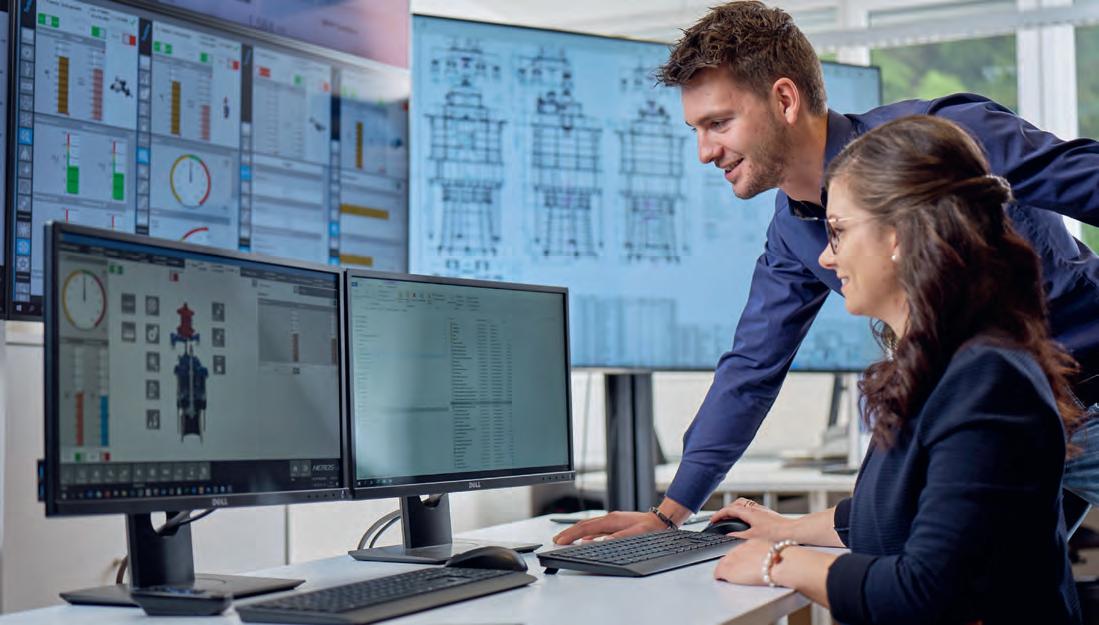
11 minute read
Upper Austrian hydropower specialist determined to digitalise
from zek HYDRO 2022
by zek Magazin
Global Hydro is a widely-acknowledged specialist in the field of hydropower and has been investing in a comprehensive programme of digital transformation for several years. Today the company is recognised as a pioneer in the fields of Machine Learning and Predictive Maintenance.
All photos: photo credits: Global Hydro
‘Digovation 2025’ is the banner under which the Upper Austrian hydropower experts at Global Hydro are combining digitalisation and innovation to achieve some very ambitious goals. As well as innovations under consideration in machine building, geometry and manufacturing technology – efforts are also being invested in various digitalisation projects. These include popular trending developments in the thematic field of artificial intelligence: machine learning and predictive maintenance. Self-driven learning processes are soon expected to enable machines to make their own decisions and to predict probable scenarios. These are no longer visions of the future at Global Hydro, since several power plants are already testing their prototypes.
Over the past few years very few issues have fascinated the hydropower industry more than the phenomenon of digitalisation. There has been feverish activity in the various R&D departments of the industry’s leading companies on hearing buzzwords and phrases like ‘Digital Twin’, ‘Big Data’, ‘Machine Learning’ and ‘Predictive Maintenance’. One company pioneering these developments is Global Hydro, the well-known hydropower allrounder from the Upper Austrian town of Niederranna. The specialists from the Mühlviertel area coined the phrase ‘Digovation 2025’ a couple of years ago as they launched an ambitious plan that divided digitalisation transformation thematically – on the one hand into digital workplaces, and the digitalisation of power stations and products on the other. Richard Frizberg is Managing Director at Global Hydro and responsible for business development, finances, HR and the allocation of expertise within the company. He explains: “In order to be able to implement these projects we established our own new department, ‘HydroLab’, and formed a new ‘DataScience’ team within the software development department. Depending on each task and each set of requirements there is collaboration between members of various teams from data engineering, data science, software engineering and gauging technology.”
