ANewTypeofNeutrosophicSetinPythagoreanFuzzyEnvironment andApplicationstoMulti-criteriaDecisionMaking
MahmutCanBozy˙ ı ˘ g ˙ ıt 1,MuratOlgun2,FlorentinSmarandache3 ,Mehmet ¨ Unver2
1AnkaraYıldırımBeyazıtUniversity,FacultyofEngineeringandNaturalSciences,Departmentof Mathematics,06010AnkaraTurkey
2AnkaraUniversity,FacultyofScience,DepartmentofMathematics,06100AnkaraTurkey
3 MathematicsDepartment,UniversityofNewMexico,705GurleyAve.,Gallup,NM87301,USA
Emails:mcbozyigit@aybu.edu.tr,olgun@ankara.edu.tr,smarand@unm.edu,munver@ankara.edu.tr
Abstract
Inthispaper,weintroducetheconceptsofPythagoreanfuzzyvaluedneutrosophicset(PFVNS)andPythagorean fuzzyvaluedneutrosophic(PFVNV)constructedbyconsideringPythagoreanfuzzyvalues(PFVs)insteadof numbersforthedegreesofthetruth,theindeterminacyandthefalsity,whichisanewextensionofintuitionisticfuzzyvaluedneutrosophicset(IFVNS).BymeansofPFVNSs,thedegreesofthetruth,theindeterminacy andthefalsitycanbegiveninPythagoreanfuzzyenvironmentandmoresensitiveevaluationsaremadebya decisionmakerindecisionmakingproblemscomparedtoIFVNSs.Inotherwords,suchsetsenableadecision makertoevaluatethedegreesofthetruth,theindeterminacyandthefalsityasPFVstomodeltheuncertainty intheevaluations.Firstofall,weproposetheconceptsofPythagoreanfuzzy t-normand t-conormandshow thatsomePythagoreanfuzzy t-normsand t-conormsareexpressedviaordinarycontinuousArchimedean tnormsand t-conorms.ThenwedefinetheconceptsofPFVNSandPFVNVandprovideatooltoconstruct aPFVNVfromanordinaryneutrosophicfuzzyvalue.Wealsodefinesomesettheoreticoperationsbetween PFVNSsandsomealgebraicoperationsbetweenPFVNVsvia t-normsand t-conorms.Withthehelpofthese algebraicoperationsweproposesomeweightedaggregationoperators.TomeasurediscriminationinformationofPFVNVs,wedefineasimplifiedneutrosophicvaluedmodifiedfuzzycross-entropymeasure.Moreover, weintroduceamulti-criteriadecisionmakingmethodinPythagoreanfuzzyvaluedneutrosophicenvironment andpracticetheproposedtheorytoareallifemulti-criteriadecisionmakingproblem.Finally,westudythe comparisonanalysisandthetimecomplexityoftheproposedmethod.
Keywords: Pythagoreanfuzzyvaluedneutrosophicset;aggregationoperators;multi-criteriadecisionmaking
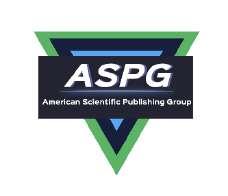
1Introduction
Zadeh42 introducedtheconceptoffuzzyset(FS)definedbyamembershipfunction µA fromauniversal set X toclosedunitinterval [0, 1] inordertohandletheuncertaintyinvariousreal-worldproblems.Then usingamembershipfunction µA : X → [0, 1] andanon-membershipfunction νA : X → [0, 1] under thecondition µA(x)+ νA(x) ≤ 1 for x ∈ X,Atanassov2 proposedtheconceptofintuitionisticfuzzyset (IFS)whichisanextensionoftheconceptofFS.Thepair ⟨µA(x),νA(x)⟩ iscalledanintuitionisticfuzzy value(IFV)forafixed x ∈ X.ThetheoryofIFShasbeenusedtosolveproblemsinmanyapplications asmulti-criteriadecisionmaking(MCDM),classification,patternrecognitionandclusteringandithasbeen studiedinmanyareasbyresearchers.Theconceptsofentropyandcross-entropyareimportantmeasurement methodsusedintheinformationtheoryandtheseconceptswereproposedbyShannon.23,24 Thentheconcept ofcross-entropywasimprovedbyKullbackandLeibler13,14 anditwasmodifiedbyLin.15 Inordertomeasure https://doi.org/10.54216/IJNS.200208 Received:July08,2022Accepted:January11,2023
Figure1:Thedevelopmentoftheoryofneutrosophicset discriminationinformationbetweenIFSs,WeiandYe31 introducedanimprovedintuitionisticfuzzycrossentropytoovercomethedrawbackofintuitionisticfuzzycross-entropygivenbyVlachosandSergiadis.30 The conceptofaggregationoperatorthattransformsseveralinputvaluesintoasingleoutputvalueisanimportant toolinthedecisionmakingtheory.Aseriesofaggregationoperatorshavebeenproposedbymanyresearchers. Particularly,variousgeneralizationsofaggregationoperatorsforIFSs(seee.g.3,7)weredefinedviaseveral typesof t-normsand t-conormson [0, 1].FurtherstudiesonMCDMwithFSsandaggregationoperatorscan befoundin.1,9,17,27,29,39–41
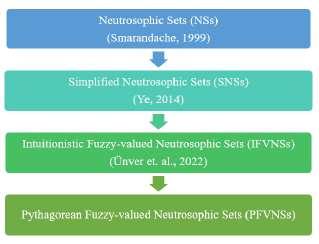
AsageneralizationofIFS,theconceptofPythagoreanfuzzyset(PFS)wasdevelopedbyYager,33,34 whichis definedbyamembershipfunction µA : X → [0, 1] andanon-membershipfunction νA : X → [0, 1] underthe condition µ2 A(x)+ ν2 A(x) ≤ 1 for x ∈ X.APFSismorecapablethananIFStoexpressuncertainty.Inother words,PFSsenhanceflexibilityandpracticabilityofIFSsandhavewiderareathanIFSswhiledescribing theuncertainty.ThereforetheoryofPFShasbeenstudiedbymanyresearcherstomodeltheuncertainty.
LaterYager34,35 proposedarangeofaggregationoperatorsforPFSs.Afterthat,Penget.al.20 presentedthe axiomaticdefinitionsofdistancemeasure,similaritymeasure,entropyandinclusionmeasureforPFSs.
