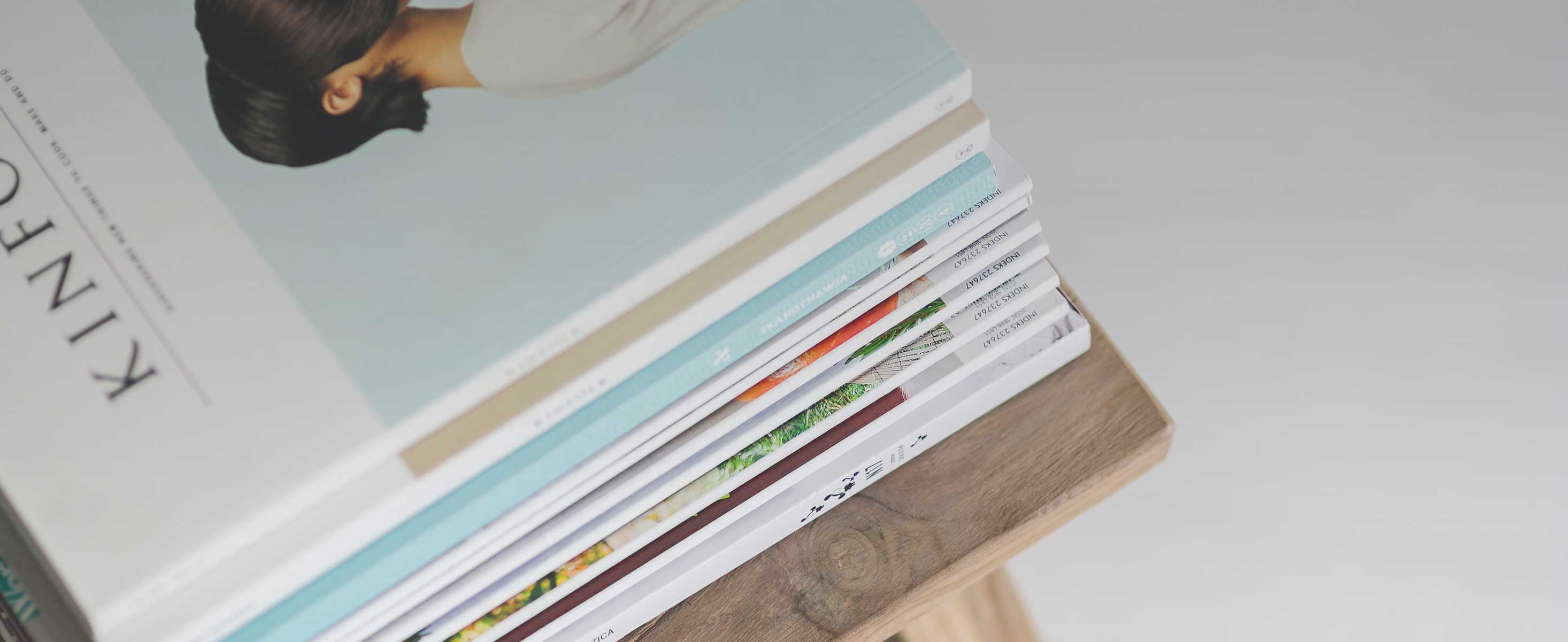
8 minute read
Using Quantitative Systems Pharmacology Modelling to Accelerate Drug Development
Model informed drug development (in silico modelling) continues to be a game changer in accelerating drug development. Quantitative Systems Pharmacology (QSP) is one such technology, which employs virtual patients in virtual clinical trials, allowing more approaches to be investigated than would be possible in the real world. Examples described here demonstrate the impact on some of today’s most urgent medical needs, including evaluating a potential treatment for Alzheimer’s disease, paediatric dosing for a COVID-19 vaccine, and evaluation of novel therapies for irritable bowel disease.
Piet van der Graaf
Senior Vice President, Quantitative Systems Pharmacology, Certara
Quantitative systems pharmacology (QSP) combines computational modelling and experimental methods to explore the relationship between a drug, human biology, and the disease process. QSP modelling leverages large quantities of biological and pharmacological data to increase scientific knowledge of disease pathophysiology and facilitate the investigation of different therapeutic approaches in virtual patients in virtual clinical trials. As virtual patients are used instead of real people, QSP modelling saves time and money and allows more drug doses and drug combinations to be investigated than could be practically and ethically evaluated in real life.
QSP modelling can help to identify a new drug target or biomarker, select the optimal drug dose for a vulnerable patient population or an individual patient, repurpose an existing on-market drug for a different indication or develop a new combination therapy.
QSP is a relatively new discipline, but it is already having a profound impact on decision making at many stages along the drug development continuum. In a CPT:
Treating Alzheimer’s Disease
Eisai and Biogen Inc. announced positive results from their large, global, Phase 3 confirmatory Clarity AD clinical study of lecanemab on November 29, 2022. Lecanemab is an investigational antiamyloid beta (Aβ) protofibril antibody for the treatment of mild cognitive impairment due to early Alzheimer's disease (AD) with confirmed presence of amyloid pathology in the brain. Clinical results were presented at the 2022 Clinical Trials on Alzheimer's Disease conference, in San Francisco, California.
