SomeNewBiparametricDistanceMeasureson Single-ValuedNeutrosophicSetswithApplications toPatternRecognitionandMedicalDiagnosis
HarishGarg* ID andNancy ID
SchoolofMathematics,ThaparInstituteofEngineering&Technology,DeemedUniversityPatiala, Punjab147004,India;nancyverma16@gmail.com
* Correspondence:harishg58iitr@gmail.comorharish.garg@thapar.edu;Tel.:+91-86990-31147
Received:29November2017;Accepted:11December2017;Published:15December2017
Abstract: Single-valuedneutrosophicsets(SVNSs)handlingtheuncertaintiescharacterizedbytruth, indeterminacy,andfalsitymembershipdegrees,areamoreflexiblewaytocaptureuncertainty.Inthis paper,somenewtypesofdistancemeasures,overcomingtheshortcomingsoftheexistingmeasures, forSVNSswithtwoparametersareproposedalongwiththeirproofs.Thevariousdesirablerelations betweentheproposedmeasureshavealsobeenderived.Acomparisonbetweentheproposedand theexistingmeasureshasbeenperformedintermsofcounter-intuitivecasesforshowingitsvalidity. Theproposedmeasureshavebeenillustratedwithcasestudiesofpatternrecognitionaswellas medicaldiagnoses,alongwiththeeffectofthedifferentparametersontheorderingoftheobjects.
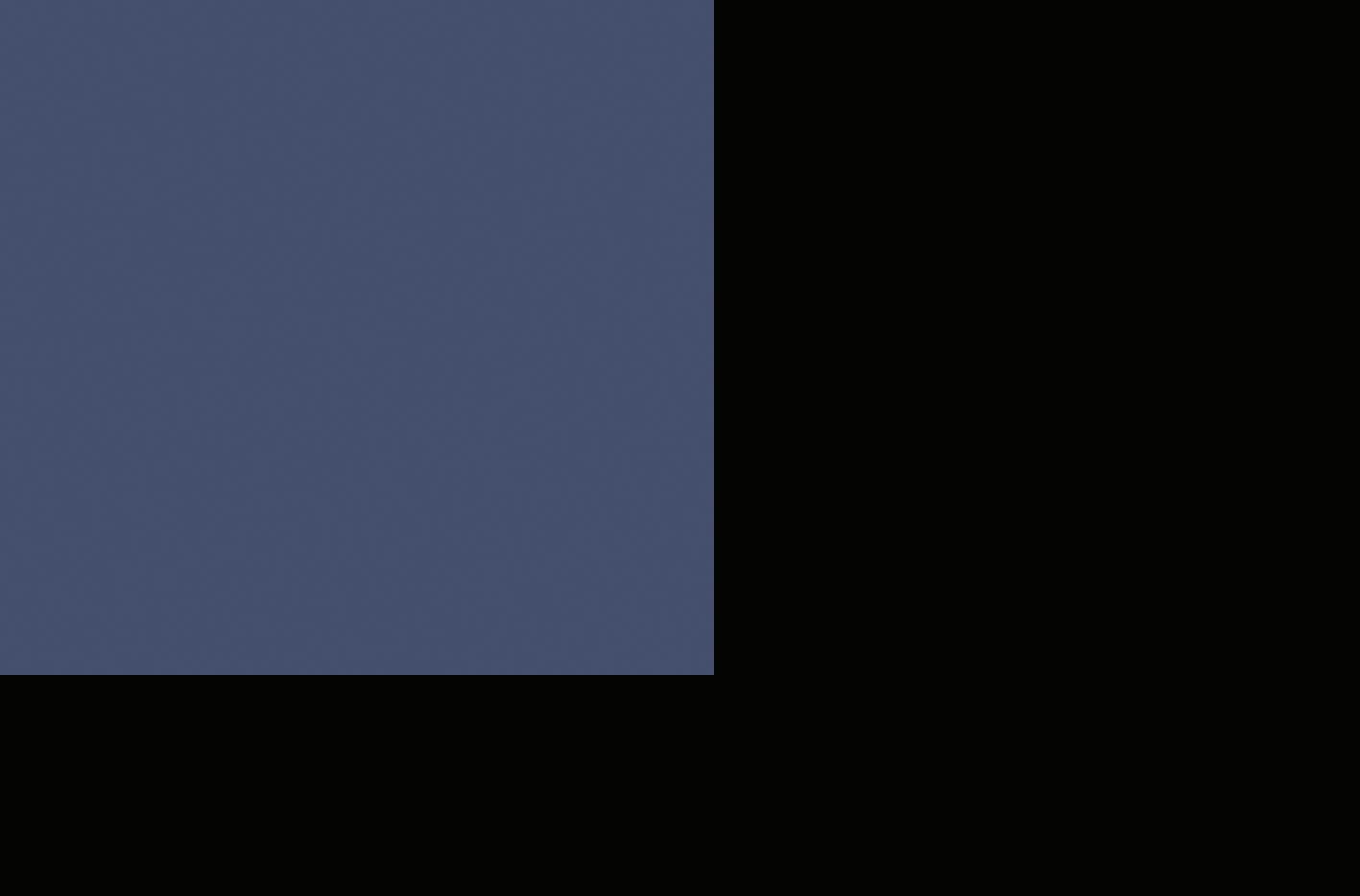
Keywords: decision-making;single-valuedneutrosophicsets;distancemeasure;patternrecognition; uncertainties
1.Introduction
Theclassicalmeasuretheoryhasbeenwidelyusedtorepresentuncertaintiesindata. However,thesemeasuresarevalidonlyforprecisedata,andhencetheymaybeunabletogive accuratejudgments fordatauncertainandimpreciseinnature.Tohandlethis,fuzzyset(FS)theory, developedby Zadeh [1],hasreceivedmuchattentionoverthelastdecadesbecauseofitscapabilityof handlinguncertainties.Afterthis,Atanassov [2] proposedtheconceptofanintuitionisticfuzzyset (IFS),whichextendsthetheoryofFSswiththeadditionofadegreeofnon-membership.AsIFStheory haswidelybeenusedbyresearchers[3–16]indifferentdisciplinesforhandlingtheuncertaintiesindata, henceitscorrespondinganalysisismoremeaningfulthanFSs’crispanalysis.Nevertheless,neitherthe FSnorIFStheoryareabletodealwithindeterminateandinconsistentinformation.Forinstance,we takeapersongivingtheiropinionaboutanobjectwith0.5beingthepossibilitythatthestatementis true,0.7 beingthepossibilitythatthestatementisfalseand0.2beingthepossibilitythatheorsheisnotsure. Toresolvethis,Smarandache [17] introducedanewcomponentcalledthe“indeterminacy-membership function”andaddedthe“truthmembershipfunction”and“falsitymembershipfunction”,allwhichare independentcomponentslyingin ]0 ,1+ [,andhencethecorrespondingsetisknownasaneutrosophic set(NS),whichisthegeneralizationoftheIFSandFS.However,withoutspecification,NSsaredifficult toapplytoreal-lifeproblems.Thus,aparticularcaseoftheNScalledasingle-valuedNS(SVNS)has beenproposedbySmarandache[17],Wangetal.[18].
Afterthispioneeringwork,researchershavebeenengagedinextensionsandapplications todifferentdisciplines.However,themostimportanttaskforthedecision-makeristorankthe objectssoastoobtainthedesiredobject(s).Forthis,researchershavemadeeffortstoenrichthe conceptofinformationmeasuresinneutrosophicenvironments.BroumiandSmarandache [19] introducedtheHausdorffdistance,whileMajumdar [20] presentedtheHammingandEuclidean
Information 2017, 8,162;doi:10.3390/info8040162 www.mdpi.com/journal/information
distanceforcomparingtheSVNSs.Ye [21] presentedtheconceptofcorrelationforsingle-valued neutrosophicnumbers(SVNNs).Additionally,Ye [22] improvedtheconceptofcosinesimilarityfor SVNSs,whichwasfirstlyintroducedbyKongetal. [23] inaneutrosophicenvironment.Nancyand Garg [24] presentedanimprovedscorefunctionforrankingtheSVNNsandappliedthemtosolvethe decision-makingproblem.GargandNancy [25] presentedtheentropymeasureoforder α andapplied themtosolvedecision-makingproblems.Recently,GargandNancy [26] presentedatechniquefor orderpreferencebysimilaritytoidealsolution(TOPSIS)methodunderanintervalNSenvironment tosolvedecision-makingproblems.Asidefromthese,variousauthorshaveincorporatedtheidea ofNStheoryintothesimilaritymeasures[27,28],distancemeasures[29,30],thecosinesimilarity measure[19,22,31],andaggregationoperators[22,31–40].
Thus,onthebasisoftheaboveobservations,ithasbeenobservedthatdistanceorsimilarity measuresareofkeyimportanceinanumberoftheoreticalandappliedstatisticalinferenceand dataprocessingproblems.Ithasbeendeducedfromstudiesthatsimilarity,entropyanddivergence measurescouldbeinducedbythenormalizeddistancemeasureonthebasisoftheiraxiomatic definitions.Ontheotherhand,SVNSsareoneofthemostsuccessfultheoriestohandletheuncertainties andcertaintiesinthesystem,butlittlesystematicresearchhasexploredtheseproblems.Thegapin theresearchmotivatesustodevelopsomefamiliesofthedistancemeasuresoftheSVNStosolve thedecision-makingproblem,forwhichpreferencesrelatedtodifferentalternativesaretakeninthe formofneutrosophicnumbers.Themaincontributionsofthisworkaresummarizedasfollows: (i)tohighlighttheshortcomingsofthevariousexistingdistancemeasuresunderthesingle-valued neutrosophicinformationthroughillustrativeexamples;(ii)toovercometheshortcomingsofthe existingmeasures,thispaperdefinessomenewseriesofbiparametricdistancemeasuresbetween SVNSs,whichdependontwoparameters,namely, p and t,where p isthe Lp normand t identifies thelevelofuncertainty.Thevariousdesirablerelationsbetweenthesehavebeeninvestigatedin detail.Then,weutilizedthesemeasurestosolvetheproblemofpatternrecognitionaswellasmedical diagnosisandcomparedtheirperformancewiththatofsomeoftheexistingapproaches.
