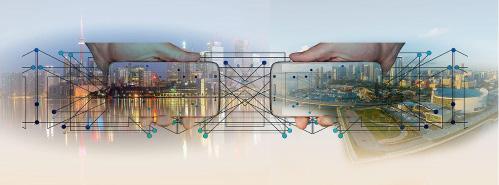
14 minute read
Stephen Adjei '25
Passive Sensing to Prevent Mental Health Issues during the COVID-19 Pandemic
BY STEPHEN ADJEI '25
Advertisement
Cover Image: Passive data sensing tools can measure behavior in individuals through the real-time collection of personal data Image Source: Pixabay
Introduction
Smart devices are now helping our healthcare system address one of the most significant challenges currently plaguing psychiatry: the difficulty of accurately labeling disease phenotypes (Torous et al., 2016). Recent developments in smart devices and wearable technologies such as smartphones allow for realtime data collection, effectively transforming smart devices into health records. Today, the healthcare system can utilize data collected from these devices to provide personalized diagnostics and proper preventive treatment. (Torous et al., 2016). Critically, this technology can enable health professionals to aid children and adolescents struggling with mental health issues during the pandemic by identifying early indicators of worsening mental health (de Figueredo et al., 2021).
This report will address both the benefits and issues associated with passive data sensing and defend an argument for its implementation. This article will outline how this technology has helped youth during the pandemic and discuss studies that demonstrate its effectiveness. Lastly, this article will address ethical issues and discuss measures the government and the healthcare industry can take to address concerns.
Passive Data Sensing
Passive Data Sensing (PDS) is the indirect acquisition of data about a person's or group's activity at a specific location in real-time. Passive data sensing tools can measure behavior in individuals through the real-time collection of personal data, which is referred to as digital phenotyping (Martinez-Martin et al., 2021). Digital phenotyping is essential to healthcare. The analysis of sleep patterns, movement, social media activity, and more can predict relapses in schizophrenia, depression, and mania, among many other mental illnesses.
The concept originated from research in the computer science field regarding 'contextaware' computing. Context-aware devices monitor changes within the environment, such as location changes, lighting, network connectivity, and noise level (Schilit et al., 1994). These devices interact with their primary users and other context-aware computers within a specified range and vicinity (Schilit et al., 1994).

Context-aware devices are beneficial because they collect, analyze, correlate, and disaggregate data for third parties.
Before using passive data sensing, researchers and psychologists typically assessed depressive symptom severity by administering questionnaires to patients. These questionnaires are distributed daily to individuals suffering from depression to allow them to self-assess their depressive symptoms. One of the most common self-assessments is the Patient Health Questionnaire (PHQ). There are two major PHQ assessments used in research: PHQ-8 and PHQ-9. PHQ-8 helps assess the depression level of a large group of people by determining how often specific depressive symptoms are scored highly among members of that group (Dhingra et al., 2011). The PHQ-9 is similarly used to assess depression but includes one question to ascertain suicidal tendencies.
Questionnaires like PHQs, however, can become tedious to administer daily. Moreover, those administering the surveys cannot be sure that patients will complete the survey with objectivity. On the other hand, PDS allows for data collection without any effort or input from patients (Cornet et al., 2018). In short, PDS reduces the burden of data collection in medical research and provides more accurate and relevant input (Cornet et al., 2018).
In the 2010s, medical researchers utilized smartphones to conduct studies on the clinical applications of passive data collection (Torous et al., 2016). A 2015 report from the International Business Times states that, at the time, there were more smartphones in use than there were people on earth, with 64% of US adults and 85% of US adolescents, ages 18-25, owning smartphones (Torous et al., 2016). Smartphones, the optimum context-aware device for PDS, contain many sensors and software services that help researchers collect data about users' activity, location, and behavioral patterns. Today, researchers use a wide variety of sources to collect data in this field. A user's calling, texting, and social media activity, for example, can be utilized to capture specific characteristics of the user's social and mental health. This information can also be used to establish correlations between mental status and expressed needs (Cornet et al., 2018).