Pharmacometrics & Systems Pharmacology paper published in November 2022, U.S. Food and Drug Administration (FDA) staff reported that there has been a notable increase in the number of regulatory submissions that contain QSP, including Investigational New Drug Applications (INDs), New Drug Applications (NDAs), and Biologics License Applications (BLAs) during the past several years. Their report concluded that QSP is increasingly applied to model and simulate both drug effectiveness and safety throughout the drug development process across disease areas.
The following case studies illustrate what all the excitement is about and demonstrate QSP’s potential to advance drug development.
Lecanemab treatment showed 31% lower risk of converting to next stage of disease by Global CDR assessment (Hazard Ratio: 0.69). A slope analysis using CDR-SB based on observed data and extrapolation to 30 months showed that lecanemab takes 25.5 months to reach same level as placebo at 18 months, indicating a 7.5 month slowing of progression.
The Certara team had started to work with Eisai about two years before these results were announced and developed a QSP model to predict and help interpret the outcomes of the trial. In fact, Certara’s
Studying Amyloid Aggregation
AD pathogenesis is widely believed to be driven by the production and deposition of the Aβ peptide. Amyloid aggregates are classified as monomers, oligomers, protofibrils and fibrils based on their size and solubility. As antiamyloid monoclonal antibodies have different affinities for these species, it could lead to different levels of standardized uptake value ratio (SUVr) reduction, and potentially different clinical outcomes.
Certara created an amyloid QSP model to study lecanemab. The model included all known features of the Aβ aggregation process, together with the mechanisms of action employed by drug candidates endeavouring to treat Alzheimer’s disease by modifying Aβ pathology.
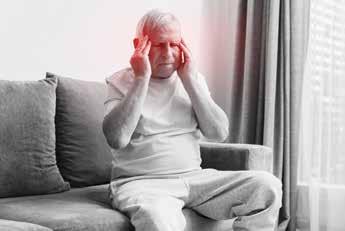
The model was validated by demonstrating that it could accurately replicate the clinical pharmacokinetic/pharmacodynamic (PK/ PD) data for several monoclonal antibodies – lecanemab, gantenerumab, solanezumab, bapineuzumab, and crenezumab. The model was then used to investigate 18 months of treatment with those antibodies, followed by 2.5 years of observation. The platform revealed significant differences in oligomer, protofibril, and plaque reduction between the monoclonal antibodies. At clinical doses, lecanemab produced pronounced reductions in protofibrils and the SUVr, which served as encouraging precursors to its phase 3 clinical results.
Other examples of using QSP modelling to predict clinical outcomes are included below.
COVID-19 Pediatric Vaccine Dosing
QSP model predicted the positive outcome of this trial about one year early, as presented at the AD PD Annual Meeting in March 2022.
At the start of the COVID-19 pandemic, Certara quickly realized that the QSP model of the human immune system that it had developed to predict immunogenicity with protein-based therapeutics, such as antibodies, could be repurposed as a vaccine model.
Immunogenicity, or the tendency to trigger an unwanted immune response, is a major problem with biological therapies because it can lower the patient’s exposure to the medicine and reduce its efficacy. Immunogenicity can also cause immune-related adverse events. While the goal of the original model was to identify drugs that generated low levels of immunogenicity, the goal of the new model was the opposite, to find vaccines that produced high levels of immune response to provide protection against the SARS-CoV-2 virus. Part of the COVID-19 virus sequence was input into the new vaccine model to demonstrate that it would generate an immune response. Then, the model was calibrated using the structure of actual COVID-19 vaccines and validated by replicating their published clinical data. The resulting COVID-19 vaccine simulator was able to predict with a virtual trial the outcomes of actual clinical trials using those vaccines.
The vaccine simulator was initially used to determine the optimal timing between COVID19 vaccine doses and whether vaccines could be combined during a shortage. Daiichi Sankyo employed Certara’s vaccine model to optimize the phase 1 study design for its COVID-19 vaccine and to predict immune responses in specific populations, such as Japanese people and older adults.
The focus later shifted to paediatrics where the simulator was used to guide dose selection in young children. That is one of the biggest challenges in vaccine development. When working with vulnerable populations, such as the elderly, young children, or babies, it is even more imperative to use exactly the right dose and not a little too much or too little. It is a delicate balancing act, selecting a dose that is high enough to be sufficiently efficacious but low enough to produce minimal side effects. The situation is more complex in children because their physiology, enzyme and transporter levels change as they mature. One of the many advantages of QSP modelling is that it allows vaccine doses and other variables to be adjusted and the results observed virtually without the need to conduct clinical trials.
Pfizer-BioNTech’s rollout of its COVID19 vaccine was extremely successful. But even Pfizer-BioNTech had to redo its first paediatric COVID-19 vaccine trial and change the dosing regimen because two 3 µg doses of its vaccine did not produce a sufficiently protective immune response in children aged two to four years. They ultimately added a third 3 µg vaccine dose, administered at least two months after the second dose, for that population.
