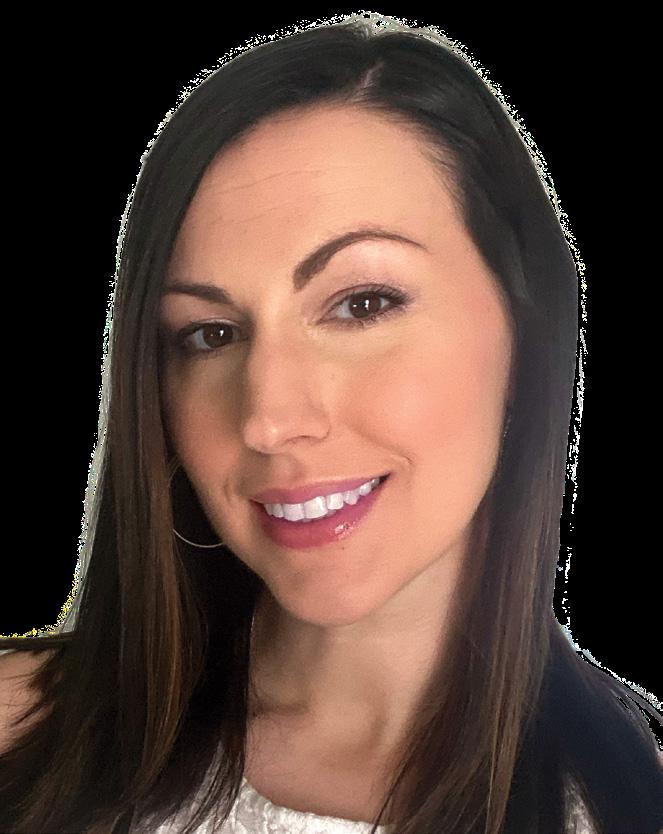
6 minute read
LEARNING
Indeed, not all automation has been helpful, especially when it comes to the intricacies involved with managing documents and data. However, machine learning technology is proving to be the difference maker. Properly applied, machine learning tools can improve and transform automated processes to create greater efficiency throughout the mortgage lifecycle.
As a product information analyst who trains machine learning tools to produce better results, I’ve had the good fortune to experience this transformation up close. And the results are pretty stunning.
Eradicating Document And Data Dilemmas
One of the costliest tasks most mortgage organizations perform is accurately classifying loan documents and extracting data from them. While automation helps, alone it can only do so much. The reason is many documents display information differently, which makes it difficult for automated technologies to classify and index these documents correctly.
Using machine learning technology—aided by human experts— document and data automation tools can “learn” to recognize certain patterns to classify documents and more accurately extract data. This includes the ability to recognize key fields in structured and unstructured documents, such as names, addresses, dates, and other relevant information, regardless of where they are displayed.
Machine learning algorithms are also being used on large volumes of documents to identify patterns, so similar documents can be classified automatically. Once classified, data extraction tools can be used to extract relevant information from these documents at several times the speed of manual, “stare-and-compare” processes. In fact, machine learning algorithms are being trained across such a breadth of document types that contain so much more data than ever before, enabling lenders to process large volumes of loan files quickly and accurately.
FASTER, SAFER LOAN PRODUCTION
When properly applied, the benefits of machine learning technology are tremendous. For example, accurate document classification and loan data verification are critically important when it comes to regulatory compliance. With the help of machine learning technology, critical loan documents can be indexed more accurately so that data can be captured and rules applied to verify missing documents and identify data consistencies, which reduces the risks of fines and penalties.
To get the most out of machine learning, however, the machine must be constantly trained, which requires an analysis of the results so the algorithms can be continually fine-tuned and improved. This is a big part of my role at LoanLogics. My counterparts and I regularly analyze the accuracy of our document classification technologies and make adjustments to improve the results. By doing so, we’re improving the accuracy of our machine learning algorithms over time, which leads to greater accuracy and confidence in the results.
This training process has proven to be highly effective in improving indexing for documents like paystubs and W-2s, which contain a lot of important information, but often have different formats and layouts. The same applies to the many different disclosure documents found in loan files. While it’s relatively easy for automated technologies to classify and read standardized disclosures like the loan estimate and closing disclosure, there are many other types of state and local disclosures that vary a great deal in terms of format, layout, and how information is presented.
In my job, there are certain techniques and training principles that help our machine learning tools learn how to classify these documents. Based on good quality samples, for example, we can review documents in batches and have both machine learning and human operators perform the indexing. At first pass, we can evaluate machine learning confidence scores and where machine learning actually failed. Then, by feeding the results of this human analysis back into the machine, the machine can be trained to produce better results.
Enhancing Secondary Market Activities
While AI and machine learning have made a growing impact on loan production in recent years, they are just now beginning to revolutionize secondary market processes. For example, because of the prevalence of manually driven tasks, the process of selling and onboarding loans is often tedious and inconsistent, making loan trading and onboarding a cumbersome endeavor. The historic lack of loan file data transparency in our industry hasn’t made things easy.
Recently, however, machine learning tools are being applied to secondary market processes that enable smoother trades and loan onboarding. For example, if an originator or investor needs to check 30 critical document types with corresponding critical data elements when selling or buying a pool of loans, they can rely on machine learning to classify documents correctly and then let data extraction and rules-driven data validation tools do the heavy lifting.
