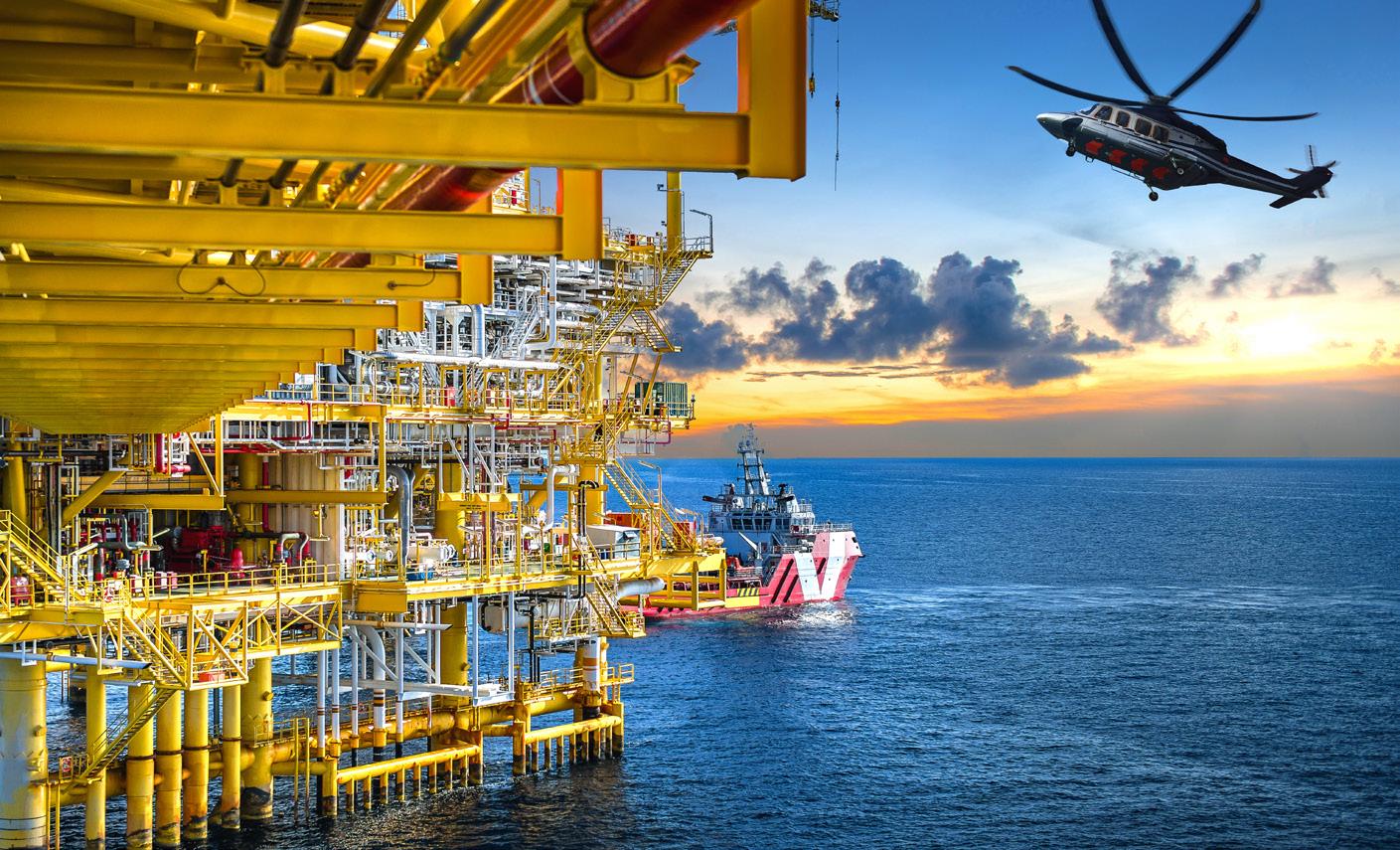
3 minute read
Walking the data tightrope
spotlight. Rob
The last couple of years has seen most of the oil and gas majors scaling digital twin solutions across their global assets. In addition to creating a data foundation for their assets, these digital twins are providing insights that de-risk project delivery, reduce maintenance burden, safeguard production, and reduce emissions.
When you consider that operators’ portfolios contain a mix of new, recent, and aging assets, with some even delivered in the days when paper copies were the norm, it is no surprise that this widespread adoption of digital twins has started to highlight numerous data challenges.
While the industry has generally been effective at managing dynamic operational data, for example by utilising process data historians and maintenance management systems, it is the lessdynamic engineering and asset data, 3D models and reality capture data that are proving to be the most challenging.
Standards only get you so far
The industry is collaborating to develop and mature data standards and interchange formats. Not only do these standards enable a level of consistency and predictability across the supply chain, but they also enable operators to decouple data from authoring systems and proprietary solutions.
Current data standards are tailored to capital project delivery and the subsequent handover to operations, but not the operating phase itself. They define the data to be collected for each type or class of equipment, with the aim of achieving a complete and compliant dataset at the point of handover. But trying to sustain this level of data quality through decades of operations is impracticable.
Finally, while data handover standards contain attributes to indicate the criticality of certain equipment, a framework for applying similar criticality ratings to individual data attributes is currently lacking.
Considering data criticality
The operations phase requires an unwavering focus on keeping people safe, reducing emissions and minimising expenditure, all while maximising reliability and production. In this context, it’s not always apparent what data is the most critical. Is it the data that can enable the greatest potential impact on the bottom line? Or the data that can help you to mitigate a risk? Or is it the data that could have the greatest potential consequence if it were incorrect and led to an incorrect decision?
