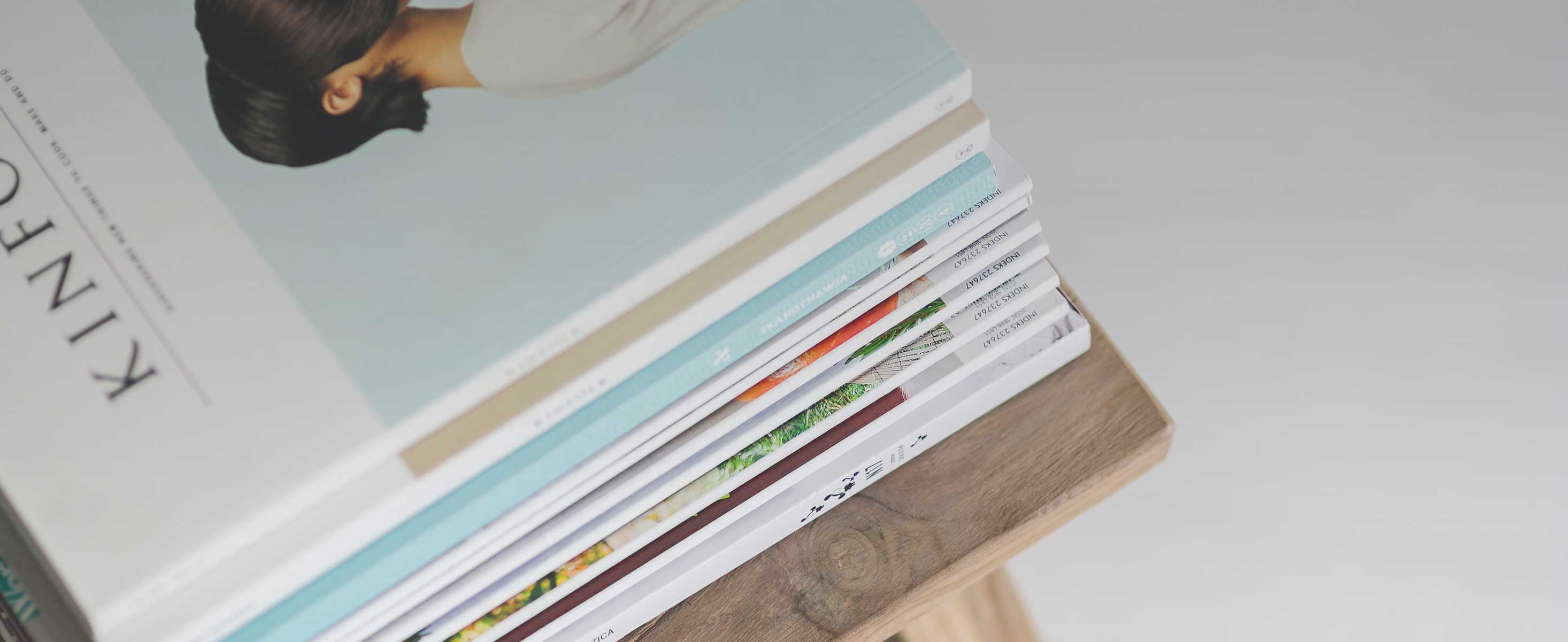
4 minute read
Understanding interactions with family background
facilities, enabling an examination of differences between management practices and incentive systems by facility ownership. The survey builds off two highly used management tools—the D-WMS and the SafeCare Standards— and was adapted to fit the Kenyan primary health care context.6 Five hundred facility managers will each participate in an hour-long phone survey, in which they will be asked a series of validated questions covering 17 domains of management practices, including leadership vision and goals, standardization of protocols, performance tracking and review, and continuous improvement. This survey not only will allow for benchmarking of management practice outcomes in Kenya against practices in other settings (given that the D-WMS methods are standardized) but also will introduce innovations to measure management practices relating to staff absenteeism and stockouts of equipment, supplies, and medicines.
Beyond management, various SDI surveys have also attempted to get a general idea of the revenue composition of both schools and health facilities—that is, whether they finance their operations with their own resources, cost recovery, transfers from the central level, transfers from decentralized levels, donations, or any other means. Although these data have proven to be extremely useful for understanding the potential implications of education and health financing reforms at the country level, their collection and cross-country comparability have been challenging because of country-specific financing and organizational structures, as well as data privacy concerns. Likewise, having a broad understanding of the composition of expenditures (human resources, equipment, and maintenance, among others) would be invaluable for understanding differences in efficiency between facilities and over time. As the SDI program continues to evolve, it will inevitably grapple with the balance between cross-country comparability and country-specific needs, particularly as they relate to financing, structural organization, and expenditures.
Learning, child development, and health are outcomes of complex processes that are based on access to quality schooling and affordable, quality health care, but also on individual and family choices. A large body of evidence shows that children in poor households experience worse human capital outcomes, and several factors can contribute to these gaps. Lack of financial resources and limited ability to borrow often prevent poor households from accessing health and education services. External shocks may force children in poor households to drop out of school or may otherwise adversely affect their learning outcomes.
Households may face significant barriers or opportunity costs in acquiring human capital. In addition, social norms about women’s roles, which may be related to socioeconomic status, shape many critical decisions related to human capital, such as fertility, breastfeeding, or schooling (World Bank 2019).
Understanding the individual and family characteristics that are likely to shape children’s learning and health is an important complement to understanding service use. In education, older surveys collected only basic information on family characteristics, such as whether a child had breakfast that morning. More recently, SDI surveys in both health and education have expanded these efforts. For instance, the SDI education surveys in the Democratic Republic of Congo (forthcoming 2021) and in Indonesia (2019) collected data using household modules from a subsample of students tested in SDI schools (Yarrow et al. 2020). These modules include questions on topics such as parental education, household characteristics (infrastructure, work and other sources of income, and household structure), assets (both agricultural, such as livestock and crops, and nonagricultural, such as ownership of a television, radio, computer, motor vehicle, or refrigerator), books at home (number and type), and type of educational expenditures (school fees, food, and others). Likewise, the forthcoming SDI health survey in Guatemala includes a household module that collects information about household characteristics, health expenditures, and demand for health services. These new initiatives will shed light on potential improvements in measurement for future SDI surveys and yield additional evidence on the link between household characteristics, demand for services, and quality of service delivery.
More innovative methods to connect with households also show promise and can inform the evolution of SDI methodology in the future. For example, in the SABER–Service Delivery survey in Punjab, Pakistan (2018), parents’ phone numbers were obtained from school records, and a phone interview was conducted to document household characteristics. This information can be linked to children’s learning outcomes to explore differences by socioeconomic status. Recent evidence from the LEAPS study, also in Punjab, Pakistan, shows that information on household assets and parental education collected from students at the school level correlates well with information collected from parents at the household level during an in-person visit (Bau, Das, and Yi Chang 2021).
Finally, the analysis of health care in this book offered a preview of how the combination of SDI data and administrative data can help to identify bottlenecks in the delivery of health and education services. There may be many reasons behind drug stockouts in health centers and the absence of soap in school bathrooms, including lack of road access or inadequate power supply. Combining SDI data with administrative data and geocoded information indicators (such as road access, electrification, and water supplies) can help to improve the accountability of government overall, not only in the health and education sectors.