How does an embryo develop?
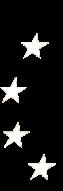
How does an embryo develop from a single cell into a highly complex structure?
How do cells self-organise to form and sustain this structure? The team behind the ERC-backed DeepEmbryo project are using innovative technologies to help build a deeper picture of embryogenesis, as Dr Hervé Turlier explains.
An intermingled sequence of biochemical and mechanical events help shape and control the development of an embryo. Mechanical events are necessary, as cells need to divide in the right manner and arrange themselves correctly, which involves a lot of movement, yet it is ultimately biochemistry that plays the central role. “There is some feedback between the two, but in the end it is biochemistry which controls the mechanics,” says Dr Hervé Turlier, leader of the Turlier lab at the Centre for Interdisciplinary Research in Biology, at the College de France in Paris. As Principal Investigator of the ERC-backed DeepEmbryo project, Dr Turlier is now working to essentially reverse-engineer the process of embryogenesis and develop a virtual embryo, building from data on cell mechanics. “I need data about the geometry of the cells, which is controlled by mechanics. We believe this is generic across different animal species,” he outlines. “From there, I can then start trying to include data about the biochemical regulation.”
Holoblastic divisions
This research is focused primarily on embryos with holoblastic or complete divisions, such as frog and mouse embryos. The idea was to start with relatively simple systems, many of which develop from a single cell, from which researchers can then look to build a deeper picture. “There are no gradients at early stages in these systems, cells communicate just through contacts,” explains Dr Turlier. As part of his work in the DeepEmbryo project, Dr Turlier is using new technological tools to investigate how a single cell is transformed into the highly complex structure of the early embryo. “Can I understand this using physical modelling? How can I extract as much biophysical information as possible from the available data?” he asks. “We use artificial neural networks, such as convolutional neural networks, which are a powerful architecture in deep learning used to analyse images. That’s a really data-driven approach. We are also exploring other types of networks, such as graph neural networks. Most of the data available in the real world comes in the form of graphs.”
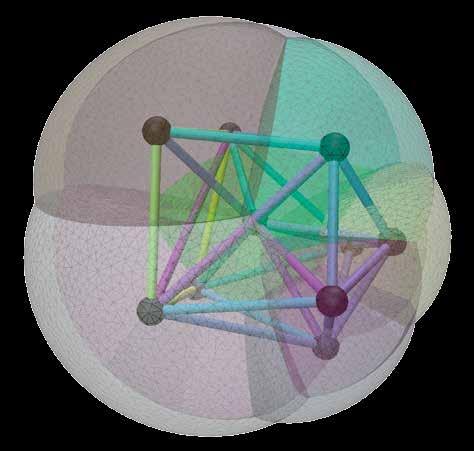
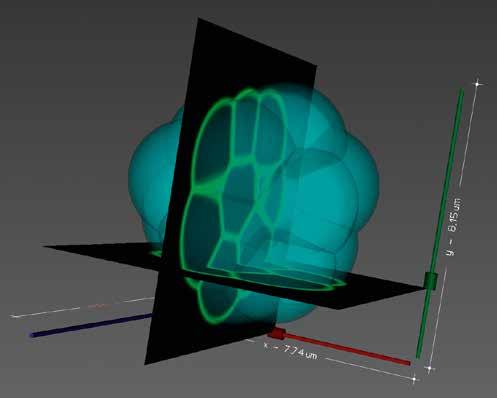
The idea here is to think of an embryo as a graph, essentially a network of entities or cells, which interact with each other mechanically and biochemically. In the early stages of the development of holoblastic embryos, cells interact largely through contacts with neighbouring cells. “You can draw each cell as a node of a graph, and each cell has a contact to its neighbour. One idea is to see these embryos and their development as a dynamic graph, where you can embed the mechanics, and also the biochemistry, as the biochemistry is also passed through the
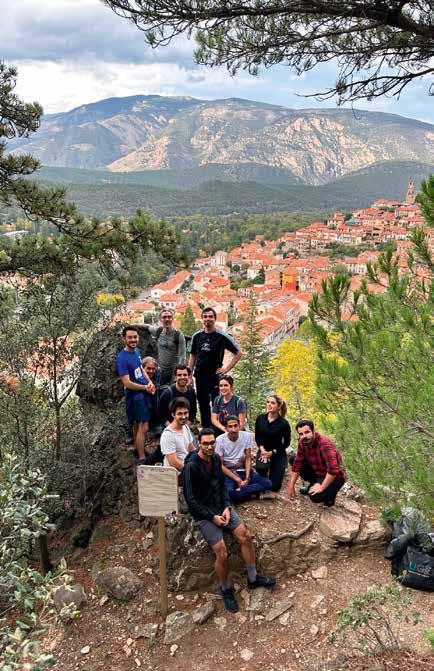
contacts,” outlines Dr Turlier. Artificial neural networks offer several advantages in terms of modelling cells, as Dr Turlier explains. “They emulate true simulations so as to scale and accelerate them. Secondly, you can combine data from different modalities very easily,” he says. “It doesn’t really matter if the data relates to protein concentrations or mechanical tension, or something else. They are represented by numbers, and they can be combined inside a neural network.”