With a high degree of confidence, trained machine learning tools can pass or fail these critical documents, ensuring that only accurate classification moves to data extraction and validation steps. Ultimately, this allows loan sellers and buyers to accelerate loan file review times and only focus their attention on managing document and data exceptions. With better data, servicers and investors can gain greater insights into their portfolios and make more informed trading decisions, especially when minimizing inherent data accuracy risks.
Remember The Two Rules
There are a couple of “twists” involved with machine learning tools that make all the difference in their effectiveness. The first twist is that machine learning will produce limited results without a sizable database of documents to train from, including unstructured documents.
When machine learning tools are built on a large enough library, they can help companies achieve triple-digit increases in productivity. So, getting back to Gates’ rule #1 — Before investing in machine learning technologies, understand how the machine is being trained and the depth of sample size being used.
The other twist? Too often, automated technologies create just as many inefficiencies as efficiencies if a lack of confidence persists in the manual processes you are trying to eliminate. There is a science behind machine learning that predictably measures confidence. So, regarding rule #2, it’s important to understand the maintenance and tuning efforts your machine learning vendor is conducting to give your teams more confidence in the technology.
Remember, it takes the proper machine learning tools — and often, the right partner — to truly excel.
As we continue to see more use of machine learning, I recently had the opportunity to ask two mortgage technology experts how it is being used to leverage more data and improve operations. Here’s what they have to say.
LYNNE MCLAIN Account Manager LoanLogics
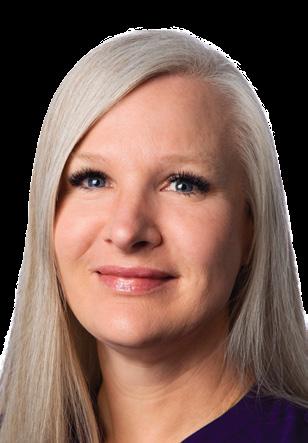
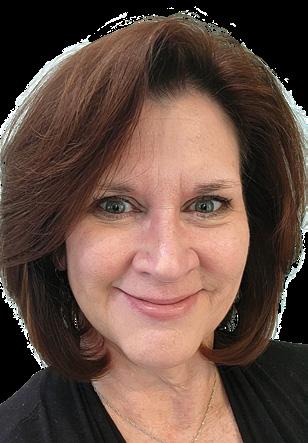
While there are still some mortgage organizations relying on clunky spreadsheets and manual processes, a growing number are embracing automation driven by new machine learning technologies to gain a competitive advantage in the marketplace. At LoanLogics, we’ve seen first-hand how machine learning tools are helping retail, wholesale and correspondent lenders, mortgage servicers, and investors improve productivity and profitability.
One area that is particularly ripe for data and document improvements is whole loan and MSR mortgage loan commerce. Many sellers are selling to many buyers, and there is no consistency on either side of that coin. Document naming, stacking order and data field naming are as varied as the organizations in the market and the loan origination and servicing systems they use. This lack of standardization creates the “heavy lifting” work that persists in the secondary market that continues to slow down transactions and increase costs.
These reasons are why machine learning holds so much promise for the secondary market. By being able to classify documents and extract data more accurately and consistently, regardless of a buyer or seller’s taxonomy, machine learning-driven automation can overcome these hurdles and help create more efficient mortgage commerce. These tools can also accommodate any subset of documents and data that might be appropriate for a given use case, allowing organizations to process once, map once, and use broadly.
Ultimately, the value of machine learning will increase for all of these participants as the breadth of document types classified and data fields extracted grows. I know our clients are just as excited about that happening as we are.
JANET MARTIN Vice President, Digital Solutions Cornerstone Servicing
How are you using machine learning tools to leverage more data to improve operations at your company or for your clients?
Cornerstone Servicing has a series of machines that supply information to improve our overall customer experience and address potential risks.
For example, AI is taking the guesswork out of why customers communicate with us here at Cornerstone. It gives us the ability to identify trends in our customer interactions, which in turn allows us to nimbly design an intuitive digital experience that meets their needs and communication preferences. Our technology analyzes every page a customer views; every conversation with our AI-driven virtual assistant and natural language IVR, and our customer care team members; and two-way email and secured message correspondence. This 360-degree view allows Cornerstone to continuously improve and personalize the experience we create for homeowners. Machine learning tools have also enhanced Cornerstone’s recently launched subservicing business. We are committed to providing our subservicing clients with full transparency into their portfolio and analytics, and we partner with them to apply learnings and take their customers’ experience to the next level. Our servicing platform also enables us to tailor the experience to a clients’ unique branding and messaging, offering consistency and helping to deepen their customer relationships for the long-term. We are also beginning to leverage AI for proactive borrower retention campaigns, which we believe will be a real game-changer. You will see Toby Wells, president of Cornerstone Servicing, mention technology in everything he says because our integrated servicing platform and these machine learning tools are core to our overall strategy. Technology is a big part of what differentiates us as a mortgage servicer. It is helping us get smarter and more efficient across our operations so we can continuously improve our customer experience. n