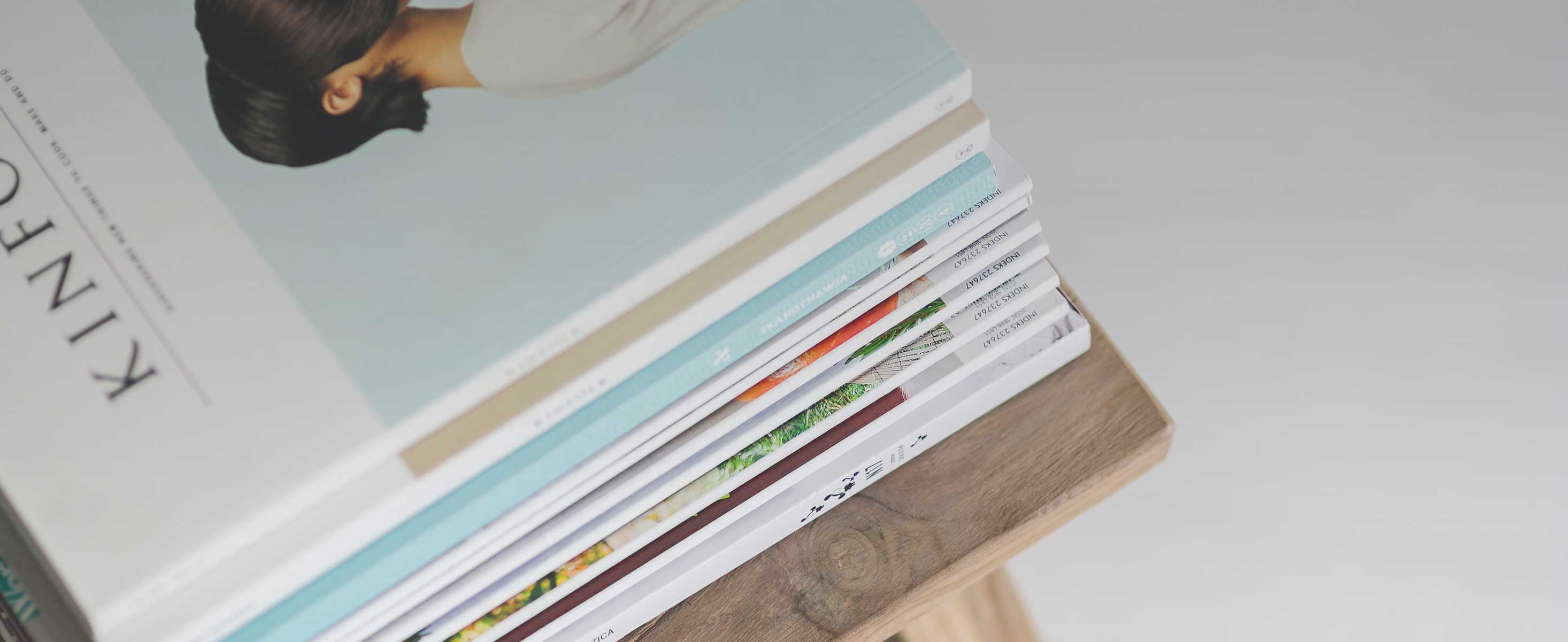
3 minute read
REFERENCES
Alaloul, W.S., Liew, M.S., Amila, N., Abdullah, W., Kennedy, I.B., 2020. Industrial Revolution 4 . 0 in the construction industry : Challenges and opportunities for stakeholders. Ain Shams Engineering
Journal 11, 225–230. https://doi.org/10.1016/j.asej.2019.08.010
Advertisement
As, I., Pal, S., Basu, P., 2018. Artificial intelligence in architecture: Generating conceptual design via deep learning. International Journal of Architectural Computing 16, 306–327. https://doi.org/10.1177/1478077118800982
Babacan, K., Chen, L., Sohn, G., 2017. Semantic Segmentation Of Indoor Point Clouds Using Convolutional Neural Network. ISPRS Annals of the Photogrammetry, Remote Sensing and Spatial Information Sciences 4, 101–108. https://doi.org/10.5194/isprs-annals-IV-4-W4-101-2017
Bier, H., Knight, T., 2014. Introduction: Data-driven design to production and operation. Footprint 1–7. https://doi.org/10.7480/footprint.8.2.807
Bilal, M., Oyedele, L.O., Qadir, J., Munir, K., Ajayi, S.O., Akinade, O.O., Owolabi, H.A., Alaka, H.A.,
Pasha, M., 2016. Big Data in the construction industry: A review of present status, opportunities, and future trends. Advanced Engineering Informatics. https://doi.org/10.1016/j.aei.2016.07.001
Brown, F.E., 1997. Space is the machine, Design Studies. https://doi.org/10.1016/s0142694x(97)89854-7
Chaillou, S., 2019. AI + Architecture.
Cutler, J., Dickenson, M., 2020. Introduction to Machine Learning with Python. https://doi.org/10.1007/978-3-030-36826-5_10
Deutsch, R., 2015. Data-driven Design and Construction.
