Single-ValuedNeutrosophicHesitantFuzzyChoquet AggregationOperatorsforMulti-Attribute DecisionMaking
XinLi 1 ID andXiaohongZhang 1,2,* ID
1 CollegeofArtsandSciences,ShanghaiMaritimeUniversity,Shanghai201306,China; Smu201631010013@163.com
2 SchoolofArtsandSciences,ShaanxiUniversityofScience&Technology,Xi’an710021,China
* Correspondence:zhangxiaohong@sust.edu.cnorzhangxh@shmtu.edu.cn
Received:12January2018;Accepted:15February2018;Published:22February2018
Abstract: Thispaperaimsatdevelopingnewmethodsformulti-attributedecisionmaking(MADM) underasingle-valuedneutrosophichesitantfuzzyenvironment,inwhicheachelementhassetsof possiblevaluesdesignedbytruth,indeterminacy,andfalsitymembershiphesitantfunctions.First, takingadvantageoftheChoquetintegralandthatitcanreflectmorecorrelationsofattributes inMADM,twoaggregationoperatorsaredefinedbasedontheChoquetintegral,specifically, thesingle-valuedneutrosophichesitantfuzzyChoquetorderedaveraging(SVNHFCOA)operator andsingle-valuedneutrosophichesitantfuzzyChoquetorderedgeometric(SVNHFCOG)operator, andtheirpropertiesarealsodiscussedindetail.Then,novelMADMapproachesbasedonthe SVNHFCOAandSVNHFCOGoperatorsareestablishedtoprocesssingle-valuedneutrosophic hesitantfuzzyinformation.Finally,thisworkprovidesanumericalexampleofinvestment alternativestovalidatetheapplicationandeffectivenessoftheproposedapproaches.
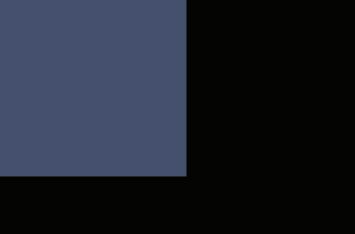
Keywords: multi-attributedecisionmaking(MADM);aggregationoperator;single-valuedneutrosophic hesitantfuzzyset(SVNHFS);theChoquetintegral
1.Introduction
Multi-attributedecisionmaking(MADM)isacommonapproachtohelppeopletoselectthe mostdesirablealternative(s)fromsomefeasiblechoices.However,inmanycases,decisionmakers finditishardtopreciselyexpresstheirpreferencesunderimpreciseanduncertainenvironment[1]. Then,fuzzyset(FS)theory,asproposedbyZadeh,isproventobeusefultodealwithfuzzyMADM problems,especiallywhensubjectiveassessmentsareinvolved[2].OnbasisofZadeh’swork,rough set(RS),fuzzymultiset(FMS),hesitantfuzzyset(HFS),Interval-valuedintuitionisticfuzzyset(IVIFS), andneutrosophicset(NS)havebeensuccessivelyproposedandsuccessfullyappliedindealingwith variousuncertainproblemsinartificialintelligence,patternrecognition,informationfusion,andso on[3–7].InthepracticalapplicationofFSsanditsextensions,utilizingvarioustechniquestoaggregate informationinMADMisofvitalimportance,andtheaggregationoperatorsareoneoftheseeffective techniquestoaggregatedataintheinformationfusionprocess[8,9].
AsageneralizationofFSs,theNSwasintroducedbySmarandachetodealwithincomplete, indeterminate,andinconsistentdecisioninformation,whichincludetruth,falsity,andindeterminacy memberships,andtheircorrespondinglymembershipfunctions TA (x), IA (x), FA (x) arenon-standard subsetsof ] 0,1+ [ [7].However,withoutaspecificdescription,itisdifficulttoapplytheNSin realscientificandotherareas.Therefore,someresearchersproposedtheintervalneutrosophicset (INS),single-valuedneutrosophicset(SVNS),multi-valuedneutrosophicset(MVNS),andrough neutrosophicset(RNS)in[10–14],andstudiedtheirrelatedpropertiesindetail,amongthem, Symmetry 2018, 10,50;doi:10.3390/sym10020050 www.mdpi.com/journal/symmetry
themembershipfunctionsofSVNSsarestandardsubsetsof [0,1].Thestudyonaggregation operatorsisapracticalsubject,whichhasbeenreceivedgrowingattention.ForSVNSs, single-valuedneutrosophicaveraging/geometrical(SVNA/G)operators,correlatedaggregation operators,Hamacheraggregationoperators,andChoquetintegraloperatorsundersingle-valued neutrosophicenvironmentareproposedinrecentyears[15–17].AsanothergeneralizationofFSs, theHFSwasdefinedbyTorra,whichpermititsmembershipfunctiontohaveasetofpossible values[18,19].Somehesitantfuzzyaggregationoperatorshavealsobeenputforward,such asthehesitantfuzzyweightedaveraging/geometric(HFWA/G)operators,thehesitantfuzzy orderedweightedaveraging/geometric(HFOWA/G)operators,thehesitantfuzzyChoquetordered averaging/geometric(HFCOA/G)operators,thegeneralizedhesitantfuzzyChoquetordered averaging/geometric(GHFCOA/G)operators,andetc,whichhavebeenwidelyusedtodealwith MADMproblemsunderhesitantfuzzyenvironment[20–23].
Furthermore,Yeproposedtheconceptofthesingle-valuedneutrosophichesitantfuzzyset (SVNHFS)bycombiningtheadvantagesoftheSVNSandHFS,whichencompassesFS,HFS,DHFS, SVNHFSasspecialcases,andpermitsitsmembershipfunctionstohavesetsofpossiblevalues,which aredenotedbytruth,indeterminacy,andfalsitymembershiphesitantfunctions[24].Onbasisof theSVNSandHFS,somepropertiesofSVNHFSswerediscussedtosolveMADMproblems;for instance,YeintroducedthebasicoperationalrelationsandcosinemeasurefunctionofSVNHFSs anddevelopedthesingle-valuedneutrosophichesitantfuzzyweightedaveraging/geometric (SVNHFWA/G)operators[24].Sahinfurtherstudiedthecorrelationandcorrelationcoefficient ofSVNHFSs[25].DistanceandsimilaritymeasuresforMADMwithsingle-valuedneutrosophic hesitantfuzzyinformationaredefinedin[26].Liu.PdefinedtheHammingdistancemeasureand neutrosophichesitantfuzzyHeronianmeanaggregationoperatorsofSVNHFSsandthenextendedthe VIKORmethodtoprocessthesingle-valuedneutrosophichesitantfuzzyinformation[27].BISWAS putforwardsomeweighteddistancemeasuresofSVNHFSs[28].Liu.Cproposedthesingle-valued neutrosophichesitantfuzzyorderedweightedaveraging/geometric(SVNHFOWA/G)operatorsand appliedthemtodealwithpracticalMADMproblems[29].