Certara’s COVID-19 vaccine simulator, which now includes 32 clinical datasets, three vaccines, eight dosing regimens, and 10 biomarkers, predicted that response. Its QSP model confirmed that two 30 µg doses of Pfizer-BioNTech’s COVID-19 vaccine would be effective in children aged 16 to 25 years (the same as an adult), two 10 µg doses (one-third the adult dose) would be effective in children aged 5 to 11 years, but two 3 µg doses (one-tenth the adult dose) would be ineffective in children aged 2 to 4 years and they would require a third 3 µg dose.
Inflammatory Bowel Disease
Inflammatory bowel disease (IBD) refers to two conditions, Crohn’s disease (CD), and ulcerative colitis (UC), which are characterized by chronic inflammation of the gastrointestinal (GI) tract. Prolonged inflammation results in damage to the GI tract. IBD symptoms include persistent diarrhoea, abdominal pain, rectal bleeding/ bloody stools, weight loss, and fatigue.
In 2015, an estimated 1.3% of US adults (three million) reported having received a diagnosis of IBD. The prevalence of IBD varies based on a person’s age, race/ethnicity, education level, employment status, nativity, poverty status, and urbanicity. IBD occurs more often in non-Hispanic White persons than in other racial/ethnic groups.
The impact of medication on IBD symptoms has traditionally been hard to measure. When a patient with IBD meets with their clinician, they are often asked to rate how they are feeling on a scale of 1 to 5 with five representing the worst/most severe symptoms. But how do you rate “not great”? How do you represent biology on a questionnaire?
Further compounding the complexity of the situation, different hospitals use different disease activity scores to measure disease severity and therapeutic outcomes. For example, the Mayo Clinic developed the Mayo Score/Disease Activity Index for Ulcerative Colitis to assess the initial disease severity, change in activity over time, and response to treatment. This scoring system factored in four elements: rectal bleeding, stool frequency, physician assessment, and endoscopic appearance. Each element was rated from 0 to 3, giving a maximum total score of 12. The higher the score, the more severe the ulcerative colitis.
But the FDA is now accepting a modified Mayo Score, which no longer includes mucosal friability in the endoscopic score or the physician’s global assessment in the composite index, and has a maximum total score of 9.
Clinicians prefer to use these scores rather than levels of biomarkers, such as fecal protectin (FCP) or serum c-reactive protein (CRP), when evaluating a patient’s progress because they also factor in clinical observations such as intestinal bleeding and ulceration.
The primary challenge in this field is how to link biological mechanisms to these subjective endpoints, which are the actual clinical development scores that clinicians use.
To achieve that goal, Certara combined QSP with model-based meta-analysis (MBMA), another in-silico technology, to create QSP models which can predict those subjective clinical endpoints in IBD. Essentially, the team used artificial intelligence and machine learning to link mechanistic QSP to clinical endpoint scores.
QSP can model the biological effects of drugs up to the biomarker level, i.e., it can determine whether cytokine levels are going up or down. Then, MBMA uses data reported from clinical trials and in the scientific literature to correlate the tissue biomarker measurements from the QSP model with disease activity scores for UC and CD. It determines in a purely statistical manner what cytokine levels correlate with specific scores on the Mayo Scale.
The Certara team included inflammatory cytokines and therapeutic antibodies in a mechanistic, multistate QSP model. Then they added published baseline and patient Mayo Score, Crohn’s Disease Active Index (CDAI), CRP, and FCP data to enable correlation between the biomarker levels and clinical measurements. A virtual patient population was created with tissue cytokine, cell density, and gut measurements to match an existing clinical patient population. The tissue biomarkers used were Treg, TNFα, neutrophil, and Th17. An algorithm then assigned the appropriate clinical score to the virtual patients based on their Mayo, CDAI score distribution in relation to FCP or CRP. The resulting virtual population helped to train a multinomial logistic regression model which was able to successfully predict clinical response and remission rates for patients prescribed anti-TNFα (adalimumab), anti-IL-23 (mirikizumab) or a combination of the two drugs in clinical trials.
The resulting predictive model can now be used to guide dose selection for IBD drug candidates in Phase I/II clinical trials.
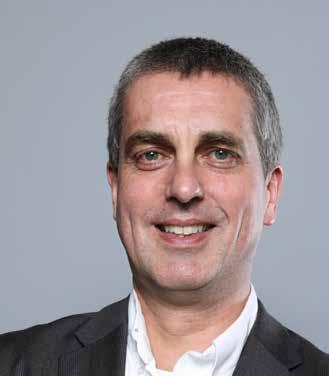
Prior to this breakthrough, most QSP model predictions ended at the biomarker level with no quantitative linkage to actual patient outcome.
Conclusion
QSP modelling is a powerful tool that can be applied throughout the drug development continuum from identifying a new drug target in the earliest stages through to predicting clinical outcomes. These advances all serve to accelerate the drug development process safely and efficiently.
Professor Piet van der Graaf is Senior Vice President, Quantitative Systems Pharmacology at Certara and Professor of Systems Pharmacology at Leiden University in the Netherlands. Before joining Certara, he was the Director of Research (CSO) of the Leiden Academic Centre for Drug Research and held various research leadership positions at Pfizer across discovery and clinical development.