Smarandache26 introducedtheconceptofneutrosophicset(NS)whereeachelementhasthedegreesoftruth, indeterminacyandfalsifyinthenon-standardunitinterval.Thereisnorestrictiononthemembershipfunctions forNSs.Duetothedifficultyofapplyingneutrosophicsetstopracticalproblems,theconceptofsimplified neutrosophicsets(SNSs)wasproposedbyYe.37 ASNSisconstructedbyatruth,anindeterminacyanda falsifymembershipfunctiondefinedfrom X to [0, 1].Ithasbeenusedinvariousnumericalapplications. Moreover,theconceptofsinglevaluedneutrosophicmulti-set(SVNMS)thatischaracterizedbysequencesof truth,indeterminacyandfalsifymembershipfunctionshasbeenproposedbyYeandYe.36
SchweizerandSklar21 introducedtheconceptoftriangularnorm(t-norm)andtriangularconorm(t-conorm), followingtheconceptofprobabilisticmetricspacesproposedbyMenger,16 whichisageneralizationofmetricspaces.ThesenotionsareusefultoolstodefinealgebraicoperationsandaggregationoperatorsforFSs. Deschrijveret.al.6 proposedtheconceptsofintuitionisticfuzzy t-normandintuitionisticfuzzy t-conorm, whichareextensionsoftheconceptsof t-normand t-conorm,respectively,byturningintointerval [0, 1] to {(x1,x2): x1,x2 ∈ [0, 1] and x1 + x2 ≤ 1}.Inthispaper,weintroducetheconceptsofPythagoreanfuzzy t-normandPythagoreanfuzzy t-conormwhichareextensionsofnotionsofbothordinaryandintuitionistic fuzzy t-normand t-conorm,respectively. Unveret.al.28 proposedtheconceptofintuitionisticfuzzyvaluedneutrosophicmulti-set(IFVNMS)bycombiningNStheoryandIFStheorywiththehelpofintuitionistic fuzzyvaluesinsteadofnumbersinmembershipsequences.Motivatingfromthisidea,weproposetheconceptsofPythagoreanfuzzyvaluedneutrosophicset(PFVNS)andPythagoreanfuzzyvaluedneutrosophic value(PFVNV)bycombiningNStheoryandPFStheory.APFVNVconsistsofatripleofPythagoreanfuzzy values(PFVs)andaPFVNSconsistsofPFVNVs.Thenwedevelopamethodtotransformfuzzyvaluesand givesomesettheoreticandalgebraicoperationswiththehelpofPythagoreanfuzzy t-normsand t-conorms. Byusingthesealgebraicoperationswedefinesomeweightedarithmeticandgeometricaggregationoperators. Entropyisausefultoolformeasuringuncertaininformation.Theconceptoffuzzyentropywasintroducedby Zadeh.43 Later,afuzzycross-entropymeasureandasymmetricdiscriminationinformationmeasurebetween FSswereproposedbyShangandJiang.22 WeiandYe31 definedamodifiedintuitionisticfuzzycross-entropy measurebetweenIFSs.Withsimilarmotivation,weintroduceasimplifiedneutrosophicvaluedmodifiedfuzzy
https://doi.org/10.54216/IJNS.200208
Received:July08,2022Accepted:January11,2023
cross-entropyforPFVNVs.Finally,usingtheseconceptsweprovideaMCDMmethodinthePythagorean fuzzyvaluedneutrosophicenvironment.
Somemaincontributionsofthepresentstudycanbelistedasfollows:
• WiththehelpofPythagoreanfuzzy t-normsand t-conormswestudyinthefuzzyenvironmentwhile conductingtheaggregationprocessindecisionmakingproblemsratherthandefuzzifiedenvironment thatprovidesuswithmoresensitivesolutions.
• BymeansofPFVNSs,thedegreesofthetruth,theindeterminacyandthefalsitycanbegiveninthe Pythagoreanfuzzyenvironmentandmoresensitiveevaluationsaremadebydecisionmakersinreal lifeproblems.Inotherwords,suchsetsenableadecisionmakerevaluatethedegreesofthetruth,the indeterminacyandthefalsityasPFVsindecisionmakingprocess.Thus,theuncertaintyinthedecision maker’sevaluationsisrepresentedwiththehelpofmorecapableFSnotion.
• Theproposedcross-entropymeasuremeasuresthediscriminationoftheinformationbetweenPFVNVs inafuzzyenvironment.Thusmoresensitiveevaluationscanbemadeindecisionmakingproblems beforedefuzzifyingtheenvironment.
Therestofthepaperisorganizedasfollows.InSection2,weintroducetheconceptsofPythagoreanfuzzy tnormand t-conormandshowthatsomePythagoreanfuzzy t-normsand t-conormsareexpressedviaordinary continuousArchimedean t-normsand t-conorms.InSection3,weintroducetheconceptofPFVNS,which isanextensionofthenotionofintuitionisticfuzzyvaluedneutrosophicset(IFVNS)whereanIFVNSis anIFVNMSwithsequencelength 1.Thenwedevelopamethodtotransformsimplifiedneutrosophicvalues (SNVs)toPFVNVs.WealsogivesomesettheoreticalandalgebraicoperationsviaPythagoreanfuzzy t-norms and t-conormsforPFVNVs.InSection4wedefinesomeweightedaggregationoperatorsusingthesealgebraic operations.InSection5,motivatingbythemodifiedintuitionisticfuzzycross-entropymeasuredefinedbyWei andYe,31 wegiveasimplifiedneutrosophicvaluedmodifiedfuzzycross-entropy.InSection6,wepropose aMCDMmethodandapplytheproposedtheorytoaMCDMproblemfromtheliterature.Wealsocompare theresultsoftheproposedmethodwiththeexistingresults.Finally,wecalculatethetimecomplexityofthe MCDMmethod.InSection7,weconcludethepaper.
2PythagoreanFuzzy t-normsand t-conorms
Inthissection,werecallsomefundamentaldefinitionsabout t-normsand t-conormsanddefinetheconcepts ofPythagoreanfuzzy t-normand t-conorm.
Definition2.1. 10,21 A t-normisafunction T :[0, 1] × [0, 1] → [0, 1] thatsatisfiesthefollowingconditions:
(T1) T (x, 1)= x forall x ∈ [0, 1],
(T2) T (x,y)= T (y,x) forall x,y ∈ [0, 1],
(T3) T (x,T (y,z))= T (T (x,y),z) forall x,y,z ∈ [0, 1],
(T4) T (x,y) ≤ T (x′,y′) whenever x ≤ x′ and y ≤ y′ .
Definition2.2. 10,21 A t-conormisafunction S :[0, 1] × [0, 1] → [0, 1] thatsatisfiesthefollowingconditions:
(S1) S(x, 0)= x forall x ∈ [0, 1],
(S2) S(x,y)= S(y,x) forall x,y ∈ [0, 1],
(S3) S(x,S(y,z))= S(S(x,y),z) forall x,y,z ∈ [0, 1],
(S4) S(x,y) ≤ S(x′,y′) whenever x ≤ x′ and y ≤ y′
https://doi.org/10.54216/IJNS.200208
Received:July08,2022Accepted:January11,2023
Definition2.3. 33,34 Afuzzycomplementisafunction N :[0, 1] → [0, 1] satisfyingthefollowingconditions:
(N1) N (0)=1 and N (1)=0,
(N2) N (a) ≥ N (b) whenever a ≤ b forall a,b ∈ [0, 1],
(N3)Continuity,
(N4) N (N (a))= a forall a ∈ [0, 1].
Thefunction N :[0, 1] → [0, 1] definedby N (a)=(1 ap)1/p where p ∈ (0, ∞) isafuzzycomplement introducedbyYager.33,34 When p =2, N (a)= √1 a2 iscalledthePythagoreanfuzzycomplement.