An artificial neural network extracts the relevant biophysical information, with researchers in the project primarily focusing on the mechanical data. During his career Dr Turlier has worked extensively on models of the mechanics of embryonic cells, in which the main ingredient is surface tension. “This is an effective surface tension created by what we call the actomyosin cortex, which is a contractile layer. By continuously contracting and renewing itself, it creates an effective tension,” he explains. Cells are fairly big in the early stages of embryogenesis, with comparatively small nuclei. “We assume that the nuclei don’t play an important role in mechanics at these stages. We take the position that all forces are near the surface, and they are all in the form of surface tension, which can be described at varying levels of complexity,” says Dr Turlier. “We have shown that we can extract this information using neural networks. Now we can create maps of the mechanics of each cell, each interface in a full embryo, up to 800 cells.”
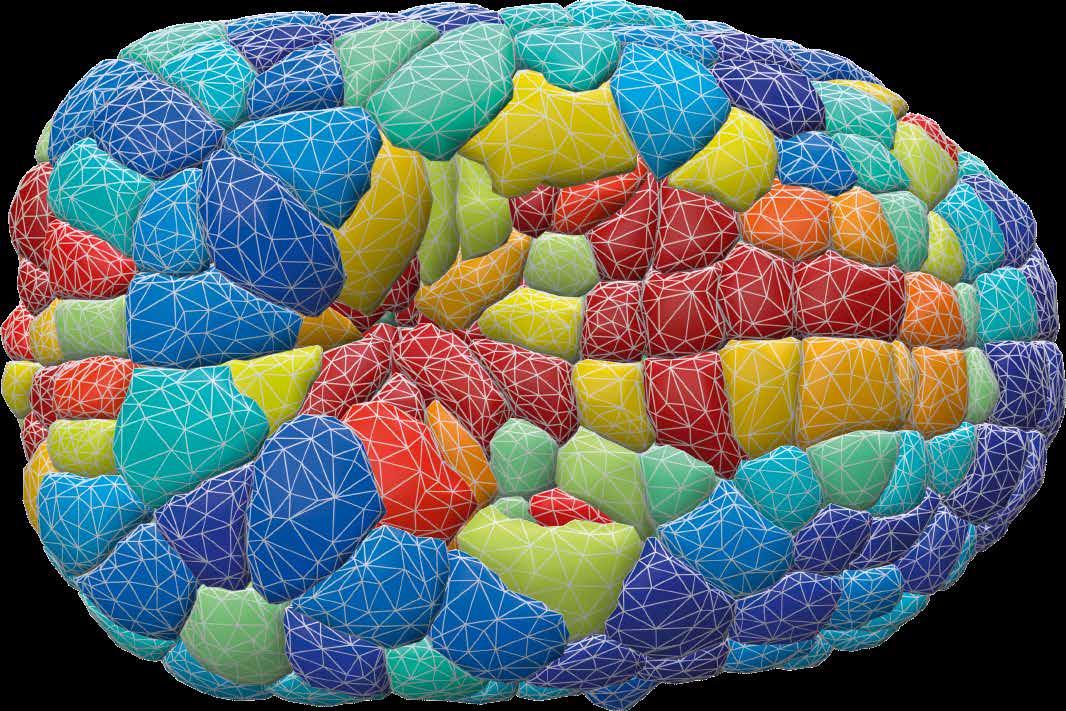
Researchers are also working to train the model itself using reinforcement learning, a technique which is mainly used currently to train robots. The idea is to learn by trial-and-error, to a point where the data and the simulations match. “A cell can be viewed as a sort of agent, like a robot is an agent. These cells are interacting agents on a graph,” says Dr Turlier. Cells are able to sense their surrounding environment, Dr Turlier and his team are looking to identify what exactly it is that they sense. “A cell can measure a concentration of ligands, like proteins which are secreted in the inter-cellular space. But do cells feel