Machine Learning Paired with Passive Data Sensing
The use of machine learning models, unique algorithms designed to analyze large data sets, in PDS studies has improved the ability to create prognoses for users (Jacobson et al., 2021). In 2014, Dartmouth's Rui Wang and a team of researchers assessed the effects of daily and weekly workload on the consequential stress levels and behaviors of 48 Dartmouth undergraduates through a smartphone app and machine learning models. To assess changes in sleep, activity, mood, and sociability, the team developed an app called StudentLife (Rui et al., 2014). StudentLife collects data from sensors contained in Android smartphones, including light sensors and the phone's accelerometer. Researchers also used mobile software like the Google location service and usage data services like Samsung Digital Wellbeing. Machine learning was instrumental in the StudentLife team’s ability to determine sleep patterns. The team implemented a method developed in 2013 called the Best Effort Sleep Model. This algorithm predicted sleep duration using smartphone usage patterns such as the length of smartphone usage, light sensors to detect prolonged instances of darkness, and
Image 1: Smartphones, the optimum context-aware device for PDS, contain many sensors and software services that help researchers collect data about users' activity, location, and behavioral patterns.. Image Source: Pixabay
Image 2: The use of Machine learning models, unique algorithms designed to analyze large data sets, in PDS studies has improved the ability to create prognoses for users (Jacobson et al., 2021) Image Source: Pixabay
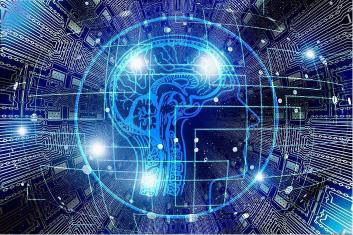
the microphone to detect long periods of conversation. They then aggregated data from these sources to determine students' need for counseling and support.
Although machine learning algorithms expedite the data collection process, its accuracy has been disputed. A team of psychologists and computer scientists from Dartmouth led by Professor Nick Jacobson utilized passive sensing data collected over 18 years to predict Generalized Anxiety Disorder and Personality disorder and their model yielded predictions with 68.7% accuracy (Jacobson et al., 2021). A team of researchers from Singapore experienced similar problems. They used a system called StressMon to collect activity data from 108 students via Wi-Fi. This system was created as a possible "detection solution" that utilized non-intrusive methods to identify highly stressed and depressed individuals in work settings (Camellia et al., 2019). In a two-year-long study, the team conducted three longitudinal studies with different groups of students, in which stress and depression questionnaires (PSS-4 and PHQ-4) were administered to participants every three days. The results, however, were lackluster; their machine learning models could not surpass an Area Under the Curve (AUC) value of 0.67, meaning that the model successfully predicted students as stressed only around 67% of the time (Camellia et al., 2019).
Researchers are now reflecting on how the data collection process may have influenced their findings. Dartmouth researcher Jacobson and his team captured data in three phases over a 17 to 18-year interval and now find that gaps between their collection phases may have led to more subtle changes in depressive symptoms (Jacobson et al., 2021). Perhaps improving continuous data collection processes, rather than altering currently used machine learning models will help fine-tune the results.
The COVID-19 Pandemic and Mental Health
Disruptions in social activities and increasingly stress-inducing conditions contribute to psychopathology development in young children (de Figueredo et al., 2021). Several studies suggest that social isolation increases the risk of developing depression and age-related health problems in adulthood and negatively affects dieting patterns (de Figueredo et al., 2021).
There are many well-established correlations between social isolation and mental health problems. In 2009, a team of UK researchers studied the correlation between adverse childhood psychosocial experiences, such as social isolation and age-related diseases. The team found that socially isolated children were more likely to become depressed. Similar correlations were also discovered in maltreated and socioeconomically disadvantaged children: the UK researchers found that 32 year old individuals with more severe adverse childhood experiences may struggle with 59-73% more agerelated disease risks than individuals with less severe experiences (Danese et al., 2009).
The team also found that predicting age-related health complications using childhood experience was more accurate than using smoking history, physical inactivity, and poor dieting at 32 years old (Danese et al., 2009). Children who have experienced socioeconomic disadvantages and maltreatment may be more at risk of developing depression or other mental health problems in adulthood (Danese et al., 2009).
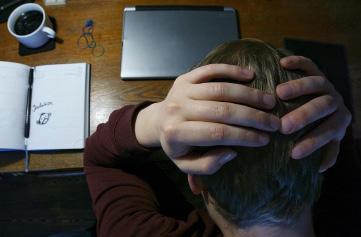
The pandemic offers opportunities to expand passive data sensing, alongside machine learning and statistical learning models, to help detect early signs of mental health issues in children and adolescents. These data collection and analysis methods have already been used in studies conducted shortly before the pandemic and have proven benefits (Huckins et al., 2020).
The StudentLife study, for example, was conducted during Dartmouth’s first academic term impacted by the pandemic – the 2020 Winter Term (January 4th to March 13th). Beginning February, students' daily lives were disrupted by many unexpected changes, including requests to social distance, vaccinate, mask up, and leave their campus dorms (Huckins et al., 2020). On February 4th, students returning to Dartmouth from China were required to isolate for 14 days. On March 11th, college-sponsored athletic competitions were canceled after the World Health Organization declared COVID-19 a pandemic. Moreover, on March 17th Dartmouth terminated all inperson, on-campus options to attend classes from March 28th to June 3rd (Huckins et al., 2020).
