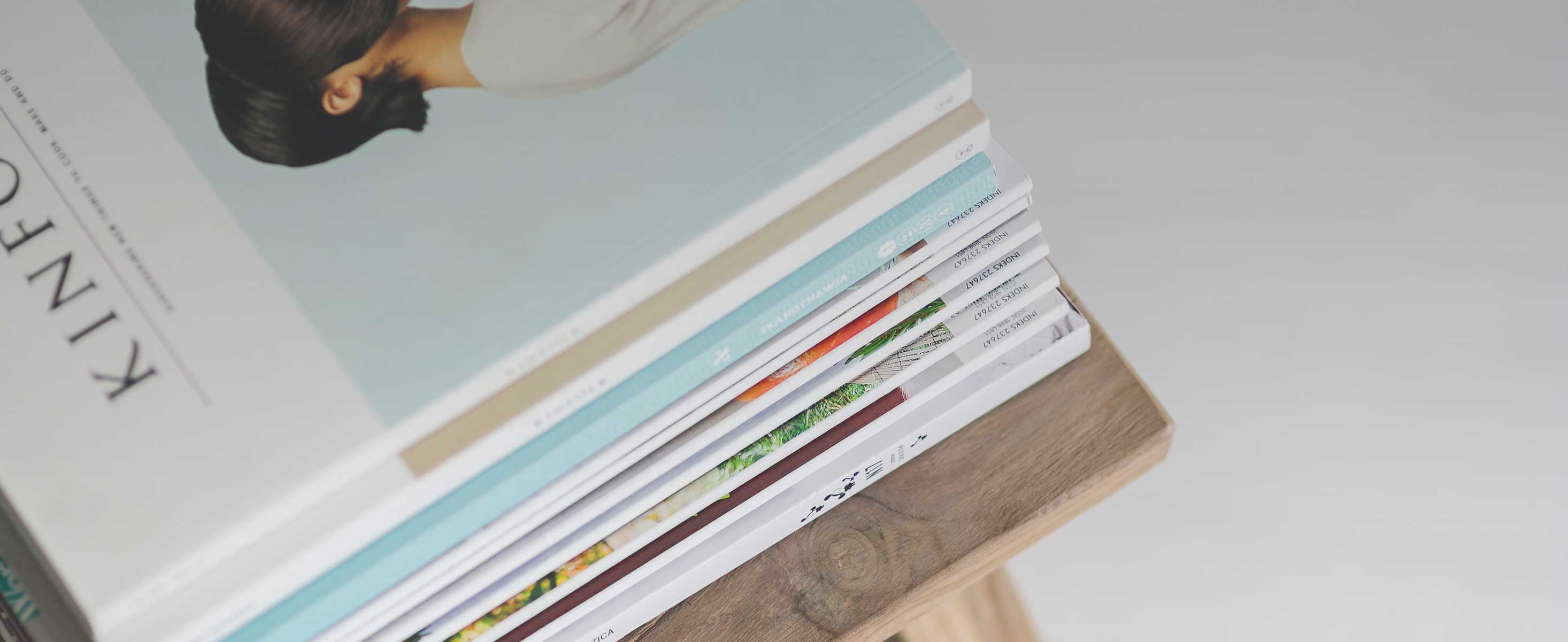
1 minute read
Annex 3F: The Simulated Method of Moments Estimator
Following Ulyssea (2018), the analysis considers S = 50 simulated data sets containing a mass of M = 30,000 potential entrants each. For each potential entrant, an observed productivity and a misperception of productivity are drawn. The stochastic components of the model are drawn only once—at the beginning of the procedure—and are kept fixed during the estimation.
To save computational time, 200 equally spaced grids are used as a representative sample of the whole population. Firms with productivity near one grid are categorized into the same grid. The economic behavior of these 200 representative firms is then calculated. The behavior of firms in the entire sample are then recovered through a spline proxy to map their productivity onto economic outcomes.
One difficulty in the estimation of discrete choice models using a simulation-based method is that simulated choices will be a step function of the parameter vector given the random raw data. Because these discontinuities are inherited by the objective function, this also precludes the use of derivative-based methods, which are more rapid and more accurate than derivative-free methods or random search algorithms (Bruins et al. 2018). To overcome these challenges, the analysis relies on the following smoothing function to correct for the choppiness of the policy function.
, , V
/ 1 /
hV m ϕ λ ( ) ( ) = + V ∑ m k k ϕ λ ϕ λ ( ) ( )
, (3F.1)
where V() ϕ is the set of net payoffs attached to the choices of firms. As the smoothing parameter l goes to zero, h(⋅) goes to 1 if alternative m provides the highest payoff, and zero otherwise. In choosing λ, the analysis follows the method of Ulyssea (2018) to balance bias and smoothness. The analysis sets λ = .05 and S = 50.
The estimator is given by the following:
ϕ ϕ ϕ ϕ { }
′ˆ ϕ () ()argmin Q g NS W g gNS NS () , (3F.2)
where ϕ ϕ = − () ()g m mNS N s . For notational convenience, the conditioning arguments are omitted.
The conditions for consistency are close to the conditions for the extremum estimator. For an extensive discussion of the weight matrix, see Gourinchas and Parker (2002), Newey and McFadden (1994), and Ulyssea (2018).