IntheexistingresearchonMADMmethodswithsingle-valuedneutrosophichesitantfuzzy information,itisgenerallyassumedthattheattributesareindependent,whicharecharacterized byanindependentindex.However,forpracticalMADMproblems,duetothedependenceamong attributes,morecorrelationsarerequiredtobeconsidered.Forexample,whenweusethreecourses asattributestomeasurecomprehensiveperformancesofstudents,i.e.,English,probabilitytheory, andmathematicalstatistics,obviously,studentswithhighperformanceinprobabilitytheoryare highlylikelytohavehighscoresinmathematicalstatistics,sothethreeindexescannotberegardedas independentfromeachother,weneedtofurtherconsidertheinterrelationshipsamongtheseattributes. TheChoquetintegralprovidesanapproachtoprocessthecorrelationamongtheattributesinMADM withrespecttofuzzymeasure,andvariouskindsofhesitantfuzzyChoquetaggregationoperators, intuitionisticfuzzyChoquetaggregationoperators,neutrosophicChoquetaggregationoperatorshave beenputforwardtodealwithMADMproblemsunderfuzzyenvironment[30–34].
Therefore,itisnecessarytodevelopsomesingle-valuedneutrosophichesitantfuzzyoperators basedonChoquetintegraltoconsidermorecorrelationsbetweenattributesinMADMproblem.Thus, thepurposesofthisarticleareto(i)introducetheChoquetintegralwithrespecttofuzzymeasuresto intoSVNHFSstoconsidermorecorrelationsamongattributesinMADM,(ii)proposeasingle-valued neutrosophichesitantfuzzyChoquetorderedaveraging(SVNHFCOA)operatorandasingle-valued neutrosophichesitantfuzzyChoquetorderedgeometric(SVNHFCOG)operator(iii)establishMADM methodsbasedontheSVNHFCOAandSVNHFCOGoperatorsundersingle-valuedneutrosophic hesitantfuzzyenvironment.
Todoso,therestofthispaperisorganizedasfollows:Section 2 recallssomebasicconcepts relatedtotheChoquetintegral,SVNS,HFS,andSVNHFS;InSection 3,theSVNHFCOAand SVNHFCOGoperatorsareputforwardandbasicpropertiesofthemarediscussed;InSection 4,
weputforwardMADMmethodsbasedontheSVNHFCOAandSVNHFCOGoperatorsunder single-valuedneutrosophichesitantfuzzyenvironment;Section 5 utilizesanillustrativeexample tovalidatetheproposedMADMapproaches.Finally,conclusionsandfutureresearchdirectionsare drawninSection 6.
2.Preliminaries
SomebasicconceptsrelatedtotheSVNHFSandChoquetintegralarebrieflyreviewedinthissection.
2.1.Single-ValuedNeutrosophicHesitantFuzzySets(SVNHFS)
AsageneralizationofFSs,theSVNHFSisacombinationoftheSVNSwithHFS.
Definition1. ([10]) LetXbeanon-emptyfixedset,aSVNSonXisdefinedas:
A = {(x, TA (x), IA (x), FA (x))|x ∈ X },(1)
where TA (x), IA (x), FA (x) ∈ [0,1], denotingthetruth,indeterminacyandfalsitymembershipdegreeofthe elementx ∈ X,respectively,andsatisfyingthelimit: 0 ≤ TA (x)+ IA (x)+ FA (x) ≤ 3.
Definition2. ([18]) LetXbeanon-emptyfixedset,aHFSonXisrepresentedby: E = { x, hE (x) |x ∈ X},(2) wherehE (x) isasetofvaluesin [0,1],denotingthemembershiphesitantdegreesofx ∈ X.
Definition3. ([24]) LetXbeanon-emptyfixedset,aSVNHFSonXisexpressedby:
N = {(x, t(x), i(x), f (x))|x ∈ X },(3) where t(x)= { γ|γ ∈ t(x)}, i(x)= { δ|δ ∈ i(x)}, f (x)= { η|η ∈ f (x)} arethreesetswithvaluesin [0,1], representingtruth,indeterminacyandfalsitymembershiphesitantdegreesoftheelement x ∈ X,whichsatisfy limits: γ ∈ [0,1], δ ∈ [0,1], η ∈ [0,1] and 0 ≤ sup γ + sup δ + sup η ≤ 3.
Forconvenience,wecall n =(t(x), i(x), f (x)) aneutrosophichesitantfuzzyelement(SVNHFE), somebasicoperationsofSVNHFEsaredefinedbyYe[24],asfollows: Definition4.
a(n)= 1 l l ∑ i=1 γi 1 q q ∑ i=1 (1 ηi );(5) wherel,p,qarethenumberofthevaluesin t, i, f .Obviously, s(n), a(n) ∈ [0,1] If s(n1) > s(n2),then n1 > n2;ifs(n1)= s(n2) anda(n1) > a(n2),then n1 > n2
2.2.TheFuzzyMeasureandChoquetIntegral
TheChoquetintegralisapowerfuloperatortoaggregatekindsoffuzzyinformationinMADM withrespecttofuzzymeasure. Definition6. ([31]) Let (X, A, µ) beameasurablespaceand µ : A→ [0,1] ,ifitsatisfiestheconditions: 1. µ(∅)= 0;
µ(A) ≤ µ(B) wheneverA ⊂ B, A, B ∈A; 3. IfA1 ⊂ A2 ⊂
⊂ An ⊂ ..., An ∈A, then µ (∪∞ n=1 An )= limn→∞ µ(An ) ; 4. IfA1 ⊃ A2 ⊃ ... ⊃ An ⊃ ..., An ∈A, then µ (∩∞ n=1 An )= limn→∞ µ(An ) ; thenwecall µ beafuzzymeasuredefinedbySugenoM.
Inaddition,toavoidtheproblemswithcomputationalcomplexity, gλ fuzzymeasure,aspecial kindoffuzzymeasure,wasproposedbySugenoM[31],whichsatisfiestheadditionalproperties: µ(A ∪ B)= µ(A)+ µ(B)+ λµ(A)µ(B), λ ∈ ( 1, ∞) forall A, B ∈A and A ∩ B = ∅.Specially, theexpressionof gλ fuzzymeasureonafinitesetcanbesimplifiedasfollows: Theorem1. ([31]) WhenXisafiniteset(X={x1, x2,..., xm}),gλ fuzzymeasurecanbeexpressedas: µ(X)= 1 λ ( ∏ i∈X (1 + λµ(xi )) 1), if λ = 0, ∑ i∈X µ(xi ), if λ = 0, (6) wherexi ∩ xj = ∅ foralli, j = 1,2, , mandi = j. Definition7. ([32]) Let µ beafuzzymeasure,X={x1, x2, , xm}beafiniteset.TheChoquetintegralofa functionf : X → [0,1] withrespecttofuzzymeasure µ isexpressedasfollows: fdµ = m ∑ i=1 (µ(Fφ(i) ) µ(Fφ(i 1) )) f (xφ(i) ),(7) where (φ(1), φ(2),..., φ(m)) isapermutationof (1,2,..., n) suchthat f (xφ(1) ) ≥ f (xφ(2) ), ,
where µφ(j) = µ(Fφ(j) ) µ(Fφ(j 1) ) and (φ(1), φ(2),..., φ(m)) isapermutationof (1,2,..., m) suchthat nφ(1) ≥ nφ(2),..., nφ(m),Fφ(i) = {xφ(1), xφ(2),..., xφ(i) } andFφ(0) = ∅.
