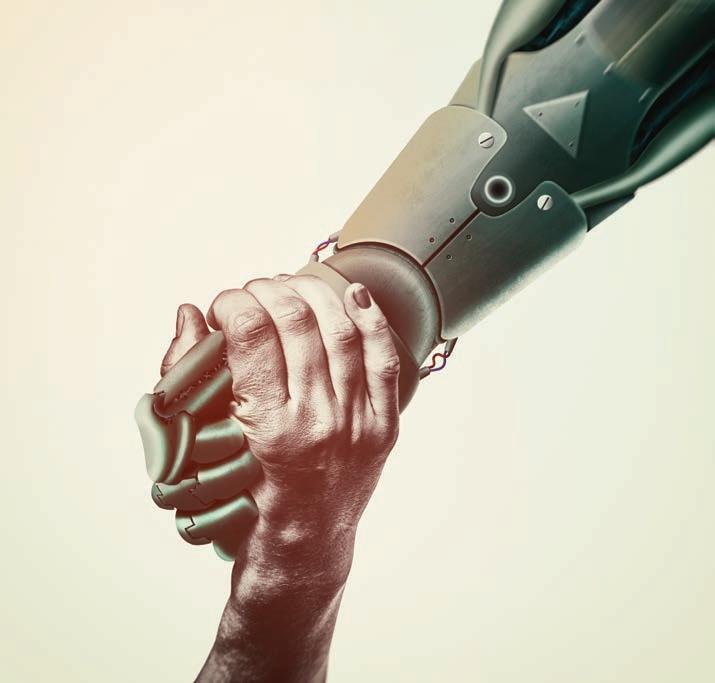
13 minute read
How artificial intelligence is being used to speed up the exploration process and improve the odds of success
How AI is being used to speed up exploration and improve the odds of success
AI TO THE RESCUE
By Alisha Hiyate
As a young geoscientist in the 1990s, Vince Gerrie could never understand why it wasn’t a common practice to collect more data during mineral exploration drilling.
“If you look at oil and gas, they don’t drill a hole without taking physical rock property measurements or sending instruments down the hole to collect in situ data. In the mining industry it’s done, but to a much less degree – many holes are drilled with minimal measurements,” said Gerrie, who went on to found DGI Geoscience, a service provider that collects and interprets data from drill holes, in 1997.
“If you’re spending all this money swiss cheesing the ground, why are you not taking advantage of that opportunity to invest in collecting more information and using that information wisely?”
There are differences between the mining and oil gas sectors that account for the difference in data collection and use, and investment in new technologies that aim to make better use of that data, says Sam Cantor, head of geology at Minerva Intelligence, a Vancouver-based cognitive AI company.
“Even though you’ve got all this modern technology today, you can still walk out there with a rock hammer, a paper map and something as simple as a GPS and find a mineral deposit,” he says. “In oil and gas – without major technology and major initiatives, you can’t really find these deposits anymore.”
That said, why shouldn’t the mining sector leverage the same technology that’s proven successful in oil and gas? That’s where artificial intelligence comes in.
Even with a slow start to embracing artificial intelligence – which encompasses machine learning, automation, and cognitive AI – AI is starting to make its way into mineral exploration, with more companies using machine learning and “explainable AI” to produce better exploration targets, speed up the exploration process, and even act as a virtual consultant.
GoldSpot was started by a team of geologists, including chief operating officer Vincent Dubé-Bourgeois – all with an interest in applying big data to mineral exploration.
“What we’ve seen is that exploration techniques have not evolved at the same rate as exploration tools in past years,” says Dubé-Bourgeois, who learned coding on nights and weekends while doing an MSc. in geology at the National Institute of Research and Science. “We now collect more geological information than ever before – either from portable XRF where you can take a geochemical analysis while you’re in the field and new geophysical surveys that collect more information. But with all this data, geologists can become overwhelmed with the sheer amount of it.”
GoldSpot’s solution uses machine learning to process geological data to discover patterns. Diverse training data, including mineral occurrences, geology and geochemistry data are used to train a machine learning algorithm that can then predict targets. While machine learning algorithms automatically learn on their own, without needing to be further programmed, GoldSpot hasn’t dispensed with geologists and geoscientists. “We try to solve geological problems using data science as well as geological skills,” Dubé-Bourgeois explains. GoldSpot’s domain experts – geochemists, geophysicists, structural geologists, 3-D modellers – are a key part of the process, helping with quality control, interpretation, selecting data to be trained, and validating results.