Definition2.4. 12 Let T bea t-normandlet S bea t-conormon [0, 1].If T (x,y)= N (S(N (x),N (y))) and S(x,y)= N (T (N (x),N (y))) forafuzzynegator N ,then T and S aresaidtobedualwithrespectto N
Remark2.5. Let T bea t-normon [0, 1] andlet N (a)= √1 a2.Thenthedual t-conormSwithrespectto N is S(x,y)= 1 T 2 1 x2 , 1 y2
Astrictlydecreasingfunction g :[0, 1] → [0, ∞] with g(1)=0 iscalledtheadditivegeneratorofa t-norm T ifwehave T (x,y)= g 1(g(x)+ g(y)) forall (x,y) ∈ [0, 1] × [0, 1]
Proposition2.6. 12 Let g :[0, 1] → [0, ∞] betheadditivegeneratorofa t-norm T ,let S bethedual tconormof T andlet N beafuzzycomplement.Thestrictlyincreasingfunction h :[0, 1] → [0, ∞] definedby h(t)= g(N (t)) istheadditivegeneratorof S andso S (x,y)= h 1 (h (x)+ h (y))
Notethat T isanArchimedean t-normifandonlyif T (x,x) <x forall x ∈ (0, 1) and S isanArchimedean t-conormifandonlyif S(x,x) >x forall x ∈ (0, 1) 10 KlementandMesiar11 provedthatcontinuous Archimedean t-normshaveusefulrepresentationsviatheiradditivegeneratorsasfollows.
Theorem2.7. 11 Let T bea t-normon [0, 1].Thefollowingareequivalent:
(i) T isacontinuousArchimedean t-norm.
(ii) T hasacontinuousadditivegenerator.
BeforeintroducingtheconceptsofPythagoreanfuzzy t-normand t-conormwerecalltheconceptofPFS. Throughoutthispaperweassume X = {x1,...,xn} isafiniteset.
Definition2.8. 33,34 APFS A on X isdefinedby
A = {⟨xj ,µA(xj ),νA(xj )⟩ : j =1,...,n} where
: X → [0, 1] arethemembershipandnon-membershipfunctionsrespectivelywithcondition that
Motivatingby6 wenowintroducethenotionsofPythagoreanfuzzy t-normandPythagoreanfuzzy t-conorm. Considertheset P ∗ definedby
Definition2.9. APythagoreanfuzzy t-normisafunction T : P ∗ × P ∗ → P ∗ thatsatisfiesthefollowing conditionsforany x =(x1,x2),y =(y1,y2),z =(z1,z2) ∈ P ∗
(PT1) T (x, (1, 0))= x forall x ∈ P ∗ ,
(PT2) T (x,y)= T (y,x) forall x,y ∈ P ∗ ,
(PT3) T (x, T (y,z))= T (y, T (x,z)) forall x,y,z ∈ P ∗ ,
(PT4) T (x,y) ⪯T (x′,y′) whenever x ⪯ x′ and y ⪯ y′ .
Definition2.10. APythagoreanfuzzy t-conormisafunction S : P ∗ × P ∗ → P ∗ thatsatisfiesthefollowing conditionsforany x =(x1,x2),y =(y1,y2),z =(z1,z2) ∈ P ∗ .
(PS1) S(x, (0, 1))= x forall x ∈ P ∗ ,
(PS2) S(x,y)= S(y,x) forall x,y ∈ P ∗ ,
(PS3) S(x, S(y,z))= S(y, S(x,z)) forall x,y,z ∈ P ∗ ,
(PS4) S(x,y) ⪯S(x′,y′) whenever x ⪯ x′ and y ⪯ y′ WedefinetheconceptofPythagoreanfuzzynegatorasanextensionofthenotionoffuzzynegator.
Definition2.11. APythagoreanfuzzynegatorisafunction N : P ∗ → P ∗ satisfyingthefollowingconditions:
(PN1) N ((1, 0))=(0, 1) and N ((0, 1))=(1, 0),
(PN2) N (x) ⪰N (y) whenever x ⪯ y.
If N (N (x))= x forall x ∈ P ∗,thenwecall N involutive.Themapping Ns : P ∗ → P ∗ givenby Ns((x1,x2))=(x2,x1) isaninvolutivePythagoreanfuzzynegatorwhichiscalledthestandardnegator.
FollowingtheoremshowsthataPythagoreanfuzzy t-conormcanbeobtainedfromaPythagoreanfuzzy t-norm viaPythagoreanfuzzynegators.
Theorem2.12. Let T beaPythagoreanfuzzy t-normandlet N beaninvolutivePythagoreanfuzzynegator. Thefunction S definedby S(x,y)= N (T (N (x), N (y))) forany x,y ∈ P ∗ isa t-conorm.
Proof. (PS1)Forall x =(x1,x2) ∈ P ∗ weget
S x, (0, 1)
= N (T (N (x1,x2), N (0, 1)))
= N (T (N (x1,x2), (1, 0)))
= N (N (x1,x2))
= x.
(PS2)Forall x,y ∈ P ∗ weobtain
S(x,y)= N T (N (x), N (y))
= N T (N (y), N (x))
= S(y,x)
https://doi.org/10.54216/IJNS.200208
Received:July08,2022Accepted:January11,2023
InternationalJournalofNeutrosophicScience(IJNS)Vol.20,No.02,PP.107-134,2023
(PS3)Forall x,y,z ∈ P ∗ wehave S(x, S(y,z))= S x, N (T (N (y), N (z))
= N T (N (x), N N (T (N (y), N (z)
= N T (N (x), (T (N (y), N (z))
= N T (N (y), (T (N (x), N (z))
= N T (N (y), N N (T (N (x), N (z)
= S y, N (T (N (x), N (z))
= S(y, S(x,z))
(PS4)Forall x,x′,y,y′ ∈ P ∗ wehave x ⪯ x ′ and y ⪯ y ′ ⇒N (x) ⪰N (x ′) and N (y) ⪰N (y ′)
⇒T N (x), N (y) ⪰T N (x ′), N (y ′)
⇒N T N (x), N (y) ⪯N T N (x ′), N (y ′)
⇒S(x,y) ⪯S(x ′ ,y ′).
Therefore, S isaPythagoreanfuzzy t-conorm.
The t-conorm S inTheorem2.12iscalledthedualPythagoreanfuzzy t-conormof T withrespecttonegator N .FollowingtheoremstatesthatPythagoreanfuzzy t-normsand t-conormscanbeproducedbyordinary t-normsand t-conorms.
Theorem2.13. Let T bea t-normandlet
Therefore T isaPythagoreanfuzzy t-norm.Similarly,itcanbeshownthat S isaPythagoreanfuzzy tconorm.
ContinuousArchimedean t-normsand t-conormson [0, 1] canbegeneratedbytheiradditivegenerators.Sowe canconstructaPythagoreanfuzzy t-normandaPythagoreanfuzzy t-conormusingtheseadditivegenerators. Notethatifa t-norm T anda t-conorm S aredualwithrespecttothePythagoreanfuzzycomplement,then(1) issatisfied.
Corollary2.14. Let g :[0, 1] → [0, ∞] betheadditivegeneratorofacontinuousArchimedean t-norm T andlet h :[0, 1] → [0, ∞] betheadditivegeneratorofthedualcontinuousArchimedean t-conorm S where h(t)= g(√1 t2).Thenthefunction
isthedualPythagoreanfuzzy t-conormof S withrespectto NS .Inthiscase T and S arecalledPythagorean fuzzy t-normandPythagoreanfuzzy t-conormgeneratedby g and h,respectively.
Proof. ItistrivialfromTheorem2.13andRemark2.5.