Theorem2. Let nj (j = 1,2,..., m) beacollectionofSVNHFEs,thentheaggregatedvalueobtainedbythe SVNHFCOAoperatorisalsoaSVNHFE,and SVNHFCOAµ {n1, n2,..., nm } = ⊕m j=1((µ(Fφ(j) ) µ(Fφ(j 1) ))nφ(j) ) = ∪ γφ(j) ∈tφ(j) , σφ(j) ∈iφ(j) ,ηφ(j) ∈ fφ(j) {{1 m ∏ j=1 (1 γφ(j) )µφ(j) }, { m ∏ j=1 σφ(j) µφ(j) }, { m ∏ j=1 ηφ(j) µφ(j) }} (9)
Proof. Bymeansofmathematicalinduction,theproofofTheorem2canbedone,i.e.,theaggregated valuewithSVNHFCOAoperatorisalsoaSVNHFE.
(a) For m = 1,since SVNHFCOAµ {n1} =(µ(Fφ(1) ) µ(Fφ(0) ))nφ(1) = nφ(1), Obviously,Equation(9)holdsfor m = 1. (b) When m = 2,since µφ(1) nφ(1) = ∪ γφ(1) ∈tφ(1) , σφ(1) ∈iφ(1) ,ηφ(1) ∈ fφ(1) {1 (1 γφ(1) )µφ(1) , σφ(1) µφ(1) , ηφ(1) µφ(1) }, µφ(2) nφ(2) = ∪ γφ(2) ∈tφ(2) , σφ(2) ∈iφ(2) ,ηφ(2) ∈ fφ(2)
{1 (1 γφ(2) )µφ(2) , σφ(2) µφ(2) , ηφ(2) µφ(2) } then,wehave
SVNHFCOAµ {n1, n2} = µφ(1)nφ(1) ⊕ µφ(2)nφ(2) = ∪ γφ(j) ∈tφ(j), σφ(j) ∈iφ(j),ηφ(j) ∈ fφ(j) {{1 (1 γφ(1))µφ(1) (1 γφ(2))µφ(2) }, {σφ(1) µφ(1) σφ(2) µφ(2) }, {ηφ(1) µφ(1) ηφ(2) µφ(2) }} Thus,Equation(9)holdsfor m = 2.
(c) IfEquation(9)holdsfor m = k,then
SVNHFCOAµ {n1, n2,..., nk } = ⊕k j=1µφ(j) nφ(j) = ∪ γφ(j) ∈tφ(j) , σφ(j) ∈iφ(j) ,ηφ(j) ∈ fφ(j) {{1 k ∏ j=1 (1 γφ(j) )µφ(j) }, { k ∏ j=1 σφ(j) µφ(j) }, { k ∏ j=1 ηφ(j) µφ(j) }}
When m = k + 1, for ∀γφ(j) ∈ tφ(j), σφ(j) ∈ iφ(j), ηφ(j) ∈ fφ(j) ,then, SVNHFCOAµ {n1, n2,..., nk+1} = ⊕k+1 j=1 µφ(j) nφ(j) = ∪ γφ(j) ∈tφ(j) , σφ(j) ∈iφ(j) ,ηφ(j) ∈ fφ(j) {{1 k ∏ j=1 (1 γφ(j) )µφ(j) }, { k ∏ j=1 σφ(j) µφ(j) }, { k ∏ j=1 ηφ(j) µφ(j) }}⊕ ∪ γφ(k+1) ∈tφ(k+1) , σφ(k+1) ∈iφ(k+1) ,ηφ(k+1) ∈ fφ(k+1) {1 (1 γφ(k+1) )µφ(k+1) , σφ(k+1) µφ(k+1) , ηφ(k+1) µφ(k+1) }
whichcanbeobtainedby (⊕) calculation,
SVNHFCOAµ {n1, n2,..., nk+1} = ⊕k+1 j=1 µφ(j) nφ(j) = ∪ γφ(j) ∈tφ(j) , σφ(j) ∈iφ(j) ,ηφ(j) ∈ fφ(j) {{1 k+1 ∏ j=1 (1 γφ(j) )µφ(j) }, { k+1 ∏ j=1 σφ(j) µφ(j) }, { k+1 ∏ j=1 ηφ(j) µφ(j) }}. i.e.,Equation(9)holdsform = k + 1,thusweconfirmEquation(9)holdsforallm.
SomespecialcasesoftheSVNHFCOAoperatoraregivenasfollows:
1. If µ(F) ≡ 1,thenSVNHFCOAµ {n1, n2,..., nm } = max{n1, n2,..., nm }; 2. If µ(F) ≡ 0,thenSVNHFCOAµ {n1, n2,..., nm } = min{n1, n2,..., nm };
3. TheSVNHFCOAoperatorreducestothesingle-valuedneutrosophichesitantfuzzyweighted averaging(SVNHFWA)operator,iftheindependentcondition µ(xφ(j))= µ(Fφ(j)) µ(Fφ(j 1)) holds SVNHFWA{n1, n2,..., nm } = ⊕m j=1(µ(xj ) · nj ) = ∪ γj ∈tJ ,σj ∈lZ J ,ηj ∈ f J 1 m ∏ j=1 (1 γj )µ(xj ) , m ∏ j=1 σj µ(xj ) , m ∏ j=1 ηj µ(xj )
4. If µ(xj)= 1/m, forj = 1,2, , m,thenboththeSVNHFCOAandSVNHFWAoperatorsreduce tothesingle-valuedneutrosophichesitantfuzzyaveraging(SVNHFA)operator,whichisshown asfollows:
SVNHFWA{n1, n2,..., nm } = ∪ γj ∈tj , σj ∈ij ,ηj ∈ f j {{1 m ∏ j=1 (1 γj ) 1 m }, { m ∏ j=1 (σj ) 1 m }, { m ∏ j=1 (ηj ) 1 m }}
5. If µ(F)= ∑|F| j=1 ωj forall F ⊆ X, where |F| isthenumberofelementsinF,then ωj = µ(Fφ(j) ) µ(Fφ(j 1) ), j = 1,2, , m, where ω =(ω1, ω2,..., ωm)T suchthat ωj ≥ 0 and ∑m j=1 ωj = 1 Inthiscase,theSVNHFCOAoperatorreducestothesingle-valuedneutrosophichesitantfuzzy orderedweightedaveraging(SVNHFOWA)operatoras:
SVNHFOWA{n1, n2,..., nm } = ∪ γj ∈tj , σj ∈izj ,ηj ∈ f j {{1 m ∏ j=1 (1 γωj )ωj }, { m ∏ j=1 σj ωj }, { m ∏ j=1 ηj ωj }};
Particularly,if µ(F)= |F|/m,forall F ⊆ X, thenboththeSVNHFCOAandSVNHFOWAoperators reducetotheSVNHFAoperator.
Theorem3. TheSVNHFCOAoperatorhasthefollowingdesirableproperties: 1. (Idempotency)Let nj = nforallj = 1,2,..., m, and n = {{γ}, {σ}, {η}},then: SVNHFCOAµ {n1, n2,..., nm } = {{γ}, {σ}, {η}} 2. (Boundedness)Let n = {min{γj }, max{σj }, max{ηj }}, n+ = { max{ γj }, min{σj }, min{ηj }}, so: n ≤ SVNHFCOAµ {n1, n2,..., nm }≤ n+ 3. (Commutativity)If {n1, n2,..., nm } isapermutationof {n1, n2,..., nm },then, SVNHFCOAµ {n1, n2,..., nm } = SVNHFCOAµ {n1, n2,..., nm }
4. (Monotonity)If nj ≤ nj for ∀j ∈{1,2,..., n},then, SVNHFCOAµ {n1, n2,..., nm }≤ SVNHFCOAµ {n1, n2,..., nm }.
