See discussions, stats, and author profiles for this publication at: https://www researchgate net/publication/311931059
VECTOR SIMILARITY MEASURES FOR SIMPLIFIED NEUTROSOPHIC HESITANT FUZZY
SET AND THEIR...
Article December 2016
CITATIONS 0 READS 126
3 authors:
Qaisar Khan
International Islamic University, Islamabad 11 PUBLICATIONS 1 CITATION
SEE PROFILE
Jun Ye Shaoxing University
129 PUBLICATIONS 2,572 CITATIONS
SEE PROFILE
Tahir Mahmood
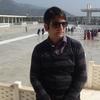
International Islamic University, Islamabad 39 PUBLICATIONS 73 CITATIONS
SEE PROFILE
Some of the authors of this publication are also working on these related projects:
The project of neutrosophic theory, decision making, and applications sponsored by the National Natural Science Foundation of P.R. China (No. 71471172). View project
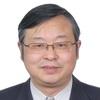
All content following this page was uploaded by Tahir Mahmood on 28 December 2016.
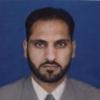
The user has requested enhancement of the downloaded file
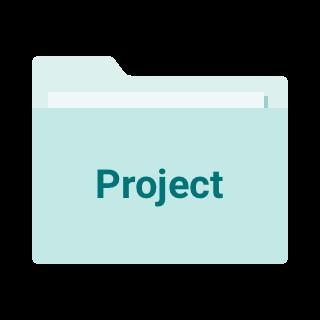
JournalofInequalitiesandSpecialFunctions
ISSN:2217-4303,URL:http://ilirias.com/jiasf Volume7Issue4(2016),Pages176-194.
VECTORSIMILARITYMEASURESFORSIMPLIFIED NEUTROSOPHICHESITANTFUZZYSETANDTHEIR APPLICATIONS
TAHIRMAHMOOD,JUNYEANDQAISARKHAN
Abstract. Inthisarticlewepresentthreesimilaritymeasuresbetweensimplifiedneutrosophichesitantfuzzysets,whichcontaintheconceptofsingle valuedneutrosophichesitantfuzzysetsandintervalvaluedneutrosophichesitantfuzzysets,basedontheextensionofJaccardsimilaritymeasure,Dice similaritymeasureandCosinesimilarityinthevectorspace.Thenbasedon thesethreedefinedsimilaritymeasureswepresentamultipleattributedecision makingmethodtosolvethesimplifiedneutrosophichesitantfuzzymultiplecriteriadecisionmakingproblem,inwhichtheevaluatedvaluesofthealternative withrespecttothecriteriaisrepresentedbysimplifiedneutrosophichesitant fuzzyelements.Furtherweappliedtheproposedsimilaritymeasurestopatternrecognition.Attheendanumericalexamplesarediscussedtoshowthe effectivenessoftheproposedsimilaritymeasures.
1. Introduction
Theevidenceprovidedbypeopletoexamineissuesisfrequentlyfuzzyandcrisp, andthefuzzyset(FS)hasbeenverifiedtobeveryimportantinmulti-criteria decisionmaking. FS theorywasfirstpresentedbyL.A.Zadehin1965[49]seeing degreesofmembershiptoprogressivelyassesthemembershipofelementsinaset. Aftertheintroductionof FS,atanassov[1]presentedtheconceptofintuitionistic fuzzyset(IFS)byconsideringmembershipandnon-membershipdegreesofan elementtotheset.Atanassovetal.extended IFS tointervalvaluedintuitionistic fuzzyset(IVIFS)[2].Aftertheintroductionof IFS alotofsimilaritymeasures areproposedbymanyresearchersastheextensionofthesimilaritymeasuresfor FS,asasimilaritymeasureisanimportanttoolfordeterminingsimilaritybetween objects.Liandcheng[20]presentedsimilaritymeasurefor IFS whichisthefirstto appliedthemtopatternrecognition.S.KDeetal.gavesomeapplicationof IFS tomedicaldaignosis[19].Ye[36]presentedcosinesimilaritymeasurefor IFS and appliedittomedicaldiagnosisandpatternrecognition.Ye[37,38]alsopresented
2010 MathematicsSubjectClassification. 90B50,91B06,91B10,62C99.
Keywordsandphrases. Vectorsimilaritymeasure;Jaccardsimilaritymeasure;Dicesimilarity measure;Cosinesimilaritymeasure;hesitantfuzzysets;intervalvaluedhesitantfuzzysets;Single valuedneutrosophichesitantfuzzysets;intervalneutrosophichesitantfuzzysets;multi-criteria decisionmaking;patternrecognition.
c 2016UniversitetiiPrishtin¨es,Prishtin¨e,Kosov¨e. SubmittedMay1,2016.PublishedNovember17,2016.
cosinesimilaritymeasureandvectorsimilaritymeasuresfor IVIFS andtrapezoidal IFN andappliedthemtomultiplecriteriadecisionmakingproblem. Neutrosophicset( NS)wasfirstpresentedbyF.Smarandache[25,26]which simplifiestheconceptofcrispset, FS, IFS, IVIFS,andsoon.In NS itsindeterminacyiscomputedunambiguouslyanditstruth-membership,indeterminacymembershipandfalsity-membershiparesignifiedfreely. NS simplifiesalltheabove definedsetsfromphilosophicalpointofview.Severalsimilaritymeasuresfor NS hasbeendefinedbyBroumi.S.etal.[3].Broumi.S.etal.[6,7,8,9,10,11] singlevaluedneutrosophicgraph,BipolarSingleValuedNeutrosophicGraphs, IsolatedSingleValuedNeutrosophicGraphs,BipolarSingleValuedNeutrosophic GraphTheory,SingleValuedNeutrosophicGraphs:Degree,OrderandSize,and AnIntroductiontoBipolarSingleValuedNeutrosophicGraphTheory.Majumdar etal.[21]presentedsimilarityandentropyfor NS. NS isdifficulttoapplyinreallife andengineeringproblem.Theconceptofsinglevaluedneutrosophicsets SVNSs wasintroducedforthefirsttimebyF.Smarandachein1998[25].Wangetal. [30,31]introducedtheconceptofintervalneutrosophicsets( INSs)andprovided basicoperationandpropertiesof SVNSs and INSs.The SVNSs and INSs isasubclassof NS.SahinR.etal.[24]definedsubsethoodfor SVNSs.Zhang etal[50]alsodefinedsomeoperationfor INS.Ye[39,40,41,42]definedcorrelationcoefficient,improvedcorrelation,entropyandsimilaritymeasurefor SVNS and INS andgavetheirapplicationinmultipleattributedecisionmakingproblems(MCDM ).Broumi.S.etal.[4,5]presentedcorrelationandcosinesimilarity measurefor INS.Ye[43]presentedtheconceptofsimplifiedneutrosophicsets( SNSs)whichcontainedtheconceptof SVNS and INS anddefinedsomebasic operationfor SNSs andappliedthemtomulti-criteriadecisionmakingproblem. Ye[44,45]alsodefinedvectorsimilaritymeasuresfor SNSs andimprovedcosine similaritymeasuresfor SNSs.Pengetal[18]findsomedrawbacksoftheoperation for SNS definedbyYeandpresentednewoperationsfor SNS.
Hesitantfuzzyset( HFS)isanothergeneralizationof FS proposedbyTorra andNarukawa[28,29].Theadvantegeof HFS isthatitauthorizationsthemembershipdegreeofanelementtoagivensetwithalimiteddifferentvalues,which canascendinagroupdecisionmakingproblem.Recentlya HFS hasacknowledgedmoreandmoreconsiderationsinceitspresence.XiaandXu[32,33,34,35] presentedhesitantfuzzyinformation,similaritymeasuresfor HFS andcorrelation for HFS.Chenetal[13]presentedsomecorrelationcoefficientfor HFS andappliedthemtoclusteringanalysis.Ye[46]presentedvectorsimilaritymeasuresfor HFS.Chenetal.[12]furtherextendedHFStointervalvaluedhesitantfuzzyset. ( IVHFS)Farhadinia.B[15,16]definedinformationmeasuresfor HFS,and IVHFS. anddistancemeasuresforhighorder HFS.