Example2.15. Considerthefunctions g :[0, 1] → [0, ∞] definedby g(t)= log t2 and h :[0, 1] → [0, ∞] definedby h(t)= log(1 t2).ThentheAlgebraicPythagoreanfuzzy t-normis T (x,y)= x1y
andtheAlgebraicdualPythagoreanfuzzy t-conormwithrespectto Ns is
3PythagoreanFuzzyValuedNeutrosophicSets
WestartthissectionrecallingtheconceptofIFVNSobtainedbytakingthesequencelengthequalto 1 in IFVNMSs.ThenwedefinetheconceptsofPFVNSandPFVNV.
https://doi.org/10.54216/IJNS.200208 Received:July08,2022Accepted:January11,2023
Definition3.1. 28 AnIFVNS A on X isdefinedby
However,anIFVNScannotberepresentedasastandardSNS(see,e.g.,Example3.2).
Insomedecisionmakingproblems,thesumofthemembershipandnon-membershipdegreesthataredeterminedbythedecisionmakerscanbelargerthan1.Therefore,PFVsaremorecapablethanIFVsformodelling vaguenessinthedegreesofthetruth,theindeterminacyandthefalsityinthepracticalproblemsasshownin Figure2.UsingPFVsforthedegreeofthetruth,theindeterminacyandthefalsity,wedefinetheconceptof PFVNSandproposesomesettheoreticalandalgebraicoperationsbetweenPFVNSs.ByutilizingPFVNSs, theuncertaintyinthedecisionmaker’sevaluationscanbemodeledinamorecapableenvironment.
Definition3.4. APFVNS A on X isdefinedby
arethetruth,theindeterminacyandthefalsitymembershippairsofPFVs,respectively,
Figure2:ComparisonofconceptsofPFVandIFVforthetruth,theindeterminacyandthefalsitymembership degrees
Remark3.6. EachIFVNSisaPFVNSbuttheconverseofthisstatementisnottrueingeneral.Forexample, A isaPFVNS,but A isnota IFVNS inExample3.5since 0 7+0 6=1 3 > 1 for I2 A =(0 7, 0 6)
SNVscanbeconvertedtoPFVNVswiththefollowingmethod.AsimilarmethodwasproposedforIFSsin.25 Proposition3.7. Let ρ :[0, 1] → [0, 1] beafunctionsuchthat ρ(t) ≤ √t forany t ∈ [0, 1].Considera number µα ∈ [0, 1].Then
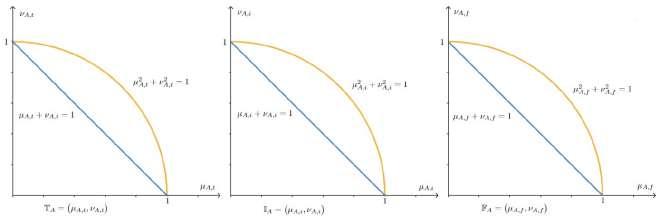
)⟩ isaPFV.
Proof. Let µα ∈ [0, 1]
So α isa PFV
Wecantransforma SNV intoa PFVNV byusingProposition3.7asinthefollowingexample.
Example3.8. ConsidertheSNV A = ⟨0 5, 0 3, 0 6⟩ andthefunction ρ :[0, 1] → [0, 1] definedby ρ(t)= sint.FromProposition3.7,weobtainaPFVNVfrom A asfollows:
WevisualizethistransformationinFigure3.
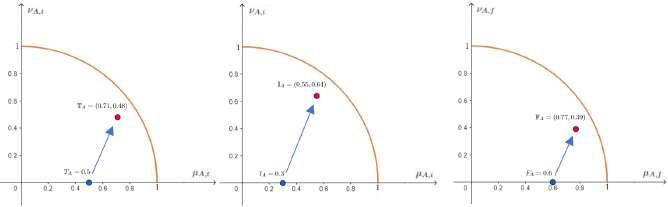
NowwedefinethesetoperationsbetweenPFVNSs.ThroughoutthispaperthesetoperationsforPFSsare denotedby ⊂(pyt), ∪(pyt), ∩(pyt), ( )c(pyt) ”(see,34).
https://doi.org/10.54216/IJNS.200208
Received:July08,2022Accepted:January11,2023
Definition3.9. Let
a)
b)
c) Ac = xj , Fj A, Ij A c(pyt) , Tj A : j =1,...,n whereforall j =1,...,n
Ij A c(pyt) = νA,i(xj ),µA,i(xj )
d) A ∪ B = xj , Tj A ∪(pyt) Tj B , Ij A ∩(pyt) Ij B , Fj A ∩(pyt) Fj B : j =1,...,n where
Tj A ∪(pyt) Tj B = max µA,t(xj ),µB,t(xj ) , min νA,t(xj ),νB,t(xj )
Ij A ∩(pyt) Ij B = min µA,i(xj ),µB,i(xj ) , max νA,i(xj ),νB,i(xj )
Fj A ∩(pyt) Fj B = min µA,f (xj ),µB,f (xj ) , max νA,f (xj ),νB,f (xj ) .
e) A ∩ B = xj , Tj A ∩(pyt) Tj B , Ij A ∪(pyt) Ij B , Fj A ∪(pyt) Fj B : j =1,...,n where
Tj A ∩(pyt) Tj B = min µA,t(xj ),µB,t(xj ) , max νA,t(xj ),νB,t(xj )
Ij A ∪(pyt) Ij B = max µA,i(xj ),µB,i(xj ) , min νA,i(xj ),νB,i(xj )
Fj A ∪(pyt) Fj B = max µA,f (xj ),µB,f (xj ) , min νA,f (xj ),νB,f (xj )
Example3.10. Let X = {
NowweintroducesomealgebraicoperationsforPFVNVsviaPythagoreanfuzzy
α, Iβ , S Fα, Fβ
Remark3.13. If g istheadditivegeneratorofacontinuousArchimedean t-normand h(t)= g(√1 t2), thenfromCorollary2.14weget
α
FollowingpropositionisthevalidationofthatthesumandtheproductoftwoPFVNVsarealsoPFVNVs.
Proposition3.14. Let α and β betwoPFVNVs,let T beAPythagoreanfuzzy t-normandlet S bethedual Pythagoreanfuzzy t-conormwithrespecttoaPythagoreanfuzzynegator N .Then, α ⊕ β and α ⊗ β arealso PFVNVs.
Proof. Since T and S haverange P ∗ , α ⊕ β and α ⊗ β arePFVNVs.
Nextwedefinemultiplicationbynon-negativeconstantandnon-negativepowerofPFVNVsusingadditive generatorsofcontinuousArchimedean t-normand t-conormon [0, 1]
https://doi.org/10.54216/IJNS.200208 Received:July08,2022Accepted:January11,2023
Definition3.15. Let α = ⟨Tα, Iα, Fα⟩
ThefollowingpropositionverifiesthatmultiplicationbyconstantandpowerofPFVNVsarealsoPFVNVs.
whichyieldsthat Tλα isaPFV.Similarly,itcanbeeasilyshownthat
, Iαλ and Fαλ arePFVs. Therefore λα isaPFVNV.
Followingtheoremgivessomebasicpropertiesofalgebraicoperations.
Theorem3.17.
