Vol. 11, No. 2, PP. 62-75, 2020
An Analysis on Novel Corona Virus by a Plithogenic Approach to Fuzzy Cognitive Map
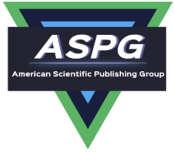
R.Sujatha1,*, S.Poomagal2, G.Kuppuswami3 and Said Broumi 4
1Department of Mathematics, Sri Sivasubramaniya Nadar College of Engineering, Chennai, INDIA ; sujathar@ssn.edu.in
2Department of Mathematics, University College of Engineering – Kancheepuram, Kancheepuram,INDIA; poomagal777@gmail.com
3 Department of Mathematics, Sri Venkateswaraa College of Technology, Sriperumbudur, INDIA. gkuppuswamiji@gmail.com
4Laboratory of Information Processing, Faculty of Science Ben MSik, University Hassan II, Casablanca, Morocco. e-mail:broumisaid78@gmail.com
* Correspondence: sujathar@ssn.edu.in
Abstract
In this paper a Plithogenic approach to Fuzzy cognitive map has been proposed to analyse the impact of novel corona virus.Contradiction degree is an advantageous feature of Plithogenic sets which highly deals with the uncertainty and it substantially increases the accuracy of results. In this research, a new approach is proposed to accumulate the opinions of experts.Using contradiction degree between opinions of experts and plithogenicoperator,the connection matrices obtained from distinct experts are aggregated to improvise a degree of uncertainty. An Analysis on covid-19 (causes, spread and precaution) is done based on the proposed methodology.
Keywords: Fuzzy cognitive maps,Plithogenic sets, Covid-19, Plithogenic operators
1.Introduction
The outbreak of the 2019 coronavirus (COVID-19) infections has spread worldwide, causing fever, severe respiratory illness and pneumonia. The virus is related to severe acute respiratory syndrome coronavirus (SARSCoV) [27], as compared to MERS-CoV, and SARS-CoV, COVID-19 exhibits faster human transmission, leading to the declaration of a world-wide health emergency by world health organisation (WHO) [28]. No specific drugs or vaccines are available till date. The Clinical evidences of COVID-19 are characterized by cough, fever, bilateral infiltrates on chest imaging. After testing positive, majority of the affected individuals were experiencing moderate symptoms, whereas 20% of the affected people show severe respiratory failure and septic shock [29], gastrointestinal, lymphopenia, myalgias, and lung abnormalities [30]. The death causing ability of the virus is dependent on chronic disorders [29]. The infection has been reported to cause moralities in aged persons [32].
Axelrod [1] has initiated the concept of cognitive maps (CM), a directed graph which represents the causal relationship between the data in a specific field. In the cognitive maps the concepts and causal relationship are represented by the nodes and edges respectively. The edges have the weights representing the intensity of the causal relationship among the concepts. Regardless, CMs applicability is restrained as it has the limitation of disability to
International Journal of Neutrosophic Science (IJNS)
define the strength of interrelationship between the concepts/factors. To overcome the inadequacy, kosko[2] with the zadeh’s concept of fuzzy[3] introduced fuzzy cognitive maps, as an extension of conventional CMs by defining the strength by fuzzy numbers. To construct the FCM the experts opinions were obtained based their experience in their field and the strength of the causal relationship between the factors can be estimated [4]. Several approaches are available for the specification of weights in FCM, in order to overcome the difficulty of assigning a crisp real number to express their views with regard to the strength of relationships which are uncertain, linguistic variables were preferred [5]. Triangular Fuzzy Numbers (TFNs) were used to represent relationships between the concepts instead of fuzzy singletons to get by efficiently with uncertainty and practice of fuzzy numbers will lead to more precise and information wealthy FCMs than the conventional FCMs[6] [7][8].FCM modelling has a wide range of application in the field of medicine and extensively reviewed in [20]. FCM was developed for medical decision support systems[21][22], prediction of probability occurance of stroke[23],for risk management in breast cancer[24], to define challenges and articulating solutions in nursing discipline[25] and to study the symptoms of migraine[26].
