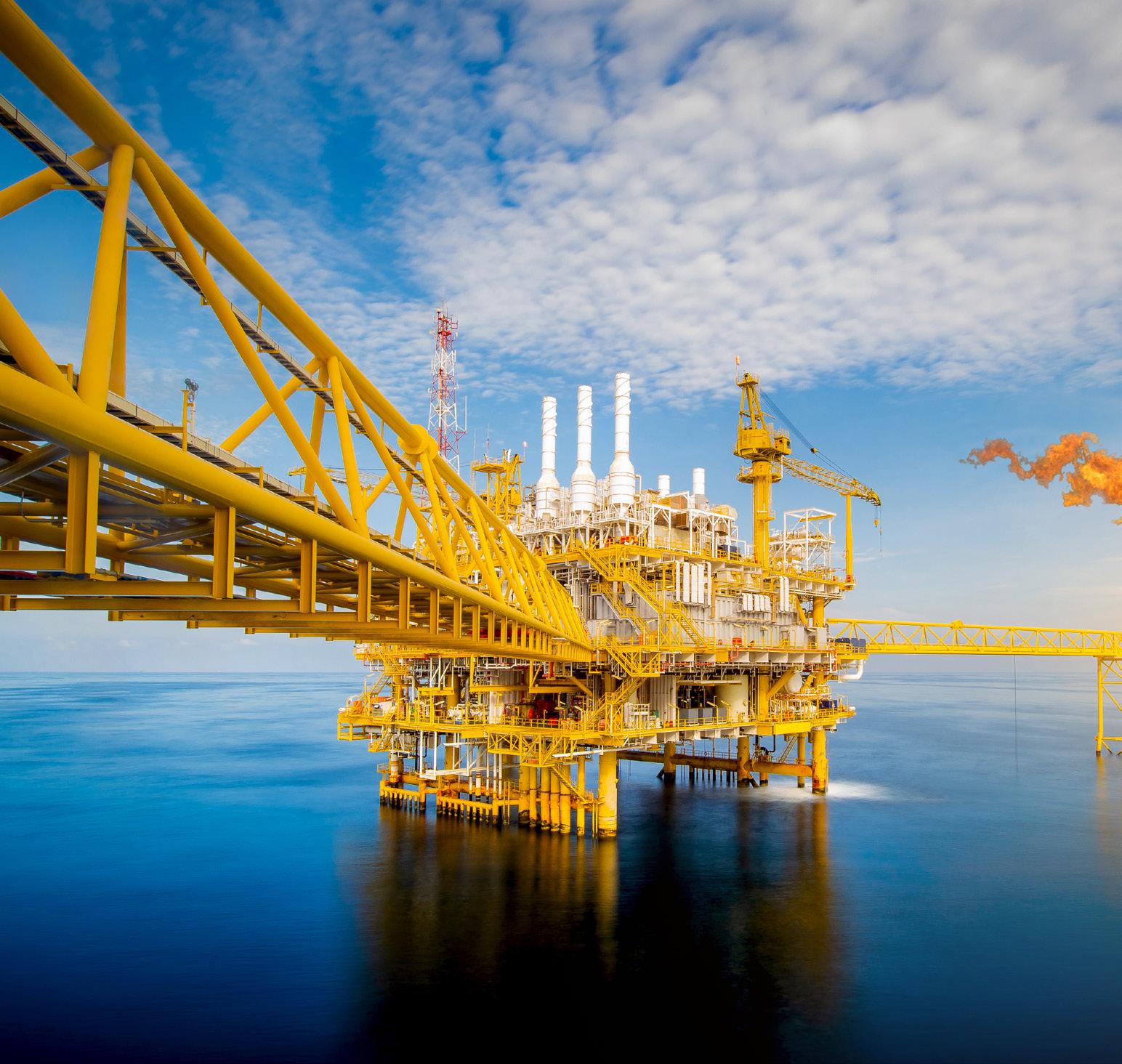
4 minute read
Transforming the Future of Work in Energy: From Buzz to Business-as-usual?
AI and its compatriots—including natural language processing (NLP), large language models (LLM), hybrid machine learning (ML), and generative AI—comprise a formidable team of transformational technologies.
Within the broader energy industry, the possibilities are endless:
Faster, more reliable data processing and verification, with more sophisticated data-sharing infrastructures
Connected data sources as a foundation for accelerated innovation and collaboration
Increased autonomy and automated services in operations and maintenance
Integrated physics-based and datadriven digital twin models that together with AI augment human decisionmaking
Improved asset performance management and reliability for plants from upstream to downstream Bi-directional data flows between systems and equipment, with Generative AI enhancing interaction to increase situational and operational awareness
Supply chain transparency and traceability across the energy value chain
Predictive analytics for improved energy efficiency
When we look at the value that can be extracted from a transformed digital strategy driven by AI, it’s easy to envision the short- and longterm benefits that are sure to follow – faster information flows and data processing, less risk, improved communication and accelerated automation. What’s difficult is identifying the path to push past the buzz and embed these sophisticated technologies into future ways of working in a way that makes sense for the industry. How will AI fit in as part of day-to-day operational processes?
As Kongsberg Digital explain It is not just about data and dashboards
We have heard it before that what matters most is not the data you have but how you use that data. Data standards are an important part of our digital future, enabling companies to collaborate and coinnovate through system interfaces that integrate in the back end. Many progressive companies have put in place a solid data infrastructure and added select applications like a digital twin on top, making data more contextualised and accessible through simplified dashboards that make data easy to find, filter and apply.