“We use all their skills and enhance that with data science,” Dubé-Bourgeois says. “An example would be an algorithm with image recognition, we use different deep learning algorithms to classify rocks or structures and data sets.”
Initially, GoldSpot worked with larger companies with producing assets on brownfield targets. But in early 2019, the company started to work with juniors as well.
Dubé-Bourgeois points to the company’s work with Yamana Gold and New Found Gold as its biggest successes.
GoldSpot partnered with Yamana Gold in 2018 at its El Penon mine in Chile to find more new mineralization to leader in applying machine learning to mineral exploration to generate, improve and prioritize exploration targets, and better understand the resource potential of a property. The company, which was a runner-up in the #DisruptMining Challenge in 2017, and went public in early 2019, counts Eric Sprott, Frank Holmes and US Global, as well as Hochschild Mining as some of its biggest shareholders. Its clients include Hochschild Mining, Vale and Yamana Gold.
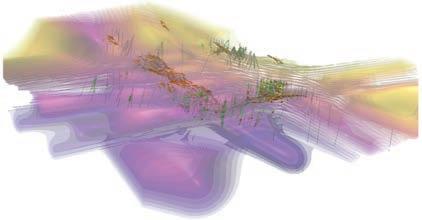
Top: A 3-D structural model predicting structural intensity using a machine learning algorithm. Below: Goldspot’s process. CREDIT: GOLDSPOT DISCOVERIES

extend the life of the mine. Using historical mine data such as drill data, geophysical data, geological and geochemistry data, a predictive lithological map was created of the property that helped speed up target definition.
“We have had multiple successes with them in helping to find new zones and targets that they are currently exploring.”
Yamana has credited GoldSpot’s technology with improving exploration targeting at El Penon, where it has recently added reserves and resources.
Earlier this year, New Found Gold, of which GoldSpot president, CEO and cofounder Dennis Laviolette is also a founder and president, reported its maiden drill hole at its Queensway project in Newfoundland cut 19 metres of 92.86 g/t gold.
The 10 hole drill program tested several targets generated from geochemical, geophysical and structural analysis, combined with machine learning. Starting with an outdated geological map, GoldSpot used additional magnetic and electromagnetic survey data, in combination with field validation, its team of experts, and machine learning to produce a deep learning bedrock map with geophysical targets.
“For some of the juniors, our work can be doing geological mapping for them, where we’d send geologists in the field, validate the targets that we did in our interpretation, and try to collect as much new information as we can to help our model afterwards,” Dubé-Bourgeois says.
GoldSpot has also started a portfolio of royalties and equity stakes from juniors who want to access GoldSpot technology.
The company is also expanding into the Australian market through a partnership with RSC Mining.
Dubé-Bourgeois says GoldSpot has many products in development, including a program that would help relog and reinterpret historical core from pictures. The company is also developing an “outcrop detector” that works with satellite imagery for very early stage exploration.
Minerva Intelligence Vancouver-based Minerva Intelligence was founded in 2017 and went public last May. The company offers four products in its TERRA Mining AI suite for mining clients, including Target, a cognitive AI system that manages exploration data and generates exploration targets, and Driver, which analyzes drill data to extract intelligence from multiple elements.
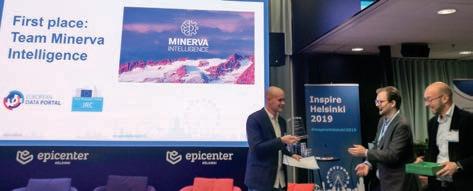
Jake McGregor, COO of Minerva Intelligence accepting first prize for the Helsinki Challenge in 2019. Minerva’s geohazard division designed an application identifying areas in Veneto, Italy, that are susceptible to landslides. CREDIT: MINERVA INTELLIGENCE
Sam Cantor, head of geology for the company, distinguishes between what Minerva does – knowledge engineering or “explainable AI” – and machine learning. Cantor explains that it’s difficult to train an algorithm to identify copper deposits through machine learning, which requires a large training set of data – thousands or millions of examples to learn from. “There aren’t millions of copper deposits for you to train an algorithm on and all these deposits are so different,” he says.