λ,γ ≥ 0,let g betheadditivegeneratorofacontinuousArchimedean t-normandlet h(t)= g(√1 t2).Then,
i) α ⊕ β = β ⊕ α
ii) α ⊗ β = β ⊗ α
iii) (α ⊕ β) ⊕ θ = α ⊕ (β ⊕ θ)
iv) (α ⊗ β) ⊗ θ = α ⊗ (β ⊗ θ)
v) λ(α ⊕ β)= λα ⊕ λβ
vi) (λ + γ)α = αλ ⊕ γα
vii) (α ⊗ β)λ = αλ ⊗ βλ
viii) αλ ⊗ αγ = αλ+γ
Proof. (i)and(ii)aretrivial.
iii) ItisclearfromDefinition3.12that
Tα⊕β = h 1 h(µα,t)+ h(µβ,t) ,g 1 g(να,t)+ g(νβ,t) . https://doi.org/10.54216/IJNS.200208 Received:July08,2022Accepted:January11,2023
InternationalJournalofNeutrosophicScience(IJNS)Vol.20,No.02,PP.107-134,2023
Therefore,weobtain
T(α⊕β)⊕θ = S(Tα⊕β , Tθ)
= h 1 h h 1 h(µα,t)+ h(µβ,t) + h µθ,t ,
g 1 g g 1 g(να,t)+ g(νβ,t) + g νθ,t
= h 1 h(µα,t)+ h(µβ,t)+ h(µθ,t) ,
g 1 g(να,t)+ g(νβ,t)+ g νθ,t
= h 1 h(µα,t)+ h h 1 h(µβ,t)+ h(µθ,t) ,
g 1 g(να,t)+ g g 1 g(νβ,t)+ g νθ,t
= S(Tα, Tβ⊕θ) = Tα⊕(β⊕θ)
Similarlyitcanbeeasilyobtainedthat I(α⊕β)⊕θ = Iα⊕(β⊕θ) and F(α⊕β)⊕θ = Fα⊕(β⊕θ) iv) ItisclearfromDefinition3.12that
Therefore,wehave
Tα⊗β = g 1 g(µα,t)+ g(µβ,t) ,h 1 h(να,t)+ h(νβ,t)
T(α⊗β)⊗θ = T (Tα⊗β , Tθ)
= g 1 g g 1 g(µα,t)+ g(µβ,t) + g µθ,t , h 1 h h 1 h(να,t)+ h(νβ,t) + h νθ,t
= g 1 g(µα,t)+ g(µβ,t)+ g(µθ,t) , h 1 h(να,t)+ h(νβ,t)+ h νθ,t
= g 1 g(µα,t)+ g g 1 g(µβ,t)+ g(µθ,t) , h 1 h(να,t)+ h h 1 h(νβ,t)+ h νθ,t = T (Tα, Tβ⊗θ)
= Tα⊗(β⊗θ)
Similarlyitcanbeseenthat I(α⊗β)⊗θ = Iα⊗(β⊗θ) and F(α⊗β)⊗θ = Fα⊗(β⊗θ)
v) ItisclearfromDefinition3.12andDefinition3.15that
Tα⊕β =(µα⊕β,t,να⊕β,t)= h 1 h(µα,t)+ h(µβ,t) ,g 1 g(να,t)+ g(νβ,t) and Tλα =(µλα,t,νλα,t)= h 1 λh µα,t ,g 1 λg να,t
Therefore,weget
Tλ(α⊕β) = h 1 λh(µ
Similarlyitcanbeshownthat
vi)
vii)
4WeightedAggregationOperatorsforPFVNVs
Aggregationoperatorshaveavitalrolewhiletransforminginputvaluesrepresentedbyfuzzyvaluestoa singleoutputvalue.Inthissection,weintroduceaweightedarithmeticaggregationoperatorandaweighted geometricaggregationoperatorforcollectionsofPFVNVsbyusingalgebraicoperationsgiveninSection3.
4.1AWeightedArithmeticAggregationOperator
Asseeninthenexttheorem,weightedarithmeticaggregationoperatorscanbeexpressedviaadditivegeneratorswhenever t-normand t-conormarecontinuousArchimedean.
Proof. ItisclearfromPropositions3.14and3.16that WA PFVVNV (
1,...,αn) isaPFVNV.Using mathematicalinductionweshowthatthesecondpartisvalid.If n =2,weobtain
https://doi.org/10.54216/IJNS.200208
Remark4.3. Usingparticularadditivegeneratorsweobtainsomeparticularcasesof WA PFVNV as follows:
a) Letgbetheadditivegeneratordefinedby g(t)= log t2.ThenwegetAlgebraicweightedarithmetic aggregationoperatorgivenby
b) Letgbetheadditivegeneratordefinedby g(t)=log( 2 t2 t2 ).ThenwegetEinsteinweightedarithmetic aggregationoperatorgivenby
where w =(w1,...,wn)T isaweightvectorsuchthat 0 ≤ wj ≤
Asseeninthenexttheorem,weightedgeometricaggregationoperatorscanbeexpressedviaadditivegeneratorswhenever t-normand t-conormarecontinuousArchimedean.
Theorem4.5.
Proof. ItcanbeprovedsimilartoTheorem4.2. Remark4.6. Byusingparticularadditivegeneratorswealsoobtainsomeparticularcasesof
5ASimplifiedNeutrosophicValuedModifiedFuzzyCross-EntropyMeasureforPFVNVs
ThenotionofSNSwasproposedbyYe37 torelievethedifficultyofapplyingNSstopracticalproblems.
InternationalJournalofNeutrosophicScience(IJNS)Vol.20,No.02,PP.107-134,2023
Definition5.1. 37 ASNS A on X isgivenby
A = {⟨xj ,TA(xj ),IA(xj ),FA(xj )⟩ : j =1,...,n}
where TA,IA,FA : X → [0, 1] arethetruth,indeterminacyandfalsifymembershipfunctions.Forconvenience,aSNVisdenotedby
α = ⟨Tα,Iα,Fα⟩ = ⟨TA(xj ),IA(xj ),FA(xj )⟩
forafixed xj ∈ X
SomealgebraicoperationsofSNVisrecalledinthenextdefinition.
Definition5.2. 18 Let A = ⟨TA,IA,FA⟩ and B = ⟨TB ,IB ,FB ⟩ beSNVs.Assumethat g :[0, 1] → [0, ∞] istheadditivegeneratorofacontinuousArchimedean t-normand h :[0, 1] → [0, ∞] istheadditivegenerator ofacontinuousArchimedean t-conorm.SomealgebraicoperationsbetweenSNVsaredefinedasfollow:
Thefollowingpropositionverifiesthatasimplifiedneutrosophicvaluedmodifiedfuzzycross-entropymeasure between α and β isaSNV.
Proposition5.4. E(α,β) isaSNV.
Proof. AccordingtoShannon’sinequality,15 weknowthateachcomponentof E(α,β) isequalorgreaterthan 0.Ontheotherhand,sincetherelation
istrue,eachcomponentof E(α,β) isequalorlessthan1.Therefore E(α,β) isaSNV.
Itisclearthat E(α,β) = E(β,α) ingeneral.Tomaketheconceptsymmetricwecandefinethefollowing.
Definition5.5. Let α and β betwoPFVNVs.Asimplifiedneutrosophicvaluedsymmetricdiscrimination informationmeasurebasedon E isgivenby
wheremultiplicationbyscalar 1 2 isinthesenseof(iii)ofDefinition5.2.
https://doi.org/10.54216/IJNS.200208
E∗ canbeexpressedviaadditivegeneratorsofcontinuousArchimedean t-normsand t-conorms.
Theorem5.6. Let α and β betwoPFVNVs,let g betheadditivegeneratorofacontinuousArchimedean t-normand h(t)= g(1 t).Then
Byusingparticularadditivegeneratorswecanobtainsomeparticularcasesof E∗ .