Dualhesitantfuzzysets(DHFS)waspresentedbyZhu[51,52]whichisthe generalizationof HFS, FS, IFS andfuzzymultisetsarespecialcasesof DHFS RecentlySu.Z[27]presentedsimilaritymeasurefor DHFSs andapplieditto
patternrecognition.Correlationof DHFS waspresentedbyYe[47]andapplied themto MCDM under DHF information.Asmentionedabovethathesitancy isthemostcommonproblemindecisionmakingforwhich HFS isasuitable meansbyallowingseveralpossiblevaluesforanelementtoaset.Howeverin HFS theyconsideronlyonetruth-membershipfunctionanditcannotexpressthis problemwithafewdifferentvaluesassignedbytruth-membershiphesitantdegree, indeterminacy-membershipdegree,andfalsity-membershipdegreesduetodoubtsof decisionmakers.andalsoin DHFS theyconsidertwofunctionsthatismembership andnon-membershipfunctionsandcannotconsiderindeterminacy-membership function.TooverawedthisproblemandthedecisionmakercangetmoreinformationYe[48]presentedtheconceptofsinglevaluedneutrosophichesitantfuzzysets (SVNHF )anddefinedsomebasicoperations,aggregationoperatorsandapplied itto MCDM under SVNHF .environment.LuiP.[22]presentedtheconceptofintervalneutrosophichesitantfuzzyset INHF anddefinedsomebasicoperations, aggregationoperatorsandappliedthemtomulticriteriadecisionmakingproblem.
Sincevectorsimilaritymeasuresplayedanimportantroleindecisionmaking,so wepresentvectorsimilaritymeasuresfor SNHF andthengiveitsapplicationsin MCDM andpatternrecognition.
Therestofthearticleisorganizedasfollows.Insection2wedefinedsomebasic definitionsrelatedtoourwork.Insection3wedefinedvectorsimilaritymeasures forsimplifiedneutrosophichesitantfuzzysets.Insection4weapplytheproposed similaritymeasurestomultiattributedecisionmakingproblemundersimplified neutrosophicenvironment.insection5weapplytheproposedsimilaritymeasures topatternrecognition.Attheendwegivesomenumericalexamplestoshowthe effectivenessoftheproposedsimilaritymeasures,conclusionandreferencesare given.
2. Preliminaries
Inthissectionwedefinesomebasicdefinitionsabouthesitantfuzzysets,interval valuedhesitantfuzzyset,singlevaluedneutrosophichesitantfuzzyset,interval neutrosophichesitantfuzzysetsandthevectorsimilaritymeasures,suchasJaccard similaritymeasure,Dicesimilaritymeasure,Cosinesimilaritymeasure.
Definition2.1. [28,29]Let U beafixedset,ahesitantfuzzyset ˆ M on U isdefined intermsofafunction f ˆ M (a) thatwhenappliedto U returnsafinitesubsetsof [0, 1]. Hesitantfuzzysetismathematicallyrepresentedas, ˆ M = { a,fM (a)|a ∈ U },
Where fM (a)isasetofsomedifferentvaluesin[0, 1], denotingthepossible membershipdegreesoftheelement a ∈ U to ˆ M.
Definition2.2. [12]Let U beafixedset,anintervalvaluedhesitantfuzzyset ˆ D on U isdefinedintermsofafunction f ˆ M (a) thatwhenappliedto U returnsafinite setofsubintervalsof [0, 1]. Anintervalvaluedhesitantfuzzysetismathematically representedas,
ˆ D = { a,fD(a)|a ∈ U },
Where fD(a)isasetofsomedifferentvaluesin[0, 1], denotingthepossible membershipdegreesoftheelement a ∈ U to ˆ D.
Definition2.3. [48]Let U beanonemptyfixedset.Asinglevaluedneutrosophic hesitantfuzzyset ˆ N isthestructureoftheform: ˆ N = { a, ˘ t(a), ˘ ı(a), ˘ f (a) } Inwhich ˘ t(a), ˘ ı(a)and ˘ f (a)arethreesetsofsomevaluesintherealunitinterval [0, 1], representingthepossibletruth-membershiphesitantdegrees,indeterminacymembershiphesitantdegreesandfalsity-membershiphesitantdegreeoftheelement a ∈ U totheset ˆ N, respectively,withtheconditionthat0 ≤ ϕ,χ,ψ ≤ 1and 0 ≤ ϕ+ +χ+ +ψ+ ≤ 3, where ϕ ∈ ˘ t(a),χ ∈ ˘ ı(a),ψ ∈ ˘ f (a),ϕ+ = ∪ϕ∈ ˘ t max{ϕ},χ+ = ∪ χ∈i max{χ},ψ+ = ∪ψ∈f max{ψ}.
Definition2.4. [22]Let U beanonemptyfixedset.Aintervalneutrosophichesitantfuzzyset ˆ H isstructureoftheform ˆ H = { a, ˘ t(a), ˘ ı(a), ˘ f (a) }
Inwhich ˘ t(a), ˘ ı(a)and ˘ f (a)arethreesetsofsomeintervalvaluesinthesetof realunitinterval[0, 1], representingthepossibletruth-membershiphesitantdegrees, indeterminacy-membershiphesitantdegreesandfalsity-membershiphesitantdegree oftheelement a ∈ U totheset ˆ H, respectively,withtheconditionthat ϕ = [ϕl,ϕu] ∈ ˘ t(a),χ =[χl,χu] ∈ ˘ ı(a),ψ =[ψl,ψu] ∈ ˘ f (a) ⊆ [0, 1]and0 ≤ sup ϕ+ + sup χ+ +sup ψ+ ≤ 3,ϕ+ = ∪ϕ∈ ˘ t max{ϕ},χ+ = ∪ χ∈i max{χ},ψ+ = ∪ψ∈f max{ψ}.
2.1. Vectorsimilaritymeasures. Let A =(c1,c2,...,cm) andB =(e1,e2,...,em) betwovectorsoflengthis m whereallthecoordinatesarepositive.Thejaccard similaritymeasureofthesetwovectors[17]isgivenby ˜ J(A,B)= A.B ||A||2 2 + ||B||2 2 A.B =
m k=1 ckek m k=1 c2 k + m k=1 e2 k m k=1 ckek (2.1)
Where A.B = m k=1 ckek istheinnerproductofthevectors A and B and ||A||2 = m k=1 c2 and ||B||2 = m k=1 e2 aretheEuclideannormsof A and B.
ThentheDicesimilaritymeasure[14]isdefinedasfollows: D(A,B)= 2A.B ||A||2 2 + ||B||2 2 = 2 m k=1 ckek m k=1 c2 k + m k=1 e2 k (2.2)
andtheCosinesimilaritymeasure[23]isdefinedasfollows:
C(A,B)= A.B ||A||2 ||B||2 =
m k=1 ckek m k=1 c2 k m k=1 e2 k
(2.3)
TheCosinesimilaritymeasureisnothingjustthecosineanglebetweentwo vectors.
theaboveformulasaresameinthesenseeachtakethevalueintheunitinterval [0, 1] Thejaccardanddiceandcosinesimilaritymeasuresarerespectivelyundefined if ck = ek =0,fork =1, 2...mandck =0 orek =0,k =1, 2...m. Nowweassume thatthecosinesimilaritymeasureequaltozerowhen ck =0 orek =0,k =1, 2...m. Thesevectorsimilaritymeasuresatisfythefollowingpropertiesforthevectors AandB.
