(T, S)-BasedSingle-ValuedNeutrosophicNumber EquivalenceMatrixandClusteringMethod
JiongmeiMoandHan-LiangHuang*
SchoolofMathematicsandStatistics,MinnanNormalUniversity,Zhangzhou363000,China; mojiongmei123@126.com
* Correspondence:huanghl@mnnu.edu.cn
Received:10November2018;Accepted:28December2018;Published:2January2019
Abstract: Fuzzyclusteringiswidelyusedinbusiness,biology,geography,codingfortheinternetand more.Asingle-valuedneutrosophicsetisageneralizedfuzzyset,anditsclusteringalgorithmhas attractedmoreandmoreattention.Anequivalencematrixisacommontoolinclusteringalgorithms. Atpresent,thereexistnoresultsconstructingasingle-valuedneutrosophicnumberequivalence matrixusingt-normandt-conorm.First,theconceptofa (T, S)-basedcompositionmatrixisdefined inthispaper,where (T, S) isadualpairoftriangularmodules.Then,a (T, S)-basedsingle-valued neutrosophicnumberequivalencematrixisgiven.A λ-cuttingmatrixofsingle-valuedneutrosophic numbermatrixisalsointroduced.Moreover,theirrelatedpropertiesarestudied.Finally,anexample andcomparisonexperimentaregiventoillustratetheeffectivenessandsuperiorityofourproposed clusteringalgorithm.

Keywords: single-valuedneutrosophicset;clustering;dualtriangularmodule;equivalencematrix
1.Introduction
In1965,Zadeh[1]proposedtheconceptofafuzzyset(FS)fordealingwithuncertaininformation. Intuitionisticfuzzysets(IFS)wereintroducedbyAtanassov[2]in1986.DifferentfromaFS,anIFS considersmembershipfunctions,non-membershipfunctionsandhesitantfunctions,whichismore suitableforexpressingfuzzyinformation,suchasavotingsystem.Toconsidermoreinformation, AtanassovandGargov[3]extendedIFSstointerval-valuedintuitionisticfuzzysets(IVIFS).However, therearemanysituationswithindeterminateandinconsistentinformationinapplication,whichcan notbehandledbyFS,IFSorIVIFS.Forexample,foraphenomenoninwhichquantumparticlesare neitherexistentnornon-existent,abetterdescriptioncanbeobtainedbyusingthe“neutrosophic” attributeinsteadofthe“fuzzy”attribute.Therefore,theconceptofaneutrosophicset(NS)was developedbySmarandache[4].Wangetal.[5]proposedthesingle-valuedneutrosophicset(SVNS), whichcanbeappliedwithmoreflexibly.Inrecentyears,FS,IFS,IVIFSandSVNShavebeenwidely appliedinmedicaldiagnosis[6,7],anddecisionmaking[8–12].
Clusteringisaprocessofclusteringsimilarthingsintothesamecategory[13–29].ForFS, Seising[17]presentedtheinterwovenhistoricaldevelopmentsofthetwomathematicaltheories, whichopenedupintofuzzypatternclassificationandfuzzyclustering.Chenetal.[18]summedup theFSequivalencematrixclusteringalgorithm.ForIFS,Zhangetal.[19]proposedanintuitionistic fuzzyequivalencematrixclusteringalgorithm.Guanetal.[20]proposedtheintuitionisticfuzzy agglomerativehierarchicalclusteringalgorithmforrecommendationusingsocialtagging.Combining themetaheuristicwithkernelintuitionisticfuzzyc-meansalgorithm,Kuoetal.[21]introducedan evolutionary-basedclusteringalgorithm.Xuetal.[22]developedanorthogonalclusteringalgorithm forintuitionisticfuzzyinformation.Zhaoetal.[23]presentedanintuitionisticfuzzyminimum spanningtreeclusteringalgorithm.ForIVIFS,Sahuetal.[24]developedaprocedureforconstructing
Mathematics 2019, 7,36;doi:10.3390/math7010036
www.mdpi.com/journal/mathematics
ahierarchicalclusteringforIVIFSmax-minsimilarityrelations.Zhang[25]introducedac-means clusteringalgorithminIVIFS.ForSVNS,Ye[26]developedafuzzyequivalencematrixclustering algorithmforSVNS.Guoetal.[27]presentedanovelimagesegmentationalgorithmbasedon neutrosophicc-meansclusteringandtheindeterminacyfilteringmethod.Alietal.[28]proposeda SVNSorthogonalalgorithmforsegmentationofdentalX-Rayimages.Ye[29]introducedaminimum spanningtreeclusteringalgorithminSVNS.
Thetriangularmodule[30–32]isausefulintegrationtoolandiswidelyusedininformation integrationandclustering.Basedonthetriangularmodule,theIFSoperationprinciple[33],thespecific intuitionisticfuzzyaggregationoperator[34],thegeneralizedintuitionisticfuzzyinteractivegeometric interactionoperator[35],theintuitionisticfuzzyheronianmeanoperator[36],thesingle-valued neutrosophicnumberweightedaveragingoperatorandtheweightedgeometricoperator[37]aregiven bymanyresearchers.Huang[38]introducedthe (T, S)-basedIVIFcompositionmatrix,the (T, S)-based IVIFequivalencematrixanditsapplicationforclustering,where (T, S) isadualtriangularmodule.
Atpresent,noonehasstudiedtheconstructionofanequivalencematrixbasedonthedual triangularmoduleunderanSVNSenvironment.Therefore,themainworkofthispaperistopropose theoperationofthe (T, S)-basedcompositionmatrixand (T, S)-basedsingle-valuedneutrosophic numberequivalencematrix.Bycomparingwiththemethodsintheliterature[18,19,28],ourmethod hasthefollowingadvantages:(1)Theclassificationresultsarestablewhen λ isinacertainrange. (2)Themethodsoftheliterature[18,19]canonlydivideobjectsintothreeclassifications,andthemethod ofliterature[28]canonlydivideobjectsintofourclassifications,whileourclusteringalgorithmcan divideobjectsintofiveclassifications.(3)Manyexistingcompositionmatricesarefuzzymatrix,while ourproposedcompositionmatrixisasingle-valuedneutrosophicnumbermatrix,whichcanbetter preservetheprimitivenessofthedata.
Thestructureofthepaperisasfollows:InSection 2,somebasicnotions,operationsandrelations arereviewed.InSection 3,intheSVNSenvironment,theconceptsof (T, S)-basedcompositionmatrix and (T, S)-basedsingle-valuedneutrosophicnumberequivalencematrixaredefined,where (T, S) isa dualpairoftriangularmodule.Theirpropertiesareinvestigated.InSection 4,aclusteringalgorithm with (T, S)-basedsingle-valuedneutrosophicnumberequivalencematrixisproposed.InSection 5, wegiveanumericalexampletoillustratetheeffectivenessoftheproposedmethod.Comparedwith otherexistingmethods,ourmethodhasbetterclassificationabilityandmorereasonableclassification results.InSection 6,weconcludethispaper.
2.Preliminaries
Inthissection,webrieflyintroducesomeofthebasicdefinitionstobeusedinthispaper. Tofacilitatereading,someconceptsareabbreviatedinthispaper.
FS:fuzzyset
FEM:fuzzyequivalencematrix
IFS:intuitionisticfuzzyset
IVIFS:interval-valuedintuitionisticfuzzyset
IFEM:intuitionisticfuzzyequivalencematrix
NS:neutrosophicset
SVNS:single-valuedneutrosophicset
SVNN:single-valuedneutrosophicnumber
SVNNM:single-valuedneutrosophicnumbermatrix
SVNNSM:single-valuedneutrosophicnumbersimilaritymatrix
(T, S)-SVNNEM: (T, S)-basedsingle-valuedneutrosophicnumberequivalencematrix
TheNSwasproposedin1995bySmarandache.