Therestofthispaperisorganizedasfollows.Section 2 brieflydescribestheconceptsof NSs,SVNSsandtheircorrespondingexistingdistancemeasures.Section 3 presentsafamilyof thenormalizedandweightednormalizeddistancemeasuresbetweentwoSVNSs.Someoftheir desirablepropertieshavealsobeeninvestigatedindetail,whilegeneralizeddistancemeasureshave beenproposedinSection 4.Thedefinedmeasuresareillustrated,byanexampleinSection 5,usingthe fieldofpatternrecognitionandmedicaldiagnosisfordemonstratingtheeffectivenessandstabilityof theproposedmeasures.Finally,aconcreteconclusionhasbeendrawninSection 6
2.Preliminaries
AnoverviewofNSsandSVNSsisaddressedhereontheuniversalset X
2.1.BasicDefinitions
Definition1 ([17,41]). Aneutrosophicset(NS) A in X isdefinedbyitstruthmembershipfunction (TA (x)), anindeterminacy-membershipfunction (IA (x)) andafalsitymembershipfunction (FA (x)),whereallare subsetsof ]0 ,1+ [.Thereisnorestrictiononthesumof TA (x), IA (x) and FA (x);thus 0 ≤ sup TA (x)+ sup IA (x)+ sup FA (x) ≤ 3+ forallx ∈ X.Here, sup representsthesupremumoftheset.
Wangetal.[18],Smarandache[41]definedtheSVNS,whichisaninstanceofaNS.
Definition2 ([18,41]) Asingle-valuedneutrosophicset(SVNS)Aisdefinedas A = { x, TA (x), IA (x), FA (x) | x ∈ X}
where TA : X → [0,1], IA : X → [0,1] and FA : X → [0,1] with TA (x)+ IA (x)+ FA (x) ≤ 3 forall x ∈ X. Thevalues TA (x), IA (x) and FA (x) denotethetruth-membershipdegree,theindeterminacy-membershipdegree andthefalsity-membershipdegreeof x to A,respectively.Thepairsofthesearecalledsingle-valuedneutrosophic numbers(SVNNs),whicharedenotedby α = µA, ρA, νA ,andclassofSVNSsisdenotedby Φ(X).
Definition3. Let A = µA, ρA, νA and B = µB, ρB, νB betwosingle-valuedneutrosophicsets(SVNSs). Thenthefollowingexpressionsaredefinedby[18]:
(i) A ⊆ Bifandonlyif(iff) µA (x) ≤ µB (x), ρA (x) ≥ ρB (x) and νA (x) ≥ νB (x) forallxinX; (ii) A = BiffA ⊆ BandB ⊆ A; (iii) Ac = { νA (x),1 ρA (x), µA (x) | x ∈ X }; (iv) A ∩ B = min(µA (x), µB (x)),max(ρA (x), ρB (x)),max(νA (x), νB (x)) ; (v) A ∪ B = max(µA (x), µB (x)),min(ρA (x), ρB (x)),min(νA (x), νB (x))
2.2.ExistingDistanceMeasures
Definition4. Arealfunction d : Φ(X) × Φ(X) → [0,1] iscalledadistancemeasure[19],where d satisfies thefollowingaxiomsforA, B, C ∈ Φ(X):
(P1) 0 ≤ d(A, B) ≤ 1; (P2) d(A, B)= 0 iffA = B; (P3) d(A, B)= d(B, A);
(P4) IfA ⊆ B ⊆ C,thend(A, C) ≥ d(A, B) andd(A, C) ≥ d(B, C)
Onthebasisofthis,severalresearchershaveaddressedthevarioustypesofdistance andsimilaritymeasuresbetweentwoSVNSs A = xi, µA (xi ), ρA (xi ), νA (xi )|xi ∈ X and B = xi, µB (xi ), ρB (xi ), νB (xi )|xi ∈ X , i = 1,2,..., n,whicharegivenasfollows:
(i) TheextendedHausdorffdistance[19]:
DH (A, B)= 1 n
n ∑ i=1 max |µA (xi ) µB (xi )|, |ρA (xi ) ρB (xi )|, |νA (xi ) νB (xi )| (1)
(ii) ThenormalizedHammingdistance[20]:
DNH (A, B)= 1 3n
n ∑ i=1 |µA (xi ) µB (xi )| + |ρA (xi ) ρB (xi )| + |νA (xi ) νB (xi )| (2)
(iii) ThenormalizedEuclideandistance[20]:
DNE (A, B)= 1 3n
n ∑ i=1 (µA (xi ) µB (xi ))2 +(ρA (xi ) ρB (xi ))2 +(νA (xi ) νB (xi ))2 1/2 (3)
(iv) Thecosinesimilarities[22]:
SCS1(A, B)= 1 n
n ∑ i=1 cos π |µA (xi ) µB (xi )|∨|ρA (xi ) ρB (xi )|∨|νA (xi ) νB (xi )| 2 (4) and SCS2(A, B)= 1 n
n ∑ i=1 cos π |µA (xi ) µB (xi )| + |ρA (xi ) ρB (xi )| + |νA (xi ) νB (xi )| 6 (5) andtheircorrespondingdistancesdenotedby DCS1 = 1 SCS1 and DCS2 = 1 SCS2
(v) Thetangentsimilarities[42]:
ST1(A, B)= 1 1 n
n ∑ i=1 tan π |µA (xi ) µB (xi )|∨|ρA (xi ) ρB (xi )|∨|νA (xi ) νB (xi )| 4 (6) and ST2(A, B)= 1 1 n
n ∑ i=1 tan π |µA (xi ) µB (xi )| + |ρA (xi ) ρB (xi )| + |νA (xi ) νB (xi )| 12 (7) andtheircorrespondingdistancesdenotedby DT1 = 1 ST1 and DT2 = 1 ST2
2.3.ShortcomingsoftheExistingMeasures
Theabovemeasureshavebeenwidelyused;however,simultaneouslytheyhavesomedrawbacks, whichareillustratedwiththenumericalexamplethatfollows.
Example1. Considertwoknownpatterns A and B,whicharerepresentedbySVNSsinauniverse X givenby A = x,0.5,0.0,0.0 | x ∈ X , B = x,0.0,0.5,0.0 | x ∈ X .Consideranunknownpattern C ∈ SVNSs(X), whichisrecognizedwhere C = x,0.0,0.0,0.5 | x ∈ X ;thenthetargetofthisproblemistoclassifythepattern C inoneoftheclasses A or B.Ifweapplytheexistingmeasures[19,20,22,42]definedinEquations (1)–(7) above,thenweobtainthefollowing:
PairDH DNH DNE DCS1 DCS2 DT1 DT1 (A,C)0.50.33330.40480.29290.13400.41420.2679 (B,C)0.50.33330.40480.29290.13400.41420.2679
Thus,fromthis,weconcludethattheseexistingmeasuresareunabletoclassifythepatternCwithAand B.Hencethesemeasuresareinconsistentandunabletoperformranking.
Example2. ConsidertwoSVNSsdefinedontheuniversalset X givenby A = x,0.3,0.2,0.3 | x ∈ X and B = x,0.4,0.2,0.4 | x ∈ X .Ifwereplacethedegreeoffalsitymembershipof A (0.3)with0.4,andthatof B (0.4)with0.3,thenweobtainnewSVNSsas C = x,0.3,0.2,0.4 | x ∈ X and D = x,0.4,0.2,0.3 | x ∈ X Now,byusingthedistancemeasuresdefinedinEquations (1)–(7),weobtaintheircorrespondingvalues asfollows:
PairDH DNH DNE DCS1 DCS2 DT1 DT1 (A,B)0.10.0660.0770.0130.0060.0780.052 (C,D)0.10.0660.0770.0130.0060.0780.052
Thus,ithasbeenconcludedthatbychangingthefalsitydegreeofSVNSsandkeepingtheother degreesunchanged,thevaluesoftheircorrespondingmeasuresremainthesame.Thus,thereisno effectofthedegreeoffalsitymembershiponthedistancemeasures.Similarly,wecanobservethe sameforthedegreeofthetruthmembershipfunctions.
Thisseemstobeworthlesstocalculatedistanceusingthemeasuresmentionedabove.Thus,there isaneedtobuildupanewdistancemeasurethatovercomestheshortcomingsoftheexistingmeasures.
3.SomeNewDistanceMeasuresbetweenSVNSs
Inthissection,wepresenttheHammingandtheEuclideandistancesbetweenSVNSs,whichcan beusedinrealscientificandengineeringapplications.