of the project’s work. “We are reaching a point where we are able to emulate the mechanics of an embryo with a dynamic graph that has been trained with a neural network. We can then start implementing this general idea of training a model to reach a certain target, like a certain tissue model,” says Dr Turlier. This research could also hold wider relevance to the field of tissue engineering, a topic Dr Turlier is keen to explore further in future. “Could we design tissue with certain characteristics on computers? In tissue engineering, protocols are often developed by hand, and there is a lack of reliable computer models,
“We are reaching a point where we are able to emulate the mechanics of an embryo with a dynamic graph that has been trained with a neural network . We can then start implementing this general idea of training a model to reach a certain target, like a certain tissue model.”
geometry in some way?” he continues. “This may then influence a cell’s behaviour – for example whether it will divide, if it will secrete another ligand, if it will move away from being a pluripotent cell to being more specialised. A cell starts to differentiate based on its memory of the stimuli that it has undergone - it starts to take a certain task, and to specify its fate.”
Multi-agent system
The wider goal in the project is to model an embryo as a multi-agent system, on a graph, and to train it from data using reinforcement learning. A lot of energy has been devoted to improving the models, while significant progress has also been made on the graph neural network aspect
which can cost time and money,” he explains. “One idea is to use the same type of tools to better understand the tissue system. Then we can add data on the gene regulatory pathways for example, as that may be something you want to modify.” This could allow researchers to tune the biochemistry or mechanics in a certain manner, so that they can achieve a certain target shape or function of their computerdesigned tissue. A key challenge here is developing models that accurately describe the structure of tissue. “Once the models have been improved, can we then use them to design tissues? Researchers today can design molecules to perform specific functions. Can we do something similar for tissue?” continues Dr Turlier.
