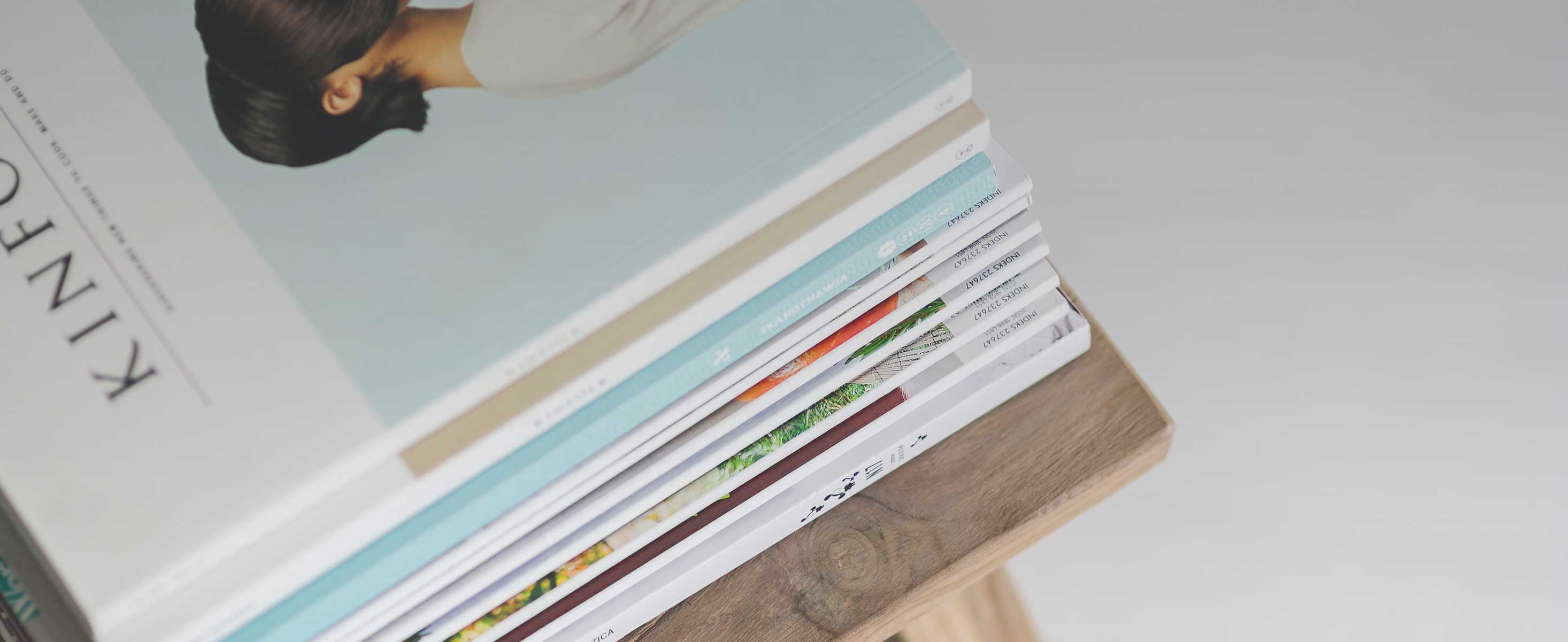
2 minute read
Can AI help us detect solar storms?
By: Dunia Alatoom
The Sun provides the light and energy needed for life to exist on Earth. However, it's also possible for people to encounter risks from our nearest star. Large amounts of electrically charged particles are expelled into space when the magnetic field lines near the Sun's visible surface tangle and break. These "solar storms" have the potential to interact with Earth's magnetic field and seriously affect satellites, radio networks, and power grids, which are essential to our daily lives.
Advertisement
A team of researchers is currently studying an active phase of the solar cycle, which is a cycle that alternates between quiet and active phases every eleven years, and will peak in 2025. Solar storm effects are typically limited to stunning auroral light displays at the poles due to Earth's magnetic field and atmosphere. However, a powerful solar storm could bring the failure of about %10 of all satellites.
Although still in its infancy, the analysis and classification of planetary datasets using artificial intelligence (AI) are gaining ground. Algorithms can be trained using machine learning to analyze vast volumes of data and produce predictions and novel solutions. However, very few tools are specifically designed for this field of study. Over the past ten years, prospective applications of machine learning in planetary science have increased.
The Europlanet 2024 Research Infrastructure (RI) Machine Learning's goal is to mine a large amount of data from space missions, computer simulations, and laboratory tests for knowledge. Europlanet intends to encourage the extensive use of machine-learning technologies in data-driven space research by creating several machine-learning tools that aid planetary scientists in their work.
Solar storms may occasionally travel less than a day to Earth. The relative orientation of the storm's magnetic field, particularly its north-south Bz magnetic field component, determines how much energy is transferred from the solar storm to Earth's magnetic field. The likelihood of a powerful geomagnetic storm that could inflict damage increases with the size of the southbound Bz component. Before a solar storm hits Earth, a group of researchers created a method to help provide prior notice. This technique uses machine learning to forecast the Bz magnetic field component within the first few hours of an in-situ measurement. The program has been trained and tested using information gathered during 348 distinct solar storms since 2007 by the Wind, STEREO-A, and STEREO-B spacecraft.
The next phase of the research will involve using more AI techniques to detect solar storms in real time since they still require human eyes to recognize when a solar storm is occurring. In the upcoming years, satellite missions will produce more data, improving the projections even more. Their prediction tool, however, already demonstrates the enormous potential of machine learning in enhancing space weather forecasting and, in the event of a powerful solar storm, giving impacted areas early notice and minimizing catastrophic damage.