(1) J(A,B)= J(B,A),D(A,B)= D(B,A),andC(A,B)= C(B,A) (2)0 ≤ J(A,B),D(A,B),C(A,B) ≤ 1
(3) J(A,B)= D(A,B)= C(A,B)=1 ifA = B
3. VectorSimilarityMeasureForSimplifiedNeutrosophichesitant fuzzySets
Ifthesimplifiedneutrosophichesitantfuzzysetcontainsfinitesetofsinglepoints, thatissinglevaluedneutrosophichesitantfuzzyset.ThentheJaccard,Diceand Cosinesimilaritymeasuresaredefinedasfollows: Definition3.1. Let A and B betwo SVNHFSs ontheuniversalset U = {u1,u2,...,um}, respectivelydenotedby A = { uj ,τA(uj ),ιA(uj ),κA(uj )|uj ∈ U } and B = { uj ,τB (uj ),ιB (uj ),κB (uj )|uj ∈ U } forall j =1, 2,...,m.Thenwedefinethejaccard,Diceandcosinesimilaritymeasuresfor SVNHFSs A and B asfollows: JSVNHFS (A, B)= 1 m
m j=1
lej i=1 τAσ(i)(˜ uj )¯ τBσ(i)(˜ uj )+ lej i=1 ιAσ(i)(˜ uj )¯ ιBσ(i)(¯ uj ) + lej i=1 κAσ(i)(˜ uj )¯ κBσ(i)(˜ uj ) lej i=1 τAσ(i)(˜ uj ) 2 + lej i=1 ιAσ(i)(˜ uj ) 2 + lej i=1 κAσ(i)(˜ uj ) 2 + lej i=1 τBσ(i)(˜ uj ) 2 + lej i=1 ιBσ(i)(˜ uj ) 2 + lej i=1 κBσ(i)(˜ uj ) 2 lej i=1 τAσ(i)(˜ uj )¯ τBσ(i)(˜ uj )+ lej i=1 ιAσ(i)(˜ uj )¯ ιBσ(i)(˜ uj )+ lej i=1 κAσ(i)(˜ uj )¯ κBσ(i)(˜ uj )
DSVNHFS (A, B)= 1 m
m j=1
CSVNHFS (A, B)= 1 m
m j=1
lej i=1 τAσ(i)(˜ uj ) 2 + lej i=1 ιAσ(i)(˜ uj ) 2 + lej i=1 κAσ(i)(˜ uj ) 2 + lej i=1 τBσ(i)(˜ uj ) 2 + lej i=1 ιBσ(i)(˜ uj ) 2 + lej i=1 κBσ(i)(˜ uj ) 2 (2)
2 lej i=1 τAσ(i)(˜ uj )¯ τBσ(i)(˜ uj )+ lej i=1 ιAσ(i)(˜ uj )¯ ιBσ(i)(˜ uj ) + lej i=1 κAσ(i)(˜ uj )¯ κBσ(i)(˜ uj )
lej i=1 τAσ(i)(˜ uj )¯ τBσ(i)(˜ uj )+ lej i=1 ιAσ(i)(˜ uj )¯ ιBσ(i)(˜ uj )+ lej i=1 κAσ(i)(˜ uj )¯ κBσ(i)(˜ uj ) lej i=1 τAσ(i)(˜ uj ) 2 + lej i=1 ιAσ(i)(˜ uj ) 2 + lej i=1 κAσ(i)(˜ uj ) 2 + lej i=1 τAσ(i)(˜ uj ) 2 + lej i=1 ιAσ(i)(˜ uj ) 2 + lej i=1 κAσ(i)(˜ uj ) 2 (3)
Here lej =max(le(¯ τA(˜ uj ), ιA(˜ uj ), κA(˜ uj )),le(¯ τB (˜ uj ), ιB (˜ uj ), κB (˜ uj )))forall˜ uj ∈ U, where le(¯ τA(˜ uj ), ιA(˜ uj ), κA(˜ uj ))and le(¯ τB (˜ uj ), ιB (˜ uj ), κB (˜ uj )),respectivelyrepresentsthenumberofvaluesin¯ τA(˜ uj ), ιA(˜ uj ), κA(˜ uj )and¯ τB (˜ uj ), ιB (˜ uj ), κB (˜ uj ) Whenthenumberofvaluesin¯ τA(˜ uj ), ιA(˜ uj ), κA(˜ uj )and¯ τB (˜ uj ), ιB (˜ uj ), κB (˜ uj ) are notequalthatis le(¯ τA(˜ uj ), ιA(˜ uj ), κA(˜ uj )) = le(¯ τB (˜ uj ), ιB (˜ uj ), κB (˜ uj )). Thenthe pessimisticaddtheminimumvaluewhiletheoptimisticaddthemaximumvalue. Thisdependonthedecisionmakersthatwassuccessfullyappliedforhesitantfuzzy setsby[34].
Theabovedefinedthreevectorsimilaritymeasuressatisfytheconditiondefined inYe[46].
(A1) JSVNHFS (A, B)= JSVNHFS (B, A), DSVNHFS (A, B)= DSVNHFS (B, A), CSVNHFS (A, B)= CSVNHFS (B, A)
(A2)0 ≤ JSVNHFS (A, B), DSVNHFS (A, B), CSVNHFS (A, B) ≤ 1
(A3) JSVNHFS (A, B)= DSVNHFS (A, B)= CSVNHFS (A, B)=< 1, 0, 0 > iff A = B
Proof. (A1)obviouslyitistrue.
(A2)obviouslythepropertyistrueduetotheinequality˜ x2 +˜ y2 ≥ 2˜xy foreq1 andeq2andthecosinevalueforeq3.
(A3)When A = B then¯ τA(˜ uj )=¯ τB (˜ uj ), ιA(˜ uj )=¯ ιB (˜ uj )and¯ κA(˜ uj )=¯ κB (˜ uj ) foreach˜ uj ∈ U,j =1, 2,...,m. Sothereare JSVNHFS (A, B)=1, DSVNHFS (A, B)=1, and CSVNHFS (A, B)=1 Inreallifeproblem,theelements˜ uj (j =1, 2,...,m)inauniversalset U = {˜ u1, ˜ u2,..., ˜ uj } havedifferentweights.Let =( 1, 2,..., j )T betheweightvectorof˜ uj (j =1, 2,...,m)with j ≥ 0,j =1, 2,...,m,and m j=1 j =1. Thereforewe
extendtheabovethreesimilaritymeasurestoweightedvectorsimilaritymeasures for SVNHFSs.