Letting Φ(X) betheclassofSVNSsovertheuniversalset X,thenwedefinethedistancesfor SVNSs, A = µA (xi ), ρA (xi ), νA (xi ) | xi ∈ X and B = µB (xi ), ρB (xi ), νB (xi ) | xi ∈ X ,byconsidering theuncertaintyparameter t,asfollows:
(i) Hammingdistance:
d1(A, B)= 1 3(2 + t) n ∑ i=1
t(µA (xi ) µB (xi ))+(ρA (xi ) ρB (xi ))+(νA (xi ) νB (xi )) + t(ρA (xi ) ρB (xi )) (νA (xi ) νB (xi ))+(µA (xi ) µB (xi )) + t(νA (xi ) νB (xi )) (ρA (xi ) ρB (xi ))+(µA (xi ) µB (xi ))
(ii) NormalizedHammingdistance:
d2(A, B)= 1 3n(2 + t) n ∑ i=1
(iii)
t(µA (xi ) µB (xi ))+(ρA (xi ) ρB (xi ))+(νA (xi ) νB (xi )) + t(ρA (xi ) ρB (xi )) (νA (xi ) νB (xi ))+(µA (xi ) µB (xi )) + t(νA (xi ) νB (xi )) (ρA (xi ) ρB (xi ))+(µA (xi ) µB (xi ))
(8)
(iv) NormalizedEuclideandistance:
(11) where t ≥ 3isaparameter. Then,onthebasisofthedistancepropertiesasdefinedinDefinition 4,wecanobtainthe followingproperties:
Proposition1. Theabove-defineddistance d2(A, B),betweentwoSVNSs A and B,satisfiesthefollowing properties(P1)–(P4): (P1) 0 ≤ d2(A, B) ≤ 1, ∀A, B ∈ Φ(X); (P2) d2(A, B)= 0 iffA = B; (P3) d2(A, B)= d2(B, A); (P4) IfA ⊆ B ⊆ C,thend2(A, C) ≥ d2(A, B) andd2(A, C) ≥ d2(B, C) Proof. FortwoSVNSs A and B,wehave (P1) 0 ≤ µA (xi ), µB (xi ) ≤ 1, 0 ≤ ρA (xi ), ρB (xi ) ≤ 1 and 0 ≤ νA (xi ), νB (xi ) ≤ 1.Thus, | µA (xi ) µB (xi ) |≤ 1, | ρA (xi ) ρB (xi ) |≤ 1, | νA (xi ) νB (xi ) |≤ 1 and | t(µA (xi ) µB (xi )) |≤ t. Therefore,
(2 + t) | (tνA (xi )+ ρA (xi ) µA (xi )) (tνB (xi )+ ρB (xi ) µB (xi )) |≤ (2 + t)
Hence,bythedefinitionof d2,weobtain0 ≤ d2(A, B) ≤ 1. (P2) Firstly,weassumethat A = B,whichimpliesthat µA (xi )= µB (xi ), ρA (xi )= ρB (xi ),and νA (xi )= νB (xi ) for i = 1,2,.., n.Thus,bythedefinitionof d2,weobtain d2(A, B)= 0. Conversely,assumingthat d2(A, B)= 0fortwoSVNSs A and B,thisimpliesthat
|− t(µA (xi ) µB (xi ))+(ρA (xi ) ρB (xi ))+(νA (xi ) νB (xi ))| + |− t(ρA (xi ) ρB (xi )) (νA (xi ) νB (xi ))+(µA (xi ) µB (xi ))| + |− t(νA (xi ) νB (xi )) (ρA (xi ) ρB (xi ))+(µA (xi ) µB (xi ))|
= 0 or |− t(µA (xi ) µB (xi ))+(ρA (xi ) ρB (xi ))+(νA (xi ) νB (xi ))| = 0 |− t(ρA (xi ) ρB (xi )) (νA (xi ) νB (xi ))+(µA (xi ) µB (xi ))| = 0 |− t(νA (xi ) νB (xi )) (ρA (xi ) ρB (xi ))+(µA (xi ) µB (xi ))| = 0
Aftersolving,weobtain µA (xi ) µB (xi )= 0, ρA (xi ) ρB (xi )= 0 and νA (xi ) νB (xi )= 0, whichimplies µA (xi )= µB (xi ), ρA (xi )= ρB (xi ) and νA (xi )= νB (xi ).Therefore, A = B.Hence d2(A, B)= 0iff A = B (P3) Thisisstraightforwardfromthedefinitionof d2. (P4) If A ⊆ B ⊆ C,then µA (xi ) ≤ µB (xi ) ≤ µC (xi ), ρA (xi ) ≥ ρB (xi ) ≥ ρC (xi ) and νA (xi ) ≥ νB (xi ) ≥ νC (xi ),whichimpliesthat µA (xi ) µB (xi ) ≥ µA (xi ) µC (xi ), νA (xi ) νB (xi ) ≤ νA (xi ) νC (xi ),and ρA (xi ) ρB (xi ) ≤ ρA (xi ) ρC (xi ). Therefore,
|− t(µA (xi ) µB (xi ))+(ρA (xi ) ρB (xi ))+(νA (xi ) νB (xi ))| ≤|− t(µA (xi ) µc (xi ))+(ρA (xi ) ρC (xi ))+(νA (xi ) νC (xi ))|
|− t(ρA (xi ) ρB (xi )) (νA (xi ) νB (xi ))+(µA (xi ) µB (xi ))|
≤|− t(ρA (xi ) ρC (xi )) (νA (xi ) νC (xi ))+(µA (xi ) µC (xi ))| |− t(νA (xi ) νB (xi )) (ρA (xi ) ρB (xi ))+(µA (xi ) µB (xi ))|
≤|− t(νA (xi ) νC (xi )) (ρA (xi ) ρC (xi ))+(µA (xi ) µC (xi ))|
Byadding,weobtain d2(A, B) ≤ d2(A, C).Similarly,weobtain d2(B, C) ≤ d2(A, C)
Proposition2. Distanced4 asdefinedinEquation (11) isalsoavalidmeasure.
Proof. FortwoSVNSs A and B,wehave
(P1) 0 ≤ µA (xi ), µB (xi ) ≤ 1, 0 ≤ ρA (xi ), ρB (xi ) ≤ 1 and 0 ≤ νA (xi ), νB (xi ) ≤ 1 Thus, | µA (xi ) µB (xi ) |≤ 1, | ρA (xi ) ρB (xi ) |≤ 1, | νA (xi ) νB (xi ) |≤ 1 and | t(µA (xi ) µB (xi )) |≤ t.Therefore, |− t(µA (xi ) µB (xi ))+(ρA (xi ) ρB (xi ))+(νA (xi ) νB (xi ))|2 ≤ (2 + t)2 |− t(ρA (xi ) ρB (xi )) (νA (xi ) νB (xi ))+(µA (xi ) µB (xi ))|2 ≤ (2 + t)2 |− t(νA (xi ) νB (xi )) (ρA (xi ) ρB (xi ))+(µA (xi ) µB (xi ))|2 ≤ (2 + t)2
Hence,bythedefinitionof d4,weobtain0 ≤ d4(A, B) ≤ 1. (P2) Assumingthat A = B impliesthat µA (xi )= µB (xi ), ρA (xi )= ρB (xi ) and νA (xi )= νB (xi ) for i = 1,2, ... , n,andhenceusingEquation (11),weobtain d4(A, B)= 0.Conversely,assuming that d4(A, B)= 0implies
|− t(µA (xi ) µB (xi ))+(ρA (xi ) ρB (xi ))+(νA (xi ) νB (xi ))|2 = 0
|− t(ρA (xi ) ρB (xi )) (νA (xi ) νB (xi ))+(µA (xi ) µB (xi ))|2 = 0 |− t(νA (xi ) νB (xi )) (ρA (xi ) ρB (xi ))+(µA (xi ) µB (xi ))|2 = 0
Aftersolvingthese,weobtain µA (xi ) µB (xi )= 0, ρA (xi ) ρB (xi )= 0 and νA (xi ) νB (xi )= 0; thatis, µA (xi )= µB (xi ), ρA (xi )= ρB (xi ) and νA (xi )= νB (xi ) for t ≥ 3.Hence A = B. Therefore, d4(A, B)= 0iff A = B. (P3) Thisisstraightforwardfromthedefinitionof d4. (P4) If A ⊆ B ⊆ C,then µA (xi ) ≤ µB (xi ) ≤ µC (xi ), ρA (xi ) ≥ ρB (xi ) ≥ ρC (xi ),and νA (xi ) ≥ νB (xi ) ≥ νC (xi ).Therefore
|− t(µA (xi ) µB (xi ))+(ρA (xi ) ρB (xi ))+(νA (xi ) νB (xi ))|2
≤|− t(µA (xi ) µc (xi ))+(ρA (xi ) ρC (xi ))+(νA (xi ) νC (xi ))|2 |− t(ρA (xi ) ρB (xi )) (νA (xi ) νB (xi ))+(µA (xi ) µB (xi ))|2 ≤|− t(ρA (xi ) ρC (xi )) (νA (xi ) νC (xi ))+(µA (xi ) µC (xi ))|2 |− t(νA (xi ) νB (xi )) (ρA (xi ) ρB (xi ))+(µA (xi ) µB (xi ))|2
≤|− t(νA (xi ) νC (xi )) (ρA (xi ) ρC (xi ))+(µA (xi ) µC (xi ))|2
Hencebythedefinitionof d4,weobtain d4(A, B) ≤ d4(A, C).Similarly,weobtain d4(B, C) ≤ d4(A, C)
Now,onthebasisoftheseproposeddistancemeasures,weconcludethatthissuccessfully overcomestheshortcomingsoftheexistingmeasuresasdescribedabove.
Example3. Ifweapplytheproposeddistancemeasures d2 and d4 onthedataconsideredinExample 1 toclassify thepattern C,thencorrespondingtotheparameter t = 3,weobtain d2(A, C)= 0.3333, d2(B, C)= 0.1333, d4(A, C)= 0.3464 and d4(B, C)= 0.1633.Thus,thepattern C isclassifiedwiththepattern B andhenceis abletoidentifythebestpattern.
Example4. Ifweutilizetheproposeddistances d2 and d4 fortheabove-consideredExample 2,thentheir correspondingvaluesare d2(A, B)= 0.0267, d2(C, D)= 0.0667, d4(A, B)= 0.0327 and d4(C, D)= 0.6930. Therefore,thereisasignificanteffectofthechangeinthefalsitymembershiponthemeasurevaluesandhence consequentlyontherankingvalues.
Proposition3. Measuresd1 andd3 satisfythefollowingproperties: (i) 0 ≤ d1 ≤ n; (ii) 0 ≤ d3 ≤ n1/2
Proof. Wecaneasilyobtainthat d1(A, B)= nd2(A, B),andthusbyProposition 1,weobtain 0 ≤ d1(A, B) ≤ n.Similarly,wecanobtain0 ≤ d3(A, B) ≤ n1/2 .
However,inmanypracticalsituations,thedifferentsetsmayhavetakendifferentweights, andthusweight ωi (i = 1,2, , n) oftheelement xi ∈ X shouldbetakenintoaccount.Inthe following,wedevelopaweightedHammingdistanceandthenormalizedweightedEuclideandistance betweenSVNSs. (i) ThenormalizedweightedHammingdistance:
5(A, B
1/2 (13) where t ≥ 3isaparameter.