A TEAM OF EXPERTS HydroLab was set up in its present form more than a year ago. It is now responsible for the
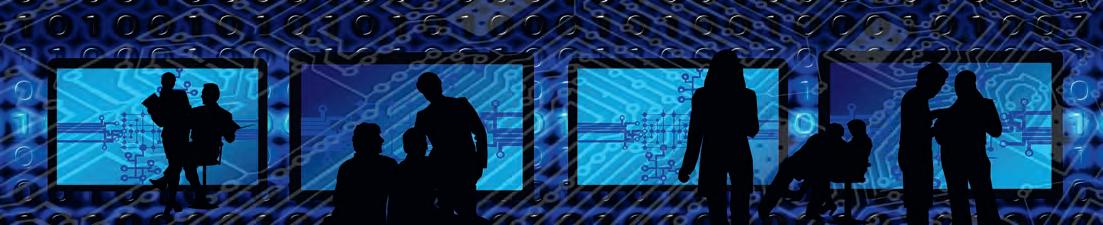
graphics: Pixabay
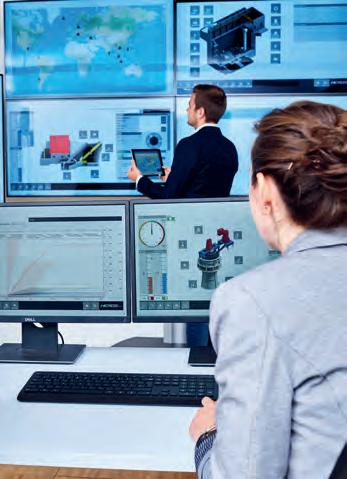
Teamwork is decisive for the success enjoyed by Global Hydro.
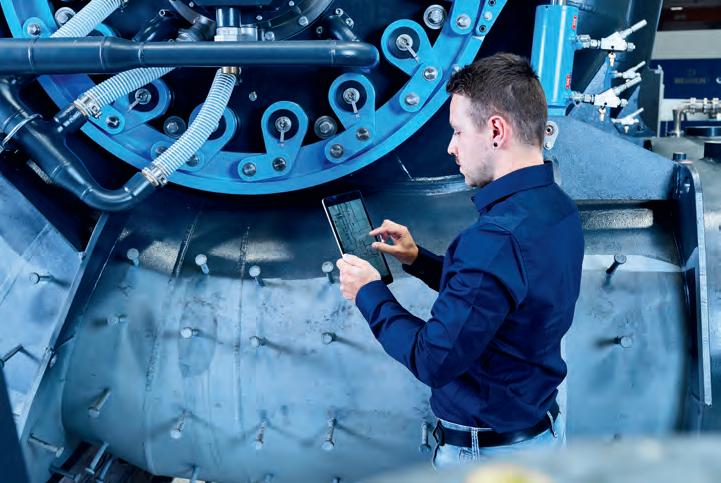
overall development of basics, for testing and for the interpretation of serial test results in order to gain a basic understanding of how development can progress. Thomas Stütz, Head of Electrical Engineering and Software Development sketches out the modus operandi: “Most of our team members are experts in the fields of hydraulic engineering and measuring technology. The DataScience team takes these data, attempts to generate interpretations based on the data, and develops machine learning models.” This is done in regular cooperation with highly-reputed research institutes, universities and technology partners.