Proof. Suppose (1,2,..., m) isapermutationsuchthat n1 ≥ n2,..., ≥ nm
1. For n = {{γ}, {σ}, {η}},accordingtoTheorem1,itfollowsthat SVNHFCOAµ {n1, n2,..., nm} = {{1 (1 γ)∑m j 1 (µ(Fj ) µ(Fj 1)) }, {σ∑m j 1 (µ(Fj ) µ(Fj 1)) }, {η∑m j 1 (µ(Fj ) µ(Fj 1)) }} = {{γ}, {σ}, {η}}
2. Since y = xa (0 < a < 1) isamonotoneincreasingfunctionwhen x > 0,therefore,itholds 1 m ∏ j=1 (1 min{γj })(µ(Fj ) µ(Fj 1)) ≤ 1 m ∏ j=1 (1 γj )(µ(Fj ) µ(Fj 1)) ≤ 1 m ∏ j=1 (1 max{γj })(µ(Fj ) µ(Fj 1)) , whichisequivalentto 1 (1 min{γj })∑m j=1 (µ(Fj ) µ(Fj 1)) ≤ 1 m ∏ j=1 (1 γj )(µ(Fj ) µ(Fj 1)) ≤ 1 (1 max{γj })∑m j 1 (µ(Fj ) µ(Fj 1)) i.e., min{γj }≤ 1 m ∏ j=1 (1 γj )(µ(Fj ) µ(Fj 1)) = γ ≤ max{γj } Analogously,wehave min{σj }≤ m ∏ j=1 σj (µ(Fj ) µ(Fj 1)) ≤ max{σj } andmin{ηj }≤ m ∏ j=1 ηj (µ(Fj ) µ(Fj 1)) ≤ max{ηj } Since s(n ) ≤ S(n) ≤ s(n+ ),namely, n ≤ SVNHFCOAµ {n1, n2,..., nm }≤ n+
3. Suppose (φ(1), φ(2),..., φ(m)) isapermutationofboth {n1, n2,..., nm } and {n1, n2,..., nm }, suchthat nφ(1) ≥ nφ(2),..., ≥ nφ(m), Fφ(i) = {xφ(1), xφ(2),..., xφ(i) },then, SVNHFCOAµ {n1, n2,..., nm} = SVNHFCOAµ {n1, n2,..., nm} = ⊕m j=1((µ(Fφ(j) ) µ(Fφ(j 1) ))nφ(j) )
4. Considering nj ≤ nj for ∀j ∈{1,2,..., n},wehave γ = 1 m ∏ j=1 (1 γj )(µ(Fj ) µ(Fj 1)) ≤ 1 m ∏ j=1 (1 γj )(µ(Fj ) µ(Fj 1)) = γ , σ = m ∏ j=1 σj µ(Fj ) µ(Fj 1) ≥ m ∏ j=1 (σj )µ(Fj ) µ(Fj 1) = σ , η = m ∏ j=1 ηj µ(Fj ) µ(Fj 1) ≥ m ∏ j=1 (ηj )µ(Fj ) µ(Fj 1) = η . Since s(nj ) ≤ s(nj ),namely, SVNHFCOAµ {n1, n2,..., nm }≤ SVNHFCOAµ {n1, n2,..., nm }
3.2.Single-ValuedNeutrosophicHesitantFuzzyChoquetOrderedGeometric(SVNHFCOG)Operator
Similarly,wecandeveloptheSVNHFCOGoperatorforSVNHFSs: Definition9. Let nj (j = 1,2,..., m) beacollectionofSVNHFEs,Xbethesetofattributesand µ befuzzy measuresonX,thentheSVNHFCOGoperatorisdefinedasfollows:
SVNHFCOGµ {n1, n2,..., nm } = ⊗m j=1(nφ(j) (µ(Fφ(j) ) µ(Fφ(j 1) )) ),(10) where µφ(j) = µ(Fφ(j) ) µ(Fφ(j 1) ) and (φ(1), φ(2),..., φ(m)) isapermutationof (1,2,..., m) suchthat nφ(1) ≥ nφ(2),..., nφ(m), Fφ(i) = {xφ(1), xφ(2),..., xφ(i) } andFφ(0) = ∅
Theorem4. Let nj (j = 1,2,..., m) beacollectionofSVNHFEs,thentheaggregatedvalueobtainedbythe SVNHFCOAoperatorisalsoaSVNHFE,and SVNHFCOGµ {n1, n2,..., nm} = ⊗m j=1(nφ(j) (µ(Fφ(j) ) µ(Fφ(j 1) ))) = ∪ γφ(j) ∈ tφ(j), σφ(j) ∈ iφ(j), ηφ(j) ∈ fφ(j)
{{ m ∏ j=1 (γφ(j))µφ(j) }, {1 m ∏ j=1 (1 σφ(j))µφ(j) }, {1 m ∏ j=1 (1 ηφ(j))µφ(j) }} (11)
SomespecialcasesoftheSVNHFCOAoperatoraregivenasfollows:
1. If µ(F) ≡ 1,thenSVNHFCOGµ {n1, n2,..., nm } = max{n1, n2,..., nm };
2. If µ(F) ≡ 0,thenSVNHFCOGµ {n1, n2,..., nm } = min{n1, n2,..., nm };
3. TheSVNHFCOGoperatorreducestothesingle-valuedneutrosophichesitantfuzzyweighted geometric(SVNHFWG)operator,iftheindependentcondition µ(xφ(j) )= µ(Fφ(j) ) µ(Fφ(j 1) ) holds.
SVNHFWG{n1, n2,..., nm } = ⊗m j=1(µ(xj ) ⊗ nj ) = ∪ γj ∈tj , σj ∈izj ,ηj ∈ f j
m ∏ j=1 γj µ(xj ) , 1 m ∏ j=1 (1 σj )µ(xj ) , 1 m ∏ j=1 (1 ηj )µ(xj )
4. If µ(xj )= 1/m, for j = 1,2, ... , m,thenboththeSVNHFCOGandSVNHFWGoperatorsreduce tothesingle-valuedneutrosophichesitantfuzzygeometric(SVNHFG)operator,whichisshown asfollows:
SVNHFWG{n1, n2,..., nm } = ∪ γj ∈tj , σj ∈ij ,ηj ∈ f j
m ∏ j=1 (γj ) 1 m , 1 m ∏ j=1 (1 σj ) 1 m , 1 m ∏ j=1 (1 ηj ) 1 m .
5. If µ(F)= ∑|F| j=1 ωj forall F ⊆ X, where |F| isthenumberofelementsinF,then ωj = µ(Fφ(j) ) µ(Fφ(j 1) ), j = 1,2, , m, where ω =(ω1, ω2,..., ωm)T suchthat ωj ≥ 0 and ∑m j=1 ωj = 1 Then,theSVNHFCOGoperatorreducestothesingle-valuedneutrosophichesitantfuzzyordered weightedgeometric(SVNHFOWG)operatorasfollows:
SVNHFOWG{n1, n2,..., nm }
= ∪ γj ∈tj , σj ∈izj ,ηj ∈ f j
m ∏ j=1 (γj )ωj , 1 m ∏ j=1 (1 σj )ωj , 1 m ∏ j=1 (1 ηj )ωj
Particularly,if µ(F)= |F|/m,forall F ⊆ X, thentheSVNHFCOGandSVNHFOWGoperators reducetotheSVNHFGoperator.