However, data and dashboards are not enough of a springboard for AI to have the measurable value or ROI that companies expect. Instead, we need to start with a value-focused approach that zooms in on the specific use cases and services where AI can have the most influence through a digital operating model that builds on digital twin technology backed by physics-based and data-driven models. The successful implementation of an AI-infused digital strategy needs to be driven by desired business outcomes.
Driving transformation with a value-focused approach
The ability to identify the areas where AI can evolve into more than business as usual requires a degree of familiarity with today’s complex energy landscape. As a technology provider with domain and technical expertise, we can point to areas where we see the biggest potential for the industry: Safety
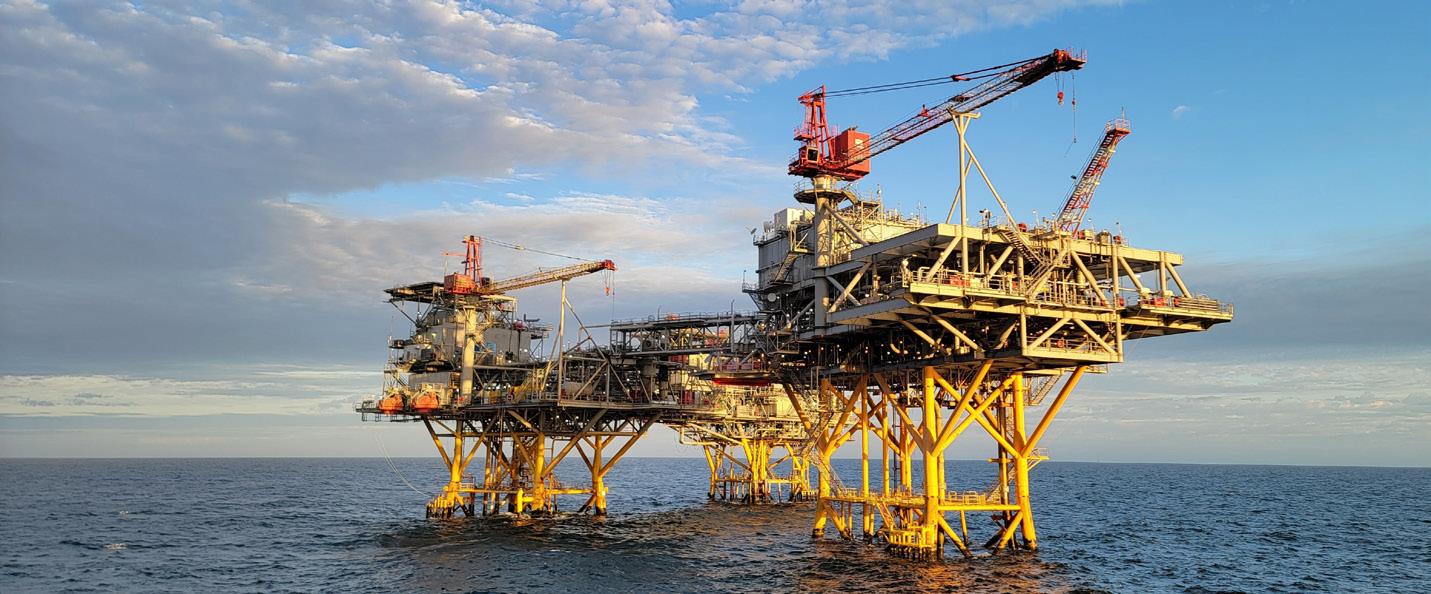
• Operations and Maintenance
• Performance Monitoring Supply Chain Management Design and Engineering
• Emissions Management
Once specific services within these potential impact areas have been identified – typically those that occur frequently and thus create identifiable, repeatable data patterns that can be used for learning and incremental automation – it becomes easier to understand how AI and its cohorts can be used to build out value-driven applications that extract information, process it and provide informed recommendations for actionable items that can be executed independently to contribute to overall improved energy efficiency.
Beyond the buzz, it is important to keep humans at the center of work but augmented by technology that is agnostic to different data types and sources. That way, technology is a supporting function that puts the right information – not too much, and not too little, just what is necessary – in front of the right user to enable decision-making at a faster pace, with lower risk. The cascading effects can be exponential depending on the use case, leading to everything from minimized emissions to earlier intervention on predicted maintenance failures.
A glimpse into the AI-driven future of energy operations
Let us take the example of an emissions management workflow for methane emissions. Imagine an emissions reduction team manages ten assets for a large E&P company, monitoring these facilities continuously to trend both the individual and overall carbon footprint of operations. Their primary work tool: a cloud-based dynamic digital twin, where they can access an emissions management cockpit that has been configured to show the biggest energy consumers at any given time. More than just dashboards, this cockpit shows not only where there are trends towards heightened consumption but also critical incidents that need intervention, along with recommended courses of action based on continuous real-time data feeds and informed by historical and synthetic data.
The cockpit shows a main gas turbine in one of the facilities that is consuming more energy than it should, increasing the asset’s overall carbon score. An investigation kicks off, and the team dives into the data available in the twin. Using a built-in AIpowered chat function, they make a few queries to locate the correct information in just a few clicks.
Armed with these insights, they fly to different locations virtually, looking at the flare stack or vents to identify the troublesome consumer. Data flows back and forth behind the scenes, masking the complexity of these queries. The team investigates the simulator view, seeing simulated versus actual values to get prescriptions on where to intervene. With these prescriptive outputs, they have exactly what they need: instructions on what to do and the reasoning for why.
Transforming the future of work in energy
Data turns to insights, insights turn to actions, actions turn to outcomes, and outcomes turn to prescriptive tasks that the team can execute with full transparency into the reasoning process – for almost any use case driven by business needs and expected value-based outcomes. Some customers are already starting this journey. It’s better than business as usual.