Definition1 ([4]). Let X beauniverseofdiscourse,withagenericelementin X denotedby x.ANS A in X is
A(x)= x|(tA (x), iA (x), f A (x)) ,
where tA (x) denotesthetruth-membershipfunction, iA (x) denotestheindeterminacy-membershipfunction, andf A (x) denotesthefalsity-membershipfunction.tA (x),iA (x),andf A (x) arerealstandardornonstandard subsetsof ] 0,1+ [.Thereisnolimitationonthesumof tA (x), iA (x),and f A (x),so 0 ≤ tA (x)+ iA (x)+ f A (x) ≤ 3+
ToapplytheNS,Wangetal.developedtheconceptofSVNS.SomerelationshipsofSVNSs werediscussed.
Definition2 ([5]). LetXbeauniverseofdiscourse,withagenericelementinXdenotedbyx.ASVNSAin Xisdepictedbythefollowing:
A(x)= x|(tA (x), iA (x), f A (x)) ,
where tA (x) denotesthetruth-membershipfunction, iA (x) denotestheindeterminacy-membershipfunction, and f A (x) denotesthefalsity-membershipfunction.Foreachpoint x in X,wehave tA (x), iA (x), f A (x) ∈ [0,1] and 0 ≤ tA (x)+ iA (x)+ f A (x) ≤ 3.Forconvenience,wecanuse α =(t, i, f ) torepresentaSVNN.
Definition3 ([5]). Let A = { x, tA (x), iA (x), f A (x) |x ∈ X} and B = { x, tB (x), iB (x), fB (x) |x ∈ X} betwoSVNSs.Thefollowingrelationshold:
(1) Inclusion:A ⊆ BifonlyiftA (x) ≤ tB (x),iA (x) ≥ iB (x),f A (x) ≥ fB (x). (2) Complement:Ac = { x, f A (x),1 iA (x), tA (x) |x ∈ X} (3) Union:A ∪ B = { x, tA (x) ∨ tB (x), iA (x) ∧ iB (x), f A (x) ∧ fB (x) |x ∈ X} (4) Intersection:A ∩ B = { x, tA (x) ∧ tB (x), iA (x) ∨ iB (x), f A (x) ∨ fB (x) |x ∈ X}
InordertodevelopSVNNSM,theneutrosophicnumbersimilarityrelationandsimilaritymatrix arereviewed.
Definition4 ([28]). LetAandBbetwoNSs.Thefollowing
R = { (a, b), tR (a, b), iR (a, b), fR (a, b) |a ∈ A, b ∈ B} iscalledaneutrosophicrelation,where tR : A × B → [0,1], iR : A × B → [0,1], fR : A × B → [0,1]; 0 ≤ tR (a, b)+ iR (a, b)+ fR (a, b) ≤ 3,forany (a, b) ∈ A × B.
Definition5 ([28]). LetAandBbetwoNSsandRbeaneutrosophicrelation.If (a) (Reflexivity)tR (a, a)= 1, iR (a, a)= 0, fR (a, a)= 0,foranya ∈ A; (b) (Symmetry)tR (a, b)= tR (b, a), iR (a, b)= iR (b, a), fR (a, b)= fR (b, a),forany (a, b) ∈ A × B.
ThenRiscalledaneutrosophicsimilarityrelation.
Definition6 ([28]). Given A and B betwoNSsonauniverse U and R isaneutrosophicsimilarityrelation. Amatrix M =(rpq )n×n iscalledaneutrosophicsimilaritymatrixif rpq = R(yp, yq ),where p, q = 1,2,..., n and tpq, ipq, f pq arethetruth,indeterminacyandfalsehoodmembershipvaluesofanelement (yp, yq ) ∈ A × B respectively.Equivalently,R(yp, yq )=(tpq, ipq, f pq ) thatimpliesrpq =(tpq, ipq, f pq )
Theaboveintroductioncanbeextendedtothefollowingconclusion.
If pij =(tij, iij, fij )(i, j = 1,2,..., n) areSVNNs,then P =( pij )n×n iscalledaSVNNM, where pij =(tij, iij, fij )(i, j = 1,2,..., n).Further,if P satisfiesthefollowingconditions:
(1) Reflexivity: pii =(1,0,0)(i = 1,2,..., n);
(2) Symmetry: pij = pji,i.e., (tij, iij, fij )=(tji, iji, f ji )(i, j = 1,2,..., n)
Then P isaSVNNSM.
KlirandYuanproposedthet-normandt-conormin1995.
Definition7 ([30]). Afunction T : [0,1] × [0,1] → [0,1] iscalledatriangularnorm,ifitsatisfiesthe followingconditions:
(1) T(0,0)= 0,T(1,1)= 1;
(2) T(x, y)= T(y, x),foranyxandy;
(3) T(x, T(y, z))= T(T(x, y), z),foranyx, yandz; (4) ifx1 ≤ x2, y1 ≤ y2,thenT(x1, y1) ≤ T(x2, y2).
Furthermore,forany a ∈ [0,1], T isat-normif T(a,1)= a, T isat-conormif T(0, a)= a.Inthis paper,denote T, S asthet-normandt-conorm,respectively.
Lemma1 ([31]) Foranyx, y ∈ [0,1],wehave
(1) 0 ≤ T(x, y) ≤ x ∧ y ≤ 1,thatisT ≤∧ andT(0, y)= 0; (2) 0 ≤ x ∨ y ≤ S(x, y) ≤ 1,thatis ∨≤ SandS(1, y)= 1.
Definition8 ([31]). Let x ∈ [0,1], x = 1 x isthecomplementof x.If T and S satisfy T(x, y) = S(x , y ), foranyx,y∈ [0,1],thenTandSiscalledadualpairoftriangularmodule.
Following,wecalladualpairoftriangularmodulesasadualtriangularmoduleforshort,denoted by (T, S)
Further,basedonadualtriangularmodule,Smarandacheproposedtheconceptsofgeneralized unionandintersection.
Definition9 ([32]). Let αi =(ti, ii, fi )(i = 1,2) beanytwoSVNNs,thenthegeneralizedunionand intersectionaredefinedasfollows:
(1) α1 α2 =(S(t1, t2), T(i1, i2), T( f1, f2)); (2) α1 α2 =(T(t1, t2), S(i1, i2), S( f1, f2))
(1)
Severalcommonlyuseddualtriangularmodulesarelistedbelow:
TypeI(minandmaxt-normandt-conorm): T(a, b)= min(a, b), S(a, b)= max(a, b);
TypeII(Algebraict-normandt-conorm): T(a, b)= ab, S(a, b)= a + b ab; (3) TypeIII(Einsteint-normandt-conorm): T(a, b)= ab 1+(1 a)(1 b) , S(a, b)= a+b 1+ab ; (4) TypeIV(Hamachert-normandt-conorm): T(a, b)= ab γ+(1 γ)(a+b ab) , S(a, b)= a+b ab (1 γ)ab 1 (1 γ)ab (γ ∈ (0, +∞))
(2)
BasedonDefinition 9 andthedualtriangularmodulesofthetypeI-IV,thecorresponding generalizedunionandintersectionareexpressedas: α1 w α2, α1 w α2(w = I, II, III, IV).
3.MainResults
Inthissection,wefirststudysomepropertiesofgeneralizedunionsandintersections.Moreover, weintroducetheconceptofa (T, S)-basedSVNNcompositionmatrixandinvestigateitsproperties. Finally,wedevelopthe λ-cuttingmatrixandthe (T, S)-SVNNEM.Theirrelationshipisalsostudied.
