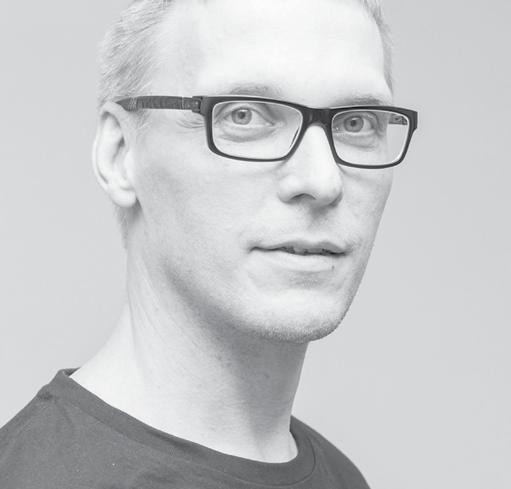
4 minute read
Harnessing the value of data: calculating KPIs
from AMT APR/MAY 2022
by AMTIL
According to a 2021 report by Fictiv, 91% of manufacturers have increased digital transformation investments over the past year. Companies are more aware of the data their facilities produce — but do they know how to use it? Johan Jonzon, CMO and co-founder of Crosser, explores how manufacturers can calculate key performance indicators (KPIs) using edge analytics to make the most of their data.
The message is clear: to be successful in a turbulent market, manufacturers must digitalise. However, collecting data alone isn’t enough. To gain true value from digitalisation, manufacturers must analyse the data collected. They can do this by calculating KPIs, which are measurable metrics that help businesses focus on what’s important. KPIs can track and analyse equipment performance to identify inefficiencies and improve future process runs. Overall equipment effectiveness
Arguably the most fundamental KPI to calculate, and therefore the best place to begin, is overall equipment effectiveness (OEE), which measures an asset’s level of productivity. Some KPIs can be a single measurement, but OEE is actually a combination of multiple measurements. It’s essentially three KPIs in one: availability (A), performance (P) and quality (Q).
A × P × Q = OEE
Availability reflects how long a machine operates out of the total available time. For instance, a machine may have been expected to run continuously for eight hours per day, but in fact only operated for one hour. Thus availability would be one divided by eight, with the result being 0.125.
Availability = Run time ÷ Planned production time
Now we have how long the machine performed for, we can work out how well it performed while it was active — the performance part of the OEE equation. A machine’s performance is measured by the number of units it produced within a given time against the optimal number of units it should be able to produce.
Performance = Number of units produced ÷ Optimal number of units
The final part of the OEE equation, quality, measures the machine’s yield, which is the ratio of products produced that can actually be used without requiring rework or being scrapped.
Quality = Adequate products ÷ Total products
Enter edge analytics
While calculating equations for KPIs such as OEE may seem straightforward, applying them to the plethora of data generated by machines can be a complicated process. Edge analytics serves as a powerful tool to both collect and analyse data from all pieces of equipment and generate KPIs in real time. Calculating KPIs at the edge allows rapid results, as the analysis is performed close to the source of the data. The real-time KPI values generated at the edge can be used to trigger quick action when anomalous behavior is detected. This helps manufacturers to rapidly overcome areas of inefficiency, such as a faulty machine, to ensure productivity is constantly optimised. Alternatively, the data can be sent to on-premise or cloud systems for direct visualisation and/or storage for trend analysis. It’s important to select an edge analytics platform that is low code to make creating data flows for calculating KPIs as straightforward as possible. For instance, the Crosser low code edge analytics platform has a selection of pre-built modules that can be arranged using a simple drag and drop function — removing the need for complex code. Within the Crosser Flow Studio there are also a library of pre-designed data flows (templates) called FlowApps. These FlowApps enable even faster implementation of analytics and KPIs like OEE and others. The platform was designed with simplicity in mind, and its intuitive nature empowers employees across the organisation to be able to work on the platform, without the need for extensive coding experience. An intuitive system
The prebuilt modules can easily be arranged to gather information from data, providing a shortcut to working out KPIs. For instance, a scheduler module can be used to outline when KPI measurements should be active, such as by defining shift hours. The module will generate a ‘start’ message at the beginning of the active period and a ‘stop’ message at the end of it. A message counter module, as the name implies, can count messages received within a given time period. The module can count the total number of messages delivered over the time period, and also how many messages were received for each value of a specified property. For instance, for calculating the quality part of the OEE equation, the module can record how many messages were received reporting an adequate or faulty part. These values could also be used to calculate other KPIs, such as scrap rate. In contrast, a time counter module measures the time a machine has spent in different states by looking at a specific signal such as ‘machine stopped’ or ‘machine running’, measured over a time period. At the end of the time period, the recorded signals are used to calculate the relative time spent in each state. The COVID-19 pandemic has highlighted the importance of productive and flexible processes, causing manufacturers to increase investment in digitalisation. However, to truly transform processes, manufacturers must extract value from the data being collected by calculating KPIs. A low code edge analytics platform can simplify applying many different equations to a whirlwind of data, providing a shortcut to ultimate efficiency and adaptability.