“Instead, you can read PhD theses and top world experts’ writings on what a copper deposit is, how they’re zoned, what they look like, and then engineer that knowledge into a computer that can then take in new data and then compare it against this internal ‘copper expert’ brain you’ve built. Then you don’t need these hundreds, thousands or millions of training examples, you just need that knowledge.”
Minerva’s approach takes different layers of data and turns them into little “chunks” of knowledge, which it calls semantic networks, then compares those against all the mineral deposit knowledge the company has in its database. The predictions that are produced are explainable – i.e., you can see the reasoning behind them, in contrast to the “black box” of machine learning, where you can’t be sure why a certain prediction was made, says Cantor.
Because Minerva takes a different tack focusing on explainable or “cognitive AI,” also called knowledge engineering, the company’s offerings focus heavily on standardizing language, an essential step in preparing data for its AI applications.
Cantor says that geologists and miners often use different terms to describe the same sort of rock or the same phenomenon in the rocks, which makes it hard for other people or computer systems to actually use this data.
“A lot of our services and a lot of our focus is really on getting control on the language of the science. That comes down to standard terminology and one of the things our system allows for is to take a project, a collection of projects, or even a whole portfolio from a company and help harmonize all of the geoscience words that they use so that a description of a copper deposit in Project A is actually comparable to all the geology work in project B.”
Minerva’s Solace data standardization application and its Leo document management and custom tagging systems both focus on language, with both available as software as a service (SaaS).
westpromachinery.com
DESIGN - BUILD - INSTALL - COMMISSION
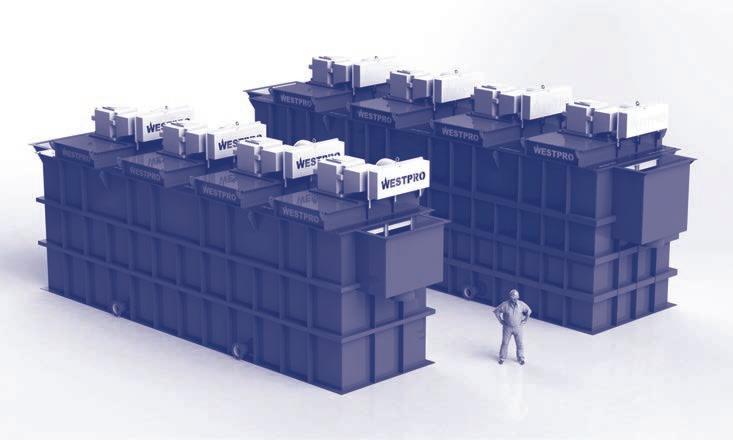
World’s Largest Attrition Scrubbers.
Supplied globally by Westpro. Proven technology providing intense agitation and delivering superior scrubbing performance in a variety of industries.
One of the big benefits of Minerva’s knowledge engineering approach is that it can help alleviate some of the knowledge loss that comes when experienced geologists leave the industry because of a downturn, and can help make up for a reduction in mentorship programs where there would be more knowledge transfer to younger geos. Both are key factors in why exploration programs have become less successful, Cantor says.
“Our type of AI is specifically tuned to capture expert knowledge and retain it in a way that can be used for either a computer system or other geologists,” he says.
“Like an actual expert on the topic, they can look at what you have and say, ‘Hmm, what you’re missing to make this a really good match for this model or that, is this specific piece of information, so you should go out and collect it.’ That’s the same type of advice, given the same way, that a copper deposit expert consultant would give you.”
The Yukon Mineral Targets is a public example of Minerva’s Target product for exploration (yukonmineraltargets.com), and, along with a similar mapping project completed for Brazil, is one of the company’s biggest successes.
The project is an updated version of something that the Yukon government commissioned Clinton Smyth, one of the founders of Minerva who has a background in both geology and computer science, to create 14 years ago. The company updated the program to give potential clients a better idea of Minerva’s capabilities.
The project identified 2,374 exploration targets generated using data from 22,144 stream-sediment samples. The map also identifies terrane, geology and any available mineral data for each target site and compares the target with the deposit types that it best matches.
“People’s reactions to the Yukon Mineral Targets has been really great: we have people come up to the booths at Roundup and PDAC who have properties in the Yukon and say, ‘Your system got my deposit type perfectly right’ – and we didn’t train it on anything, we just used our deposit knowledge. Those have been exciting successes.”