3.1.SomePropertiesofGeneralizedUnionsandIntersections
Thepropertiesofgeneralizedunionsandintersectionshavenotbeenstudiedinliterature[32]. Sowefirstinvestigatesomepropertiesofgeneralizedunionsandintersections.
SomeoperationalpropertiesofgeneralizedunionsandintersectionsareproposedinTheorem 1
Theorem1. Let αi =(ti, ii, fi )(i = 1,2,3) beanythreeSVNNs,then
(1) α1 α2 = α2 α1; (2) α1 α2 = α2 α1; (3) (α1 α2) α3 = α1 (α2 α3); (4) (α1 α2) α3 = α1 (α2 α3); (5) (α1 α2)c = αc 1 αc 2; (6) (α1 α2)c = αc 1 αc 2 Proof. (1)and(2)areobvious.Weonlyprove(3)and(5).Theproofsof(4)and(6)areanalogous. (3) (α1 α2) α3 =(S(t1, t2), T(i1, i2), T( f1, f2)) (t3, i3, f3) =(S(S(t1, t2), t3), T(T(i1, i2), i3), T(T( f1, f2), f3)) =(S(t1, S(t2, t3)), T(i1, T(i2, i3)), T( f1, T( f2, f3))) = α1 (α2 α3) (5) (α1 α2)c =(S(t1, t2), T(i1, i2), T( f1, f2))c =(T( f1, f2),1 T(i1, i2), S(t1, t2)) αc 1 αc 2 =( f1,1 i1, t1) ( f2,1 i2, t2) =(T( f1, f2), S(1 i1,1 i2), S(t1, t2)) =(T( f1, f2),1 T(i1, i2), S(t1, t2)) =(α1 α2)c Therefore,wecompletetheproof.
Basedon(5)and(6)inTheorem 1,wehaveCorollary 1
Corollary1. If {Aτ : τ ∈ Λ}(Λ = {1,2,..., n}) isasetofSVNNs,then (1) ( n τ=1 ατ )c = n τ=1 αc τ ; (2) ( n τ=1 ατ )c = n τ=1 αc τ .
Theorem2. Let α1, α2, α3 beanythreeSVNNs,fortypeIdualtriangularmodule,thatis T(a, b)= min(a, b), S(a, b)= max(a, b),thefollowingequationshold.
(1) (α1 I α2) I α3 =(α1 I α3) I (α2 I α3); (2) (α1 I α2) I α3 =(α1 I α3) I (α2 I α3) Proof. AccordingtoDefinition 9,when T(a, b)= min(a, b), S(a, b)= max(a, b),weobtain (1) (α1 I α2) I α3 =(min{max{t1, t2}, t3}, max{min{i1, i2}, i3}, max{min{ f1, f2}, f3}) =(max{min{t1, t3}, min{t2, t3}}, min{max{i1, i3}, max{i2, i3}}, min{max{ f1, f3}, max{ f2, f3}}) =(α1 I α3) I (α2 I α3) and (2) (α1 I α2) I α3 =(max{min{t1, t2}, t3}, min{max{i1, i2}, i3}, min{max{ f1, f2}, f3}) =(min{max{t1, t3}, max{t2, t3}}, max{min{i1, i3}, min{i2, i3}}, max{min{ f1, f3}, min{ f2, f3}}) =(α1 I α3) I (α2 I α3) Therefore,wecompletetheproof.
FortypeII-IVdualtriangularmodule, (α1 α2) α3 =(α1 α3) (α2 α3) and (α1 α2) α3 = (α1 α3) (α2 α3) donothold.SeeExample 1.
Example1. Let α1 =(0.7,0.4,0.1), α2 =(0.5,0.3,0.3), α3 =(0.4,0.8,0.2) bethreeSVNNs. IfT(a, b)= ab, S(a, b)= a + b ab,then
(α1 II α2) II α3 =(0.34,0.824,0.224) =(0.424,0.7568,0.1232)=(α1 II α3) II (α2 II α3). (α1 II α2) II α3 =(0.61,0.464,0.074) =(0.574,0.4832,0.0788)=(α1 II α3) II (α2 II α3).
IfT(a, b)= ab 1+(1 a)(1 b) ,S(a, b)= a+b 1+ab ,then (α1 III α2) III α3 =(0.3333,0.4687,0.2351) =(0.3773,0.7983,0.101)=(α1 III α3) III (α2 III α3). (α1 III α2) III α3 =(0.6279,0.4651,0.0521) =(0.6227,0.4681,0.0501)=(α1 III α3) III (α2 III α3).
IfT(a, b)= ab γ+(1 γ)(a+b ab) ,S(a, b)= a+b ab (1 γ)ab 1 (1 γ)ab , λ = 4,then (α1 IV α2) IV α3 =(0.3276,0.832,0.2131) =(0.3077,0.8497,0.0857)=(α1 IV α3) IV (α2 IV α3). (α1 IV α2) IV α3 =(0.6471,0.4665,0.0354) =(0.6948,0.4323,0.029)=(α1 IV α3) IV (α2 IV α3).
3.2.A (T, S)-BasedSingle-ValuedNeutrosophicNumberCompositionMatrixandItsProperties
Weintroducethenewconceptofa (T, S)-basedcompositionmatrixanditsrelated propertiesbelow.
Definition10. Let Pl =(pl ij )n×n (l = 1,2) betwoSVNNMs,where pl ij =(tl ij, il ij, f l ij )(i, j = 1,2,..., n; l = 1,2).ThenP = P1 × P2 =(pij )n×n iscalleda (T, S)-basedcompositionmatrixofP1 andP2,where pij = ∨n k=1(p1 ik p2 kj )=(∨n k=1T(t1 ik, t2 kj ), ∧n k=1S(i1 ik, i2 kj ), ∧n k=1S( f 1 ik, f 2 kj ))
BasedonDefinition 10 andthedualtriangularmodulesofthetypeI-IV,thecorresponding (T, S)-basedcompositionmatrixisexpressedas: P = P1 ×w P2(w = I, II, III, IV)
ByDefinitions 11 and 10,somepropertiesof (T, S)-basedcompositionmatrixarestudied.
Theorem3. Let Pl =(pl ij )n×n (l = 1,2) betwoSVNNMs,where pl ij =(tl ij, il ij, f l ij )(i, j = 1,2,..., n; l = 1,2). Thenthe (T, S)-basedcompositionmatrixofP1 andP2 isstillaSVNNM.
Proof. By
0 ≤ T(tik, tkj ) ≤ 1,0 ≤ S(iik, ikj ) ≤ 1,0 ≤ S( fik, fkj ) ≤ 1(k = 1,2,..., n), wehave
0 ≤∨n k=1T(tik, tkj ) ≤ 1,0 ≤∧n k=1S(iik, ikj ) ≤ 1,0 ≤∧n k=1S( fik, fkj ) ≤ 1. Thatmeans 0 ≤∨n k=1T(tik, tkj )+ ∧n k=1S(iik, ikj )+ ≤∧n k=1S( fik, fkj ) ≤ 3, whichcompletestheproof.
AccordingtoTheorem 3,wegetCorollary 2.
Corollary2. Let Pl (l = 1,2) beanytwoSVNNSMs.Thenthe (T, S)-basedcompositionmatrixof P1 and P2 isalsoaSVNNM.