Theorem5. TheSVNHFCOGoperatorhasthefollowingdesirableproperties:
1. (Idempotency)Let nj = nforallj = 1,2,..., m, and n = {{γ}, {σ}, {η}},then: SVNHFCOGµ {n1, n2,..., nm } = {{γ}, {σ}, {η}}
2. (Boundedness)Let n = {min{γj }, max{σj }, max{ηj }}, n+ = { max{ γj }, min{σj }, min{ηj }}, so: n ≤ SVNHFCOGµ {n1, n2,..., nm }≤ n+
3. (Commutativity)If {n1, n2,..., nm } isapermutationof {n1, n2,..., nm },then, SVNHFCOGµ {n1, n2,..., nm } = SVNHFCOGµ {n1, n2,..., nm }
4. (Monotonity)If nj ≤ nj for∀j ∈{1,2,..., n},then, SVNHFCOGµ {n1, n2,..., nm }≤ SVNHFCOGµ {n1, n2,..., nm }.
Theorem6. Let nj (j = 1,2,..., m) beacollectionofSVNHFEs,Xbethesetofattributesandmbeafuzzy measureonX,thenwehave SVNHFCOGµ {n1, n2,..., nm }≤ SVNHFCOAµ {n1, n2,..., nm }
Proof. BasedonLemma1, ∀ nj = {{γj }, {σj }, {ηj }}, (j = 1,2,..., m),itfollowsthat m ∏ j=1 γj µφ(j) ≤ m ∑ j=1 µφ(j) γj = 1 m ∑ j=1 (µφ(j) )(1 γj ) ≤ 1 m ∏ j=1 (1 γj )(µφ(j) ) Analogously,wehave m ∏ j=1 σj µφ(j) ≤ 1 m ∏ j=1 (1 σj )µφ(j) ; m ∏ j=1 ηj µφ(j) ≤ 1 m ∏ j=1 (1 ηj )µφ(j) Next,bycalculationoftheirscorefunctions,wecanknowthat SVNHFCOGµ {n1, n2,..., nm }≤ SVNHFCOA
4.ApproachesforMADMwithSingle-ValuedNeutrosophicHesitantFuzzyInformation
ForaMADMproblemwithsingle-valuedneutrosophichesitantfuzzyinformation,assumethat thereare n alternatives A = {a1, a2, , an } and m interrelatedattributes X = {x1, x2,..., xm }.Thus, let N =(nij = {tij, iij, fij })n×m denotesvaluesassignedto n alternativeswithrespectto m attributes, indetail, tij, iij, fij indicatethetruth,indeterminacyandfalsitymembershiphesitantfunctionsof ai satisfying xj givenbydecision-makers,respectively.Then,todeterminethemostdesirable alternative(s),theSVNHFCOAandSVNHFCOGoperatorsareutilizedtoestablishMADMmethods withsingle-valuedneutrosophichesitantfuzzyinformation,whichinvolvesthefollowingsteps:
Step1.ReordertheDecisionMatrix
Reorder m SVNHFEs nij fromsmallesttolargestof ai (i = 1,2,..., n) in N =(nij )n×m:(1)if theirscorevalues s(nij ) aredifferent,wecanrankthembyEquation(12);(2)ifsomeoftheirscore valuesaresame,wecontinuetocalculateaccuracyvalues a(nij ) byEquation(13).Then,thereorder sequenceisexpressedas niφ(1), niφ(2), , niφ(m), where (iφ(1), iφ(2),..., iφ(m)) isapermutationof (i1, i2,..., im),suchthat niφ(1) ≥ niφ(2),..., ≥ niφ(m) s(nij )= 1 #γ
#γ ∑ l=1 γij + 1 #σ
#σ ∑ p=1 (1 σij )+ 1 #η
#γ ∑ l=1 γij 1 #η
Step2.ConfirmFuzzyMeasuresof m Attributes
#η ∑ q=1 (1 ηij ).(13)
#η ∑ q=1 (1 ηij ) /3,(12) a(nij )= 1 #γ
SincetheexistingMADMmethodsusingsingle-valuedneutrosophichesitantfuzzyinformation ignoretheinterrelationshipsofattributes,thus,bycombiningtheChoquetintegralwithrespectto fuzzymeasures,morecorrelationscanbeconsideredintheMADMprocess.So,weuse gλ fuzzy measuretodeterminefuzzymeasures µ of X. µiφ(j) = µ(Fiφ(j) ) µ(Fiφ(j 1) ), (i = 1,2,... n, j = 1,2,..., m) (14)
Step3.AggregateAllDecisionInformationbySVNHFCOAorSVNHFCOGOperator
Aggregate m SVNHFEs niφ(j) of ai basedontheSVNHFCOAorSVNHFCOGoperatorin Equation(15)or(16). ni = SVNHFCOAµ {ni1, ni2,..., nim } = ⊕m j=1((µ(Fiφ(j) ) µ(Fiφ(j 1) ))niφ(j) ),(15) ni = SVNHFCOGµ {ni1, ni2,..., nim } = ⊗m j=1(niφ(j) (µ(Fiφ(j) ) µ(Fiφ(j 1) )) ).(16)
Step4.RanktheAlternatives
Calculateandrank n alternativestoselectthemostdesirableone:(1)iftheirscorevalues s(nj ) aredifferent,wecanrank n alternativesbyEquation(17);(2)ifsomeoftheirscorevalues s(nj ) are same,wecontinuetocalculateaccuracyvalues a(nj ) byEquation(18)togetthebestchoice.
AnillustrativeexampleaboutinvestmentalternativesforaMADMproblemadaptedfromYe[24] isutilizedtoillustratetheapplicationsoftheproposedapproachesinthispaper,andtodemonstrate theirfeasibilityandeffectiveness. Supposethatthereisaproblemtodealwithpotentialevaluationofemergingtechnology commercialization,whichisatypicalMADMproblem.Thereisapanelwithfourpossibleemerging
technologyenterprisesdenotedby a1, a2, a3, a4. Duringthedecisionmakingprocess,threeattributes needtobeconsidered:(1) x1 isthepotentialmarketandmarketrisk;(2) x2 istheindustrialization infrastructure,humanresources,andfinancialconditions;(3) x3 istheemploymentcreationand developmentofscienceandtechnology.