Itisstraightforwardtocheckthatthenormalizedweighteddistance dk (A, B)(k = 5,6) between SVNSs A and B alsosatisfiestheaboveproperties(P1)–(P4). Proposition4. Distancemeasuresd2 andd5 satisfytherelationd5 ≤ d2 Proof. Because ωi ≥ 0, ∑n i=1 ωi = 1,thenforanytwoSVNSs A and B,wehave d5(A, B)= 1 3n(2+t) ∑n i=1 ωi |− t(µA (xi ) µB (xi ))+(ρA (xi ) ρB (xi ))+(νA (xi ) νB (xi ))| + |− t(ρA (xi ) ρB (xi )) (νA (xi ) νB (xi ))+(µA (xi ) µB (xi ))| + |− t(νA (xi ) νB (xi )) (ρA (xi ) ρB (xi ))+ (µA (xi ) µB (xi ))| ≤ 1 3n(2+t) ∑n i=1 |− t(µA (xi ) µB (xi ))+(ρA (xi ) ρB (xi ))+(νA (xi ) νB (xi ))| + |− t(ρA (xi ) ρB (xi )) (νA (xi ) νB (xi ))+(µA (xi ) µB (xi ))| + |− t(νA (xi ) νB (xi )) (ρA (xi ) ρB (xi ))+(µA (xi ) µB (xi ))| ;thatis, d5(A, B) ≤ d2(A, B).
Proposition5. LetAandBbetwoSVNSsinX;thend5 andd6 arethedistancemeasures.
Proof. Because ωi ∈ [0,1] and n ∑ i=1 ωi = 1 thenwecaneasilyobtain 0 ≤ d5(A, B) ≤ d2(A, B). Thus, d5(A, B) satisfies(P1).Theproofsof(P2)–(P4)aresimilartothoseofProposition 1.Similaris truefor d6
Proposition6. Thedistancemeasuresd4 andd6 satisfytherelationd6 ≤ d4 Proof. TheprooffollowsfromProposition 4. Proposition7. Thedistancemeasuresd2 andd4 satisfytheinequalityd4 ≤ √d2. Proof. FortwoSVNSs A and B,wehave |− t(µA (xi ) µB (xi ))+(ρA (xi ) ρB (xi ))+(νA (xi ) νB (xi ))|2 ≤ (2 + t)2
t(ρA (xi ) ρB (xi )) (νA (xi ) νB (xi ))+(µA (xi ) µB (xi ))|2 ≤ (2 + t)2
t(νA (xi ) νB (xi )) (ρA (xi ) ρB (xi ))+(µA (xi ) µB (xi ))|2 ≤ (2 + t)2 whichimpliesthat t(µA (xi ) µB (xi ))+(ρA (xi ) ρB (xi ))+(νA (xi ) νB (xi )) 2 + t 2 ≤ 1 t(ρA (xi ) ρB (xi )) (νA (xi ) νB (xi ))+(µA (xi ) µB (xi )) 2 + t 2 ≤ 1 t(νA (xi ) νB (xi )) (ρA (xi ) ρB (xi ))+(µA (xi ) µB (xi )) 2 + t 2 ≤ 1
Forany a ∈ [0,1],wehave a2 ≤ a.Therefore, t(µA (xi ) µB (xi ))+(ρA (xi ) ρB (xi ))+(νA (xi ) νB (xi )) 2 + t 2 ≤ t(µA (xi ) µB (xi ))+(ρA (xi ) ρB (xi ))+(νA (xi ) νB (xi )) 2 + t t(ρA (xi ) ρB (xi )) (νA (xi ) νB (xi ))+(µA (xi ) µB (xi )) 2 + t 2 ≤ t(ρA (xi ) ρB (xi )) (νA (xi ) νB (xi ))+(µA (xi ) µB (xi )) 2 + t and t(νA (xi ) νB (xi )) (ρA (xi ) ρB (xi ))+(µA (xi ) µB (xi )) 2 + t 2 ≤ t(νA (xi ) νB (xi )) (ρA (xi ) ρB (xi ))+(µA (xi ) µB (xi )) 2 + t
Byaddingtheseinequalitiesandbythedefinitionof d4,wehave d4(A, B)=
1 3n(2 + t)2
n ∑ i=1
t(µA (xi ) µB (xi ))+(ρA (xi ) ρB (xi ))+(νA (xi ) νB (xi )) 2 + t(ρA (xi ) ρB (xi )) (νA (xi ) νB (xi ))+(µA (xi ) µB (xi )) 2 + t(νA (xi ) νB (xi )) (ρA (xi ) ρB (xi ))+(µA (xi ) µB (xi )) 2
1/2 ≤ 1 3n(2 + t) n ∑ i=1
t(µA (xi ) µB (xi ))+(ρA (xi ) ρB (xi ))+(νA (xi ) νB (xi )) + t(ρA (xi ) ρB (xi )) (νA (xi ) νB (xi ))+(µA (xi ) µB (xi )) + t(νA (xi ) νB (xi )) (ρA (xi ) ρB (xi ))+(µA (xi ) µB (xi ))
1/2 ≤ (d2(A, B))1/2
As A and B arearbitrarySVNSs,thusweobtain d4 ≤ √d2
Proposition8. Measuresd6 andd5 satisfytheinequalityd6 ≤ √d5.
i
Proposition9. Thedistance dH 1 (A, B) definedinEquation (14) fortwoSVNSs A and B isavalid distancemeasure.
Proof. Theabovemeasuresatisfiesthefollowingproperties:
(P1) As A and B areSVNSs,so | µA (xi ) µB (xi ) |≤ 1, | ρA (xi ) ρB (xi ) |≤ 1 and | νA (xi ) νB (xi ) |≤ 1.Thus, | (tµA (xi ) νA (xi ) ρA (xi )) (tµB (xi ) νB (xi ) ρB (xi )) |≤ (2 + t) | (tρA (xi )+ νA (xi ) µA (xi )) (tρB (xi )+ νB (xi ) µB (xi )) |≤ (2 + t) | (tνA (xi )+ ρA (xi ) µA (xi )) (tνB (xi )+ ρB (xi ) µB (xi )) |≤ (2 + t)
Hence,bythedefinitionof dH 1 ,weobtain0 ≤ dH 1 (A, B) ≤ 1. (P2) SimilartotheproofofProposition 1. (P3) ThisisclearfromEquation(14). (P4) Let A ⊆ B ⊆ C,whichimplies µA (xi ) ≤ µB (xi ) ≤ µC (xi ), ρA (xi ) ≥ ρB (xi ) ≥ ρC (xi ) and νA (xi ) ≥ νB (xi ) ≥ νC (xi ).Therefore, |− t(µA (xi ) µB (xi ))+(ρA (xi ) ρB (xi ))+(νA (xi ) νB (xi ))|≤|− t(µA (xi ) µc (xi ))+(ρA (xi ) ρC (xi ))+(νA (xi ) νC (xi ))|, |− t(ρA (xi ) ρB (xi )) (νA (xi ) νB (xi ))+(µA (xi ) µB (xi ))|≤|− t(ρA (xi ) ρC (xi )) (νA (xi ) νC (xi ))+ (µA (xi ) µC (xi ))| and |− t(νA (xi ) νB (xi )) (ρA (xi ) ρB (xi ))+(µA (xi ) µB (xi ))|≤ |− t(νA (xi ) νC (xi )) (ρA (xi ) ρC (xi ))+(µA (xi ) µC (xi ))|,whichimpliesthat max i |− t(µA (xi ) µB (xi ))+(ρA (xi ) ρB (xi ))+(νA (xi ) νB (xi ))|, |− t(ρA (xi ) ρB (xi )) (νA (xi ) νB (xi ))+(µA (xi ) µB (xi ))|, |− t(νA (xi ) νB (xi )) (ρA (xi ) ρB (xi ))+(µA (xi ) µB (xi ))| ≤ max i |− t(µA (xi ) µc (xi ))+(ρA (xi ) ρC (xi ))+(νA (xi ) νC (xi ))|, |− t(ρA (xi ) ρC (xi )) (νA (xi ) νC (xi ))+(µA (xi ) µC (xi ))| and |− t(νA (xi ) νC (xi )) (ρA (xi ) ρC (xi ))+ (µA (xi ) µC (xi ))| .Hence dH 1 (A, B) ≤ dH 1 (A, C).Similarly,weobtain dH 1 (B, C) ≤ dH 1 (A, C)
Proposition10. ForA, B ∈ Φ(X),dH 2 ,dH 3 anddH 4 arethedistancemeasures.
Proof. Theprooffollowsfromtheaboveproposition.
Proposition11. ThemeasuresdH 2 anddH 1 satisfythefollowinginequality:dH 2 ≤ dH 1 Proof. Because wi ∈ [0,1],therefore dH 2 (A, B)= 1 3n(2 + t) n ∑ i=1 wi max i |− t(µA (xi ) µB (xi ))+(ρA (xi ) ρB (xi ))+(νA (xi ) νB (xi ))|, |− t(ρA (xi ) ρB (xi )) (νA (xi ) νB (xi ))+(µA (xi ) µB (xi ))|, |− t(νA (xi ) νB (xi )) (ρA (xi ) ρB (xi ))+(µA (xi ) µB (xi ))| ≤ 1 3n(2 + t) n ∑ i=1 max i |− t(µA (xi ) µB (xi ))+(ρA (xi ) ρB (xi ))+(νA (xi ) νB (xi ))|, |− t(ρA (xi ) ρB (xi )) (νA (xi ) νB (xi ))+(µA (xi ) µB (xi ))|, |− t(νA (xi ) νB (xi )) (ρA (xi ) ρB (xi ))+(µA (xi ) µB (xi ))| = dH 1 (A, B) Hence, dH 2 ≤ dH 1
Proposition12. ThemeasuresdH 3 anddH 4 satisfytheinequalitydH 4 (A, B) ≤ dH 3 (A, B) Proof. TheprooffollowsfromProposition 11.