However,the (T, S)-basedcompositionmatrixoftwoSVNNSMsmaynotbeaSVNNSM.See Example 2
Example2. Let P1 =
(1,0,0)(0.3,0.4,0.2)(0.5,0.4,0.7) (0.3,0.4,0.2)(1,0,0)(0.8,0.2,0.1) (0.5,0.4,0.7)(0.8,0.2,0.1)(1,0,0)
(1,0,0)(0.1,0.2,0.2)(0.4,0.5,0.2) (0.1,0.2,0.2)(1,0,0)(0.3,0.3,0.1) (0.4,0.5,0.2)(0.3,0.3,0.1)(1,0,0)
Thenboth P1 and P2 areSVNNSMs.WhenwechoosetypeIandIIdualtriangularmodules,their (T, S)-basedcompositionmatrixis
P = P1 ×I P2 = P1 ×II P2 =
(1,0,0)(0.3,0.2,0.2)(0.5,0.4,0.2) (0.3,0.2,0.2)(1,0,0)(0.8,0.2,0.1) (0.5,0.36,0.2)(0.8,0.2,0.1)(1,0,0) p13 = p31 whichmeansPisnotaSVNNSM.
Fortunately,if P isaSVNNSM,then (T, S)-basedcompositionmatrixof P and P isstillaSVNNSM.
Theorem4. Let P =(pij )n×n (i, j = 1,2,..., n) beaSVNNSM.Thenthe (T, S)-basedcompositionmatrix P2 = P × PisalsoaSVNNSM.
Proof. Let P2 =(qij )n×n and pij =(tij, iij, fij )(i, j = 1,2,..., n).
(1) ByCorollary 2,weknowthat P2 isaSVNNM.
(2) ByDefinition 10,for i = 1,2,..., n,wehave qii =(∨n k=1T(tik, tki ), ∧n k=1S(iik, iki ), ∧n k=1S( fik, fki )). When k = i,wehave pii =(tii, iii, fii )=(1,0,0).Since T(1,1)= 1 and S(0,0)= 0,wecanget qii =(1,0,0)(i = 1,2,..., n).
(3) Since P isaSVNNSM, pik = pki,i.e., (tik, iik, fik )=(tki, iki, fki )(i, k = 1,2,..., n).Thenwehave qij =(∨n k=1T(tik, tkj ), ∧n k=1S(iik, ikj ), ∧n k=1S( fik, fkj )) =(∨n k=1T(tkj, tik ), ∧n k=1S(ikj, iik ), ∧n k=1S( fkj, fik )) =(∨n k=1T(tjk, tki ), ∧n k=1S(ijk, iki ), ∧n k=1S( f jk, fki )) = qji
Thus, P2 isaSVNNSM.
Fromdefinition 10 andTheorem 3,wecangetProperty 1.
Property1. LetPl =(pl ij )n×n (l = 1,2) beanytwoSVNNMs.ThenP1 × P2 = P2 × P1
TherelationshipofSVNNMsisdefinedinthefollowing:
Definition11. Let Pl =(pl ij )n×n (l = 1,2) beanytwoSVNNMs,where pl ij =(tl ij, il ij, f l ij )(i, j = 1,2,..., n; l = 1,2).Ifp1 ij ≤ p2 ij,i.e.,t1 ij ≤ t2 ij, i1 ij ≥ t2 ij, f 1 ij ≥ f 2 ij,thenP1 ⊆ P2.
AccordingtoDefinition 11,Theorem 3 andLemma 1,wehavethefollowingproperty:
Property2. LetPl (l = 1,2,3) beanythreeSVNNMsandP1 ⊆ P2.ThenP1 × P3 ⊆ P2 × P3 ⊆ P2 ×I P3 3.3. (T, S)-SVNNEMand λ-CuttingMatrix
Inordertocluster,wedevelop (T, S)-SVNNEMand λ-cuttingmatrix.Wealsoinvestigatetheir relatedproperties.
Definition12. Let P =(pij )n×n beaSVNNM,where pij =(tij, iij, fij )(i, j = 1,2,..., n).If P satisfiesthe followingconditions:
(1) Reflexivity:pii =(1,0,0)(i = 1,2,..., n); (2) Symmetry:pij = pji,i.e. (tij, iij, fij )=(tji, iji, f ji )(i, j = 1,2,..., n);
(3) (T, S)-Transitivity: P2 = P × P ⊆ P,i.e. ∨n k=1T(tik, tkj ) ≤ tij, ∧n k=1S(iik, ikj ) ≥ iij, ∧n k=1S( fik, fkj ) ≥ fij. ThenPiscalleda (T, S)-SVNNEM.
Ingeneral,aSVNNSMmaynota (T, S)-SVNNEM.
Example3. Let P =
(1,0,0)(0.2,0.4,0.6)(0.9,0.5,0.7) (0.2,0.4,0.6)(1,0,0)(0.4,0.2,0.5) (0.3,0.5,0.7)(0.4,0.2,0.5)(1,0,0)
thenPsatisfiesreflexivityandsymmetry.FordualtriangularmodulesoftypeI-IV,weobtain P ×I P = (1,0,0)(0.4,0.4,0.6)(0.9,0.4,0.6) (0.4,0.4,0.6)(1,0,0)(0.4,0.2,0.5) (0.9,0.4,0.6)(0.4,0.2,0.5)(1,0,0)
⊆ P P ×II P = (1,0,0)(0.36,0.4,0.6)(0.9,0.5,0.7) (0.36,0.4,0.6)(1,0,0)(0.4,0.2,0.5) (0.9,0.5,0.7)(0.4,0.2,0.5)(1,0,0) ⊆ P P ×III P = (1,0,0)(0.34,0.4,0.6)(0.9,0.5,0.7) (0.36,0.4,0.6)(1,0,0)(0.4,0.2,0.5) (0.9,0.5,0.7)(0.4,0.2,0.5)(1,0,0)
⊆ P and P ×IV P =
(1,0,0)(0.31,0.4,0.6)(0.9,0.5,0.7) (0.36,0.4,0.6)(1,0,0)(0.4,0.2,0.5) (0.9,0.5,0.7)(0.4,0.2,0.5)(1,0,0) ⊆ P where λ = 4. ItshowsthatPisnota (T, S)-SVNNEMfordualtriangularmodulesoftypeI-IV.
Inordertoobtainclusteringalgorithm,wegivethe λ-cuttingmatrix. Definition13. Let P =(pij )n×n =(tij, iij, fij )(i, j = 1,2,..., n) beaSVNNMand λ beaSVNN.Wecall Pλ =(( pij )λ )n×n isa λ-cuttingmatrixofP,where (pij )λ =((tij )λ , (iij )λ , ( fij )λ )= (1,0,0), if λ ⊆ pij, (0,1,1), if λ ⊆ pij
Following,wewillstudytherelationshipbetweenSVNNSMandthe λ-cuttingmatrix ofSVNNSM.
Theorem5. P =(pij )n×n (i, j = 1,2,..., n) isaSVNNSMifandonlyifPλ isaSVNNSMforanySVNN λ Proof. ” ⇒ ”Let P beaSVNNSM.Then pii =(1,0,0), pij = pji.
(1) Reflexivity:Since pii =(1,0,0)(i = 1,2,..., n),wehave λ ⊆ pii foreachSVNN λ ByDefinition 13,weget (pii )λ =(1,0,0)
(2) Symmetry:By pij = pji,wecaneasilygetthat (pij )λ =(pji )λ (i, j = 1,2,..., n) foreachSVNN λ
Thatis, Pλ isaSVNNSM. ” ⇐ ”Let Pλ beaSVNNSM.Then (Pii )λ =(1,0,0), (pij )λ =(pji )λ (i, j = 1,2,..., n)
(1) Reflexivity:Since (Pii )λ =(1,0,0)(i = 1,2,..., n),weknowthat λ ⊆ pii =(tii, iii, fii ) foreach SVNN λ =(t, i, f ),thatis t ≤ tii, i≥iii and f ≥ fii.Let λ =(1,0,0),then pii =(1,0,0)(i = 1,2,..., n).