Fuzzy cognitive maps is extended to plithogenic fuzzy cognitive map (PFCM) which integrates the contradiction degree to the concepts and the methodology of FCM[9]. Smarandache [10] introduces the concept of Plithogenic sets, a generalization of crisp, fuzzy, intuitionistic fuzzy and neutrosophic sets. Plithogenic sets rely on the principle of quantification of the qualitative aspects and degree of contradiction is also the distinguishing feature of Plithogenic sets.Abdel-Basset integrates the concept of Plithogenic sets in decision making for selecting supply chain sustainability metrics[11],evaluation of hospital medical care systems[12] and for evaluating the performance of IoT based supply chain[13], Plithogenic MCDM based onTOPSIS –CRITIC model for sustainable supply chain risk management[14].In another study, Plithogenic sets used for multi variable data analysis in [32-35].FCM depends totally on the expert’s opinion, itlapse due to its uncertainty associate with the responses of each experts and contradiction between them.
This paper proposes a Plithogenic approach to fuzzy cognitive map to reform the drawback. The proposed model follows the methodology of FCM, in addition it merges the experts’ opinionby using the Plithogenic operators, based on the contradiction degree between them
2. Materials and methods
2.1 Fuzzy cognitive maps
Fuzzy cognitive maps(FCMS) are well developed computational method which combines the neural networks and fuzzy logic. FCMs are the fuzzy directed graphs with nodes representing the factors of the system considered and edges represents the causal relationship between the concepts/factors. The edges are characterised by the weights , which represents the strength of the causality between the concepts.The values of FCM are fuzzy, so the weights are represented in terms of linguistic variables [15]. Fuzzy linguistic scales are then interpreted to the fuzzy numbers through the information provided by the experts. A simple example of FCM is depicted in Figure-1.
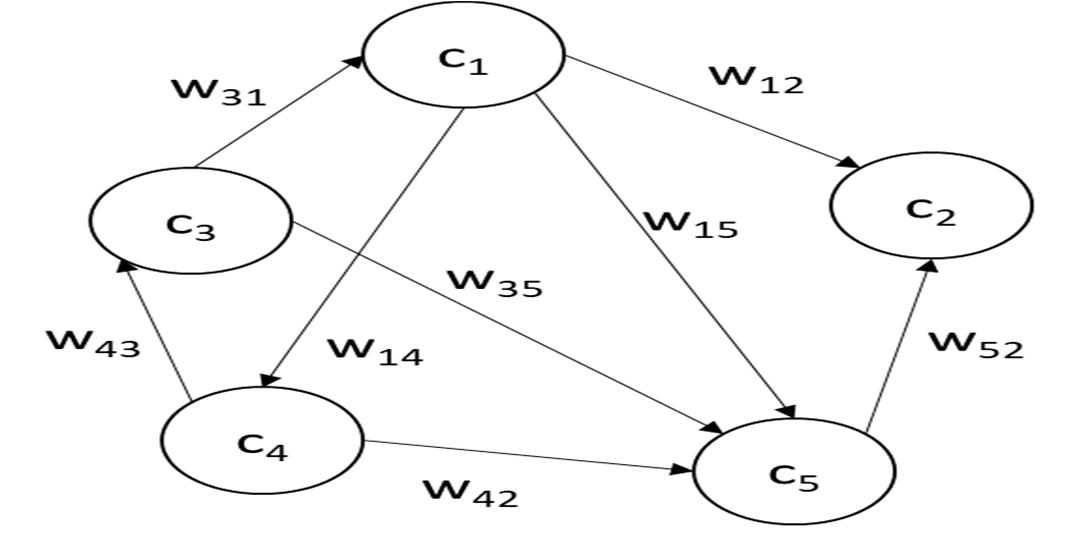
Figure 1. Fuzzy Cognitive Map
Vol. 11, No. 2, PP. 62-75, 2020 DOI:
International Journal of Neutrosophic Science (IJNS)
The influence of an individual concept on other concepts of the system is calculated by using the equation (1), the inference of FCM.