TheweightedJaccardsimilaritymeasurefor SVNHFSs A and B asfollows: JSVNHFS (A, B)= m j=1 j
lej i=1 τAσ(i)(˜ uj )¯ τBσ(i)(˜ uj )+ lej i=1 ιAσ(i)(˜ uj )¯ ιBσ(i)(˜ uj )+ lej i=1 κAσ(i)(˜ uj )¯ κBσ(i)(˜ uj ) lej i=1 τAσ(i)(˜ uj ) 2 + lej i=1 ιAσ(i)(˜ uj ) 2 + lej i=1 κAσ(i)(˜ uj ) 2 + lej i=1 τBσ(i)(˜ uj ) 2 + lej i=1 ιBσ(i)(˜ uj ) 2 + lej i=1 κBσ(i)(˜ uj ) 2 lej i=1 τAσ(i)(˜ uj )¯ τBσ(i)(˜ uj )+ lej i=1 ιAσ(i)(˜ uj )¯ ιBσ(i)(˜ uj )+ lej i=1 κAσ(i)(˜ uj )¯ κBσ(i)(˜ uj ) (4)
TheDicesimilaritymeasurefor SVNHFSs A and B asfollows:
DSVNHFS (A, B)= m j=1 j
2 lej i=1 τAσ(i)(˜ uj )¯ τBσ(i)(˜ uj )+ lej i=1 ιAσ(i)(˜ uj )¯ ιBσ(i)(˜ uj ) + lej i=1 κAσ(i)(˜ uj )¯ κBσ(i)(˜ uj )
lej i=1 τAσ(i)(˜ uj ) 2 + lej i=1 ιAσ(i)(˜ uj ) 2 + lej i=1 κAσ(i)(˜ uj ) 2 + lej i=1 τBσ(i)(˜ uj ) 2 + lej i=1 ιBσ(i)(˜ uj ) 2 + lej i=1 κBσ(i)(˜ uj ) 2 (5)
TheCosinesimilaritymeasurefor SVNHFSs A and B asfollows:
lej i=1 τAσ(i)(˜ uj )¯ τBσ(i)(˜ uj )+ lej i=1 ιAσ(i)(˜ uj )¯ ιBσ(i)(˜ uj )+ lej i=1 κAσ(i)(˜ uj )¯ κBσ(i)(˜ uj ) lej i=1 τAσ(i)(˜ uj ) 2 + lej i=1 ιAσ(i)(˜ uj ) 2 + lej i=1 κAσ(i)(˜ uj ) 2 lej i=1 τAσ(i)(˜ uj ) 2 + lej i=1 ιAσ(i)(˜ uj ) 2 + lej i=1 κAσ(i)(˜ uj ) 2 (6) Ifthe SNHFS containasetofintervalvaluesinsteadofasetofsinglepoints. Then
CSVNHFS (A, B)= m j=1 j
m j=1
lej i=1 τ L Aσ(i)(˜ uj )¯ τ L Bσ(i)(˜ uj )+ lej i=1 τ U Aσ(i)(˜ uj )¯ τ U Bσ(i)(˜ uj ) + lej i=1 ιL Aσ(i)(˜ uj )¯ ιL Bσ(i)(˜ uj )+ lej i=1 ιU Aσ(i)(˜ uj )¯ ιU Bσ(i)(˜ uj )+ lej i=1 κL Aσ(i)(˜ uj )¯ κL Bσ(i)(˜ uj )+ lej i=1 κU Aσ(i)(˜ uj )¯ κU Bσ(i)(˜ uj ) lej i=1 τ L Aσ(i)(˜ uj ) 2 + lej i=1 τ U Aσ(i)(˜ uj ) 2 + lej i=1 ιL Aσ(i)(˜ uj ) 2 + lej i=1 ιL Aσ(i)(˜ uj ) 2 + lej i=1 κL Aσ(i)(˜ uj ) 2 + lej i=1 κU Aσ(i)(˜ uj ) 2 + lej i=1 τ L Bσ(i)(˜ uj ) 2 + lej i=1 τ U Bσ(i)(˜ uj ) 2 + lej i=1 ιL Bσ(i)(˜ uj ) 2 + lej i=1 ιU Bσ(i)(˜ uj ) 2 + lej i=1 κL Bσ(i)(˜ uj ) 2 + lej i=1 κU Bσ(i)(˜ uj ) 2
DSVNHFS (A, B 2
le
j i
+
+
lej i=1 τ L Aσ(i)(˜ uj )¯ τ L Bσ(i)(˜ uj )+ lej i=1 τ U Aσ(i)(˜ uj )¯ τ U Bσ(i)(˜ uj ) + lej i=1 ιL Aσ(i)(˜ uj )¯ ιL Bσ(i)(˜ uj )+ lej i=1 ιU Aσ(i)(˜ uj )¯ ιU Bσ(i)(˜ uj )+ lej i=1 κL Aσ(i)(˜ uj )¯ κL Bσ(i)(˜ uj )+ lej i=1 κU Aσ(i)(˜ uj )¯ κU Bσ(i)(˜ uj ) )= 1 4m
(7) Thedicesimilaritymeasurefor INHFSs m j=1
lej i=1 τ L Aσ(i)(˜ uj ) 2 + lej i=1 τ U Aσ(i)(˜ uj ) 2 + lej i=1 ιL Aσ(i)(˜ uj ) 2 + lej i=1 ιL Aσ(i)(˜ uj ) 2 + lej i=1 κL Aσ(i)(˜ uj ) 2 + lej i=1 κU Aσ(i)(˜ uj ) 2 + lej i=1 τ L Bσ(i)(˜ uj ) 2 + lej i=1 τ U Bσ(i)(˜ uj ) 2 + lej i=1 ιL Bσ(i)(˜ uj ) 2 + lej i=1 ιU Bσ(i)(˜ uj ) 2 + lej i=1 κL Bσ(i)(uj ) 2 + lej i=1 κU Bσ(i)(uj ) 2 (8)
κ
Thecosinesimilaritymeasurefor INHFSs. CSVNHFS (A, B)= 1 2m
m j=1
lej i=1 τ L Aσ(i)(˜ uj )¯ τ L Bσ(i)(˜ uj )+ lej i=1 τ U Aσ(i)(˜ uj )¯ τ U Bσ(i)(˜ uj )+ lej i=1 ιL Aσ(i)(˜ uj )¯ ιL Bσ(i)(˜ uj )+ lej i=1 ιU Aσ(i)(˜ uj )¯ ιU Bσ(i)(˜ uj )+ lej i=1 κL Aσ(i)(˜ uj )¯ κL Bσ(i)(˜ uj )+ lej i=1 κU Aσ(i)(˜ uj )¯ κU Bσ(i)(˜ uj ) lej i=1 τ L Aσ(i)(˜ uj ) 2 + lej i=1 τ U Aσ(i)(˜ uj ) 2 + lej i=1 ιL Aσ(i)(˜ uj ) 2 + lej i=1 ιL Aσ(i)(˜ uj ) 2 + lej i=1 κL Aσ(i)(˜ uj ) 2 + lej i=1 κU Aσ(i)(˜ uj ) 2 +
lej i=1 τ L Bσ(i)(˜ uj ) 2 + lej i=1 τ U Bσ(i)(˜ uj ) 2 + lej i=1 ιL Bσ(i)(˜ uj ) 2 + lej i=1 ιU Bσ(i)(˜ uj ) 2 + lej i=1 κL Bσ(i)(˜ uj ) 2 + lej i=1 κU Bσ(i)(˜ uj ) 2
TheweightedJaccardsimilaritymeasuresfor INHFS
lej i=1 τ L Aσ(i)(˜ uj )¯ τ L Bσ(i)(˜ uj )+ lej i=1 τ U Aσ(i)(˜ uj )¯ τ U Bσ(i)(˜ uj )+ lej i=1 ιL Aσ(i)(˜ uj )¯ ιL Bσ(i)(˜ uj )+ lej i=1 ιU Aσ(i)(˜ uj )¯ ιU Bσ(i)(˜ uj )+ lej i=1 κL Aσ(i)(˜ uj )¯ κL Bσ(i)(˜ uj )+ lej i=1 κU Aσ(i)(˜ uj )¯ κU Bσ(i)(˜ uj ) lej i=1 τ L Aσ(i)(˜ uj ) 2 + lej i=1 τ U Aσ(i)(˜ uj ) 2 + lej i=1 ιL Aσ(i)(˜ uj ) 2 + lej i=1 ιL Aσ(i)(˜ uj ) 2 + lej i=1 κL Aσ(i)(˜ uj ) 2 + lej i=1 κU Aσ(i)(˜ uj ) 2 + lej i=1 τ L Bσ(i)(˜ uj ) 2 + lej i=1 τ U Bσ(i)(˜ uj ) 2 + lej i=1 ιL Bσ(i)(˜ uj ) 2 + lej i=1 ιU Bσ(i)(˜ uj ) 2 + lej i=1 κL Bσ(i)(˜ uj ) 2 + lej i=1 κU Bσ(i)(˜ uj ) 2
(10) TheweightedDicesimilaritymeasure
WDSVNHFS (A, B)= m j=1 j
2
lej i=1 τ L Aσ(i)(˜ uj )¯ τ L Bσ(i)(˜ uj )+ lej i=1 τ U Aσ(i)(˜ uj )¯ τ U Bσ(i)(˜ uj ) + lej i=1 ιL Aσ(i)(˜ uj )¯ ιL Bσ(i)(˜ uj )+ lej i=1 ιU Aσ(i)(¯ uj )¯ ιU Bσ(i)(˜ uj ) + lej i=1 κL Aσ(i)(˜ uj )¯ κL Bσ(i)(˜ uj )+ lej i=1 κU Aσ(i)(˜ uj )¯ κU Bσ(i)(˜ uj )
lej i=1 τ L Aσ(i)(˜ uj ) 2 + lej i=1 τ U Aσ(i)(˜ uj ) 2 + lej i=1 ιL Aσ(i)(˜ uj ) 2 + lej i=1 ιL Aσ(i)(˜ uj ) 2 + lej i=1 κL Aσ(i)(˜ uj ) 2 + lej i=1 κU Aσ(i)(˜ uj ) 2 + lej i=1 τ L Bσ(i)(˜ uj ) 2 + lej i=1 τ U Bσ(i)(˜ uj ) 2 + lej i=1 ιL Bσ(i)(˜ uj ) 2 + lej i=1 ιU Bσ(i)(˜ uj ) 2 + lej i=1 κL Bσ(i)(uj ) 2 + lej i=1 κU Bσ(i)(uj ) 2 (11)
TheweightedCosinemeasure
lej i=1 τ L Aσ(i)(˜ uj )¯ τ L Bσ(i)(˜ uj )+ lej i=1 τ U Aσ(i)(˜ uj )¯ τ U Bσ(i)(˜ uj )+ lej i=1 ιL Aσ(i)(˜ uj )¯ ιL Bσ(i)(˜ uj )+ lej i=1 ιU Aσ(i)(˜ uj )¯ ιU Bσ(i)(˜ uj )+ lej i=1 κL Aσ(i)(˜ uj )¯ κL Bσ(i)(˜ uj )+ lej i=1 κU Aσ(i)(˜ uj )¯ κU Bσ(i)(˜ uj ) lej i=1 τ L Aσ(i)(˜ uj ) 2 + lej i=1 τ U Aσ(i)(˜ uj ) 2 + lej i=1 ιL Aσ(i)(˜ uj ) 2 + lej i=1 ιL Aσ(i)(˜ uj ) 2 + lej i=1 κL Aσ(i)(˜ uj ) 2 + lej i=1 κU Aσ(i)(˜ uj ) 2 +
(12) 4.