(2) Symmetry:If pij = pji,then tij = tji or iij = iji or fij = f ji.Suppose tij < tji, λ =( tij +tji 2 , iji, f ji ). Then tij < tij +tji 2 < tji.So (pij )λ =(0,1,1), (pji )λ =(1,0,0) (pij )λ =(pji )λ ,whichisa contradiction.Therefore, tij = tji (i, j = 1,2,..., n)
Analogously,wecanprovethat iij = iji and fij = f ji shouldbeheldsimultaneously.Itimplies that pij = pji (i, j = 1,2,..., n). Thatis, P isaSVNNSM.
WhentheconditionandconclusionofTheorem 5 arestrengthened,theTheorem 6 holds. Theorem6. IfPλ isa (T, S)-SVNNEMforanySVNN λ,thenPisa (T, S)-SVNNEM.
Proof. ReflexivityandsymmetryareprovedinTheorem 5.Following,weprove P satisfies (T, S)-Transitivity. (T, S)-Transitivity:Since Pλ =(( pij )λ )n×n =((tij )λ , (iij )λ , ( fij )λ )(i, j = 1,2,..., n) satisfies (T, S)-Transitivity,wehave
∨n k=1T((tik )λ , (tkj )λ ) ≤ (tij )λ , ∧n k=1S((iik )λ , (ikj )λ ) ≥ (iij )λ , ∧n k=1S(( fik )λ , ( fkj )λ ) ≥ ( fij )λ
Nowwewillprovethat
∨n k=1T(tik, tkj ) ≤ tij, ∧n k=1S(iik, ikj ) ≥ iij, ∧n k=1S( fik, fkj ) ≥ fij
Assumethereexist i0 and j0 suchthat ∨n k=1(ti0k ∧ tkj0 ) > ti0 j0 .Furtherweknowthatthereexists l suchthat ti0l ∧ tlj0 > ti0 j0 .Suppose ti0l ≥ tlj0 > ti0 j0 and λ =(tlj0 , ii0l ∨ ilj0 , fi0l ∨ flj0 ),then (pi0l )λ =(plj0 )λ =(1,0,0) and (pi0 j0 )λ =(0,1,1) hold.Theseimplythat
∨n k=1T((ti0k )λ , (tkj0 )λ )= ∨n k=1T(1,1)= 1 > 0 =(ti0 j0 )λ , whichproducesthecontradictionwith
∨n k=1T((tik )λ , (tkj )λ ) ≤ (tij )λ Sowehave
∨n k=1T(tik, tkj ) ≤∨n k=1(tik ∧ tkj ) ≤ tij Assumethereexist i1 and j1 suchthat ∧n k=1(ii1k ∨ ikj1 ) < ii1 j1 .Furtherweknowthatthereexists m suchthat ii1m ∨ imj1 < ii1 j1 .Suppose ii1m ≤ imj1 < ii1 j1 and λ =(ti1m ∧ tmj1 , imj1 , fi1m ∨ fmj1 ),then (pi1m )λ =(pmj1 )λ =(1,0,0) and (pi1 j1 )λ =(0,1,1) hold.Theseimplythat
∧n k=1S((ii1k )λ , (ikj1 )λ )= ∧n k=1S(0,0)= 0 < 1 =(ii1 j1 )λ , whichproducesthecontradictionwith
∧n k=1S((iik )λ , (ikj )λ ) ≥ (iij )λ Sowehave
∧n k=1S(iik, ikj ) ≥∧n k=1(iik ∨ ikj ) ≥ iij.
Analogouslywecanprovethat ∧n k=1S( fik, fkj ) ≥ fij. Thus,wecancompletetheproof.
Conversely,if P isa (T, S)-SVNNEM,then Pλ maynota (T, S)-SVNNEM.SeeExample 4,where wechoosedualtriangularmoduleoftypeIIforexplanation. Example4. Let P =
(1,0,0)(0.3,0.2,0.1)(0.6,0.5,0.2) (0.3,0.2,0.1)(1,0,0)(0.7,0.4,0.2) (0.6,0.5,0.2)(0.7,0.4,0.2)(1,0,0) beaSVNNSM. P ×II P = (1,0,0)(0.3,0.2,0.1)(0.6,0.5,0.2) (0.3,0.2,0.1)(1,0,0)(0.7,0.4,0.2) (0.6,0.5,0.2)(0.7,0.4,0.2)(1,0,0) = P
P satisfies (T, S)-Transitivity,wherewechoose T(a, b)= ab, S(a, b)= a + b ab.Therefore, P isa (T, S)-SVNNEM.When λ =(0.6,0.5,0.2),wehave Pλ = (1,0,0)(0,1,1)(1,0,0) (0,1,1)(1,0,0)(1,0,0) (1,0,0)(1,0,0)(1,0,0) Then P2 λ = Pλ ×II Pλ = (1,0,0)(1,0,0)(1,0,0) (1,0,0)(1,0,0)(1,0,0) (1,0,0)(1,0,0)(1,0,0) (p2 12)λ =(1,0,0) ≤ (p12)λ =(0,1,1).ItshowsthatP2 λ ⊆ Pλ .SoPλ isnota (T, S)-SVNNEM.
BytheideaofYeinliterature[26],wecaneasilyhaveTheorem 7
Theorem7. Let P beaSVNNSM,thenafterafiniteof (T, S)-basedcompositions: P → P2 → → P2k → , thereexistsapositiveintegerksuchthatP2k = P2k+1 .Moreover,P2k isa (T, S)-SVNNEM.
4.AAlgorithmforSingle-ValuedNeutrosophicNumberClustering
Thissectionproposesaclusteringalgorithmbasedon (T, S)-SVNNEMunderasingle-valued neutrosophicenvironment. Let Y = {y1, y2,..., yn } beafinitesetofalternatives,and X = {x1, x2,..., xm } bethesetofattributes. Supposethecharacteristicsoftheobjects yi (i = 1,2,..., n) withrespecttotheattributes xj (j = 1,2,..., m) areexpressedas rij =(tij, iij, fij ).Thedecisionmatrix R =(rij )n×m isaSVNNM.
Step1 Calculatethesimilaritymeasureforeachpairof yi and yk (i, k = 1,2,..., n) by Sik = Sim(rij, rkj )=(Simt (rij, rkj ), Simi (rij, rkj ), Sim f (rij, rkj ))(seeliterature[11]):
Simt (rij, rkj )= 1 ∑m j=1 |trij (xi ) trkj (xi )| ∑m j=1(trij (xi )+trkj (xi )) ;
Simi (rij, rkj )= ∑m j=1 |irij (xi ) irkj (xi )| ∑m j 1(irij (xi )+irkj (xi )) ;
Sim f (rij, rkj )= ∑m j=1 | frij (xi ) frkj (xi )| ∑m j=1( frij (xi )+ frkj (xi )) ,
where xj ∈ X, j = 1,2,..., m.ThenaSVNNSM P =(Sik )n×n isconstructed.
Step2 Chooseappropriate (T, S) dualtriangularmodual.Thenweverifywhether P isa (T, S)-SVNNEMornot.Ifnot,stopcalculatingthe (T, S)-basedcompositions P → P2 → → P2k → until P2k = P2k+1 .Weobtaina (T, S)-SVNNEM P2k
Step3 Takeanappropriateintervalof λ,andcalculate (P2k )λ byDefinition 13.Basedon (P2k )λ , if (pij )λ =(ptj )λ (j = 1,2,..., n),then yi and yt canbedividedintothesamecategory.
5.IllustrativeExampleandComparativeAnalysis
Inthissection,wegiveanexampletoshowtheeffectivenessandrationalityofourproposed method,anddemonstrateitssuperioritybycomparingitwithotherexistingmethods.