Proposition13. ThemeasuresdH 3 anddH 1 satisfytheinequalitydH 3 ≤ dH 1 Proof. Becauseforany a ∈ [0,1], a2 ≤ a ≤ a1/2,theremainingprooffollowsfromProposition 7
Proposition14. ThemeasuresdH 4 anddH 2 satisfytheinequalitydH 4 ≤ dH 2 . Proof. TheprooffollowsfromProposition 13
Proposition15. ThemeasuresdH 1 andd2 satisfythefollowinginequality: dH 1 ≤ d2 Proof. Forpositivenumbers ai, i = 1,2,..., n,wehave max i {ai }≤ n ∑ i=1 ai.Thus,foranytwo SVNSs A and B,wehave dH 1 (A, B)= 1 3n(2+t) ∑n i=1 maxi |− t(µA (xi ) µB (xi ))+(ρA (xi ) ρB (xi ))+ (νA (xi ) νB (xi ))
Proposition16. ThemeasuresdH 3 andd4 satisfythefollowinginequality: dH 3 ≤ d4 Proof. TheprooffollowsfromProposition 15. Proposition17. Themeasuresd2,d5 anddH 1 satisfythefollowinginequalities:
(i) d2 ≥ d5+dH 1 2 ; (ii) d2 ≥ d5 dH 1 Proof. Because d2 ≥ d5 and d2 ≥ dH 1 ,byaddingtheseinequalities,weobtain d2 ≥ d5 + dH 1 2 .Onthe otherhand,bymultiplyingthese,weobtain d2 ≥ d5 dH 1
4.GeneralizedDistanceMeasure
Theabove-definedHammingandEuclideandistancemeasuresaregeneralizedforthetwoSVNSs A and B ontheuniversalset X asfollows: dp (A, B)= 1 3n(2 + t)p
n ∑ i=1 |− t(µA (xi ) µB (xi ))+(ρA (xi ) ρB (xi ))+(νA (xi ) νB (xi ))|p +|− t(ρA (xi ) ρB (xi )) (νA (xi ) νB (xi ))+(µA (xi ) µB (xi ))|p (18) +|− t(νA (xi ) νB (xi )) (ρA (xi ) ρB (xi ))+(µA (xi ) µB (xi ))|p 1/p where p ≥ 1isan Lp normand t ≥ 3representstheuncertaintyindexparameters. Inparticular,if p = 1 and p = 2,thentheabovemeasure,giveninEquation (18),reducesto measures d2 and d4 definedinEquations(9)and(11),respectively.
Proposition18. Theabove-defineddistance dp (A, B),betweenSVNSs A and B,satisfiesthefollowing properties(P1)–(P4): (P1) 0 ≤ dp (A, B) ≤ 1, ∀A, B ∈ Φ(X); (P2) dp (A, B)= 0, iffA = B; (P3) dp (A, B)= dp (B, A); (P4) IfA ⊆ B ⊆ C,thendp (A, C) ≥ dp (A, B) anddp (A, C) ≥ dp (B, C)
Proof. For p ≥ 1and t ≥ 3,wehavethefollowing:
(P1) ForSVNSs, | µA (xi ) µB (xi ) |≤ 1, | ρA (xi ) ρB (xi ) |≤ 1 and | νA (xi ) νB (xi ) |≤ 1.Thus,we obtain (2 + t) ≤ t(µA (xi ) µB (xi )) (νA (xi ) νB (xi )) (ρA (xi ) ρB (xi )) ≤ (2 + t) (2 + t) ≤−t(ρA (xi ) ρB (xi )) (νA (xi ) νB (xi ))+(µA (xi ) µB (xi )) ≤ (2 + t) (2 + t) ≤−t(νA (xi ) νB (xi )) (ρA (xi ) ρB (xi ))+(µA (xi )) µB (xi ) ≤ (2 + t)
whichimpliesthat
0 ≤ t(µA (xi ) µB (xi )) (νA (xi ) νB (xi )) (ρA (xi ) ρB (xi )) p ≤ (2 + t)p 0 ≤ t(ρA (xi ) ρB (xi )) (νA (xi ) νB (xi ))+(µA (xi ) µB (xi )) p ≤ (2 + t)p 0 ≤ t(νA (xi ) νB (xi )) (ρA (xi ) ρB (xi ))+(µA (xi )) µB (xi ) p ≤ (2 + t)p
Thus,byaddingtheseinequalities,weobtain0 ≤ dp (A, B) ≤ 1. (P2) Assumingthat A = B ⇔ µA (x)= µB (xi ), ρA (xi )= ρB (xi ),and νA (x)= νB (xi ), thus, dp (A, B)= 0.
Conversely,assumingthat dp (A, B)= 0impliesthat
|− t(µA (xi ) µB (xi ))+(ρA (xi ) ρB (xi ))+(νA (xi ) νB (xi ))| = 0 |− t(ρA (xi ) ρB (xi )) (νA (xi ) νB (xi ))+(µA (xi ) µB (xi ))| = 0 |− t(νA (xi ) νB (xi )) (ρA (xi ) ρB (xi ))+(µA (xi ) µB (xi ))| = 0 andhence,aftersolving,weobtain µA (xi )= µB (xi ), ρA (xi )= ρB (xi ) and νA (xi )= νB (xi ). Thus, A = B. (P3) Thisisstraightforward. (P4) Let A ⊆ B ⊆ C;then µA (xi ) ≤ µB (xi ) ≤ µC (xi ), ρA (xi ) ≥ ρB (xi ) ≥ ρC (xi ) and νA (xi ) ≥ νB (xi ) ≥ νC (xi ).Thus, µA (xi ) µB (xi ) ≥ µA (xi ) µC (xi ), ρA (xi ) ρB (xi ) ≤ ρA (xi ) ρC (xi ) and νA (xi ) νB (xi ) ≤ νA (xi ) νC (xi ).Hence,weobtain |− t(µA (xi ) µB (xi ))+(ρA (xi ) ρB (xi ))+(νA (xi ) νB (xi ))|p ≤|− t(µA (xi ) µc (xi ))+(ρA (xi ) ρC (xi ))+(νA (xi ) νC (xi ))|p |− t(ρA (xi ) ρB (xi )) (νA (xi ) νB (xi ))+(µA (xi ) µB (xi ))|p ≤|− t(ρA (xi ) ρC (xi )) (νA (xi ) νC (xi ))+(µA (xi ) µC (xi ))|p and |− t(νA (xi ) νB (xi )) (ρA (xi ) ρB (xi ))+(µA (xi ) µB (xi ))|p ≤|− t(νA (xi ) νC (xi )) (ρA (xi ) ρC (xi ))+(µA (xi ) µC (xi ))|p Thus,weobtain dp (A, B) ≤ dp (A, C).Similarly, dp (B, C) ≤ dp (A, C)
Iftheweightvector ωi, (i = 1,2, , n) ofeachelementisconsideredsuchthat ωi ∈ [0,1] and ∑i ωi = 1,thenageneralizedparametricdistancemeasurebetweenSVNSs A and B takesthe followingform: dp w (A, B)= 1 3n(2 + t)p
n ∑ i=1 ωi |− t(µA (xi ) µB (xi ))+(ρA (xi ) ρB (xi ))+(νA (xi ) νB (xi ))|p +|− t(ρA (xi ) ρB (xi )) (νA (xi ) νB (xi ))+(µA (xi ) µB (xi ))|p +|− t(νA (xi ) νB (xi )) (ρA (xi ) ρB (xi ))+(µA (xi ) µB (xi ))|p 1/p (19)
Inparticular,if p = 1and p = 2,Equation(19)reducestoEquations(12)and(13),respectively. Proposition19. Let ω =(ω1, ω2, , ωn )T betheweightvectorof xi, (i = 1,2, , n) with ωi ≥ 0 and n ∑ i=1 ωi = 1;thenthegeneralizedparametricdistancemeasurebetweentheSVNSs A and B definedby Equation (19) satisfiesthefollowing: (P1) 0 ≤ dp w (A, B) ≤ 1, ∀A, B ∈ Φ(X); (P2) dp w (A, B)= 0 iffA = B; (P3) dp w (A, B)= dp w (B, A); (P4) A ⊆ B ⊆ Cthendp w (A, C) ≥ dp w (A, B) anddp w (A, C) ≥ dp w (B, C) Proof. TheprooffollowsfromProposition 18
5.IllustrativeExamples
Inordertoillustratetheperformanceandvalidityoftheabove-proposeddistancemeasures,two examplesfromthefieldsofpatternrecognitionandmedicaldiagnosishavebeentakenintoaccount.