The criticality of data will change depending on the particular asset, the lifecycle phase, ongoing activities, and risks being managed. For example, on a recent project Wood deployed a reliability solution that supported removal of over 75,000 hours of maintenance, but this solution was wholly dependent on us integrating crucial sampling and monitoring data from ten standalone systems.
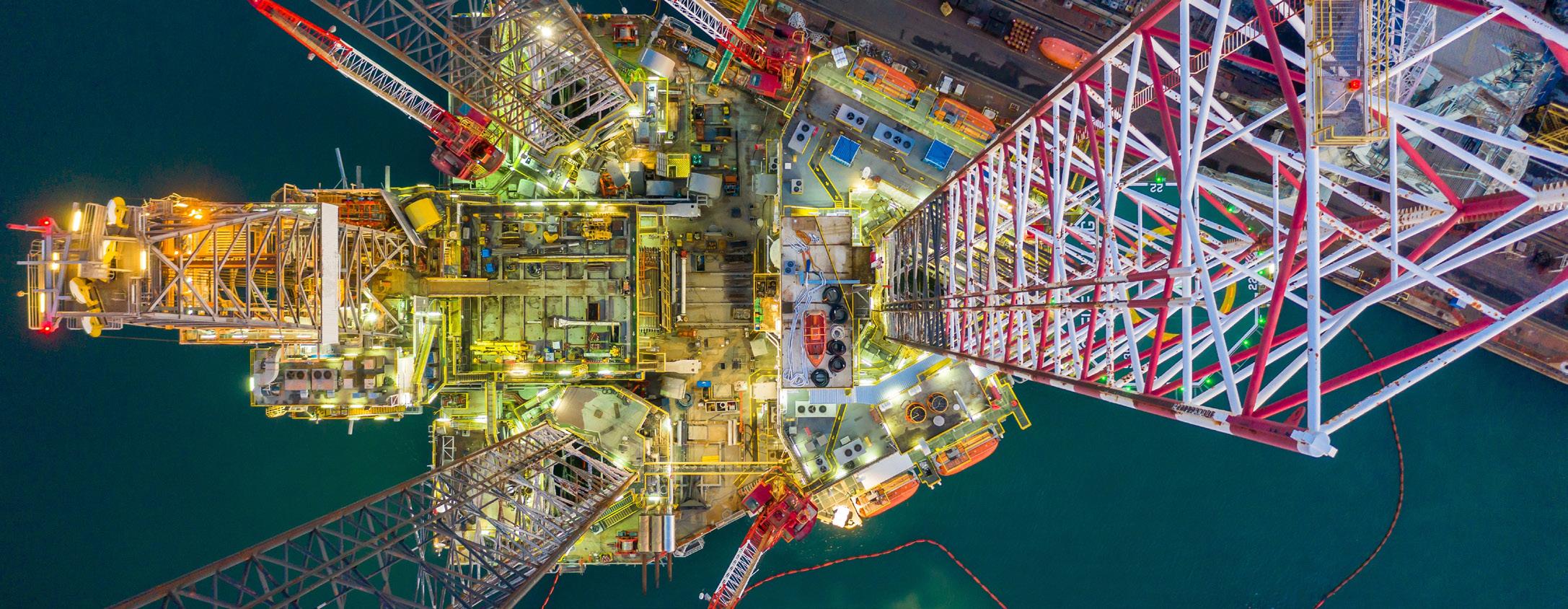
Given the dynamic nature of operations, it is simply not practicable to foresee all the data you may need and to know it will be up to date all the time. But might it be possible to define and focus efforts on a core set of data most critical for asset operations, and likewise identify other data sets as less critical?
Maintaining and sustaining digital assets
Current industry philosophies towards data maintenance are varied, ranging from the blanket approach of striving to keep all data up to date, to extremely targeted approaches that only update data on request when it is needed. Anecdotally, regardless of the approach there is still a widespread challenge with keeping critical data up to date, particularly when changes are made to the physical asset.
Just as how physical assets are maintained, might it be beneficial to take a more balanced approach to maintaining digital assets that strikes a balance between criticality, reliability, the potential consequences of failure, and cost?
Reframing users’ relationship with data
Digital twin users are exposed to large amounts of data, information, and insights. While only some of it influences decisions about how to operate and maintain the asset, our experience shows that any data errors (even those that are not critical) can cause user distrust of the entire digital twin.
To support a more balanced approach to data management, it is imperative that users have visibility of the provenance of each piece of data, including when it was last updated and validated, enabling them to gauge its reliability. We have found that supporting this with a process that enables users to query data and request updates helps to build trust and gain buy-in from users, enhancing digital twin adoption.
Conclusion
Digital twin solutions are a powerful way for organisations to gain insights, improve decision-making and optimise the operation and maintenance of their assets. However, realising these potential benefits not only requires the right technology and data, but also needs operating teams to integrate them effectively into their work.
At Wood, we take a transformation-led approach to digital twin that combines decades of project delivery and operating experience with deep domain knowledge and digital expertise. Moving to the balanced data maintenance approach outlined in this article requires this clear understanding of users, the jobs they perform and the decisions they need to make.
Just like we do on their physical counterparts, we need to focus the maintenance of our digital assets on the areas that have the biggest impact on safety, risk, reliability, and the bottom line. Only then can we sustain digital twins and the value they deliver over the long term.
Digital twin is just one of Wood’s core digital solutions that we are applying to transform industry. Discover how we can solve your data challenges and decode your digital future: woodplc.com/solutions/digitalisation