HOW THE MACHINE LEARNS When asked how to define machine learning in the context of hydropower technology, Global Hydro’s head engineer explains it’s just one field of A.I. – artificial intelligence, the foremost task being to devise models that are capable of learning without assistance. The model recognises patterns from previous event data, enabling it to make better sense of current events and, if successful, to make meaningful predictions for the future. “Generally speaking, machine learning is continuing to grow in importance, although the exercise is nothing new. Some of the algorithms currently in use were developed in the 1960s”, states Stefan Plank, a Global Hydro Data Scientist. Ultimately, the answer to the question of how machines learn is less thrilling than the question itself: The same way humans learn – through repetition and optimisation. “There’s always a concrete training data set linked to a target vector. One common example is the way machine learning allows handwritten figures to be recognised. In this case, the training data consist of lots of hand-written numbers. The target vector always contains the correct number the model is expected to recognise. An algorithm is used to solve the test with the training data. This is a process of optimisation that is repeated until the desired result is achieved”, outlines Plank.

Depending on the task in hand, experts from the new HydroLab and Data- Science departments collaborate with specialists from various other fields.
CUSTOMER BENEFITS AT THE FOREFRONT Although there may still be several obstacles en route to the self-organising hydropower plant, the goals are clear – certainly for the decisionmakers at Global Hydro. “For us digital transformation is about two main goals: The findings we require to develop our products, and about how to optimise the operation and maintenance of plant infrastructure for our customers. Our primary objective is to guarantee benefits for our customers”, Managing Director Richard Frizberg emphasises. He enlarges the perspective to declare the intent to provide comprehensive solutions: “What this means in practice is that instead of concentrating on just a turbine or an auxiliary generator, we aim to deliver all-round solutions for the entire plant – meaning the whole network of engines, generators and electrical infrastructure.”
INITIAL PRACTICAL APPLICATIONS Several impressive findings and practical observations of customer benefits have already been made, not least in the field of hydraulics, as Thomas Stütz details: “Most control mechanisms in a turbine are driven hydraulically. The hydraulic pressure this requires is stored intermediately in a hydraulic accumulator from which it is sourced when required. Activity during the filling procedure is analysed very precisely using an ML model in order to guarantee very precise hydraulic system main-
Artificial intelligence (AI) enables new systems to learn autonomously about how a power station can be operated more efficiently.
Mit der Situierung des neuen Krafthauses unmittelbar vor dem Öllschützenspeicher fällt das zuvor bemängelte Schwank-Sunk-Problem weg.
tenance, particularly servicing the hydraulic accumulator. The results facilitate an estimate of bladder accumulator status, whether a nitrogen refill is necessary, or if the bladder is defective.” Another practical example considers the numerous physical forces and conditions that need to be measured and monitored in the course of managing the turbine – such as pressure, temperature, momentum etc. The ML model is trained on the basis of these data, and is able to predict a result using the wide range of input features. “Finally, an anomaly recognition check is run to identify deviations between the predictions and the actual readings. This allows the system to discover problems long before dangerous levels are reached. In turn, in many cases they are problems that can be solved quickly, cheaply, and without the need for the long and expensive downtimes required by extensive repair work”, he rounds off.
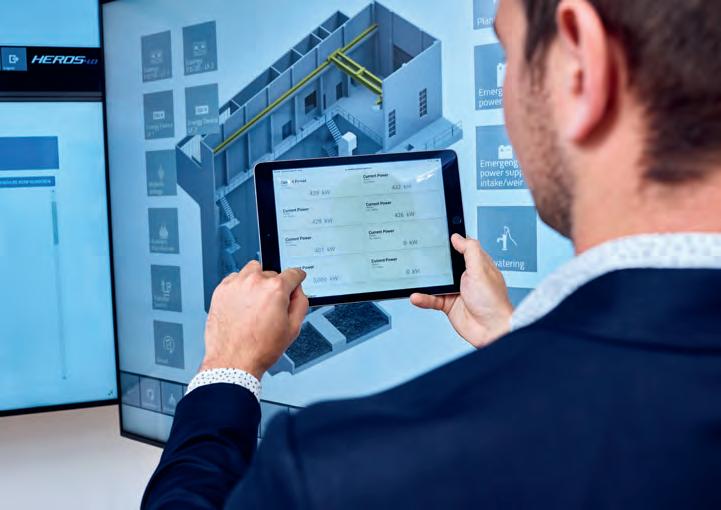
FOUNDATIONS FOR ONGOING DEVELOPMENT As well as being primarily intended for integration into hydropower plant operations, the research findings from machine learning models also deliver core information for the development of Global Hydro products. As Richard Frizberg emphasises: “It’s also importFoto: zek ant for us to learn these lessons within the company in order to continue to refine our own solutions.” One comprehensive example is the need to record and contextualise the entirety of physical forces, torque and momentum levels to which the control mechanisms and generator are subjected, both during normal operation, and in the case of the emergency shut-down of the turbine, since these are generally extremely difficult to calculate. Thomas Stütz details the benefits of ML in such cases: “To enhance calculation methodologies it often makes sense to calibrate readings. In the future, Global Hydro will ensure turbines automatically send these readings to a cloud server where corresponding calculations and visualisations are stored, thus enabling construction designers to use these data to continually improve turbine construction. Furthermore, it will also be possible to use ML models to make meaningful estimates regarding the forces working on, and generated by, similar hydropower systems. More specifically – construction designers calculating the forces and momentums to which a system may be subjected, can compare them with ML model findings and check their plausibility.”