5.1.Example1:ApplicationofDistanceMeasureinPatternRecognition
Considerthreeknownpatterns A1, A2 and A3,whicharerepresentedbythefollowingSVNSsin agivenuniverse X = {x1, x2, x3, x4}:
A1 = { x1,0.7,0.0,0.1 , x2,0.6,0.1,0.2 , x3,0.8,0.7,0.6 , x4,0.5,0.2,0.3 }
A2 = { x1,0.4,0.2,0.3 , x2,0.7,0.1,0.0 , x3,0.1,0.1,0.6 , x4,0.5,0.3,0.6 }
A3 = { x1,0.5,0.2,0.2 , x2,0.4,0.1,0.2 , x3,0.1,0.1,0.4 , x4,0.4,0.1,0.2 }
Consideranunknownpattern B ∈ SVNS(X),whichwillberecognizedwhere
B = { x1,0.4,0.1,0.4 , x2,0.6,0.1,0.1 , x3,0.1,0.0,0.4 , x4,0.4,0.4,0.7 }
Thenthetargetofthisproblemistoclassifythepattern B inoneoftheclasses A1, A2 or A3.Forthis, proposeddistancemeasures, d1, d2, d3, d4, dH 1 and dH 3 ,havebeencomputedfrom B to Ak (k = 1,2,3) correspondingto t = 3,andtheresultsaregivenasfollows:
d1(A1, B)= 0.5600; d1(A2, B)= 0.2932; d1(A3, B)= 0.4668 d2(A1, B)= 0.1400; d2(A2, B)= 0.0733; d2(A3, B)= 0.1167 d3(A1, B)= 0.3499; d3(A2, B)= 0.1641; d3(A3, B)= 0.3120 d4(A1, B)= 0.1749; d4(A2, B)= 0.0821; d4(A3, B)= 0.1560 dH 1 (A1, B)= 0.0633; dH 1 (A2, B)= 0.0300; dH 1 (A3, B)= 0.0567 dH 3 (A1, B)= 0.1252; dH 3 (A2, B)= 0.0560; dH 3 (A3, B)= 0.1180
Thus,fromthesedistancemeasures,weconcludethatthepattern B belongstothepattern A2.Ontheotherhand,ifweassumethattheweightsof x1, x2, x3 and x4 are0.3,0.4,0.2and0.1, respectively,thenweutilizethedistancemeasures d5, d6, dH 2 and dH 4 forobtainingthemostsuitable patternasfollows:
d5(A1, B)= 0.0338; d5(A2, B)= 0.0162; d5(A3, B)= 0.0233 d6(A1, B)= 0.0861; d6(A2, B)= 0.0369; d6(A3, B)= 0.0604 dH 2 (A1, B)= 0.0148; dH 2 (A2, B)= 0.0068; dH 2 (A3, B)= 0.0117 dH 4 (A1, B)= 0.0603; dH 4 (A2, B)= 0.0258; dH 4 (A3, B)= 0.0464
Thus,therankingorderofthethreepatternsis A2, A3 and A1,andhence A2 isthemostdesirable patterntobeclassifiedwith B.Furthermore,itcanbeeasilyverifiedthattheseresultsvalidatethe above-proposedpropositionsonthedistancemeasures.
ComparisonofExample1ResultswithExistingMeasures
Theabove-mentionedmeasureshavebeencomparedwithsomeexistingmeasuresunderaNS environmentforshowingthevalidityoftheapproachwhoseresultsaresummarizedinTable 1 Fromtheseresults,ithasbeenshownthatthefinalorderingofthepatterncoincideswiththeproposed measures,andhenceitshowstheconservativenatureofthemeasures.
Methods
Table1. OrderingvalueofExample1.
MeasureValueof B from RankingOrder A1 A2 A3
DH (definedinEquation (1))[19]0.32500.12500.2500 A2 A3 A1
Correlationcoefficient[19]0.78830.96750.8615 A2 A3 A1
DNE (definedinEquation (3))[20]0.52510.76740.6098 A1 A3 A2
SCS1 (definedinEquation (4))[22]0.82090.97850.8992 A2 A3 A1
SCS2 (definedinEquation (5))[22]0.89490.99110.9695 A2 A3 A1
ST1 (definedinEquation (6))[42]0.72750.90140.7976 A2 A3 A1
ST2 (definedinEquation (7))[42]0.91430.96730.9343 A2 A3 A1
5.2.Example2:ApplicationofDistanceMeasureinMedicalDiagnosis
Considerasetofdiseases Q = {Q1(Viralfever), Q2(Malaria), Q3(Typhoid), Q4(StomachProblem), Q5 (Chestproblem)} andasetofsymptoms S = {s1 (Temperature), s2 (HeadAche), s3 (StomachPain), s4 (Cough), s5 (Chestpain)}.Supposeapatient,withrespecttoallthesymptoms,canberepresented bythefollowingSVNS:
P(Patient)= {(s1,0.8,0.2,0.1), (s2,0.6,0.3,0.1), (s3,0.2,0.1,0.8), (s4,0.6,0.5,0.1), (s5,0.1,0.4,0.6)} andeachdiseases Qk (k = 1,2,3,4,5) isasfollows:
Q1(Viralfever)= {(s1,0.4,0.6,0.0), (s2,0.3,0.2,0.5), (s3,0.1,0.3,0.7), (s4,0.4,0.3,0.3), (s5,0.1,0.2,0.7)}
Q2(Malaria)= {(s1,0.7,0.3,0.0), (s2,0.2,0.2,0.6), (s3,0.0,0.1,0.9), (s4,0.7,0.3,0.0), (s5,0.1,0.1,0.8)}
Q3(Typhoid)= {(s1,0.3,0.4,0.3), (s2,0.6,0.3,0.1), (s3,0.2,0.1,0.7), (s4,0.2,0.2,0.6), (s5,0.1,0.0,0.9)}
Q4(Stomachproblem)= {(s1,0.1,0.2,0.7), (s2,0.2,0.4,0.4), (s3,0.8,0.2,0.0), (s4,0.2,0.1,0.7), (s5,0.2,0.1,0.7)}
Q5(Chestproblem)= {(s1,0.1,0.1,0.8), (s2,0.0,0.2,0.8), (s3,0.2,0.0,0.8), (s4,0.2,0.0,0.8), (s5,0.8,0.1,0.1)}
Now,thetargetistodiagnosethediseaseofpatient P among Q1, Q2, Q3, Q4 and Q5.Forthis, proposeddistancemeasures, d1, d2, d3, d4, dH 1 and dH 3 ,havebeencomputedfrom P to Qk (k = 1,2, ,5) andaregivenasfollows:
d1(Q1, P)= 0.6400; d1(Q2, P)= 0.9067; d1(Q3, P)= 0.6333; d1(Q4, P)= 1.4600; d1(Q5, P)= 1.6200 d2(Q1, P)= 0.1280; d2(Q2, P)= 0.1813; d2(Q3, P)= 0.1267; d2(Q4, P)= 0.2920; d2(Q5, P)= 0.3240 d3(Q1, P)= 0.3626; d3(Q2, P)= 0.4977; d3(Q3, P)= 0.4113; d3(Q4, P)= 0.7566; d3(Q5, P)= 0.8533 d4(Q1, P)= 0.1622; d4(Q2, P)= 0.2226; d4(Q3, P)= 0.1840; d4(Q4, P)= 0.3383; d4(Q5, P)= 0.3816 dH 1 (Q1, P)= 0.0613; dH 1 (Q2, P)= 0.0880; dH 1 (Q3, P)= 0.0627; dH 1 (Q4, P)= 0.1320; dH 1 (Q5, P)= 0.1400 dH 3 (Q1, P)= 0.1175; dH 3 (Q2, P)= 0.1760; dH 3 (Q3, P)= 0.1373; dH 3 (Q4, P)= 0.2439; dH 3 (Q5, P)= 0.2661
Thus,fromthesedistancemeasures,weconcludethatthepatient P suffersfromthedisease Q3 Ontheotherhand,ifweassignweights0.3,0.2,0.2,0.1and0.2correspondingto Qk (k = 1,2,...,5),respectively,thenweutilizethedistancemeasures d5, d6, dH 2 and dH 4 forobtaining themostsuitablepatternas
d5(Q1, P)= 0.0284; d5(Q2, P)= 0.0403; d5(Q3, P)= 0.0273; d5(Q4, P)= 0.0625; d5(Q5, P)= 0.0684 d6(Q1, P)= 0.0795; d6(Q2, P)= 0.1101; d6(Q3, P)= 0.0862; d6(Q4, P)= 0.1599; d6(Q5, P)= 0.1781 dH 2 (Q1, P)= 0.0135; dH 2 (Q2, P)= 0.0200; dH 2 (Q3, P)= 0.0129; dH 2 (Q4, P)= 0.0276; dH 2 (Q5, P)= 0.0289 dH 4 (Q1, P)= 0.0572; dH 4 (Q2, P)= 0.0885; dH 4 (Q3, P)= 0.0636; dH 4 (Q4, P)= 0.1139; dH 4 (Q5, P)= 0.1226
Thus,onthebasisoftherankingorder,weconcludethatthepatient P suffersfromthedisease Q3
ComparisonofExample2ResultswithExistingApproaches
Inordertoverifythefeasibilityoftheproposeddecision-makingapproachbasedonthedistance measure,weconductedacomparisonanalysisbasedonthesameillustrativeexample.Forthis, variousmeasuresaspresentedinEquations (1)–(7) weretaken,andtheircorrespondingresultsare summarizedinTable 2,whichshowsthatthepatient P suffersfromthedisease Q1
Table2. Comparisonofdiagnosisresultusingexistingmeasures.
ApproachRankingOrder
DH (definedinEquation (1))[19] Q1 Q3 Q2 Q4 Q5 Correlation[19] Q1 Q2 Q3 Q4 Q5
Distancemeasure[27] p = 1 Q3 Q1 Q2 Q4 Q5 p = 2 Q1 Q3 Q2 Q4 Q5 p = 3 Q1 Q3 Q2 Q4 Q5 p = 5 Q1 Q3 Q2 Q4 Q5
DNH (definedinEquation (2))[20] Q3 Q1 Q2 Q4 Q5 DNH (definedinEquation (3))[20] Q1 Q3 Q2 Q4 Q5
SCS1 (definedinEquation (4))[22] Q1 Q3 Q2 Q4 Q5 SCS1 (definedinEquation (5))[22] Q1 Q2 Q3 Q4 Q5 ST1 (definedinEquation (6))[42] Q1 Q3 Q2 Q4 Q5 ST1 (definedinEquation (7))[42] Q1 Q3 Q2 Q4 Q5
5.3.EffectoftheParameterspandtontheOrdering
However,inordertoanalyzetheeffectoftheparameters t and p onthemeasurevalues,an experimentwasperformedbytakingdifferentvaluesof p (p = 1,1.5,2,3,5,10)correspondingtoa differentvalueoftheuncertaintyparameter t (t = 3,5,7).Onthebasisofthesedifferentpairsof parameters,distancemeasureswerecomputed,andtheirresultsaresummarizedinTables 3 and 4, respectively,forExamples1and2correspondingtodifferentcriterionweights.
Fromthese,thefollowinghavebeencomputed:
(i) Forafixedvalueof p,ithasbeenobservedthatthemeasurevaluescorrespondingtoeach alternativeincreasewiththeincreaseinthevalueof t.Ontheotherhand,byvaryingthevalueof t from3to7,correspondingtoafixedvalueof p,thisimpliesthatvaluesofthedistancemeasures ofeachdiagnosisfromthepatient P increase.