Most recently, in April, the company landed a contract with White Gold, a Yukon explorer headed by Shawn Ryan, who discovered the Coffee gold deposit in the Yukon. The companies will use Minerva’s technology to identify multi-element zones in White Gold’s data, associate those zones with relevant lithologies and structures, and then compare the results with deposits around the world to identify promising drill targets.
Kore Geosystems Rather than exploration targeting, the concept behind Kore GeoSystems is about speeding up and automating drill core logging, and eliminating and streamlining the many tedious data entry tasks associated with it – allowing geologists more time for interpretation.
Kore began as the data analytics division of DGI Geoscience and became its own entity in 2015. Like GoldSpot Discoveries, Kore really got started with the 2017 Disrupt Mining Challenge.

COUNT ON GIW FOR RELIABLE PERFORMANCE.
GIW ® pumps deliver reliable performance in the most aggressive slurries.
GIW Industries is built tough too. We are more committed than ever to providing our partners with an uninterrupted supply of pumps and parts. We will stop at nothing to ensure the success of your operation.
Learn more at www.giwminerals.com.
Simulations: See what you need to avoid operational bottlenecks.
After being crowned as one of the winners of the competition, Goldcorp invested $1 million in Kore, and in less than one year, the company went from a concept and a “crude drawing” on a napkin to a successful deployment of a commercial product, says CEO Vince Gerrie.
Kore’s Spector system is comprised of Spector Optics image capturing system, a machine that core is fed into; Spector Geo visual core logging software, which has an intuitive touch screen interface for rapid data entry; and Spector AI, a machine learning suite of applications that assists with geotechnical logging and rock classification.
In designing Kore’s Spector suite of products, Gerrie visited core shacks of both majors and juniors and asked geologists to identify the most frustrating parts of their work flow. The biggest complaint was that they felt like “overpriced data entry clerks,” he says.
“Our focus is to eliminate a lot of those repetitive mundane tasks and let the geologists spend more time on geology,” he says. “If you can reduce a geologist’s data entry workload by 50%, he or she would have way more time to spend on interpreting data. We want them to be using their brains interpreting and consuming data, not entering data which they barely have time to interpret.”
Instead of taking core photos and manually entering data (core depth, rock type, etc.) separately into another database, both tasks are done at the same time with classifications superimposed directly on the images with Spector Geo. The program also automates depth referencing – another time-consuming task that geologists normally do manually.
By integrating the images and data, and streamlining the process, Kore estimates its Spector suite of products reduces data entry time for geologists by 90%.
“We can now present them with an image – they just click on the rock and click on the legend and it’s classified. They don’t have to type in depths – all the images are depth referenced so if you touch the rock image, it will tell you what depth it is downhole.”
The data is uploaded to the Cloud and available in real time, anywhere in the world, facilitating collaboration and remote work – an attractive option during the current COVID-19 pandemic.
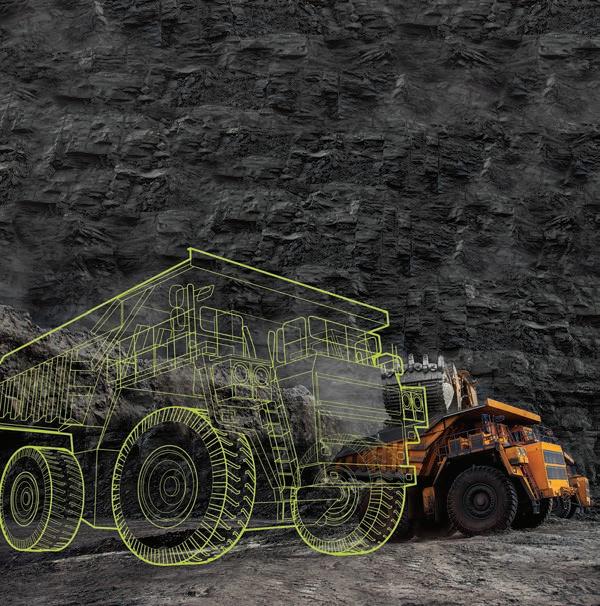
The system also speeds and automates the process of RQD logging, where a geologist assesses the competence of the rock from a rock mechanics perspective, and references it to the core image. The Spector Geo system makes the RQD calculation over top of the image – so it’s possible to go back to the image later and see where all the interpretations and calculations were