MultiattributedecisionmakingWithsinglevaluedneutrosophic hesitantinformation
Ye[46]toutilizethevectorsimilaritymeasuresfor SVNHFSs and INHFSs with SVNHF and INHF informationrespectively. Fora MCDM problemwith SVNHF and INHF informationrespectively,let A = {A1, A2,..., An} beasetofalternativesand ˘ C = { ˘ C1, ˘ C2,..., ˘ Cm} beaset ofattributes.Ifforthealternatives ˇ Aj (forj =1, 2,...,n)thedecisionmakers provideseveralvaluesunderthecriteria ˘ Ci (fori =1, 2,...,m), theneachvalue isconsideredasa SVNHF elementand INHF element(¯ τji, ιji, κji)( forj = 1, 2,...,n,i =1, 2,...,m).
Thereforewecanstimulatea SVNHF and INHF decisionmatrixrespectively D =(¯ τji, ιji, κji)n×m, where(¯ τji, ιji, κji)( forj =1, 2,...,n,i =1, 2,...,m) isin theformof SVNHF elementsor INHF elements.
Fortheselectionofbestalternativesinmultiple-criteriadecisionmakingenvironments,theconceptofidealpointhasbeenusedinthedecisionset.Although inrealworldtheidealpointdoesnotexists.Theidealpointdeliverasuitable theoreticalhypothesistoevaluatealternatives.Thereforewedefineeachvalue ineachideal SVNHF or INHF element(¯ τ ∗ j , ι∗ j , κ∗ j )fortheidealalternative
C} as¯ τ ∗ jσ(k) =1, ι∗ jσ(k) =0, κ∗ jσ(k) =0,or τ ∗ jσ(k) = [1, 1], ι∗ jσ(k) =[0, 0], κ∗ jσ(k) =[0, 0],fork =1, 2,...,lei, where lei isthenumberof valuesorintervalvaluesin(¯ τji, ιji, κji)( forj =1, 2,...,n,i =1, 2,...,m). Forthedifferentimportanceofeachcriteriatheweightingvectorofcriteriais givenasˇ =( 1, 2,..., m)T , where i ≥ 0,i =1, 2,...,m,and m i=1 i =1 Thenweexploitthethreeweightedvectorsimilaritymeasuresfor SVNHFSs or INHFSs for MCDM under SVNHF or INHF information,whichcanbe definedasfollows: Step1. Calculateoneofthethreevectorsimilaritymeasuresbetweenthe alternative Aj (forj =1, 2,...,n)andtheidealalternative ˘ A∗ byusingoneofthe threeformulas:
lej i=1 τAσ(i)(˜ uj )¯ τ ∗ ˘ A∗ σ(i)(˜ uj )+ lej i=1 ιAσ(i)(˜ uj )¯ ι∗ ˘ A∗ σ(i)(˜ uj ) + lej i=1 κAσ(i)(˜ uj )¯ κ∗ A∗ σ(i)(˜ uj ) lej i=1 τAσ(i)(˜ uj ) 2 + lej i=1 ιAσ(i)(˜ uj ) 2 + lej i=1 κAσ(i)(˜ uj ) 2 + lej i=1 τ ∗ ˘ A∗ σ(i)(˜ uj ) 2 + lej i=1 ι∗ ˘ A∗ σ(i)(˜ uj ) 2 + lej i=1 κ∗ ˘ A∗ σ(i)(˜ uj ) 2 (13)
D( ˇ Aj , ˘ A∗)= m j=1 i
2 lej i=1 τAσ(i)(˜ uj )¯ τ ∗ A∗ σ(i)(˜ uj )+ lej i=1 ιAσ(i)(˜ uj )¯ ι∗ A∗ σ(i)(˜ uj ) + lej i=1 κAσ(i)(˜ uj )¯ κ∗ A∗ σ(i)(˜ uj )
lej i=1 τAσ(i)(˜ uj ) 2 + lej i=1 ιAσ(i)(˜ uj ) 2 + lej i=1 κAσ(i)(˜ uj ) 2 + lej i=1 τ ∗ ˘ A∗ σ(i)(˜ uj ) 2 + lej i=1 ι∗ ˘ A∗ σ(i)(˜ uj ) 2 + lej i=1 κ∗ ˘ A∗ σ(i)(˜ uj ) 2 (14)
C( ˇ Aj , ˘ A∗)= m j=1 i
lej i=1 τAσ(i)(˜ uj )¯ τ ∗ A∗ σ(i)(˜ uj )+ lej i=1 ιAσ(i)(˜ uj )¯ ι∗ A∗ σ(i)(˜ uj )+ lej i=1 κAσ(i)(˜ uj )¯ κ∗ A∗ σ(i)(˜ uj ) lej i=1 τAσ(i)(˜ uj ) 2 + lej i=1 ιAσ(i)(˜ uj ) 2 + lej i=1 κAσ(i)(˜ uj ) 2 lej i=1 τ ∗ ˘ A∗ σ(i)(˜ uj ) 2 + lej i=1 ι∗ ˘ A∗ σ(i)(˜ uj ) 2 + lej i=1 κ∗ ˘ A∗ σ(i)(˜ uj ) 2 (15)
WeusethefollowingformulasundertheSVNHF information. Under INHF information,weusethefollowingformulas:
DINHFS (A, B)=
j=1 i 2
lej i=1 τ L Aσ(i)(˜ uj )¯ τ ∗L A∗ σ(i)(˜ uj )+ lej i=1 τ U Aσ(i)(˜ uj )¯ τ ∗U A∗ σ(i)(˜ uj )+ lej i=1 ιL Aσ(i)(˜ uj )¯ ι∗L A∗ σ(i)(˜ uj )+ lej i=1 ιU Aσ(i)(˜ uj )¯ ι∗U A∗ σ(i)(˜ uj ) + lej i=1 κL Aσ(i)(˜ uj )¯ κ∗L A∗ σ(i)(˜ uj )+ lej i=1 κU Aσ(i)(˜ uj )¯ κ∗U A∗ σ(i)(˜ uj )
lej i=1 τ L Aσ(i)(˜ uj ) 2 + lej i=1 τ U Aσ(i)(˜ uj ) 2 + lej i=1 ιL Aσ(i)(˜ uj ) 2 + lej i=1 ιL Aσ(i)(˜ uj ) 2 + lej i=1 κL Aσ(i)(˜ uj ) 2 + lej i=1 κU Aσ(i)(˜ uj ) 2 + lej i=1 τ ∗L ˘ A∗ σ(i)(˜ uj ) 2 + lej i=1 τ ∗U ˘ A∗ σ(i)(˜ uj ) 2 + lej i=1 ι∗L ˘ A∗ σ(i)(˜ uj ) 2 + lej i=1 ι∗U ˘ A∗ σ(i)(˜ uj ) 2 + lej i=1 κ∗L ˘ A∗ σ(i)(˜ uj ) 2 + lej i=1 κ∗U Bσ(i)(˜ uj ) 2
(16) (17)
CINHFS (A, B)= m j=1 i
lej i=1 τ L Aσ(i)(˜ uj )¯ τ ∗L A∗ σ(i)(˜ uj )+ lej i=1 τ U Aσ(i)(˜ uj )¯ τ ∗U A∗ σ(i)(˜ uj )+ 2 lej i=1 ιL Aσ(i)(˜ uj )¯ ι∗L A∗ σ(i)(˜ uj )+ lej i=1 ιU Aσ(i)(˜ uj )¯ ι∗U A∗ σ(i)(˜ uj ) + lej i=1 κL Aσ(i)(˜ uj )¯ κ∗L A∗ σ(i)(˜ uj )+ lej i=1 κU Aσ(i)(˜ uj )¯ κ∗U A∗ σ(i)(˜ uj )
lej i=1 τ L Aσ(i)(˜ uj ) 2 + lej i=1 τ U Aσ(i)(˜ uj ) 2 + lej i=1 ιL Aσ(i)(˜ uj ) 2 + lej i=1 ιL Aσ(i)(˜ uj ) 2 + lej i=1 κL Aσ(i)(˜ uj ) 2 + lej i=1 κU Aσ(i)(˜ uj ) 2