Remark5.7. Let g betheadditivegeneratordefinedby g(t)= log t.ThenwegetAlgebraicsimplified neutrosophicvaluedsymmetricdiscriminationinformationmeasurebasedon E givenby
Since E∗ isaSNV,ascorefunctionisneededtorankthevaluesof E∗.Inthispaper,weusethefollowing scorefunction.
Definition5.8. 32 Let A =(TA,IA,FA) beaSNV.Ascorefunctionisdefinedby
Theorem5.9. Let α and β betwoPFVNVs.Themodifiedcross-entropymeasure E∗ satisfiesthefollowing properties.
i) E∗(α,α)= ⟨0, 1, 1⟩,
ii) 0 ≤ N (E∗(α,β)) ≤ 1,
iii) E∗(α,β)= E∗(β,α),
iv) N (E∗(α,α))=0
Proof.
Weknowthat
6AnApplicationof PFVNVs ToAMCDMProblem
Inthissection,weprovideaMCDMmethodinPythagoreanfuzzyvaluedneutrosophicenvironment.Then wesolveaMCDMproblemfromtheliteraturebyusingtheproposedmethod.
https://doi.org/10.54216/IJNS.200208
Table1:SNVsofalternativesaccordingtocriteria
6.1AMCDMmethod
Inthissub-section,wegiveaMCDMmethodinthePythagoreanfuzzyvaluedneutrosophicenvironment.The proposedmethodisappliedtoaMCDMproblemadaptedfromtheliterature37,38 toseetheeffectivenessof theproposedmethod.Thestepscanbesummarizedasfollows.
Step1: FormaMCDMproblemwithalternatives
Step2: Theweightsofcriteriaaredetermined.
Step3: Theevaluationresultsofthealternativesareexpressedbytheexpertas SNVs foreachcriterion.
Step4: UsingProposition3.7,evaluationresultsofalternativesinStep3areconvertedfromSNVsto PFVNVs.
Step5: EvaluationvaluesexpressedasPFVNVsforeachalternativeaccordingtocriteriaaretransformedtoa valueexpressedasaPFVNVbyutilizingproposedweightedaggregationoperators.
Step6: Positiveidealalternativeforeachsampleisdefinedby
Here,allofthecriteriaareassumedtobebenefitcriteria.Ifthereexistsacostcriterion,thenwecantake compliment.
Step7: Byusing E∗ definedinDefinition5.5thesymmetricdiscriminationinformationmeasurebetween aggregatedvalueofeachalternativeandpositiveidealalternativearecalculated.
Step8: Thescoresofthese SNVs areobtainedbyusingascorefunction.
Step9: Alternativesarerankedsothattheminimumscorevalueisthebestalternative.
6.2Anapplication
Asanapplication,weconsideraninvestmentcompanywhichwantstoinvestasumofmoneyinthebest opinion.Thisproblemisadaptedfrom.37,38 Therearefouralternativesforinvestingthemoney:(1) A1 (a carcompany);(2) A2 (afoodcompany);(3) A3 (acomputercompany);and(4) A4 (anarmscompany).The investmentcompanywantstomakeadecisionusingthefollowingthreecriteria:(1) C1 (therisk);(2) C2 (thegrowth);and(3) C3 (thetheenvironmentalimpact).Theweightvectorofthecriteriaisconsideredby w =(0 35, 0 25, 0 4) andthedecisionmatrixgiveninTable1isusedasin.37,38
Let fδ(t)= sinδt with δ ∈ [0, 1].Itisclearthat fδ(t)= sinδt ≤ √t for t ∈ [0, 1] (seealsoFigure4).
Nowweconvert SNVs ofalternativesinTable1to PFVNVs byusingProposition3.7.For δ =1,we
https://doi.org/10.54216/IJNS.200208
Received:July08,2022Accepted:January11,2023
alternatives
Figure4:Thegraphsof y = √t and fδ with δ ∈ [0, 1]

Table2:PFVNVsofalternativesobtainedfor
https://doi.org/10.54216/IJNS.200208
Table5:ScoresofAlgebraicsymmetricdiscriminationinformationmeasuresbetween A+ and Aj (j = 1, 2, 3, 4)
Table6:Thecomparisonofsomepreviousmethodswithproposedmethod obtainTable2.When PFVNVs ofeachalternativewithrespecttocriteriaareaggregatedbyutilizing WAA PFVNV ,Table3containingthepositiveidealalternativeisobtained.
Algebraicsymmetricdiscriminationinformationmeasure E∗ A betweenpositiveidealalternative A+ andalternativesarecalculated.TheresultsareshowninTable4.
Scorefunction N recalledinDefinition5.8isusedtorankthevalueof E∗ A.Thesmallerthevalueof N (E∗ A(Aj ,A+)) is,thebetterthealternative Aj is.Intheotherwords,thealternative Aj isclosertopositiveidealalternative A+ as N (E∗ A(Aj ,A+)) getssmaller.TheresultsofscorefunctionareshowninTable5. Asaresult, A2 isthebestalternative.
6.3Comparativeanalysis
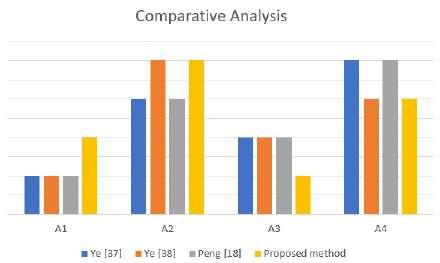
6.3.1Comparisonwiththeliterature
WecomparetheresultsofsomeexistingmethodswiththeresultsoftheproposedmethodinSub-section6.1. SoastosolvetheMCDMproblemgiveninSub-section6.2,somemethodswereproposedunderdifferent fuzzyenvironments.Ye38 gaveaweightedcorrelationcoefficientforSVNSs,utilizedittosolvethesame investmentproblemandobtainedtheranking A1 ≺ A3 ≺ A4 ≺ A2.Usingdifferentaggregationoperatorsand asimilaritymeasureforSNSs,Ye37 solvedthesameproblemandobtainedtheranking A1 ≺ A3 ≺ A2 ≺ A4. Similarly,Peng18 proposedsomeaggregationoperatorsvia t-normsand t-conorms,appliedthemtoinvestigate sameproblemandgottheranking A1 ≺ A3 ≺ A2 ≺ A4.Thebestalternativeobtainedwiththepresent methodremainssamewiththeoneof37 and38 forsome δ.Thecomparisonresultsofproposedmethodwith theexistingonesareshowninTable6andillustratedinFigure5.