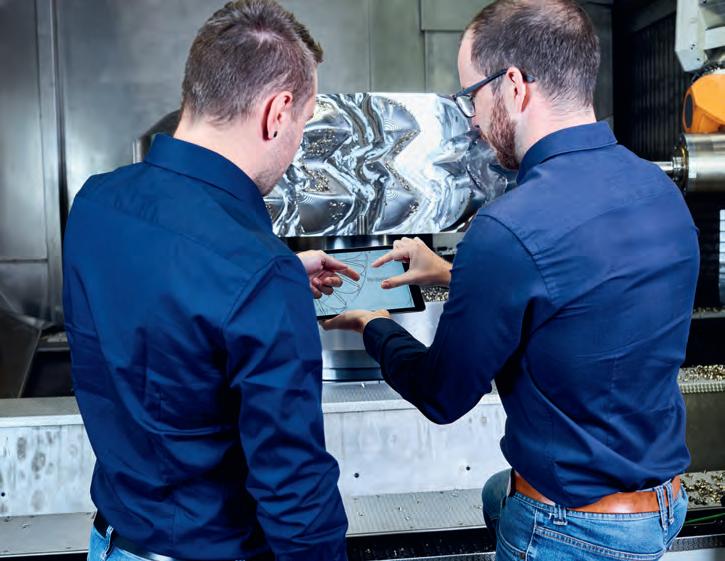
Ultimately, customer benefits lie at the heart of all developments. QUALITY BEFORE QUANTITY Accurate readings lie at the core of effective machine learning. There is no doubt that infrastructure, such as turbines, generators and auxiliary power units, delivers a far greater volume and complexity of data than was previously the case. However, just because more sensors have been installed, this doesn’t automatically guarantee better analyses. GH Head of Electrical Engineering and Software Development, Thomas Stütz, highlights the advantages: “Many of today’s sensors commonly deliver a combination of signals and readings, rather than just one single signal. Around two years ago Global Hydro reprogrammed all its sensors to IO Link technology, meaning the sensors are now hooked up to a software protocol, rather than working with hardware signals like +-10V or 4/20mA. The benefits are that this requires much less wiring and cabling, and that a single sensor can provide far more information.” Has he points out, the main goal is to collect the greatest viable volume of data in the highest possible quality, although there are limits to the amounts of data that can be processed: “Computing times rise accordingly, and the risk of receiving more noise than data can be the result. Data quality is still a greater priority than data volume.”
DEVELOPMENT OF PROPRIETARY SOFTWARE In line with Global Hydro’s general approach, all data are uploaded to a cloud solution as well as being stored at the power station. Ultimately the station requires raw data for local visualisation on power station computers. The team of Upper Austrian developers processes
A vast array of readings is combined to optimise the company’s own products.
