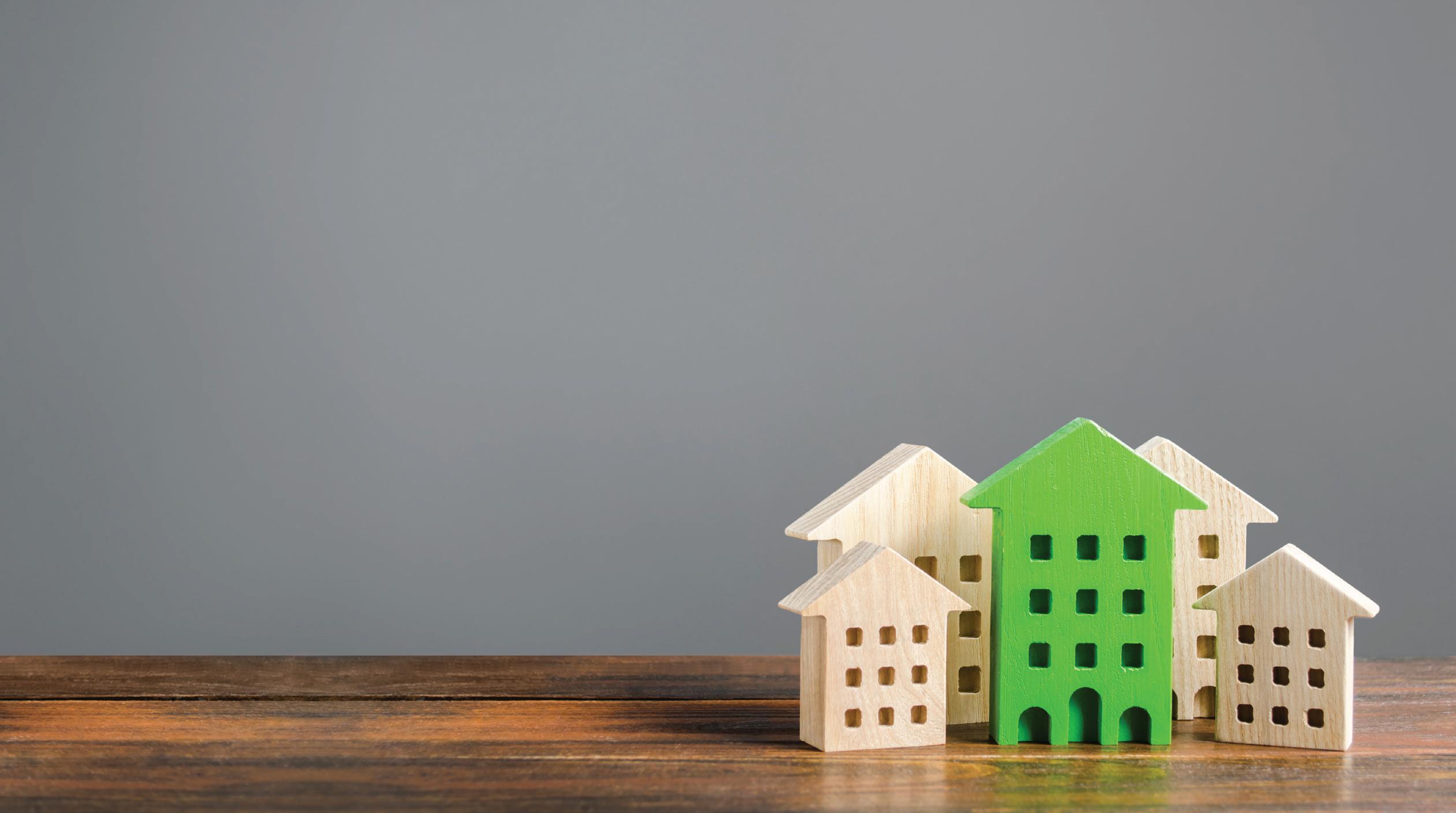
2 minute read
Cognitive Digital Twin: Advanced Building And Energy Management In A Multitenant Building
from AUGIWORLD
by AUGI, Inc.
Abstract
The complexity of modern buildings, consisting of increasingly connected and automated plant networks, is growing exponentially year by year. With this increasing complexity comes the need to manage massive volumes of data for an industry, AECO (Architecture, Engineering, Construction and Operations), that has always had a pronounced gap in digitization (McKinsey Global Institute).
Emerging technologies such as BIM, IoT (Internet of Things), Big Data Management, Data Analytics, and even artificial intelligence could offer new tools for building managers that, when implemented, could enable:
• Optimizing energy efficiency;
• Reduce operating costs;
• Extend the service life of equipment;
• Improve the experience of occupants.