Table8:Theresultswithrespectto WGA PFVNV whentheparameter δ variesfrom 0 1 and 1
6.3.2Comparisonwithrespecttoparameter δ
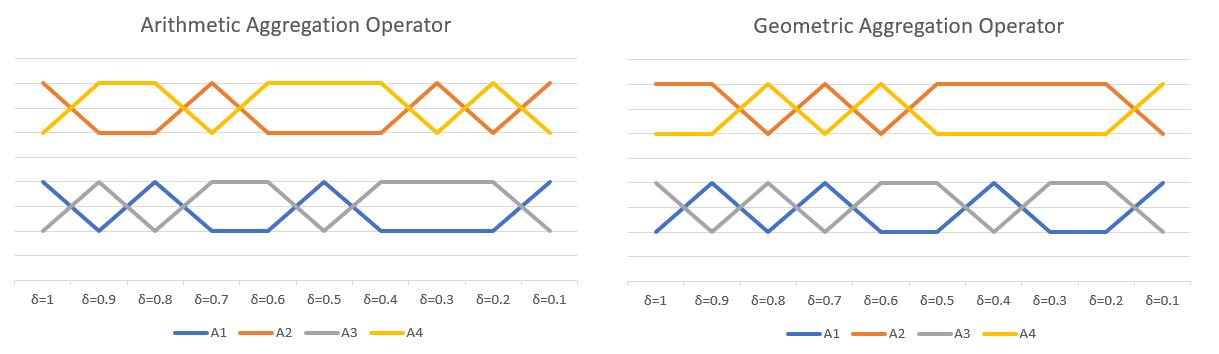
Weinvestigatetheeffectoftheparameter δ ontherankingofalternativeswithrespectto WAA PFVNV and WGA PFVNV .TheresultsaresummarizedinTable7andTable8.Fromtheseresults,itisconcluded thattherankingofthealternativeis
when
equalsto 0.1 and 1 withrespectto
.8 for
A PFVNV .When δ equalsto 0 1 and 1 withrespectto WAA PFVNV ,weconcludethat A2 isthebestalternative,whileby using WGA PFVNV ,thebestalternativeis A4 when δ equalsto 0 6 and 0 8.Thegraphicalrepresentation ofthebehaviourofthealternativeswiththe δ variationisshowninFigure6.
6.4TimecomplexityoftheproposedMCDMmethod
Inthissub-sectionweanalysisthecomplexityoftheMCDMmethodproposedinSub-section6.1.Actuallywe evaluatethetimecomplexitythatdependsonthenumberoftimesofmultiplication,exponential,summation
asin4 and.8 ConsideraMCDMproblemwith n alternativesand k criteria.InStep2weneed k operations,in Step3weneed 3kn operations,inStep4weneed 6kn operations,inStep5weneed 3n (6k +2) operations ifweusetheaggregationoperator WGA PFVNV andweneed n(75k +21) operationsifweusethe aggregationoperator WGE PFVNV .InStep6weneed 3n operations,inStep7weneed 98n operations andinStep8weneed 5n operations.Sothetimecomplexity Tnk oftheMCDMmethodis
T A nk = k +27kn +112n
fortheaggregationoperator WGA PFVNV and
T E nk = k +84kn +127n
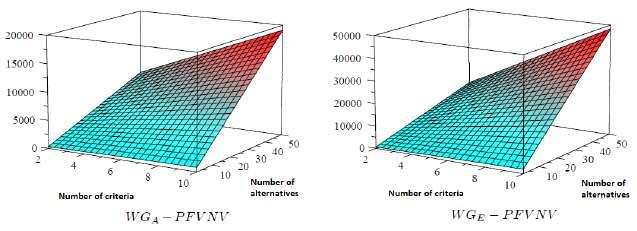
fortheaggregationoperator WGE PFVNV .Clearlythebi-variatefunctions gA,gE :[2, ∞)2 → R definedby gA(x,y)= x +27xy +112y and gE (x,y)= x +84xy +127y bothtakeabsoluteminimumat point (2, 2).Figure7illustratesthechangeofthetimecomplexitywithrespecttothechangeinthenumbers ofthealternativesandthecriteriafor WGA PFVNV and WGE PFVNV .
7Conclusion
ThemainaimofthisstudyistointroducetheconceptofPFVNSconstructedbyconsideringPFVsrather thannumbers,inspiredbyIFVNSs.Thus,aPFVNSisanextensionofIFVNSs.PFVNSsareusedtoexpress uncertaintyinamoreextendedfuzzyenvironment.Therefore,largerinformationcanbekeptwhilethedata isconvertedtoaFS.Inthisway,informationlossisprevented.Inthisstudy,somesetoperationsbetween PFVNSsareproposed.WealsointroducePythagoreanfuzzy t-normsand t-conormswithmotivationfrom intuitionisticfuzzy t-normsand t-conorms.WeshowthatsomePythagoreanfuzzy t-normsand t-conorms areexpressedviacontinuousArchimedean t-normsandcontinuousArchimedean t-conormson [0, 1].Then wedefinesomealgebraicoperationsbetweenPFVNVsbyutilizingcontinuousArchimedean t-normandcontinuousArchimedean t-conormson [0, 1].Bywayofthesealgebraicoperations,someweightedaggregation operatorsareproposed.InputvaluesrepresentedbyPFVNVsaretransformedtoasingleoutputvaluebyusingweightedaggregationoperators.Also,weintroduceamethodthatconvertsneutrosophicfuzzyvaluesto PFVNVs.Bydefiningasimplifiedneutrosophicvaluedmodifiedfuzzycross-entropymeasurewemanageto rankoutputvaluesrepresentedbyPFVNVswiththehelpofascorefunctioninsimplifiedneutrosophicenvironment.NextaMCDMmethodisgiventoseepracticabilityoftheproposedtheory.Theproposedmethodis madeuseoftosolveaMCDMproblemadaptedfromtheliteratureinPythagoreanfuzzyvaluedneutrosophic environment.Acomparisonanalysisandacomplexityanalysisarealsoprovided.Inthefuture,differentkind ofaggregationoperatorsandcross-entropymeasurescanbeconsidered.Theapplicationsoftheproposed theorycanbeextendedtosomedecisionmakingproblemssuchasfacerecognitionsystems,classificationand medicaldiagnosis.
Funding “TheresearchofMahmutCanBozyi˘githasbeensupportedbyTurkishScientificandTechnological ResearchCouncil(TUBITAK)Program2211.”
ConflictsofInterest: “Theauthorsdeclarenoconflictofinterest.”
https://doi.org/10.54216/IJNS.200208
Received:July08,2022Accepted:January11,2023
References
[1] Abdullah,L.,Fuzzymulticriteriadecisionmakinganditsapplications:abriefreviewofcategory. Procedia-SocialandBehavioralSciences,97,131-136,2013.
[2] Atanassov,K.T.,Intuitionisticfuzzysets.InIntuitionisticfuzzysets(pp.1-137).Physica,Heidelberg, 1999.
[3] Beliakov,G.,Bustince,H.,Goswami,D.P.,Mukherjee,U.K.,Pal,N.R.,Onaveragingoperatorsfor Atanassov’sintuitionisticfuzzysets.InformationSciences.181,1116-1124,2011.
[4] Chang,D.Y.,ApplicationsoftheextentanalysismethodonfuzzyAHP.Europeanjournalofoperational research,95(3),649-655,1996.
[5] Cover,T.M.,andThomas,J.A.,Elementsofinformationtheory2ndedition(wileyseriesintelecommunicationsandsignalprocessing),2006.
[6] Deschrijver,G.,Cornelis,C.,Kerre,E.E.,Ontherepresentationofintuitionisticfuzzy t-normsand t-conorms.IEEEtransactionsonfuzzysystems,12(1),45-61,2004.
[7] Garg,H.,Arora,R.,GeneralizedMaclaurinsymmetricmeanaggregationoperatorsbasedon Archimedean t-normoftheintuitionisticfuzzysoftsetinformation.ArtifIntellRev54:3173-3213,2021.