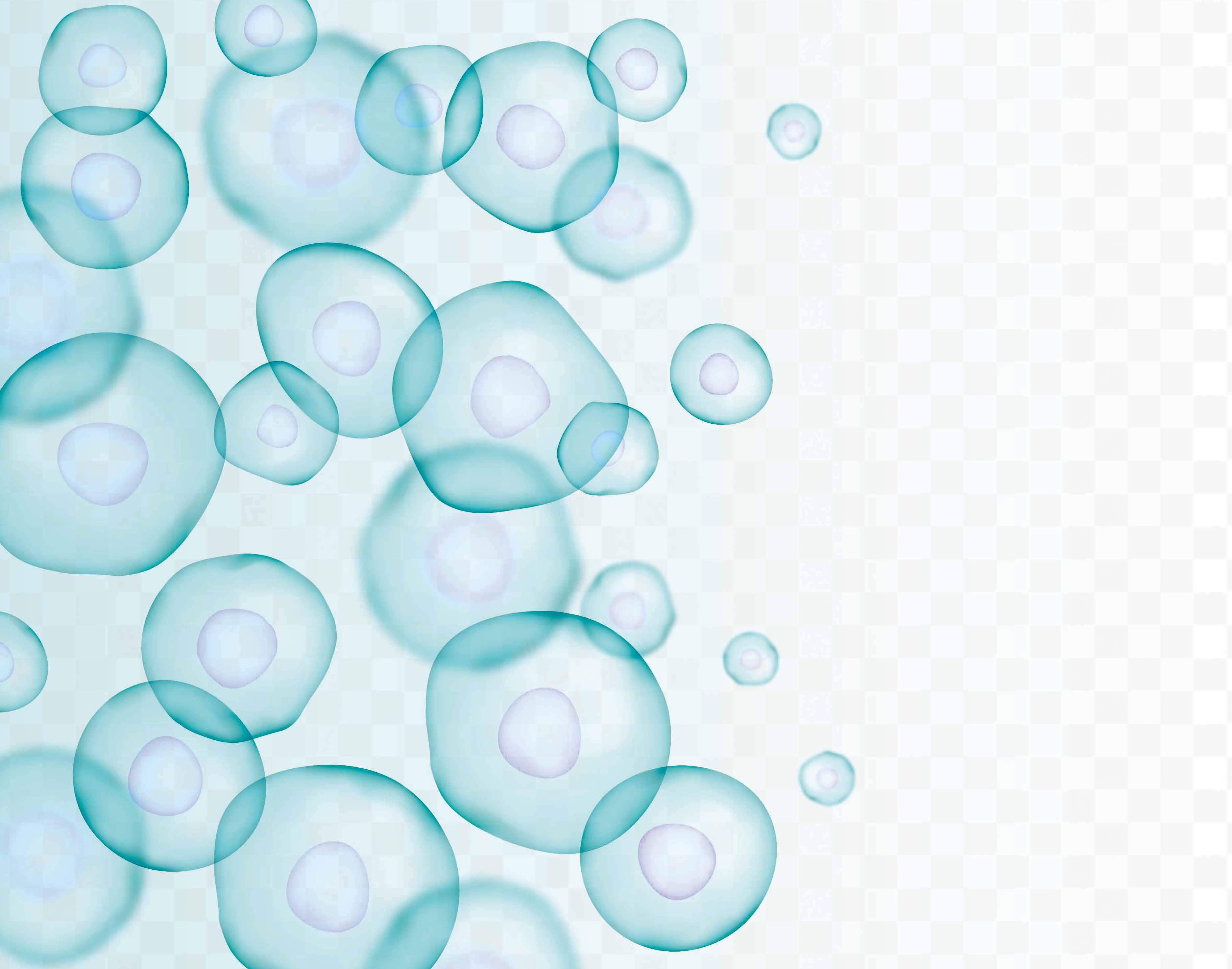
DeepEmbryo
Reverse-engineering the development of embryos with physics-informed machine learning receives
Project Objectives
Multicellular self-organisation is central to developmental biology and tissue engineering, yet extracting biophysical insights from complex imaging data remains challenging. DeepEmbryo leverages deep learning to infer cell behavior from microscopy, creating a computational platform to uncover morphogenetic mechanisms, enabling iterative refinement through virtual predictions and experimental validation of early embryo self-organisation.
Project Funding
This project has received funding from the European Union’s Horizon 2020 research and innovation programme under grant agreement No 949267.

Contact Details
Project Coordinator, Dr Hervé Turlier, PhD
Team Multiscale Physics of Morphogenesis, Center for Interdisciplinary Research in Biology Collège de France / CNRS UMR7241 / INSERM U1050
11, place Marcelin Berthelot 75231 Paris Cedex 05, FRANCE
T: +33 (0)144271410
E: herve.turlier@college-de-france.fr : @virtual_embryo
W: www.turlierlab.com
W: https://www.nature.com/articles/ s41592-023-02084-7
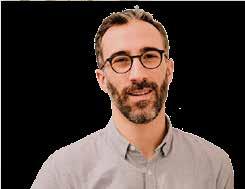
Dr Hervé Turlier after a PhD at the Institut Curie (2013) and a postdoc at EMBL on mouse embryo morphogenesis, founded the “Multiscale Physics of Morphogenesis” team at the Collège de France in 2017. Supported by an ERC Starting Grant (2020), his team combines physical modeling and AI to reverse-engineer a virtual embryo.
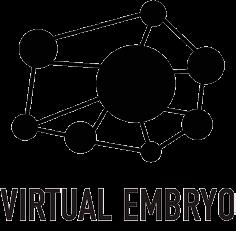
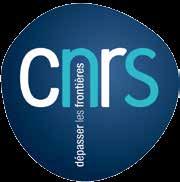