To assess the impact these changes had on undergraduate students" mental the team conducted a 10-week long-term study. The app uploaded anonymized data from smartphone sensors such as the GPS, lock/unlock status, and the accelerometer to a server and monitored behavior, sleep, and social activity changes in daily and weekly intervals (Huckins et al., 2020). The scientists found noticeable differences in behavior and mental health towards the end of the Dartmouth 2020 winter term - February 15th to March 25th (Huckins et al., 2020). Measurements of student inactivity increased, and self-reported anxiety/depression symptoms spiked during the last week of the term - March 8th to March 13th . The team also discovered that sedentary time, anxiety, and depression symptoms increased from February to March (Huckins et al., 2020). As a result, the team informed: "public health officials and individual citizens to raise public awareness about the benefits of aerobic exercise and unplugging from technology (moderating phone usage)." These activities have been "shown to have positive effects on alleviating anxiety and depression" (Huckins et al., 2020).
Ethics of Passive Sensing
While passive data collection requires consensual agreement, considerable measures must be taken to address patient privacy, thirdparty data acquisition, and usage. The healthcare sector requires more clearly stated regulations before utilizing such data to inform decisionmaking and patient services (Martinez-Martin et al., 2021).
When data collection takes place outside the healthcare sector and instead on consumer devices, digital phenotyping could capture health information outside the Health Information Portability and Accountability Act (HIPAA) (Martinez-Martin et al., 2021). HIPAA specifies that data containing personal "identifiers" should only be shared with third parties when used for health-related operations like treatment. However, this enables deidentified data, where data such as names and ages are removed, to be shared with third parties without restriction. With many nonhealthcare companies aggregating patient data, companies or outside parties could use data to link information to individuals (Martinez-
Image 3: Several studies suggest that social isolation increases the risk of developing depression and age-related health problems in adulthood and negatively affects dieting patterns (de Figueredo et al., 2021).
Image Source: Pixabay
Image 4: HIPAA specifies that data containing personal "identifiers" should only be shared with third parties when used for health-related operations like treatment.
Image Source: Pixabay
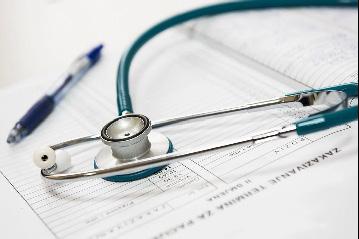
Martin et al., 2021). For instance, a user's IP address could be an identifier to third parties. Moreover, current regulations in other countries, such as the European Union's General Data Protection Regulation Act, do not address or protect individuals from companies aggregating health-related information, enabling companies to "draw health inferences from personal data" (Martinez-Martin et al., 2021).
A 2021 Stanford University study attempted to identify the most significant concerns surrounding digital phenotyping. The team implemented a modified version of the Delphi method: a technique in which field experts in mental health law, ethics, and data science respond to questionnaires and analyze them to reach a reliable consensus (Dalkey et al., 1963). The study showed strong favor among all 24 participants to develop new security and privacy guidelines for HIPAA that address personal health data collection occurring outside its current authority. Additionally, clear communication to patients and users about the risks and benefits of passive data collection was deemed essential (Martinez-Martin et al., 2021).
Many other issues were also addressed in this study. On data re-identification, the Delphi participants suggested existing frameworks should never consider data as "non-identifying." With the advent of massive public databases, personal data is always at risk of being traced to a specific person or group (Martinez-Martin et al., 2021).
Conclusion
Passive data sensing (PDS) removes complexities in data collection by enabling real-time data collection. By analyzing data collected by context-aware devices, researchers can find correlations between trends in the data and mental health deterioration. Today, the ongoing pandemic introduces significant changes and stress-inducing situations in people's lives that may negatively affect their physical and mental health. PDS, therefore, could be an effective way to detect worsening mental health in individuals earlier by analyzing changes in behavior patterns via sensors and phone services. Researchers must ensure that their machine learning models can use the data collected to predict depression with high accuracy to implement this technology. Additionally, implementing passive data sensing and machine learning to predict changes in mental health during the COVID pandemic will be particularly challenging if privacy and transparency. Nevertheless, a collective and concerted effort among healthcare service providers, researchers, and data collectors to establish regulations and protocols for HIPPA compliance is required for a more efficacious implementation of passive data sensing.