[8] Junior,F.R.L.,Osiro,L.,andCarpinetti,L.C.R.,AcomparisonbetweenFuzzyAHPandFuzzyTOPSIS methodstosupplierselection.Appliedsoftcomputing,21,194-209,2014.
[9] Kahraman,C.,(Ed.)Fuzzymulti-criteriadecisionmaking:theoryandapplicationswithrecentdevelopments(Vol.16).SpringerScienceandBusinessMedia,2008.
[10] Klement,E.P.,Mesiar,R.,Pap,E.,Triangularnorms,KluwerAcademicPublishers,Dordrecht,2002.
[11] Klement,E.P.,Mesiar,R.,Pap,E.,Triangularnorms.PositionpaperIII:continuous t-norms,FuzzySets andSystems,145(3)439-454,2004.
[12] Klir,G.Yuan,B.,FuzzySetsandFuzzyLogic:TheoryandApplications,PrenticeHall,UpperSaddle River,NJ,1995.
[13] Kullback,S.,Leibler,R.A.,Oninformationandsufficiency.AnnalsoftheInstituteofStatisticalMathematics.4:99–111,1951.
[14] Kullback,S.,Informationtheoryandstatistics.Wiley,NewYork,1959.
[15] Lin,J.,DivergencemeasuresbasedonShannonentropy,IEEETrans.InformationTheory37(1)145-151, 1991.
[16] Menger,K.,Statisticalmetrics.ProceedingsoftheNationalAcademyofSciencesoftheUnitedStatesof America,28(12),535,1942.
[17] Naz,S.,andAkram,M.,Noveldecision-makingapproachbasedonhesitantfuzzysetsandgraphtheory. ComputationalandAppliedMathematics,38(1),1-26,2019.
[18] Peng,J.J.,Wang,J.Q.,Wang,J.,Zhang,H.Y.,Chen,X.H.,Simplifiedneutrosophicsetsandtheir applicationsinmulti-criteriagroupdecision-makingproblems.Internationaljournalofsystemsscience, 47(10),2342-2358,2016.
[19] Peng,X.,Yang,Y.,SomeresultsforPythagoreanfuzzysets.InternationalJournalofIntelligentSystems, 30(11),1133-1160,2015.
[20] Peng,X.,Yuan,H.,Yang,Y.Pythagoreanfuzzyinformationmeasuresandtheirapplications.InternationalJournalofIntelligentSystems,32(10),991-1029,2017.
[21] Schweizer,B.andSklar,A.,Probabilisticmetricspaces,North-Holland,NewYork,1983.
[22] Shang,X.G.,Jiang,W.S.,Anoteonfuzzyinformationmeasures.PatternRecognitionLetters,18(5), 425-432,1997.
https://doi.org/10.54216/IJNS.200208
Received:July08,2022Accepted:January11,2023
[23] Shannon,C.,Amathematicaltheoryofcommunication.BellSyst.,Tech.J.27:379-423,1948.
[24] Shannon,C.,Weaver,W.,Themathematicaltheoryofcommunication.Urbana:UniversityofIllinois Press,1949.
[25] Singh,A.,Joshi,D.K.,Kumar,S.,Anovelconstructionmethodofintuitionisticfuzzysetfromfuzzyset anditsapplicationinmulti-criteriadecision-makingproblem.InAdvancedComputingandCommunicationTechnologies(pp.67-75).Springer,Singapore,2019.
[26] Smarandache,F.,Aunifyingfieldoflogics.Neutrosophy:Neutrosophicprobability,setandlogic.AmericanResearchPress,Rehoboth,1998.
[27] Sun,B.,Tong,S.,Ma,W.,Wang,T.,andJiang,C.,AnapproachtoMCGDMbasedonmulti-granulation Pythagoreanfuzzyroughsetovertwouniversesanditsapplicationtomedicaldecisionproblem.Artificial IntelligenceReview,55,1887–1913,2022.
[28] Unver,M.,Turkarslan,E.,Olgun,M.,Ye,J.,Intuitionisticfuzzy-valuedneutrosophicmulti-setsand numericalapplicationstoclassification.ComplexandIntelligentSystems,1-19,2022.
[29] Unver,M.,Olgun,M.,andT¨urkarslan,E.,CosineandcotangentsimilaritymeasuresbasedonChoquet integralforSphericalfuzzysetsandapplicationstopatternrecognition.JournalofComputationaland CognitiveEngineering,1(1)21-31,2022.
[30] Vlachos,I.K.,Sergiadis,G.D.,Intuitionisticfuzzyinformation-Applicationstopatternrecognition, PatternRecognitionLetters28(2007)197-206,2007.
[31] Wei,P.,Ye,J.,Improvedintuitionisticfuzzycross-entropyanditsapplicationtopatternrecognitions.In 2010IEEEInternationalConferenceonIntelligentSystemsandKnowledgeEngineering(pp.114-116). IEEE,2010.
[32] Wu,X.H.,Wang,J.Q.,Peng,J.J.,Chen,X.,H.Cross-entropyandprioritizedaggregationoperatorwith simplifiedneutrosophicsetsandtheirapplicationinmulti-criteriadecision-makingproblems.InternationalJournalofFuzzySystems,18(6),1104-1116,2016.
[33] Yager,R.R.,Pythagoreanmembershipgradesinmulticriteriadecisionmaking.IEEETransactionson FuzzySystems,22(4),958-965,2013a.
[34] Yager,R.R.,Pythagoreanfuzzysubsets.In2013jointIFSAworldcongressandNAFIPSannualmeeting (IFSA/NAFIPS)(pp.57-61).IEEE,2013b.
[35] Yager,R.R.,andAbbasov,A.M.,Pythagoreanmembershipgrades,complexnumbers,anddecision making.InternationalJournalofIntelligentSystems,28(5),436-452,2013.
[36] Ye,S.,Ye,J.,Dicesimilaritymeasurebetweensinglevaluedneutrosophicmultisetsanditsapplication inmedicaldiagnosis.NeutrosophicSetsSyst.6:48-53,2014.
[37] Ye,J.,Amulticriteriadecision-makingmethodusingaggregationoperatorsforsimplifiedneutrosophic sets.JournalofIntelligentandFuzzySystems,26(5),2459-2466,2014.
[38] Ye,J.,Multicriteriadecision-makingmethodusingthecorrelationcoefficientundersingle-valuedneutrosophicenvironment.InternationalJournalofGeneralSystems,42(4),386-394,2013.
[39] Ye,J.,Single-ValuedNeutrosophicClusteringAlgorithmsBasedonSimilarityMeasures.Journalof Classification,34(1),2017.
[40] Ye,J.,T¨urkarslan,E., Unver,M.,Olgun,M.,AlgebraicandEinsteinweightedoperatorsofneutrosophic enthalpyvaluesformulti-criteriadecisionmakinginneutrosophicmulti-valuedsetsettings.Granular Computing,1-9,2021.
[41] Yeni,F.B.,and Ozc¸elik,G.,Interval-valuedAtanassovintuitionisticFuzzyCODASmethodformulti criteriagroupdecisionmakingproblems.GroupDecisionandNegotiation,28(2),433-452,2019.
[42] Zadeh,L.A.,Fuzzysets.Informationandcontrol.8(3):338-353,1965.
[43] Zadeh,L.A.,Probabilitymeasuresoffuzzyevents.Journalofmathematicalanalysisandapplications, 23(2),421-427,1968.
https://doi.org/10.54216/IJNS.200208