(ii) Ithasalsobeenobservedfromthistablethatwhentheweightvectorhasbeenassignedtoeach criterionweight,thenthemeasurevaluesarelessthanthatofanequalweightingcase.
(iii) Finally,itisseenfromthetablethatthemeasuredvaluescorrespondingtoeachalternative Qk (k = 1,2,3,4,5) areconservativeinnature.
Foreachpair,themeasurevaluesliebetween0and1,andhence,onthebasisofthis,we concludethatthepatient P suffersfromthe Q1 disease.Therankingorderforthedecision-makeris showninthetableas(13245),whichindicatesthattheorderofthedifferentattributesisoftheform
Q1 Q3 Q2 Q4 Q5.Hence Q1 isthemostdesirable,while Q5 istheleastdesirablefordifferent valuesof t and p
Table3. Resultsofclassificationofgivensampleusingproposeddistancemeasure.
WhenEqualImportanceIsgiventoEachCriteriaWhenWeightVector (0.3,0.4,0.2,0.1)T IsTaken ptdp ( A1, B) dp ( A2, B) dp ( A3, B) Ranking dp w ( A1, B) dp w ( A2, B) dp w ( A3, B) Ranking 1 30.14000.07330.1167 A2 A3 A1 0.03380.01620.0233 A2 A3 A1 50.16670.07620.1214 A2 A3 A1 0.03870.01700.0248 A2 A3 A1 70.18150.07780.1241 A2 A3 A1 0.04140.01750.0256 A2 A3 A1 1.5 30.15980.07830.1374 A2 A3 A1 0.06200.02770.0426 A2 A3 A1 50.19240.08170.1437 A2 A3 A1 0.07230.02930.0452 A2 A3 A1 70.21160.08380.1480 A2 A3 A1 0.07840.03040.0469 A2 A3 A1 2 30.17490.08210.1560 A2 A3 A1 0.08610.03690.0604 A2 A3 A1 50.21370.08590.1646 A2 A3 A1 0.10210.03920.0644 A2 A3 A1 70.23740.08850.1705 A2 A3 A1 0.11200.04080.0671 A2 A3 A1 3 30.19700.08800.1875 A2 A3 A1 0.12290.05070.0927 A2 A3 A1 50.24690.09290.02012 A2 A3 A1 0.14970.05430.1000 A2 A3 A1 70.27850.09620.2098 A2 A3 A1 0.16720.05660.1046 A2 A3 A1 5 30.22400.09670.2314 A2 A1 A3 0.16800.06890.1469 A2 A3 A1 50.29020.10410.2526 A2 A3 A1 0.21280.07490.1605 A2 A3 A1 70.33260.10870.2650 A2 A3 A1 0.24260.07860.1685 A2 A3 A1 10 30.25640.11070.2830 A2 A1 A3 0.22030.09390.2248 A2 A1 A3 50.34210.12310.3131 A2 A3 A1 0.29150.10470.2487 A2 A3 A1 70.39420.13040.3301 A2 A3 A1 0.33560.11090.2622 A2 A3 A1
30.14650.20230.16000.31750.35740.05530.07680.05790.11540.1282 50.16120.21310.17940.33640.37780.05980.08080.06280.12020.1334 70.17110.22050.19160.34920.39130.06300.08360.06580.12370.1369 2 30.16220.22260.18400.33830.38160.07950.11010.08620.15990.1781 50.17870.23910.20380.36090.40520.08670.11830.09280.16860.1872 70.18950.25010.21680.37600.42110.09140.12380.09720.17440.1933 3 30.18700.26010.21630.37150.41420.11820.16620.13120.22760.2509 50.20610.28760.23760.40040.44210.12970.18420.14090.24360.2666 70.21750.30470.25160.41850.46010.13650.19540.14750.25350.2765 5 30.21850.31870.25310.41700.45040.16750.24710.18920.31270.3354 50.24050.36250.27820.45310.48260.18410.28170.20450.33840.3588 70.25290.38770.29400.47400.50230.19340.30160.21450.35320.3729 10 30.25190.39800.29690.47310.48960.22150.35240.25990.40950.4235 50.27710.45860.32710.51700.52520.24340.40630.28400.44640.4547 70.29120.46240.34510.54200.54660.25560.43630.29810.46750.4730
5.4.AdvantagesoftheProposedMethod
Accordingtotheabovecomparisonanalysis,theproposedmethodforaddressing decision-makingproblemshasthefollowingadvantages:
(i) ThedistancemeasureundertheIFSenvironmentcanonlyhandlesituationsinwhichthe degreeofmembershipandnon-membershipisprovidedtothedecision-maker.Thiskindof measureisunabletodealwithindeterminacy,whichcommonlyoccursinreal-lifeapplications. BecauseSVNSsareasuccessfultoolinhandlingindeterminacy,theproposeddistancemeasurein theneutrosophicdomaincaneffectivelybeusedinmanyrealapplicationsindecision-making.
(ii) Theproposeddistancemeasuredependsupontwoparameters p and t,whichhelpinadjusting thehesitationmarginincomputingdata.Theeffectofhesitationwillbediminishedoralmost neglectedifthevalueof t istakenverylarge,andforsmallervaluesof t,theeffectofhesitation willrise.Thus,accordingtorequirements,thedecision-makercanadjusttheparametertohandle
incompleteaswellasindeterminateinformation.Therefore,thisproposedapproachismore suitableforengineering,industrialandscientificapplications.
(iii) Ashasbeenobservedfromexistingstudies,variousexistingmeasuresunderNSenvironments havebeenproposedbyresearchers,buttherearesomesituationsthatcannotbedistinguishedby theseexistingmeasures;hencetheircorrespondingalgorithmmaygiveanirrelevantresult.The proposedmeasurehastheabilitytoovercometheseflaws;thusitisamoresuitablemeasureto tackleproblems.
6.Conclusions
SVNSsareappliedtoproblemswithimprecise,uncertain,incompleteandinconsistent informationexistingintherealworld.Althoughseveralmeasuresalreadyexisttodealwithsuchkinds ofinformationsystems,theyhaveseveralflaws,asdescribedinthemanuscript.Hereinthisarticle, weovercometheseflawsbyproposinganalternativewaytodefinenewgeneralizeddistancemeasures betweenthetwoSVNNs.Further,afamilyofnormalizedandweightednormalizedHammingand EuclideandistancemeasureshavebeenproposedfortheSVNSs.Somedesirablepropertiesandtheir relationshavebeenstudiedindetail.Finally,adecision-makingmethodhasbeenproposedonthe basisofthesedistancemeasures.Todemonstratetheefficiencyoftheproposedcoefficients,numerical examplesofpatternrecognitionaswellasmedicaldiagnosishavebeentaken.Acomparativestudy, aswellastheeffectoftheparametersontherankingofthealternative,willsupportthetheoryand hencedemonstratethattheproposedmeasuresareanalternativewaytosolvethedecision-making problems.Inthefuture,wewillextendtheproposedapproachtothesoftsetenvironment[43–45],the multiplicativeenvironment[46–48],andotheruncertainandfuzzyenvironments[7,49–53].
Acknowledgments: Theauthorswishtothanktheanonymousreviewersfortheirvaluablesuggestions. Thesecondauthor,Nancy,wassupportedthroughtheMaulanaAzadNationalFellowshipfundedbythe UniversityGrantCommission(No.F1-17.1/2017-18/MANF-2017-18-PUN-82613).
AuthorContributions: H.GargandNancyjointlydesignedtheideaofresearch,planneditsdevelopment. Nancyreviewedtheliteratureandfindingexamples.H.Gargwrotethepaper.Nancymadeacontributiontothe casestudy.H.Garganalyzedthedataandcheckinglanguage.Finally,alltheauthorshavereadandapprovedthe finalmanuscript.
ConflictsofInterest: Theauthordeclaresnoconflictofinterest.
References
1. Zadeh,L.A.Fuzzysets. Inf.Control 1965, 8,338–353.
2. Atanassov,K.T.Intuitionisticfuzzysets. FuzzySetsSyst. 1986, 20,87–96.
3. Xu,Z.S.Intuitionisticfuzzyaggregationoperators. IEEETrans.FuzzySyst. 2007, 15,1179–1187.
4. Garg,H.ConfidencelevelsbasedPythagoreanfuzzyaggregationoperatorsanditsapplicationto decision-makingprocess. Comput.Math.Organ.Theory 2017, 23,546–571.
5. Garg,H.Novelintuitionisticfuzzydecisionmakingmethodbasedonanimprovedoperationlawsandits application. Eng.Appl.Artif.Intell. 2017, 60,164–174.
6. Garg,H.GeneralizedintuitionisticfuzzyinteractivegeometricinteractionoperatorsusingEinsteint-norm andt-conormandtheirapplicationtodecisionmaking. Comput.Ind.Eng. 2016, 101,53–69.
7. Kumar,K.;Garg,H.TOPSISmethodbasedontheconnectionnumberofsetpairanalysis underinterval-valuedintuitionisticfuzzysetenvironment. Comput.Appl.Math. 2016,1–11, doi:10.1007/s40314-016-0402-0.
8. Grzegorzewski,P.Distancebetweenintuitionisticfuzzysetsand/orinterval-valuedfuzzysetsbasedonthe hausdorffmetric. FuzzySetsSyst. 2004, 148,319–328.
9. Garg,H.Anewgeneralizedimprovedscorefunctionofinterval-valuedintuitionisticfuzzysetsand applicationsinexpertsystems. Appl.SoftComput. 2016, 38,988–999.
10. Garg,H.ANewGeneralizedPythagoreanFuzzyInformationAggregationUsingEinsteinOperationsand ItsApplicationtoDecisionMaking. Int.J.Intell.Syst. 2016, 31,886–920.
2017, 8,162
11. Xu,Z.S.;Yager,R.R.Somegeometricaggregationoperatorsbasedonintuitionisticfuzzysets. Int.J.Gen.Syst. 2006, 35,417–433.
12. Garg,H.SomePictureFuzzyAggregationOperatorsandTheirApplicationstoMulticriteria Decision-Making. ArabianJ.Sci.Eng. 2017, 42,5275–5290.