Engin, Z., van Dijk, J., Lan, T., Longley, P.A., Treleaven, P., Batty, M., Penn, A., 2020. Data-driven urban management: Mapping the landscape. Journal of Urban Management 9, 140–150. https://doi.org/10.1016/j.jum.2019.12.001
Fergusson, K.J., Teicholz, P.M., 1993. Impact of Integration on Industrial Facility Quality. Stanford
University.
Ferrando, C., 2018. Towards a Machine Learning Framework in Spatial Analysis. School of
Architecture Carnegie Mellon University WORKING PAPER 1, 89.
Fiske, J., 2019. Towards Automated Cost Analysis , Benchmarking And Estimating In Construction : A
Machine Learning Approach. https://doi.org/10.33965/bigdaci2019
Geron, A., 2019. Hands-On Machine Learning with Scikit-Learn, Keras & TensorFlow, Hands-On
Machine Learning with R. https://doi.org/10.1201/9780367816377
Goodfellow, I., Bengio, Y., Courville, A., 2000. Deep Learning, CrossRef Listing of Deleted DOIs. https://doi.org/10.2172/1462436
Goodfellow, I., Pouget-Abadie, J., Mirza, M., Xu, B., Warde-Farley, D., Ozair, S., Courville, A., Bengio,
Y., 2014. Generative adversarial networks. Communications of the ACM 63, 139–144. https://doi.org/10.1145/3422622
Hall, D.M., Whyte, J.K., Lessing, J., 2020. Mirror-breaking strategies to enable digital manufacturing in Silicon Valley construction firms: a comparative case study. Construction Management and
Economics 38, 322–339. https://doi.org/10.1080/01446193.2019.1656814
Howard, H.C., Levitt, R.E., Paulson, B.C., Pohl, J.G., Tatum, C.B., 1989. Computer Integration:
Reducing Fragmentation in AEC Industry 3, 18–32.
Hu, Y., Castro-Lacouture, D., 2019. Clash Relevance Prediction Based on Machine Learning. Journal of Computing in Civil Engineering 33, 04018060. https://doi.org/10.1061/(asce)cp.19435487.0000810
Isola, P., Efros, A.A., Ai, B., Berkeley, U.C., 2016. Image-to-Image Translation with Conditional
Adversarial Networks.
Keshavarzi, M., Afolabi, O., Caldas, L., Yang, A.Y., Zakhor, A., 2020. GenScan: A generative method for populating parametric 3D scan datasets. arXiv 1, 91–100.
Ma, G., Liu, Y., Shang, S., 2019. A building information model (BIM) and artificial neural network (ANN) based system for personal thermal comfort evaluation and energy efficient design of interior space. Sustainability (Switzerland) 11. https://doi.org/10.3390/su11184972
Mansouri, S., Akhavian, R., 2018. The Status Quo and Future Potentials of Data Analytics in AEC/FM:
A Quantitative Analysis of Academic Research and Industry Outlook. Construction Research
Congress 2018: Infrastructure and Facility Management - Selected Papers from the Construction
Research Congress 2018 2018-April, 90–100. https://doi.org/10.1061/9780784481295.010
Mehyar, M., Rostamizadeh, A., Talwalkar, A., 2018. Foundations of Machine Learning, second edi. ed.
Massachusetts Institute of Technology All.
Nauata, N., Chang, K.H., Cheng, C.Y., Mori, G., Furukawa, Y., 2020. House-GAN: Relational
Generative Adversarial Networks for Graph-Constrained House Layout Generation. Lecture Notes in Computer Science (including subseries Lecture Notes in Artificial Intelligence and Lecture
Notes in Bioinformatics) 12346 LNCS, 162–177. https://doi.org/10.1007/978-3-030-58452-8_10
Newton, D., 2019. Generative Deep Learning in Architectural Design. Technology Architecture and
Design 3, 176–189. https://doi.org/10.1080/24751448.2019.1640536
Ramsgaard Thomsen, M., Nicholas, P., Tamke, M., Gatz, S., Sinke, Y., Rossi, G., 2020. Towards machine learning for architectural fabrication in the age of industry 4.0. International Journal of
Architectural Computing 18, 335–352. https://doi.org/10.1177/1478077120948000
Russell, S.J., Norvig, P., 2011. Artificial Intelligence. A Modern Approach. Alan Apt.
Sackey, E., Tuuli, M., Dainty, A., 2015. Sociotechnical Systems Approach to BIM Implementation in a
Multidisciplinary Construction Context. Journal of Management in Engineering 31, 1–11. https://doi.org/10.1061/(asce)me.1943-5479.0000303
Sawhney, A., 2020. A Proposed Framework for Construction 4 . 0 Based on a Review of Literature. https://doi.org/10.29007/4nk3
UCL, 2021. Space Syntax [WWW Document]. URL https://www.spacesyntax.online/
Vandegrift, R.A., 2020. Construction 4.0 An Innovation Platform for the Built Environment, Health facilities management. https://doi.org/10.4324/9781315697550-32
Zhou, J., Cui, G., Hu, S., Zhang, Z., Yang, C., Liu, Z., Wang, L., Li, C., Sun, M., 2020. Graph neural networks: A review of methods and applications. AI Open 1, 57–81. https://doi.org/10.1016/j.aiopen.2021.01.001
This page is intentionally left blank