lej i=1 τ ∗L ˘ A∗ σ(i)(˜ uj ) 2 + lej i=1 τ ∗U ˘ A∗ σ(i)(˜ uj ) 2 + lej i=1 ι∗L ˘ A∗ σ(i)(˜ uj ) 2 + lej i=1 ι∗U ˘ A∗ σ(i)(˜ uj ) 2 + lej i=1 κ∗L ˘ A∗ σ(i)(˜ uj ) 2 + lej i=1 κ∗U Bσ(i)(˜ uj ) 2
(18) 4.1. Practicalexample. ThisexampleisadoptedfromYe[46]isusedasthe demonstrationoftheeffectivenessoftheproposeddecisionmakingmethodinreal lifeproblem.
ForSVNHinformation
Thereisaninvestmentcompany,whichwantstoinvestsomemoneyinthebest option.Thereisapanelwithfourpossiblealternativestoinvestthemoney: (1) A1 isacarcompany(2) A2 isafoodcompany,(3) A4 isacomputers company,(4) A4 isanarmscompany.Thefollowingthreecriterionsonwhichthe investmentcompanymusttakedecisionare(1) C1 istherisk,(2) C2 isthegrowth, (3) C3 istheenvironmentalimpact.Theweightvectorofthecriteriaisgivenas (0.35, 0.25, 0.4)T thefourpossiblealternative
Thedecisionmatrixforattributesandalternativethedataisrepresentedby SVNHFN
C1 C2 C3 A1 {0 3, 0 4}, {0 2, 0 3}, {0.5, 0.6} {0 4, 0 5}, {0 1, 0 3}, {0.4, 0.6} {0 1, 0 2}, {0 3, 0 4}, {0.6, 0.7}
2 {0.9, 1}, {0.05, 0.07}, {0.01, 0.02} {0.8, 0.9}, {0.1, 0.2}, {0.1, 0.2} {0.7, 0.9}, {0.1, 0.2}, {0.01, 0.02}
A3 {0 5, 0 6}, {0 2, 0 3}, {0 1, 0 2}
A4 {0 9, 1}, {0 1, 0 2}, {0 15, 0 25}
{0 5, 0 7}, {0 1, 0 2}, {0 2, 0 3} {0 5, 0 7}, {0 1, 0 2}, {0 2, 0 3}
{0 8, 0 9}, {0 1, 0 2}, {0 1, 0 2} {0 4, 0 5}, {0 2, 0 4}, {0 3, 0 5}
Table1: Thevaluesobtainedthroughthedefinedweightedvectorsimilarity measuresfor SVNHFS WJSVNHF (A∗ , ˆ Ak) WDSVNHF (A∗ , ˆ Ak) WCSVNHF (A∗ , ˆ Ak) A1 0.22540.33840.4099 A2 0.94200.97620.8752 A3 0.681250.811200.8812 A4 0 730400 82050 8395 Rankingorder ˜ A2 ˜ A4 ˜ A3 ˜ A1 ˜ A2 ˜ A4 ˜ A3 ˜ A1, ˜ A3 ˜ A2 ˜ A4 ˜ A1
Thevaluesobtainedintable1showsthattheJaccardandDicesimilaritymeasureshavethesameresultthatis A2 isthebestalternativewhiletheCosinesimilaritymeasureshowsthat A3 isthebestalternative.Fromtable1weconcludethat theJaccardandDicesimilaritymeasurearebettertouseinmulti-criteriadecision making.
ForINSinformation Nowweconsidertheaboveexamplefor INHFSs, Thedecisionmatrixforattributesandalternativethedataisrepresentedby INHFN
C1 ˜ C2 ˜ C3 A1
{[0 3, 0 4], [0 4, 0 5]}, {[0 2, 0 3], [0 3, 0 4]}, {[0 5, 0 6], [0 6, 0 7]} {[0 4, 0 5], [0 5, 0 6]}, {[0 1, 0 2], [0 3, 0 4]}, {[0 4, 0 5], [0 5, 0 6]} {[0 1, 0 2], [0 2, 0 3]}, {[0 3, 0 4], [0 4, 0 5]}, {[0 6, 0 7], [0 7, 0 8]} A2 {[0 8, 0 9], [0 9, 1]}, {[0 01, 0 02], [0 02, 0 03]}, {[0.01, 0.02], [0.02, 0.03]} {[0 8, 0 9], [0 9, 1]}, {[0 1, 0 2], [0 2, 0 3]}, {[0.1, 0.2], [0.1, 0.2]} {[0 7, 0 8], [0 9, 1]}, {[0 05, 0 15], [0 15, 0 25]}, {[0.01, 0.02], [0.05, 0.09]} A3 {[0.5, 0.6], [0.6, 0.7]}, {[0.2, 0.3], [0.3, 0.4]}, {[0.1, 0.2], [0.2, 0.3]}
{[0.4, 0.5], [0.5, 0.7]}, {[0.1, 0.2], [0.2, 0.3]}, {[0.2, 0.3], [0.3, 0.4]}
{[0 7, 0 8], [0 9, 1]}, {[0 1, 0 2], [0 2, 0 3]}, {[0 1, 0 2], [0 2, 0 3]} {[0 4, 0 5], [0 5, 0 6]}, {[0 2, 0 3], [0 3, 0 5]}, {[0 3, 0 4], [0 4, 0 5]}
{[0.4, 0.5], [0.5, 0.7]}, {[0.1, 0.2], [0.2, 0.3]}, {[0.2, 0.3], [0.3, 0.4]} A4 {[0 8, 0 9], [0 9, 1]}, {[0 1, 0 2], [0 2, 0 3]}, {[0 15, 0 25], [0 25, 0 35]}
Wearealsogivenanunknownpattern ˘ Q.Ouraimistoclassify ˘ Q inoneofthe givenpattern.Theprincipleofrecognizingpatternisthatofthemaximumdegree ofsimilaritybetween SVNHFSs isdescribedby N =arg Max1≤k≤3 CSVNHFS (Ak, ˜ Q)
A1 = { a1, {0.6, 0.7}, {0.2, 0.3}, {0.3, 0.4} , a2, {0.4, 0.5}, {0.3, 0.4}, {0.4, 0.5} , a3, {0 3, 0 4}, {0 2, 0 4}, {0 5, 0 7} }
A2 = { a1, {0 4, 0 5}, {0 3, 0 5}, {0 5, 0 6} , a2, {0 6, 0 7}, {0 2, 0 3}, {0 3, 0 4} , a3, {0 5, 0 6}, {0 2, 0 3}, {0 2, 0 4} } A3 = { a1, {0.2, 0.3}, {0.3, 0.4}, {0.6, 0.7} , a2, {0.4, 0.5}, {0.3, 0.4}, {0.4, 0.5} , a3, {0.6, 0.7}, {0.2, 0.3}, {0.3, 0.4} }
˘ Q = { a1, {0 1, 0 2}, {0 3, 0 4}, {0 7, 0 8} , a2, {0 2, 0 3}, {0 3, 0 4}, {0 6, 0 7} , a3, {0 4, 0 5}, {0 3, 0 5}, {0 5, 0 6} }
(A1, ˘ Q)=0.3501, (A2, ˘ Q)=0.4317, (A3, ˘ Q)= 0.4723
Onecanobservethatthepatternshouldbeclassifiedin A3 accordingtothe patternrecognitionprinciple.ThisresultisthesameobtainedbyYe[36].