Toavoidinfluenceothers,decisionmakersarerequiredtoevaluatethefourpossible emergingtechnologyenterprises ai (i = 1,2,3,4) undertheabovethreeattributesinanonymity, andsingle-valuedneutrosophichesitantfuzzydecisionmatrix N =(nij )4 × 3 isconstructed,shownin Table 1
Table1. Single-valuedNeutrosophicHesitantFuzzyDecisionMatrix N x1 x2 x3
a1 {{0.3,0.4,0.5}, {0.1}, {0.3,0.4}}{{0.5,0.6}, {0.2,0.3}, {0.3,0.4}}{{0.2,0.3}, {0.1,0.2}, {0.5,0.6}} a2 {{0.6,0.7}, {0.1,0.2}, {0.2,0.3}}{{0.6,0.7}, {0.1}, {0.3}}{{0.6,0.7}, {0.1,0.2}, {0.1,0.2}} a3 {{0.5,0.6}, {0.4}, {0.2,0.3}}{{0.6}, {0.3}, {0.4}}{{0.5,0.6}, {0.1}, {0.3}} a4 {{0.7,0.8}, {0.1}, {0.1,0.2}}{{0.6,0.7}, {0.1}, {0.2}}{{0.3,0.5}, {0.2}, {0.1,0.2,0.3}}
Step1. Getthescorematrixof nij calculatedbyEquation(11),showninTable 2,andthereordered decisionmatrixshowninTable 3
Table2. Scorevaluesof nij. x1 x2 x3 a1 0.650.650.52 a2 0.750.750.78 a3 0.630.570.72 a4 0.830.780.67
Table3. Reordereddecisionmatrix N xœ(1) xœ(2) xœ(3)
a1 {{0.2,0.3}, {0.1,0.2}, {0.5,0.6}}{{0.3,0.4,0.5}, {0.1}, {0.3,0.4}}{{0.5,0.6}, {0.2,0.3}, {0.3,0.4}} a2 {{0.6,0.7}, {0.1,0.2}, {0.2,0.3}}{{0.6,0.7}, {0.1}, {0.3}}{{0.6,0.7}, {0.1,0.2}, {0.1,0.2}} a3 {{0.6}, {0.3}, {0.4}}{{0.5,0.6}, {0.4}, {0.2,0.3}}{{0.5,0.6}, {0.1}, {0.3}} a4 {{0.3,0.5}, {0.2}, {0.1,0.2,0.3}}{{0.6,0.7}, {0.1}, {0.2}}{{0.7,0.8}, {0.1}, {0.1,0.2}}
Since s(n11)= s(n12)= 0.65, s(n21)= s(n22)= 0.75, wecalculateaccuracyvaluesand get a(n11)= 0.25, a(n12)= 0.1; a(n21)= 0.1, a(n22)= 0.05. Thus,reordersequences for a1, a2, a3, a4 areasfollows: n1σ(1) = n13, n1σ(2) = n11,n1σ(3) = n12; n2σ(1) = n21,n2σ(2) = n22, n2σ(3) = n23; n3σ(1) = n32, n3σ(2) = n31,n3σ(3) = n33; n4σ(1) = n43,n4σ(2) = n42, n4σ(3) = n41.
Step2. Supposethatthefuzzymeasuresofattributesof X aregivenasfollows: µ(x1)= 0.362, µ(x2)= 0.2, µ(x3)= 0.438.Firstly,accordingtoEquation(7),thevalueof λ is obtained: λ = 0.856.Thus, µ(x1, x2)= 0.626, µ(x2, x3)= 0.713, µ(x1, x3)= 0.936, µ(X)= 1,thus, µ(xφ(1) )= 0.362; µ(xφ(2) )= 0.264; µ(xφ(3) )= µ(X) µ(x1, x2)= 0.374.
Step3. Aggregate nij (i = 1,2,3,4; j = 1,2,3) byusingtheSVNHFCOAoperatortoderivethe comprehensivescorevalue ni for ai (i = 1,2,3,4). Take a1 foranexample,thecomprehensivescore value n1 of a1 iscalculatedasfollows:
n1 = {{1 (1 0.2)0.362(1 0.3)0.264(1 0.5)0.374,1 (1 0.2)0.362(1 0.3)0.264(1 0.6)0.374 ,
1 (1 0.2)0.362(1 0.4)0.264(1 0.5)0.374,1 (1 0.2)0.362(1 0.4)0.264(1 0.6)0.374 ,
1 (1 0.2)0.362(1 0.5)0.264(1 0.5)0.374,1 (1 0.2)0.362(1 0.5)0.264(1 0.6)0.374 ,
1 (1 0.3)0.362(1 0.3)0.264(1 0.5)0.374,1 (1 0.3)0.362(1 0.3)0.264(1 0.6)0.374 ,
1 (1 0.3)0.362(1 0.4)0.264(1 0.5)0.374,1 (1 0.3)0.362(1 0.4)0.264(1 0.6)0.374 ,
1 (1 0.3)0.362(1 0.5)0.264(1 0.5)0.374,1 (1 0.3)0.362(1 0.5)0.264(1 0.6)0.374 {0.10.3620.10.2640.20.374,0.10.3620.10.2640.30.374,0.20.3620.10.2640.20.374,0.20.3620.10.2640.30.374}, {0.50.3620.30.2640.30.374,0.50.3620.30.2640.40.374,0.50.3620.40.2640.30.374,0.50.3620.40.2640.40.374 , 0.60.3620.30.2640.30.374,0.60.3620.30.2640.40.374,0.60.3620.40.2640.30.374,0.60.3620.40.2640.40.374}},
andobtainthefollowingcollectiveSVNHFEs:
n1 = {{0.352,0.401,0.386,0.432,0.424,0.468,0.378,0.425,0.41,0.455,0.447,0.488}, {0.127,0.147,0.157,0.181}, {0.35,0.387,0.387,0.428,0.369,0.408,0.408,0.452}}.
n2 = {{0.6,0.629,0.64,0.641,0.666,0.667,0.676,0.7}, {0.1,0.129,0.13,0.167}, {0.172,0.199,0.223,0.258}}; n3 = {{0.538,0.568,0.572,0.6}, {0.223}, {0.294,0.332}}; n4 = {{0.56,0.566,0.592,0.598,0.611,0.622,0.639,0.65}, {0.129}, {0.12,0.154,0.156,0.179,0.2,0.232}}
Step4. BasedonthescorefunctionofSVNHFEs,weget:
s(n1)= 0.599, s(n2)= 0.77, s(n3)= 0.678, s(n4)= 0.767.
Therefore,wecanseethat a2 > a4 > a3 > a1 and a2 isthebestchoice. IfweutilizetheSVNHFCOGoperatorfortheMADMproblem,thedecision-makingprocedure canbedescribedasfollows:
Step3 Aggregate nij (i = 1,2,3,4; j = 1,2,3) byusingtheSVNHFCOGoperatortoderivethe comprehensivescorevalue ni for ai (i = 1,2,3,4)
n1 = {{0.20.3620.30.2640.50.374,0.20.360.30.2640.60.374,0.20.360.40.2640.50.374,0.20.360.40.2640.60.374 , 0.20.3620.50.2640.50.374,0.20.360.50.2640.60.374,0.30.360.30.2640.50.374,0.30.360.30.2640.60.374 , 0.30.3620.40.2640.50.374,0.30.360.40.2640.60.374,0.30.360.50.2640.50.374,0.30.360.50.2640.60.374
1 (1 0.1)0.362(1 0.1)0.264(1 0.2)0.374,1 (1 0.1)0.362(1 0.1)0.264(1 0.3)0.374 , 1 (1 0.2)0.362(1 0.1)0.264(1 0.2)0.374,1 (1 0.2)0.362(1 0.1)0.264(1 0.3)0.374}, {1 (1 0.6)0.362(1 0.3)0.264(1 0.3)0.374,1 (1 0.6)0.362(1 0.3)0.264(1 0.4)0.374 ,
1 (1 0.6)0.362(1 0.4)0.264(1 0.3)0.374,1 (1 0.6)0.362(1 0.4)0.264(1 0.4)0.374 ,
1 (1 0.5)0.362(1 0.3)0.264(1 0.3)0.374,1 (1 0.5)0.362(1 0.3)0.264(1 0.4)0.374 ,
1 (1 0.5)0.362(1 0.4)0.264(1 0.3)0.374,1 (1 0.5)0.362(1 0.4)0.264(1 0.4)0.374}},
andobtainthefollowingcollectiveSVNHF n1 :
n1 = {{0.318,0.339,0.351,0.374,0.38,0.405,0.359,0.382,0.397,0.423,0.429,0.457}, {0.136,0.176,0.166,0.204}, {0.408,0.439,0.439,0.469,0.367,0.4,0.4,0.432}}
n2 = {{0.6,0.625,0.634,0.636,0.661,0.662,0.672,0.7}, {0.1,0.138,0.139,0.175}, {0.193,0.228,0.231,0.264}};
n3 = {{0.533,0.568,0.563,0.6}, {0.27}, {0.31,0.337}};
n4 = {{0.495,0.515,0.52,0.541,0.595,0.62,0.625,0.651}, {0.138}, {0.128,0.164,0.165,0.2,0.203,0.238}}
Step4 . BasedonthescorefunctionofSVNHFEs,weget:
s(n1)= 0.599,s(n2)= 0.761,s(n3)= 0.658,s(n4)= 0.75.