5.1.IllustrativeExample
AnexampleisgivenbyadaptingfromZhangetal.(2007).Wewillusethisexampletoillustrate theeffectivenessandrationalityoftheproposed (T, S)-SVNNEMclusteringalgorithm. Consideracarclassificationproblem[19].Therearefivenewcars yi (i = 1,2,3,4,5) tobeclassified. Everycarhassixevaluationattributes:(1) x1:Fueleconomy;(2) x2:Coefficientoffriction;(3) x3:Price; (4) x4:Comfort;(5) x5:Design;(6) x6:Safety.Thecharacteristicsofthefivenewcarsunderthesix attributesarerepresentedbytheformofSVNNs,showninTable 1
Table1. Thecharacteristicsofthefivenewcars. x1 x2 x3 x4 x5 x6 y1 (0.3,0.4,0.5)(0.6,0.7,0.1)(0.4,0.4,0.3)(0.8,0.3,0.1)(0.1,0.2,0.6)(0.5,0.2,0.4) y2 (0.6,0.3,0.3)(0.5,0.1,0.2)(0.6,0.5,0.1)(0.7,0.3,0.1)(0.3,0.2,0.6)(0.4,0.4,0.3) y3 (0.4,0.2,0.4)(0.8,0.3,0.1)(0.5,0.1,0.1)(0.6,0.1,0.2)(0.4,0.3,0.5)(0.3,0.2,0.2) y4 (0.2,0.5,0.4)(0.4,0.3,0.1)(0.9,0.2,0.0)(0.8,0.2,0.1)(0.2,0.1,0.5)(0.7,0.3,0.1) y5 (0.5,0.1,0.2)(0.3,0.4,0.6)(0.6,0.3,0.3)(0.7,0.3,0.1)(0.6,0.4,0.2)(0.5,0.1,0.3)
Step1 Calculatethesimilaritymeasuresforeachpairof yi and yk (i, k = 1,2,3,4,5) by similaritymeasure Simik = Sim(rij, rkj )=(Simt (rij, rkj ), Simi (rij, rkj ), Sim f (rij, rkj )),andthenget theSVNNSM P P
(1,0,0)(0.83,0.25,0.17)(0.81,0.35,0.2)(0.81,0.26,0.25)(0.78,0.26,0.35) (0.83,0.25,0.17)(1,0,0)(0.85,0.4,0.16)(0.8,0.29,0.21)(0.89,0.35,0.33) (0.81,0.35,0.2)(0.85,0.4,0.16)(1,0,0)(0.71,0.29,0.11)(0.81,0.29,0.44) (0.81,0.26,0.25)(0.8,0.29,0.21)(0.71,0.29,0.11)(1,0,0)(0.78,0.38,0.51) (0.78,0.26,0.35)(0.89,0.35,0.33)(0.81,0.29,0.44)(0.78,0.38,0.51)(1,0,0)
Step2 Choose T(a, b)= ab and S(a, b)= a + b ab,thencalculate P2 = P ×II P P2
(1,0,0)(0.83,0.25,0.17)(0.81,0.35,0.2)(0.81,0.26,0.25)(0.78,0.26,0.35) (0.83,0.25,0.17)(1,0,0)(0.85,0.4,0.16)(0.8,0.29,0.21)(0.89,0.35,0.33) (0.81,0.35,0.2)(0.85,0.4,0.16)(1,0,0)(0.71,0.29,0.11)(0.81,0.29,0.4372) (0.81,0.26,0.25)(0.8,0.29,0.21)(0.71,0.29,0.11)(1,0,0)(0.78,0.38,0.4707) (0.78,0.26,0.35)(0.89,0.35,0.33)(0.81,0.29,0.4372)(0.78,0.38,0.4707)(1,0,0)
Obviously, P2 ⊆ P,continuallycalculate P4 = P2 ×II P2 P4
(1,0,0)(0.83,0.25,0.17)(0.81,0.35,0.2)(0.81,0.26,0.25)(0.78,0.26,0.35) (0.83,0.25,0.17)(1,0,0)(0.85,0.4,0.16)(0.8,0.29,0.21)(0.89,0.35,0.33) (0.81,0.35,0.2)(0.85,0.4,0.16)(1,0,0)(0.71,0.29,0.11)(0.81,0.29,0.4372) (0.81,0.26,0.25)(0.8,0.29,0.21)(0.71,0.29,0.11)(1,0,0)(0.78,0.38,0.4707) (0.78,0.26,0.35)(0.89,0.35,0.33)(0.81,0.29,0.4372)(0.78,0.38,0.4707)(1,0,0)
Itisclearthat P4 = P2,thatis, P2 isa (T, S)-SVNNEM.
Step3 Let (0.83,0.25,0.17) < λ ≤ (1,0,0).Wehave (P2)λ =
(1,0,0)(0,1,1)(0,1,1)(0,1,1)(0,1,1) (0,1,1)(1,0,0)(0,1,1)(0,1,1)(0,1,1) (0,1,1)(0,1,1)(1,0,0)(0,1,1)(0,1,1) (0,1,1)(0,1,1)(0,1,1)(1,0,0)(0,1,1) (0,1,1)(0,1,1)(0,1,1)(0,1,1)(1,0,0)
Then yi (i = 1,2,3,4,5) canbedividedintofivecategories: {y1}, {y2}, {y3}, {y4}, {y5} Let (0.71,0.29,0.29) < λ ≤ (0.83,0.25,0.17).Wehave (P2)
(1,0,0)(1,0,0)(0,1,1)(0,1,1)(0,1,1) (1,0,0)(1,0,0)(0,1,1)(0,1,1)(0,1,1) (0,1,1)(0,1,1)(1,0,0)(0,1,1)(0,1,1) (0,1,1)(0,1,1)(0,1,1)(1,0,0)(0,1,1) (0,1,1)(0,1,1)(0,1,1)(0,1,1)(1,0,0)
Then yi (i = 1,2,3,4,5) canbedividedintofourcategories: {y1, y2}, {y3}, {y4}, {y5} Let (0,0.4,0.29) < λ ≤ (0.71,0.29,0.17).Wehave (P2)λ
(1,0,0)(1,0,0)(0,1,1)(0,1,1)(0,1,1) (1,0,0)(1,0,0)(0,1,1)(0,1,1)(0,1,1) (0,1,1)(0,1,1)(1,0,0)(1,0,0)(0,1,1) (0,1,1)(0,1,1)(1,0,0)(1,0,0)(0,1,1) (0,1,1)(0,1,1)(0,1,1)(0,1,1)(1,0,0)
Then yi (i = 1,2,3,4,5) canbedividedintothreecategories: {y1, y2}, {y3, y4}, {y5} Let (0,1,0.4707) < λ ≤ (0.71,0.4,0.29).Wehave (P2)
(1,0,0)(1,0,0)(1,0,0)(1,0,0)(0,1,1) (1,0,0)(1,0,0)(1,0,0)(1,0,0)(0,1,1) (1,0,0)(1,0,0)(1,0,0)(1,0,0)(0,1,1) (1,0,0)(1,0,0)(1,0,0)(1,0,0)(0,1,1) (0,1,1)(0,1,1)(0,1,1)(0,1,1)(1,0,0)
(P2)
When T(a, b)= min(a, b), S(a, b)= max(a, b),theclusteringresultsareasTable 2
Table2. Clusteringresultof (T, S)-SVNNEMoftypeI.