13.
Garg,H.Someseriesofintuitionisticfuzzyinteractiveaveragingaggregationoperators. SpringerPlus 2016, 5,999,doi:10.1186/s40064-016-2591-9.
14. Yager,R.R.Onorderedweightedavergaingaggregationoperatorsinmulti-criteriadecisionmaking. IEEETrans.Syst.ManCybern. 1988, 18,183–190.
15. Garg,H.Anewimprovedscorefunctionofaninterval-valuedPythagoreanfuzzysetbasedTOPSISmethod. Int.J.Uncertain.Quantif. 2017, 7,463–474.
16. Garg,H.Somemethodsforstrategicdecision-makingproblemswithimmediateprobabilitiesinPythagorean fuzzyenvironment. Int.J.Intell.Syst. 2017,1–26,doi:10.1002/int.21949.
17. Smarandache,F. AUnifyingFieldinLogics.Neutrosophy:NeutrosophicProbability,SetandLogic;American ResearchPress;Rehoboth,DE,USA,1999.
18. Wang,H.;Smarandache,F.;Zhang,Y.Q.;Sunderraman,R.Singlevaluedneutrosophicsets. Rev.Air ForceAcad. 2010, 1,10–14.
19. Broumi,S.;Smarandache,F.Correlationcoefficientofintervalneutrosophicset. Appl.Mech.Mater. 2013, 436,511–517.
20. Majumdar,P.,NeutrosophicSetsandItsApplicationstoDecisionMaking.In ComputationalIntelligence forBigDataAnalysis:FrontierAdvancesandApplications;Acharjya,D.,Dehuri,S.,Sanyal,S.,Eds.;Springer InternationalPublishing:Cham,Switzerland,2015;pp.97–115.
21. Ye,J.Multicriteriadecisionmakingmethodusingthecorrelationcoefficientundersingle-valueneutrosophic environment. Int.J.Gen.Syst. 2013, 42,386–394.
22. Ye,J.Improvedcosinesimilaritymeasuresofsimplifiedneutrosophicsetsformedicaldiagnoses. Artif.Intell.Med. 2015, 63,171–179.
23. Kong,L.;Wu,Y.;Ye,J.Misfirefaultdiagnosismethodofgasolineenginesusingthecosinesimilaritymeasure ofneutrosophicnumbers. NeutrosophicSetsSyst. 2015, 8,42–45.
24. Nancy.;Garg,H.AnimprovedscorefunctionforrankingNeutrosophicsetsanditsapplicationto decision-makingprocess. Int.J.Uncertain.Quantif. 2016, 6,377–385.
25. Garg,H.;Nancy.Onsingle-valuedneutrosophicentropyoforder α NeutrosophicSetsSyst. 2016, 14,21–28.
26. Garg,H.;Nancy.Non-linearprogrammingmethodformulti-criteriadecisionmakingproblemsunder intervalneutrosophicsetenvironment. Appl.Intell. 2017,1–15,doi:10.1007/s10489-017-1070-5.
27.
Ye,J.Multipleattributegroupdecision-makingmethodwithcompletelyunknownweightsbasedon similaritymeasuresundersinglevaluedneutrosophicenvironment. J.Intell.FuzzySyst. 2014, 27,2927–2935.
28. Majumdar,P.;Samant,S.K.OnSimilarityandentropyofneutrosophicsets. J.Intell.FuzzySyst. 2014, 26,1245–1252. 29. Ye,J.Similaritymeasuresbetweenintervalneutrosophicsetsandtheirapplicationsinmulticriteria decision-making. Int.J.Intell.FuzzySyst. 2014, 26,165–172. 30. Huang,H.L.Newdistancemeasureofsingle-valuedneutrosophicsetsanditsapplication. Int.J.Intell.Syst. 2016, 31,1021–1032. 31. Broumi,S.;Smarandache,F.Cosinesimilaritymeasureofintervalvaluedneutrosophicsets. NeutrosophicSetsSyst. 2014, 5,15–20.
32. Peng,J.J.;Wang,J.Q.;Wang,J.;Zhang,H.Y.;Chen,Z.H.Simplifiedneutrosophicsetsandtheirapplications inmulti-criteriagroupdecision-makingproblems. Int.J.Syst.Sci. 2016, 47,2342–2358.
33. Ye,J.Amulticriteriadecision-makingmethodusingaggregationoperatorsforsimplifiedneutrosophicsets. J.Intell.FuzzySyst. 2014, 26,2459–2466.
34. Li,Y.;Liu,P.;Chen,Y.Somesinglevaluedneutrosophicnumberheronianmeanoperatorsandtheir applicationinmultipleattributegroupdecisionmaking. Informatica 2016, 27,85–110.
35. Ye,J.Multipleattributedecision-makingmethodbasedonthepossibilitydegreerankingmethodand orderedweightedaggregationoperatorsofintervalneutrosophicnumbers. J.Intell.FuzzySyst. 2015, 28,1307–1317.
Information 2017, 8,162
36. Liu,P.;Liu,X.Theneutrosophicnumbergeneralizedweightedpoweraveragingoperatorandits applicationinmultipleattributegroupdecisionmaking. Int.J.Mach.Learn.Cybern. 2016,1–12, doi:10.1007/s13042-016-0508-0.
37. Peng,J.J.;Wang,J.Q.;Wu,X.H.;Wang,J.;Chen,X.H.Multi-valuedneutrosophicsetsandpoweraggregation operatorswiththeirapplicationsinmulti-criteriagroupdecision-makingproblems. Int.J.Comput.Intell.Syst. 2015, 8,345–363.
38. Nancy.;Garg,H.Novelsingle-valuedneutrosophicdecisionmakingoperatorsunderFranknormoperations anditsapplication. Int.J.Uncertain.Quantif. 2016, 6,361–375.
39. Yang,L.;Li,B.AMulti-CriteriaDecision-MakingMethodUsingPowerAggregationOperatorsfor Single-valuedNeutrosophicSets. Int.J.DatabaseTheoryAppl. 2016, 9,23–32.
40. Tian,Z.P.;Wang,J.;Zhang,H.Y.;Wang,J.Q.Multi-criteriadecision-makingbasedongeneralizedprioritized aggregationoperatorsundersimplifiedneutrosophicuncertainlinguisticenvironment. Int.J.Mach. Learn.Cybern. 2016,1–17,doi:10.1007/s13042-016-0552-9.
41. Smarandache,F. Neutrosophy.NeutrosophicProbability,Set,andLogic,ProQuestInformation&Learning; Infolearnquest:AnnArbor,MI,USA,1998.
42. Ye,J.Anettingmethodforclustering-simplifiedneutrosophicinformation. SoftComput. 2017, 21,7571–7577.
43. Garg,H.;Arora,R.DistanceandsimilaritymeasuresforDualhesistantfuzzysoftsetsandtheirapplications inmulticriteriadecision-makingproblem. Int.J.Uncertain.Quantif. 2017, 7,229–248.
44. Garg,H.;Arora,R.GeneralizedandGroup-basedGeneralizedintuitionisticfuzzysoftsetswithapplications indecision-making. Appl.Intell. 2017,1–13,doi:10.1007/s10489-017-0981-5.
45. Garg,H.;Arora,R.Anonlinear-programmingmethodologyformulti-attributedecision-making problemwithinterval-valuedintuitionisticfuzzysoftsetsinformation. Appl.Intell. 2017,1–16, doi:10.1007/s10489-017-1035-8.
46. Garg,H.ARobustRankingMethodforIntuitionisticMultiplicativeSetsUnderCrisp,IntervalEnvironments andItsApplications. IEEETrans.Emerg.Top.Comput.Intell. 2017, 1,366–374.
47. Garg,H.Generalizedinteractionaggregationoperatorsinintuitionisticfuzzymultiplicativepreference environmentandtheirapplicationtomulticriteriadecision-making. Appl.Intell. 2017,1–17, doi:10.1007/s10489-017-1066-1.
48. Garg,H.Generalizedintuitionisticfuzzymultiplicativeinteractivegeometricoperatorsandtheirapplication tomultiplecriteriadecisionmaking. Int.J.Mach.Learn.Cybern. 2016, 7,1075–1092.
49. Kumar,K.;Garg,H.ConnectionnumberofsetpairanalysisbasedTOPSISmethodonintuitionisticfuzzy setsandtheirapplicationtodecisionmaking. Appl.Intel. 2017,1–8,doi:10.1007/s10489-017-1067-0.
50. Rani,D.;Garg,H.Distancemeasuresbetweenthecomplexintuitionisticfuzzysetsanditsapplicationsto thedecision-makingprocess. Int.J.Uncertain.Quantif. 2017, 7,423–439.
51. Garg,H.GeneralizedPythagoreanfuzzyGeometricaggregationoperatorsusingEinsteint-normand t-conormformulticriteriadecision-makingprocess. Int.J.Intell.Syst. 2017, 32,597–630.
52. Garg,H.AnovelimprovedaccuracyfunctionforintervalvaluedPythagoreanfuzzysetsanditsapplications indecisionmakingprocess. Int.J.Intell.Syst. 2017, 31,1247–1260.
53. Garg,H.AnovelcorrelationcoefficientsbetweenPythagoreanfuzzysetsanditsapplicationsto Decision-Makingprocesses. Int.J.Intell.Syst. 2016, 31,1234–1252.
c 2017bytheauthors.LicenseeMDPI,Basel,Switzerland.Thisarticleisanopenaccess articledistributedunderthetermsandconditionsoftheCreativeCommonsAttribution (CCBY)license(http://creativecommons.org/licenses/by/4.0/).
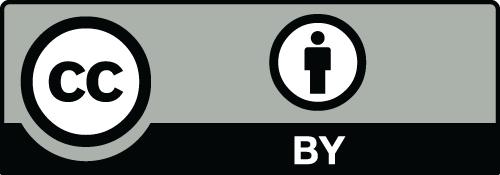