Rank ai accordingtothescorevalues a2 > a4 > a3 > a1.Therefore,wecanseethat a2 isthe bestchoice.
Obviously,theabovetwokindsofrankingordersarethesameastheonesinRef[24].Thus, thetwokindsofrankingorderscoincidewiththeresultsthatareobtainedinotherreferences;therefore, theaboveexampleclearlyindicatesthattheproposeddecision-makingmethodsareapplicableand effectiveunderasingle-valuedneutrosophichesitantfuzzyenvironment.
5.2.ComparisonAnalysisandDiscussion
TofurthervalidatethefeasibilityofaboveMADMmethods,acomparisonanalysiswasconducted withothermethods,itshouldbenotedthatalltheseapproachesarenotclarifyhowtosolveasituation wheretheattributesareinter-related.Tobespecific,thecomparativestudywasbasedonthesame illustrativeexampleinwhichtheweightofattributesis ω =(0.35,0.25,0.4).Then,theresultsby utilizingdifferentapproacheswithcompleteweightinformationareshowninTable 4.
Table4. Resultsobtainedbyutilizingthedifferentmethodsbasedonthesameillustrativeexample.
MethodsFinalRankingBestAlternativeWorstAlternative
SVNHFWAoperator[24] a4 > a2 > a3 > a1 a4 a1
SVNHFWGoperator[24] a2 > a4 > a3 > a1 a2 a1 Correlationcoefficient[25] a2 > a4 > a3 > a1 a2 a1
Hammingdistance[26] a2 > a4 > a3 > a1 a2 a1
SVNHFCOAoperator a2 > a4 > a3 > a1 a2 a1 SVNHFCOGoperator a2 > a4 > a3 > a1 a2 a1
Forthecomparedmethodsin[24],Yeproposestwokindsofaggregationoperators,theSVNHFWA andSVNHFWGoperators,whichareappliedtoMADMproblemswithsingle-valuedneutrosophic hesitantfuzzyinformationin[25].
Forthecomparedmethodsin[25],SahinandLiumotivatedbytheideaofcorrelationcoefficients derivedforHFSs,IFSs,SVNSs,theyputforwardcorrelationcoefficientsofSVNHFSs.Then,an effectiveMADMexampleisusedtodemonstrateitsvalidity.
Forthecomparedmethodsin[26],¸SahinRutilizethedistancemeasurebetweeneachalternative andidealalternativetoestablishamultipleattributedecisionmakingmethodundersingle-valued neutrosophichesitantfuzzyenvironment.
Thus,accordingtotheresultspresentedinTable 4,iftheSVNHFCOGoperator[24]isused, thedesirablealternativeis a4,andifotherfivekindsofmethodsareutilized,thebestchoiceis a2,andforallthecomparedmethods,theworstalternativeisalways a1.Therefore,forthesame single-valuedneutrosophichesitantfuzzyinformation,theresultsobtainedbytheproposedmethods inthispaperareconsistentwiththoseobtainedusingthecomparedmethodsin[24–26],whichfurther demonstratestheeffectivenessandfeasibilityoftheSVNHFCOAandSVNHFCOGoperators.
6.Conclusions
Inthispaper,weputforwardtwonovelMADMmethodsbasedontheSVNHFCOAand SVNHFCOGoperators,theiradvantagescanbesummarizedbelow.
First,theSVNHFCOAandSVNHFCOGoperatorshavedesirableproperties,like:idempotency, boundedness,commutativity,andmonotonity,andtheycanreducetotheexistingaggregation operatorsofSVNHFSs,whichillustratetheirvalidityintheory.
Second,whencomparingwithexistingmethodsforMADMproblemsunderneutrosophichesitant fuzzyenvironment,resultsobtainedbytheSVNHFCOAandSVNHFCOGoperatorsareconsistent andaccurate,whichillustratestheirpracticabilityinapplication.
Third,theexistingapproachescannotconsidertheinterrelationshipsofattributesinpractical application;theproposedmethodsforMADMinthispapercanfurtherconsidermorecorrelations betweenattributes,whichmeansthattheyhavehigheraccuracyandgreaterreferencevalue.
Finally,liketheChoquetaggregationoperatorsappliedandstudiedunderotherfuzzy environments,likehesitantfuzzyenvironments,intuitionisticfuzzyenvironment,linguisticfuzzy environment,andothers,theresearchofthispapercanlaythefoundationforthefollowingresearch, whichisofprofoundsignificance.
Acknowledgments: ThisworkwassupportedbytheNationalNaturalScienceFoundationofChina(GrantNos. 61573240,61473239).
AuthorContributions: Allauthorshavecontributedequallytothispaper.Theideaofthewholethesisisput forwardbyXiaohongZhang,healsocompletedthepreparatoryworkofthepaper.XinLianalyzedtheexisting workofsingle-valuedneutrosophichesitantfuzzysetsandwrotethepaper.Therevisionandsubmissionofthe paperwerecompletedbyXiaohongZhangandXinLi.
ConflictsofInterest: Theauthorsdeclarenoconflictofinterest.
References
1. Rao,R.V. DecisionMakingintheManufacturingEnvironmentUsingGraphTheoryandFuzzyMADMMethods; Springer:London,UK,2007;Availableonline: https://max.book118.com/html/2017/0729/124983015.shtm (accessedon29July2017).