λ
ClassificationResults
(0.89,0.33,0.26) < λ ≤ (1,0,0) {y1}, {y2}, {y3}, {y4}, {y5} (0.85,1,0.29) < λ ≤ (0.89,0.33,0.26) {y2, y5}, {y1}, {y3}, {y4} (0.83,1,1) < λ ≤ (0.85,0.33,0.29) {y2, y3, y5}, {y1}, {y4} (0.81,1,1) < λ ≤ (0.83,0.33,0.29) {y1, y2, y3, y5}, {y4} (0,1,1) ≤ λ ≤ (0.81,0.33,0.29) {y1, y2, y3, y4, y5}
Accordingtotheneutrosophicorthogonalclusteringalgorithmproposedinliterature[28], theclusteringresultsareasTable 3.
Table3. Clusteringalgorithminliterature[28]. λ ClassificationResults
(1,0,0) {y1}, {y2}, {y3}, {y4}, {y5} (0.6,0.6,0.3) {y1, y2}, {y3}, {y4}, {y5} (0.5,0.8,0.4) {y1, y2, y3, y4}, {y5} (0.2,0.8,0.5) {y1, y2, y3, y4, y5}
Tocompare (T, S)-SVNNEMclusteringalgorithmwithintuitionisticfuzzyequivalentmatrix (IFEM)clusteringalgorithminliterature[19],weassumetheindeterminacy-membershipfunction iyj (xi ) isnotconsideredinaSVNNof yj withrespecttotheattributes xi.Thenthisexamplereduces totheexampleinliterature[19].Accordingtothemethodproposedinliterature[19],theclustering resultsareasTable 4.
Table4. Clusteringalgorithminliterature[19].
λ ClassificationResults
0.78 < λ ≤ 1 {y1}, {y2}, {y3}, {y4}, {y5} 0.71 < λ ≤ 0.78 {y1, y2, y3}, {y4}, {y5} 0 ≤ λ ≤ 0.71 {y1, y2, y3, y4, y5}
Tocomparewiththefuzzyequivalentmatrix(FEM)developedinliterature[18],weonlyconsider themembershipdegreeofSVNNinformation.Accordingtothemethodproposedonliterature[18], theclusteringresultsareasTable 5
Table5. Clusteringalgorithminliterature[18]. λ ClassificationResults 0.7 < λ ≤ 1 {y1}, {y2}, {y3}, {y4}, {y5} 0.6 < λ ≤ 0.7 {y1, y2, y3, y5}, {y4} 0 ≤ λ ≤ 0.6 {y1, y2, y3, y4, y5}
4 y5}{y1, y2, y3, y4, y5} 2 {y1 y2 y3 y5} {y4}{y1 y2 y3 y4} {y5}{y1 y2 y3 y4} {y5}{y1 y2 y3 y5} {y4} failed 3 {y2, y3 y5}, {y1} {y4}{y1, y2}, {y3, y4}, {y5} failedfailed {y1, y2, y3}, {y4}, {y5} 4 {y2 y5} {y1} {y3} {y4}{y1 y2} {y3} {y4} {y5}{y1 y2} {y3} {y4} {y5} failedfailed 5 {y1}, {y2}, {y3}, {y4} {y5}{y1}, {y2}, {y3}, {y4}, {y5}{y1}, {y2} {y3}, {y4}, {y5}{y1}, {y2}, {y3}, {y4}, {y5}{y1} {y2}, {y3}, {y4}, {y5}
5.2.AnalysisofComparativeResults
FromTable 6,wecanseethattheclusteringresultsoffiveclusteringmethodsaredifferent. Themainreasoncanbegivenbyfollowingcomparativeanalysis.
(1) Forthe (T, S)-SVNNEMclusteringalgorithm,whenthedualtriangularmodulesoftypeIandII arechosen,theycanbedividedintofiveclassificationswiththesameclassificationabilitybut differentclassificationresults.Thereasonistheminandmaxoperators(typeI)easilyoverlook theinfluenceofotherSVNNinformationonthewhole.
(2) Compareourmethodwithliteratures[18,19], yi (i = 1,2,3,4,5) canonlybedividedinto threeclassificationsbytheFEMclusteringalgorithminliterature[18]andtheIFEMclustering algorithminliterature[19].ThereasonisthatintheclusteringprocessweusetheSVNNM insteadofthefuzzymatrix,whichcanbetterretaininformation.Theclassificationresultsare morereasonableandcomprehensive.
(3) Themethodinliterature[28]doesnotcalculatetheequivalencematrixbasedonthesimilarity matrix,butfromtheclassificationresults, yi (i = 1,2,3,4,5) canonlybedividedintofour classificationsbySVNNorthogonalclusteringalgorithminliterature[28],while yi (i = 1,2,3,4,5) canbedividedintofiveclassificationsbyourclusteringalgorithm.Fortheexample giveninthispaper,itshowsthatthe (T, S)-SVNNEMclusteringalgorithmclassificationresult ismoreaccuratethanSVNNorthogonalclusteringalgorithminliterature[28].
(4) Comparedourmethodwiththeexistingmethods,asthevalueof λ changes,theresult remainsstable.Thatis, λ keepstheclassificationresultwithinacertainrange.Suchas, when yi (i = 1,2,3,4,5) needstobedividedintothreeclassifications,wehave (0,0.4,0.29) < λ ≤ (0.71,0.29,0.17).Theclassificationresultsremainunchangedinthisinterval,whilein literature[18,28]itcannotbedividedintothreeclassifications.
6.Conclusions
Currently,SVNSisageneralizationofFSandIFS.Itismoresuitablefordealingwithuncertainty, imprecise,incompleteandinconsistentinformation.Inaddition,clusteringhasattractedmoreand moreattention.Inthispaper,theconceptsofa (T, S)-basedcompositionmatrixand (T, S)-based single-valuedneutrosophicnumberequivalencematrixhavebeendeveloped.Further,aclustering algorithmhasbeendeveloped.Inordertoillustratetheeffectivenessandsuperiorityofourmethod, acomparisonexamplehasbeengiven.Finally,thecomparativeresultsofanexamplehavebeen analyzedwhichshowsthesuperiorityofourmethod.
AuthorContributions: J.M.conceived,wroteandrevisedthispaper;H.-L.H.providedideasandsuggestionsfor therevisionofthispaper.
Funding: ThisresearchwasfundedbyNationalNaturalScienceFoundationProject,grantnumber(11701089); FujianNaturalScienceFoundation,grantnumber(2018J01422);ScientificResearchProjectofMinnanNormal University,grantnumber(MK201715).
Acknowledgments: ThispaperissupportedbyInstituteofMeteorologicalBigData-DigitalFujianandFujian KeyLaboratoryofDataScienceandStatistics.
ConflictsofInterest: Theauthorsdeclarenoconflictofinterest.