For INHFS
Example5.2. For INHFS weusethesameexampledefinedabovejustthepatternsarerepresentedby INHFSs.
˜ A1 = { a1, {[0 4, 0 5], [0 6, 0 7]}, {[0 1, 0 2], [0 2, 0 3]}, {[0 2, 0 3], [0 3, 0 4]} , a2, {[0.3, 0.4], [0.4, 0.5]}, {[0.3, 0.4], [0.3, 0.4]}, {[0.3, 0.4], [0.4, 0.5]} , a3, {[0.2, 0.3], [0.3, 0.4]}, {[0.1, 0.3], [0.2, 0.4]}, {[0.4, 0.6], [0.5, 0.7]} }
A2 = { a1, {[0 3, 0 4], [0 4, 0 5]}, {[0 2, 0 3], [0 4, 0 5]}, {[0 3, 0 5], [0 4, 0 6]} , a2, {[0 4, 0 6], [0 5, 0 7]}, {[0 2, 0 3], [0 3, 0 4]}, {[0 3, 0 4], [0 4, 0 5]} , a3, {[0 4, 0 5], [0 6, 0 7]}, {[0 1, 0 2], [0 2, 0 3]}, {[0 1, 0 3], [0 2, 0 4]} }
A3 = { a1, {[0 1, 0 2], [0 2, 0 3]}, {[0 2, 0 3], [0 3, 0 4]}, {[0 5, 0 6], [0 6, 0 7]} , a2, {[0.3, 0.4], [0.4, 0.5]}, {[0.2, 0.3], [0.3, 0.4]}, {[0.3, 0.4], [0.4, 0.5]} , a3, {[0.5, 0.6], [0.6, 0.7]}, {[0.1, 0.2], [0.2, 0.3]}, {[0.2, 0.3], [0.3, 0.4]} }
˘
Q = { a1, {[0 1, 0 3], [0 2, 0 4]}, {[0 2, 0 3], [0 3, 0 4]}, {[0 6, 0 7], [0 7, 0 8]} , a2, {[0 1, 0 2], [0 2, 0 3]}, {[0 2, 0 4], [0 4, 0 5]}, {[0 5, 0 7], [0 6, 0 8]} , a3, {[0 2, 0 4], [0 4, 0 6]}, {[0 3, 0 4], [0 4, 0 5]}, {[0 4, 0 5], [0 5, 0 6]} }
(A1, ˘ Q)=0.1954, (A2, ˘ Q)=0.1960, (A3, ˘ Q)= 0.2150
Onecanobservethatthepatternshouldbeclassifiedin A3 accordingtothe patternrecognitionprinciple.ThisresultisthesameobtainedbyYe[36].
6. Discussion
Assimplifiedneutrosophichesitantfuzzysetisanimportantextensionofthe Fuzzyset(FS),Intuitionisticfuzzyset(IFS),Singlevaluedneutrosophicset(SVNS), intervalneutrosophicset(INS),Hesitantfuzzyset(HFS)andDualhesitantfuzzy set(DHFS),singlevaluedneutrosophichesitantfuzzyset(SVNHFS),interval neutrosophichesitantfuzzyset(INHFS).Asmentionedabovethathesitancy isthemostcommonproblemindecisionmakingforwhich HFS isasuitable meansbyallowingsevrespossiblevaluesforanelementtoaset.Howeverin HFS theyconsideronlyonetruth-membershipfunctionanditcannotexpressthis problemwithafewdifferentvaluesassignedbytruth-membershiphesitantdegree, indeterminacy-membershipdegree,andfalsity-membershipdegreesduetodoubtsof decisionmakers.andalsoin DHFS theyconsidertwofunctionsthatismembership andnon-membershipfunctionsandcannotconsiderindeterminacy-membership function.Thereforesimplifiedneutrosophicsetsisthemoresuitablefordecision makingandcanhandleincomplete,inconsistenceandindeterminateinformation whichoccurindecisionmaking.Theotheradvantegeofthesimplifiedneutrosophic setisthatitcontaintheconceptofsinglevaluedneutrosophichesitantfuzzysets andaswellasintervalneutrosophichesitantfuzzysets.Ascomparingtoother setsthesimplifiedneutrosophichesitantfuzzysetcontainmoreinformationand thedecisionmakerscangetmoreinformationtotaketheirdecision.
7. Conclusion
Assimplifiedneutrosophichesitantfuzzyset(SNHFS)isanewextensionwhich consistsoftheconceptofFuzzyset(FS),Intuitionisticfuzzyset(IFS),Singlevaluedneutrosophicset(SVNS),intervalneutrosophicset(INS),Hesitantfuzzy set(HFS)andDualhesitantfuzzyset(DHFS),singlevaluedneutrosophicset (SVNHFS),intervalneutrosophichesitantfuzzyset(INHFS).Inthisarticle wedefinedvectorsimilaritymeasuresforsimplifiedneutrosophichesitantfuzzyset andappliedthemtomulti-criteriadecisionmakingandpatternrecognition.We alsoobservethattheJaccardandDicesimilaritymeasuresarebettertoapply inmulti-criteriadecisionmakingproblemthentheCosinesimilaritymeasure.In futureweshallapplytheabovedistancemeasurestomedicaldiagnosisandalso definedforNeutrosophicOverset,NeutrosophicUnderset,andNeutrosophicOffset.
CompliancewithEthicalStructure
Wedeclarethatwehavenocommercialorassociativeinterestthatrepresentsa conflictofinterestinconnectionwithworksubmitted.
Acknowledgments. Theauthorswouldliketothanktheanonymousrefereefor his/hercommentsthathelpedusimprovethisarticle.
References
[1]K.T.Atanassov,”Intuitionisticfuzzysets.”FuzzysetsandSystems20.1(1986):87-96.
[2]K.T.Atanassov,andGeorgiGargov.”Intervalvaluedintuitionisticfuzzysets.”Fuzzysets andsystems31.3(1989):343-349.
[3]S.BroumiandF.Smarandache.”Severalsimilaritymeasuresofneutrosophicsets.”NeutrosophicSetsandSystems1.1(2013):54-62.
[4]S.Broumi&F.Smarandache(2013).Correlationcoefficientofintervalneutrosophicset.In AppliedMechanicsandMaterialsVol.436,pp.511-517.
[5]S.Broumi&F.SmarandacheCosinesimilaritymeasureofintervalvaluedneutrosophicsets. NeutrosophicTheoryandItsApplications.,74
[6]S.Broumi,M.Talea,A.Bakali,F.Smarandache,“SingleValuedNeutrosophicGraphs,” JournalofNewTheory,N10,2016,pp.86-101.