In practice, it would be possible to aspire to the realization of a Cognitive Building capable of supporting building managers in a process of datadriven decision making.
In the prototype I made, a Digital Twin prototype of a real existing (multi-tenant) office building was implemented.
The available data allowed me to investigate the following application areas:
• Space Management
• Energy Management
• Predictive Maintenance
Based on the available data and how it was collected, a workflow was set up, the final output of which is a PowerBI dashboard to dynamically explore each area of the Digital Twin. At an initial evaluation step of the prototype implemented, building managers appreciated the communication effectiveness of the dashboard and felt that their understanding of the building and its behavior would improve if the tool were adopted so as to make their actions more targeted and effective.
Given the effectiveness of the Digital Twin prototype, an evolution of the data workflow has been studied that could make the prototype a fully mature Digital Twin capable of achieving even more advanced levels of autonomy and interconnection.
Introduction
The implementation of a “smart” building as well as its management is becoming a complex activity that requires the integration of increasingly specialized disciplines as the integrated technological components become numerous and diverse.
Modern buildings include a wide range of equipment, from HVAC systems to plumbing, lighting, and security, that all contribute to the buildings’ operation. These elements are increasingly accompanied by some degree of automation so as to provide a modern, responsive experience for the occupants.
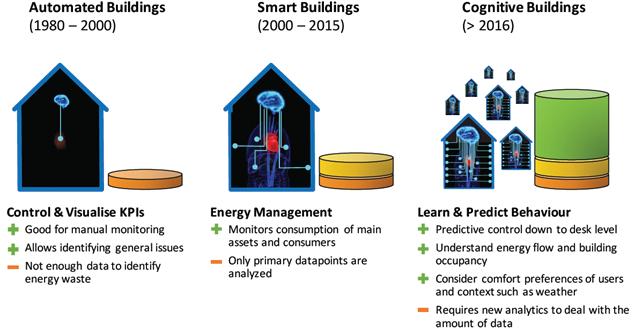
The integration of building management systems coupled with the analysis of the data they generate can allow building managers to gain visibility into complex networks of equipment, sensors, and devices.
Through a thorough process that consists of:
• Data collection and processing
• Identification of key parameters and behaviors
• Forecast generation
• Alert automation
A building manager can “see” what is happening inside a building at any given time and understand the relationships among multiple systems and variables both environmental and generated by user behavior.
Such an evolved approach cannot go without adopting a new concept of Operations that makes extensive use of methodologies such as Big Data, Data Analytics, and Machine Learning.
If skillfully set up, such an approach would give building managers access to a new set of information that would allow them to define more accurate parameters for possible alerts, simplify maintenance, and implement effective automation strategies that: optimize energy efficiency, simultaneously reduce operating costs, extend equipment life, and improve the occupant’s experience. In practice, we could aspire to the realization of Cognitive Building.
Cognitive Digital Twin
The first definition of the Digital Twin was coined in 2003 by Michael Grieves; over time it has been refined and detailed. The following definition from 2017, also by Michael Grieves, will be adopted in this article:
• The digital twin is a set of virtual information constructs that completely describe a potential or actual physical product from the atomic level to the macroscopic level. At its optimal level, any information that could be obtained from inspection of a physical product can be obtained from its digital twin.
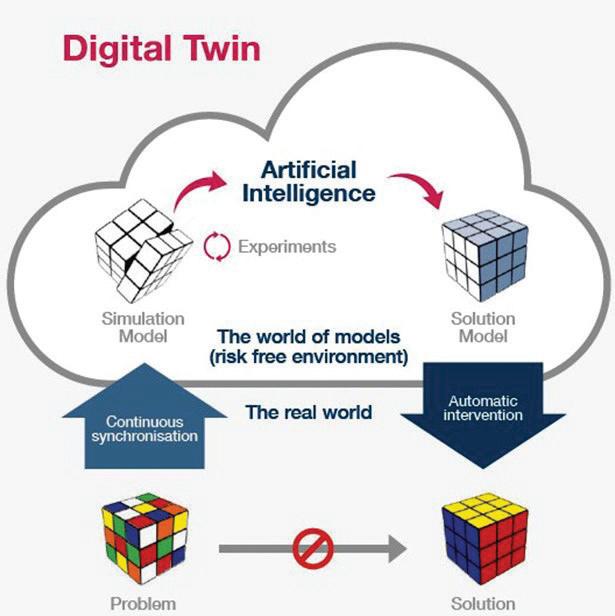