References
1. Zadeh,L.A.Fuzzysets. Inf.Control 1965, 8,338–356.[CrossRef]
2. Atanassov,K.Intuitionisticfuzzysets. FuzzySetsSyst. 1986, 20,87–96.[CrossRef]
3. Atanassov,K.;Gargov,G.Interval-valuedintuitionisticfuzzysets. FuzzySetsSyst. 1989, 31,343–349. [CrossRef]
4. Smarandache,F. AUnifyingFieldinLogics:NeutrosophyLogic;AmericanResearchPress:Rehoboth,DE,USA, 1999;pp.1–141.
5. Wang,H.;Smarandache,F.;Zhang,Y.Q.;Sunderraman,R.Singlevaluedneutrosophicsets. Multisp.Multistruct. 2010, 4,410–413.
6. Ngan,R.T.;Ali,M.;Le,H.S. δ-equalityofintuitionisticfuzzysets:anewproximitymeasureandapplications inmedicaldiagnosis. Appl.Intell. 2017, 48,1–27.[CrossRef]
7. Luo,M.;Zhao,R.Adistancemeasurebetweenintuitionisticfuzzysetsanditsapplicationinmedical diagnosis. Artif.Intell.Med. 2018, 89,34–39.[CrossRef][PubMed]
8. Huang,H.L.Newdistancemeasureofsingle-valuedneutrosophicsetsanditsapplication. Int.J.Intell.Syst. 2016, 31,1021–1032.[CrossRef]
9. Mo,J.M.;Huang,H.L.DualgeneralizednonnegativenormalneutrosophicBonferronimeanoperatorsand theirapplicationinmultipleattributedecisionmaking. Information 2018, 9,201.[CrossRef]
10. Ye,J.Intuitionisticfuzzyhybridarithmeticandgeometricaggregationoperatorsforthedecision-makingof mechanicaldesignschemes. Appl.Intell. 2017, 47,1–9.[CrossRef]
11. Broumi,S.;Smarandache,F.Severalsimilaritymeasuresofneutrosophicsets. NeutrosophicSetsSyst. 2013, 1,54–62.
12. Garg,H.;Rani,D.Arobustcorrelationcoefficientmeasureofcomplexintuitionisticfuzzysetsandtheir applicationsindecision-making. Appl.Intell. 2018.[CrossRef]
13. Kumar,S.V.A.;Harish,B.S.Amodifiedintuitionisticfuzzyclusteringalgorithmformedicalimage segmentation. J.Intell.Syst. 2017.[CrossRef]
14. Li,Q.Y.;Ma,Y.C.;Smarandache,F.;Zhu,S.W.Single-valuedneutrosophicclusteringalgorithmbasedon Tsallisentropymaximization. Axioms 2018, 7,57.[CrossRef]
15. Verma,H.;Agrawal,R.K.;Sharan,A.Animprovedintuitionisticfuzzyc-meansclusteringalgorithm incorporatinglocalinformationforbrainimagesegmentation. Appl.SoftComput. 2016, 46,543–557. [CrossRef]
16. Qureshi,M.N.;Ahamad,M.V.Animprovedmethodforimagesegmentationusingk-meansclusteringwith neutrosophiclogic. ProcediaComput.Sci. 2018, 132,534–540.[CrossRef]
17. Seising,R.Theemergenceoffuzzysetsinthedecadeoftheperceptron-lotfiA.Zadeh’sandFrank Rosenblatt’sresearchworkonpatternclassification. Mathematics 2018, 6,110.[CrossRef]
18. Chen,S.L.;Li,J.G.;Wang,X.G. FuzzySetTheoryandItsApplication;SciencePress:Beijing,China,2005; pp.94–113.
19. Zhang,H.M.;Xu,Z.S.;Chen,Q.Clusteringapproachtointuitionisticfuzzysets. ControlDecis. 2007, 22,882–888.
20. Guan,C.;Yuen,K.K.F.;Coenen,F.Towardsanintuitionisticfuzzyagglomerativehierarchicalclustering algorithmformusicrecommendationinfolksonomy.InProceedingsoftheIEEEInternationalConference onSystems,Kowloon,China,9–12October2016;pp.2039–2042.
21. Kuo,R.J.;Lin,T.C.;Zulvia,F.E.;Tsai,C.Y.Ahybridmetaheuristicandkernelintuitionisticfuzzyc-means algorithmforclusteranalysis. Appl.SoftComput. 2018, 67,299–308.[CrossRef]
22. Xu,Z.S.;Tang,J.;Liu,S.AnorthogonalalgorithmforclusteringiIntuitionisticfuzzyinformation. Int.J.Inf. 2011, 14,65–78.
23. Zhao,H.;Xu,Z.S.;Liu,S.S.;Wang,Z.IntuitionisticfuzzyMSTclusteringalgorithms. Comput.Ind.Eng. 2012, 62,1130–1140.[CrossRef]
24. Sahu,M.;Gupta,A.;Mehra,A.Hierarchicalclusteringofinterval-valuedintuitionisticfuzzyrelationsand itsapplicationtoelicitcriteriaweightsinMCDMproblems. Opsearch 2017, 54,1–29.[CrossRef]
25. Zhang,H.W.C-meansclusteringalgorithmbasedoninterval-valuedintuitionisticfuzzysets. Comput.Appl.Softw. 2011, 28,122–124.
26. Ye,J.Single-valuedneutrosophicclusteringalgorithmsbasedonsimilaritymeasures. J.Classif. 2017, 34,1–15. [CrossRef]
27. Guo,Y.;Xia,R.;¸Sengür,A.;Polat,K.Anovelimagesegmentationapproachbasedonneutrosophicc-means clusteringandindeterminacyfiltering. NeuralComput.Appl. 2017, 28,3009–3019.[CrossRef]
28. Ali,M.;Le,H.S.;Khan,M.;Tung,N.T.Segmentationofdentalx-rayimagesinmedicalimagingusing neutrosophicorthogonalmatrices. ExpertSyst.Appl. 2018, 91,434–441.[CrossRef]
29. Ye,J.Single-valuedneutrosophicminimumspanningtreeanditsclusteringmethod. J.Intell.Syst. 2014, 23,311–324.[CrossRef]
30. Klir,G.;Yuan,B. FuzzySetsandFuzzyLogic:TheoryandApplication;PrenticeHall:UpperSaddleRiver,NJ, USA,1995. 31. Chen,D.G. FuzzyRoughSetTheoryandMethod;SciencePress:Beijing,China,2013. 32. Smarandache,F.;Vl˘ad˘areanu,L.Applicationsofneutrosophiclogictorobotics:Anintroduction. InProceedingsoftheIEEEInternationalConferenceonGranularComputing,Kaohsiung,Taiwan,8–10 November2012;pp.607–612. 33. Wang,W.Z.;Liu,X.W.Someoperationsoveratanassov’sintuitionisticfuzzysetsbasedonEinsteint-norm andt-conorm. Int.J.Uncertain.FuzzinessKnowl.-BasedSyst. 2013, 21,263–276.[CrossRef] 34. Xia,M.M.;Xu,Z.S.;Zhu,B.SomeissuesonintuitionisticfuzzyaggregationoperatorsbasedonArchimedean t-conormandt-norm. Knowl.-BasedSyst. 2012, 31,78–88.[CrossRef] 35. Garg,H.GeneralizedintuitionisticfuzzyinteractivegeometricinteractionoperatorsusingEinsteint-norm andt-conormandtheirapplicationtodecisionmaking. Comput.Ind.Eng. 2016, 101,53–69.[CrossRef]
36. Liu,P.D.;Chen,S.M.Heronianaggregationoperatorsofintuitionisticfuzzynumbersbasedonthe Archimedeant-normandt-conorm. Int.Conf.Mach.Learn.Cybern. 2017.[CrossRef]
37. Liu,P.D.TheaggregationoperatorsbasedonArchimedeant-conormandt-normforsingle-valued neutrosophicnumbersandtheirapplicationtodecisionmaking. Int.J.FuzzySyst. 2016, 18,1–15.[CrossRef]
38. Huang,H.L.(T,S)-basedinterval-valuedintuitionisticfuzzycompositionmatrixanditsapplicationfor clustering. Iran.J.FuzzySyst. 2012, 9,7–19.
c 2019bytheauthors.LicenseeMDPI,Basel,Switzerland.Thisarticleisanopenaccess articledistributedunderthetermsandconditionsoftheCreativeCommonsAttribution (CCBY)license(http://creativecommons.org/licenses/by/4.0/).
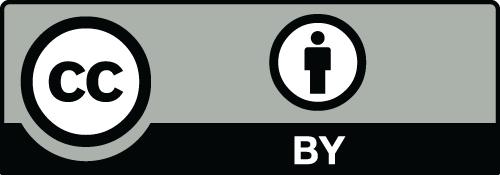