2. Zadeh,L.A.Fuzzysets. J.Inform.Control 1965, 8,338–353.[CrossRef]
3. Zhang,X.H.Fuzzyanti-groupedfiltersandfuzzynormalfiltersinpseudo-BCIalgebras. J.Intell.FuzzySyst. 2017, 33,1767–1774.[CrossRef]
4. Miyamoto,S.Remarksonbasicsoffuzzysetsandfuzzymultisets. FuzzySetsSyst. 2005, 156,427–431. [CrossRef]
5. Atanassov,K.;Gargov,G.Interval-valuedintuitionisticfuzzysets. FuzzySetsSyst. 1989, 31,343–349. [CrossRef]
6. Dubois,D.;Prade,H. FuzzySetsandSystems:TheoryandApplications;AcademicPress:NewYork,NY,USA, 1980;pp.32–154.
7. Smarandache,F.Aunifyingfieldinlogics:Neutrosophiclogic. Multi.ValuedLogic 1999, 8,489–503.
8. Ju,Y.B.;Yang,S.H.;Liu,X.Y.SomenewdualhesitantfuzzyaggregationoperatorsbasedonChoquetintegral andtheirapplicationstomultipleattributedecisionmaking. J.Intell.FuzzySyst. 2014, 27,2857–2868. [CrossRef]
9. Ju,Y.B.Anewmethodformultiplecriteriagroupdecisionmakingwithincompleteweightinformation underlinguisticenvironment. Appl.Math.Model 2014, 38,5256–5268.[CrossRef]
10. Wang,H.;Smarandache,F.;Sunderraman,R.Single-valuedneutrosophicsets. Rev.AirForceAcad. 2013, 17, 10–13.
11. Wang,H.;Madiraju,P.Interval-neutrosophicSets. J.Mech. 2004, 1,274–277.[CrossRef]
12. Peng,J.;Wang,J.;Wu,X.Multi-valuedneutrosophicsetsandpoweraggregationoperatorswiththeir applicationsinmulti-criteriagroupdecision-makingproblems. Int.J.Comput.Int.Sys. 2015, 8,345–363. [CrossRef]
13. Broumi,S.;Smarandache,F.;Dhar,M.Roughneutrosophicsets. Ital.J.Pure.Appl.Math. 2014, 32.[CrossRef]
14. Zhang,X.H.;Bo,C.X.;Smarandache,F.;Dai,J.H.Newinclusionrelationofneutrosophicsetswith applicationsandrelatedlatticestructrue. Int.J.Mach.Learn.Cybs. 2017.accepted.
15. Ye,J.Amulticriteriadecision-makingmethodusingaggregationoperatorsforsimplifiedneutrosophicsets. J.Intell.FuzzySyst. 2014, 26,2459–2466.[CrossRef]
16.
Ye,J.Multicriteriadecision-makingmethodusingthecorrelationcoefficientundersingle-valued neutrosophicenvironment. Int.J.Gen.Syst. 2013, 42,386–394.[CrossRef]
17. Huang,L.H.Newdistancemeasureofsingle-valuedneutrosophicsetsanditsapplication. J.Intell.Syst. 2016, 31,1021–1032.[CrossRef]
18. Torra,V.;Narukawa,Y.Onhesitantfuzzysetsanddecision.InProceedingsofthe18thIEEEInternational ConferenceonFuzzySystems,JejuIsland,Korea,20–24August2009;pp.1378–1382.[CrossRef]
19. Torra,V.Hesitantfuzzysets. Int.J.Intell.Syst. 2010, 25,529–539.[CrossRef]
20. Qu,G.;Li,Y.;Qu,W.SomenewShapleydualhesitantfuzzyChoquetaggregationoperatorsandtheir applicationstomultipleattributegroupdecisionmaking-basedTOPSIS. J.Intell.FuzzySyst. 2017, 33,1–21. [CrossRef]
21. Xu,Z.S.;Zhang,X.L.Hesitantfuzzymulti-attributedecisionmakingbasedonTOPSISwithincomplete weightinformation. IFIP.Trans.B. 2013, 52,53–64.[CrossRef]
22. Xia,M.M.;Xu,Z.S.Hesitantfuzzyinformationaggregationindecisionmaking. Int.J.Approx.Reason. 2011, 52,395–407.[CrossRef]
23. Zhang,N.;Wei,G.W.ExtensionofVIKORmethodfordecisionmakingproblembasedonhesitantfuzzyset. Appl.Math.Model. 2013, 37,4938–4947.[CrossRef]
24. Ye,J.Multiple-attributedecision-makingmethodunderasingle-valuedneutrosophichesitantfuzzy environment. J.Intell.Syst. 2014, 24,23–36.[CrossRef]
25. ¸Sahin,R.;Liu,P.D.Correlationcoefficientofsingle-valuedneutrosophichesitantfuzzysetsandits applicationsindecisionmaking. Neural.Comput.Appl. 2017, 28,1387–1395.[CrossRef]
26. ¸Sahin,R.Distanceandsimilaritymeasuresfor MADM withsingle-valuedneutrosophichesitantfuzzy information.In NewtrendsinNeutrosophicTheoryandApplications;Florentin,S.,Surapati,P.,Eds.; PonsEditionsBrussels:Brussels,Belgium,2016;ISBN978-1-59973-498-9.Availableonline: https: //www.researchgate.net/publication/310646060 (accessedon20November2016).
27. Liu,P.D.;Zhang,L.L.Anextendedmulti-criteriadecisionmakingmethodbasedonneutrosophichesitant fuzzyinformation. J.Intell.FuzzySyst. 2017, 32,4403–4413.[CrossRef]
28. Biswas,P.;Pramanik,S.;Giri,B.C.Somedistancemeasuresofsingle-valuedneutrosophichesitant fuzzysetsandtheirapplicationstomultipleattributedecisionmaking.In NewtrendsinNeutrosophic TheoryandApplications;Florentin,S.,Surapati,P.,Eds.;PonsEditionsBrussels:Brussels,Belgium,2016; ISBN978-1-59973-498-9.Availableonline: https://www.researchgate.net/publication/310646060 (accessed on20November2016).
29. Liu,C.F.;Luo,Y.S.Newaggregationoperatorsofsingle-valuedneutrosophichesitantfuzzysetandtheir applicationin MADM PatternAnal.Appls. 2017, 1,1–11.[CrossRef] 30. Choquet,G.Theoryofcapacities. Annalesdel.InstitutFourier. 1953, 5,131–295.[CrossRef] 31. Sugeno,M.Theoryoffuzzyintegralanditsapplication.Ph.D.Thesis,TokyoInstituteofTechnology,Tokyo, Japan,22January1975. 32. Grabisch,M.;Nguyen,H.T.;Walker,E.A.Fuzzymeasuresandintegrals.Infundamentalsofuncertainty calculiwithapplicationstofuzzyinference. SpringerNeth. 1995, 78,563–604.[CrossRef] 33. Zhang,X.H.;She,Y.H. FuzzyQuantifieswithIntegralSemantics;Sciencepress:Beijing,China,2017;ISBN 978-7-03053-480-4.Availableonline: http://product.dangdang.com/25113577.html (accessedon1July2017). 34. Qin,J.;Liu,X.;Pedrycz,W.Multi-attributegroupdecisionmakingbasedonChoquetintegralunder interval-valuedintuitionisticfuzzyenvironment. Int.J.Comput.Int.Sys. 2016, 9,133–152.[CrossRef]
© 2018bytheauthors.LicenseeMDPI,Basel,Switzerland.Thisarticleisanopenaccess articledistributedunderthetermsandconditionsoftheCreativeCommonsAttribution (CCBY)license(http://creativecommons.org/licenses/by/4.0/).
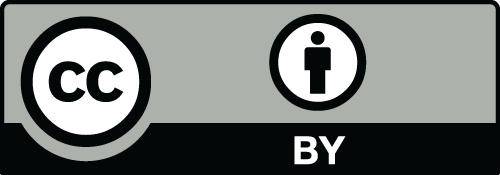