References:
B. Schilit, N. Adams, and R. Want. 1994. Context-Aware Computing Applications. In Proceedings of the 1994 First Workshop on Mobile Computing Systems and Applications (WMCSA' 94). IEEE Computer Society, USA, 85–90. DOI:https://doi.org/10.1109/ WMCSA.1994.16
Camellia Zakaria, Rajesh Balan, and Youngki Lee. 2019. StressMon: Scalable Detection of Perceived Stress and Depression Using Passive Sensing of Changes in Work Routines and Group Interactions. Proc. ACM Hum.-Comput. Interact. 3, CSCW, Article 37 (November 2019), 29 pages. https://doi.org/10.1145/3359139
Cornet, V. P., & Holden, R. J. (2018). Systematic review of smartphone-based passive sensing for health and wellbeing. Journal of biomedical informatics, 77, 120–132. https://doi. org/10.1016/j.jbi.2017.12.008
Danese, A., Moffitt, T. E., Harrington, H., Milne, B. J., Polanczyk, G., Pariante, C. M., Poulton, R., & Caspi, A. (2009). Adverse childhood experiences and adult risk factors for agerelated disease: depression, inflammation, and clustering of metabolic risk markers. Archives of pediatrics & adolescent medicine, 163(12), 1135–1143. https://doi.org/10.1001/ archpediatrics.2009.214
de Figueiredo, C. S., Sandre, P. C., Portugal, L., Mázala-de-Oliveira, T., da Silva Chagas, L., Raony, Í., Ferreira, E. S., Giestal-deAraujo, E., Dos Santos, A. A., & Bomfim, P. O. (2021). COVID-19 pandemic impact on children and adolescents' mental health: Biological, environmental, and social factors. Progress in neuro-psychopharmacology & biological psychiatry, 106, 110171. https://doi. org/10.1016/j.pnpbp.2020.110171
Dhingra, S. S., Kroenke, K., Zack, M. M., Strine, T. W., & Balluz, L. S. (2011). PHQ8 Days: a measurement option for DSM-5 Major Depressive Disorder (MDD) severity. Population health metrics, 9, 11. https://doi. org/10.1186/1478-7954-9-11
Huckins, J. F., daSilva, A. W., Wang, W., Hedlund, E., Rogers, C., Nepal, S. K., Wu, J., Obuchi, M., Murphy, E. I., Meyer, M. L., Wagner, D. D., Holtzheimer, P. E., & Campbell, A. T. (2020). Mental Health and Behavior of College Students During the Early Phases of the COVID-19 Pandemic: Longitudinal Smartphone and Ecological Momentary Assessment Study. Journal of medical Internet research, 22(6), e20185. https://doi.org/10.2196/20185
Jacobson, N. C., Lekkas, D., Huang, R., & Thomas, N. (2021). Deep learning paired with wearable passive sensing data predicts deterioration in anxiety disorder symptoms across 17-18 years. Journal of affective disorders, 282, 104–111. https://doi.org/10.1016/j. jad.2020.12.086
Martinez-Martin, N., Greely, H. T., & Cho, M. K. (2021). Ethical Development of Digital Phenotyping Tools for Mental Health Applications: Delphi Study. JMIR mHealth and uHealth, 9(7), e27343. https://doi. org/10.2196/27343
Norman Dalkey, Olaf Helmer, (1963) An Experimental Application of the DELPHI Method to the Use of Experts. Management Science 9(3):458-467. https://doi.org/10.1287/ mnsc.9.3.458 Torous J, Kiang MV, Lorme J, Onnela J New Tools for New Research in Psychiatry: A Scalable and Customizable Platform to Empower DataDriven Smartphone Research
JMIR Ment Health 2016;3(2):e16 doi: 10.2196/ mental.5165 PMID: 27150677 PMCID: 4873624
Rui Wang, Fanglin Chen, Zhenyu Chen, Tianxing Li, Gabriella Harari, Stefanie Tignor, Xia Zhou, Dror Ben-Zeev, and Andrew T. Campbell. 2014. StudentLife: assessing mental health, academic performance and behavioral trends of college students using smartphones. In Proceedings of the 2014 ACM International Joint Conference on Pervasive and Ubiquitous Computing (UbiComp '14). Association for Computing Machinery, New York, NY, USA, 3–14. https://doi.org/10.1145/2632048.2632054
Zhenyu Chen, Mu Lin, Fanglin Chen, Nicholas D. Lane, Giuseppe Cardone, Rui Wang, Tianxing Li, Yiqiang Chen, Tanzeem Choudhury, and Andrew T. Campbell. 2013. Unobtrusive sleep monitoring using smartphones. In Proceedings of the 7th International Conference on Pervasive Computing Technologies for Healthcare (PervasiveHealth' 13). ICST (Institute for Computer Sciences, Social-Informatics and Telecommunications Engineering), Brussels, BEL, 145–152. https://doi.org/10.4108/icst. pervasivehealth.2013.252148