Foto: Stockinger
With the help of ML models and the use of comparative data, Global Hydro’s construction designers will soon be able predict the physicalforces hydropower infrastructure can be expected to face.
data in the cloud where all the signals are collected and managed in a central database. “The software for the whole procedure – data generation at the hydropower station, transfer to the cloud via secure channels, and the subsequent analytics, is developed completely proprietarily in Global Hydro’s own software development department. Our main task is to create the models, and the architecture they require, to make them available to customers. We deploy a Microsoft product within the Azur Cloud to manage and analyse data. The platform draws together the entire process chain – from data storage to machine learning-generated event predictions. It also allows us to process almost unlimited volumes of data”, as Thomas Stütz explains.
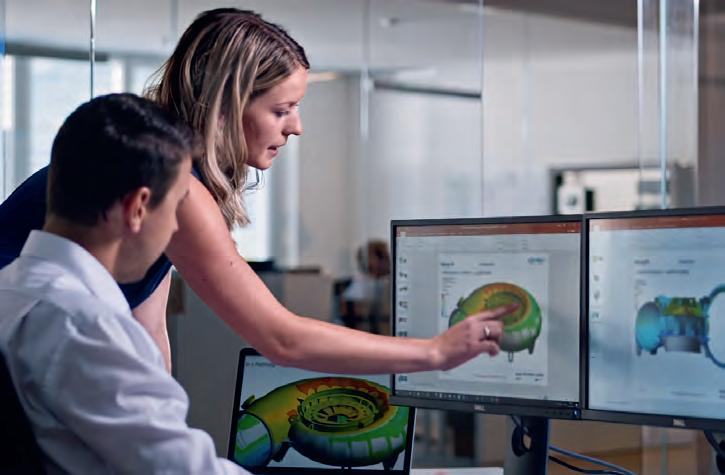
CHALLENGES REMAIN Despite the broad scope of the progress made so far, the digitalisation specialists at Global Hydro still face several challenges. The foremost challenge being the need for automation. Richard Frizberg highlights the issue: “It’s a major challenge in terms of the need to roll out our models causing as little extra work as possible for all our hydropower plants. We expect technical generalisation to be a hurdle for a while. In the end, one key to success will be ensuring all the specialist disciplines work together efficiently like gears. For example, data scientists are not capable of constructing models on their own. This requires experts in other fields, who understand the contexts causing relations between various readings, and who can make the right conclusions.”

PROGRESSING TOWARD MARKET READINESS It already looks like the new ML models will be ready for everyday operation very soon. The notion of them being futuristic is already outdated. Global Hydro is working especially intensively on the market viability of its technology. Richard Frizberg: “The entire control, operation and data conduit technology at the hydropower station is 100% linked up to this technology and the cloud infrastructure has already gone live. All of the machine learning models and data analyses methods are currently undergoing protype testing, and are already in test operation at numerous hydropower plants. We plan to make the first prototype available at the end of 2022. A subsequent test phase will then be followed by final development toward market readiness.” In the interview Global Hydro’s CEO points out that the company has always given R&D a leading role, and that the topic of digitalisation has been a central consideration for technological development for a long time. “Our pioneering power plant management system, HEROS, is further evidence of the fact that we have been leaders in the small-scale hydropower market segment for years. We were among the first people to work with these ideas, and we are taking the same approach toward machine learning, data science and predictive maintenance, digitalisation and automation. They are all highly prioritised at this company.”
READY FOR NEW CHALLENGES The Corona pandemic gave Global Hydro an added incentive to tackle the issue of digitalisation, according to Richard Frizberg, although energy transition is also important in this field: “In the short to medium term power stations will be expected to offer an increasing degree of flexibility, and to communicate and coordinate better with each other. In fact, soon intelligent systems will be essential for the development of hybrid solutions – such as hydroelectrics combined with wind power – or with photovoltaic technology. All this depends on continued developments in the field of digitalisation and on integrating certain aspects of artificial intelligence” Frizberg explains. For many years it has been claimed that hydropower has reached its technological limits and very few developmental steps can still be made. Yet the introduction of machine learning and other digital technologies has opened up a whole new scope for progress – as well as providing real benefits for plant operators. Global Hydro is a pioneer in this field, and is well on the way to setting new standards in small-scale hydropower solutions.
The first prototypes are already being tested: Global Hydro is working hard to get its new ML models ready for the market.