[7]S.Broumi,M.Talea,A.Bakali,F.Smarandache,“OnBipolarSingleValuedNeutrosophic Graphs,”JournalofNewTheory,N11,2016,pp.84-102.
[8]S.Broumi,A.Bakali,M,Talea,andF,Smarandache,IsolatedSingleValuedNeutrosophic Graphs.NeutrosophicSetsandSystems,Vol.11,2016,pp.74-78
[9]S.Broumi,F.Smarandache,M.TaleaandA.Bakali,AnIntroductiontoBipolarSingleValued NeutrosophicGraphTheory.AppliedMechanicsandMaterials,vol.841,2016,184-191.
[10]S.Broumi,M.Talea,F.SmarandacheandA.Bakali,SingleValuedNeutrosophicGraphs: Degree,OrderandSize,IEEEWorldCongressonComputationalIntelligence,2016,pp.24442451.
[11]S.Broumi,F.Smarandache,M.TaleaandA.Bakali,AnIntroductiontoBipolarSingleValued NeutrosophicGraphTheory.AppliedMechanicsandMaterials,vol.841,2016,184-191.
[12]N.Chen,Shu-wei,andLi-naCAI.”Interval-valuedHesitantFuzzySets.”FuzzySystemsand Mathematics6(2013):007.
[13]N.Chen,Z.Xu,&M.Xia,(2013).Correlationcoefficientsofhesitantfuzzysetsandtheir applicationstoclusteringanalysis.AppliedMathematicalModelling,37(4),2197-2211.
[14]L.R.Dice,(1945).Measuresoftheamountofecologicassociationbetweenspecies.Ecology, 26(3),297-302.
[15]B.Farhadinia,(2013).Informationmeasuresforhesitantfuzzysetsandinterval-valuedhesitantfuzzysets.InformationSciences,240,129-144.
[16]B.Farhadinia,(2014).Distanceandsimilaritymeasuresforhigherorderhesitantfuzzysets. Knowledge-BasedSystems,55,43-48.
[17]P.Jaccard(1901),DistributiondelaflorealpinedansleBassindesDroucesetdansquelques regionsvoisines;BulletindelaSoci´et´eVaudoisedesSciencesNaturelles,volume37,no.140, 241–272;
[18]P.J.juan,J.Q.Wang,J.Wang,H.Zhang,andX.Chen.”Simplifiedneutrosophicsetsand theirapplicationsinmulti-criteriagroupdecision-makingproblems.”InternationalJournal ofSystemsScienceahead-of-print(2014):1-17
[19]D.S.Kumar,R.Biswas,andA.R.Roy.”Anapplicationofintuitionisticfuzzysetsinmedical diagnosis.”FuzzysetsandSystems117.2(2001):209-213.
[20]D.Li,andC.Chuntian.”Newsimilaritymeasuresofintuitionisticfuzzysetsandapplication topatternrecognitions.”PatternRecognitionLetters23.1(2002):221-225.
[21]P.Majumdar&S.K.Samanta(2014).Onsimilarityandentropyofneutrosophicsets.Journal ofIntelligentandfuzzySystems,26(3),1245-1252.
[22]L.Peide,andL.Shi.”Thegeneralizedhybridweightedaverageoperatorbasedoninterval neutrosophichesitantsetanditsapplicationtomultipleattributedecisionmaking.”Neural ComputingandApplications26.2(2015):457-471.
[23]G.Salton,&M.J.McGill(1986).Introductiontomoderninformationretrieval.
[24]R.Sahin&A.Kucuk(2014).Subsethoodmeasureforsinglevaluedneutrosophicsets.Journal ofIntelligentandFuzzySystems.
[25]F.Smarandache,Neutrosophy.NeutrosophicProbability,Set,andLogic,Amer.Res.Press, Rehoboth,USA,p.7-8,1998.
[26]F.Smarandache”AUnifyingFieldinLogics:NeutrosophicLogic.”Philosophy(1999):1-141.
[27]Z.Su,Z.Xu,H.Liu,&S.Liu,(2015).Distanceandsimilaritymeasuresfordualhesitantfuzzy setsandtheirapplicationsinpatternrecognition.JournalofIntelligent&FuzzySystems, 29(2),731-745.
[28]V.Torra&Y.Narukawa(2009).Onhesitantfuzzysetsanddecision.InFuzzySystems,2009. FUZZ-IEEE2009.IEEEInternationalConferenceonpp.1378-1382.
[29]V.Torra(2010).Hesitantfuzzysets.InternationalJournalofIntelligentSystems,25(6),529539.
[30]H.Wang,F.Smarandache,Y.-Q.Zhang,R.Sunderraman;(2010)”Singlevaluedneutrosophic sets.”ReviewP.10.
[31]H.Wang,F.Smarandache,Y.-Q.Zhang,R.Sunderraman(2005);IntervalNeutrosophicSets andLogic:TheoryandApplicationsinComputing,Hexis,p.87.
[32]M.Xia,andZ.Xu.(2011)”Hesitantfuzzyinformationaggregationindecisionmaking.” InternationalJournalofApproximateReasoning52.3395-407
[33]M.Xia,Z.XuandN.Chen.”Somehesitantfuzzyaggregationoperatorswiththeirapplication ingroupdecisionmaking.”GroupDecisionandNegotiation22.2(2013):259-279.
[34]Z.Xu,&M.Xia(2011).Distanceandsimilaritymeasuresforhesitantfuzzysets.Information Sciences,181(11),2128-2138.
[35]Z.Xu&M.Xia(2011).Ondistanceandcorrelationmeasuresofhesitantfuzzyinformation. InternationalJournalofIntelligentSystems,26(5),410-425.
[36]J.Ye(2011).Cosinesimilaritymeasuresforintuitionisticfuzzysetsandtheirapplications. MathematicalandComputerModelling,53(1),91-97.
[37]J.Ye(2012).Multicriteriagroupdecision-makingmethodusingvectorsimilaritymeasures fortrapezoidalintuitionisticfuzzynumbers.GroupDecisionandNegotiation,21(4),519-530.
[38]J.Ye(2013).Interval-valuedintuitionisticfuzzycosinesimilaritymeasuresformultipleattributedecision-making.InternationalJournalofGeneralSystems,42(8),883-891.
[39]J.Ye(2013).Multicriteriadecision-makingmethodusingthecorrelationcoefficientunder single-valuedneutrosophicenvironment.InternationalJournalofGeneralSystems,42(4), 386-394.
[40]J.Ye(2013).Anotherformofcorrelationcoefficientbetweensinglevaluedneutrosophicsets anditsmultipleattributedecision-makingmethod.NeutrosophicSetsandSystems,1(1),8-12.
[41]J.Ye(2014).Similaritymeasuresbetweenintervalneutrosophicsetsandtheirapplications inmulticriteriadecision-making.JournalofIntelligentandfuzzySystems,26(1),165-172.
[42]J.Ye&Q.S.Zhang,Q.S.(2014).Singlevaluedneutrosophicsimilaritymeasuresformultiple attributedecisionmaking.NeutrosophicSetsandSystems,2,48-54.
[43]J.Ye(2014).Amulticriteriadecision-makingmethodusingaggregationoperatorsforsimplifiedneutrosophicsets.JournalofIntelligent&FuzzySystems:ApplicationsinEngineering andTechnology,26(5),2459-2466.
[44]J.Ye(2014).Vectorsimilaritymeasuresofsimplifiedneutrosophicsetsandtheirapplication inmulticriteriadecisionmaking.InternationalJournalofFuzzySystems,16(2),204-215.
[45]J.Ye(2015).Improvedcosinesimilaritymeasuresofsimplifiedneutrosophicsetsformedical diagnoses.Artificialintelligenceinmedicine,63(3),171-179.
[46]J.Ye.(2014).VectorSimilarityMeasuresforHesitantFuzzysetandtheirmultipleattribute decisionmaking.EconomicComputation&EconomicCyberneticsStudies&Research,48(4).
[47]J.Ye(2014)”Correlationcoefficientofdualhesitantfuzzysetsanditsapplicationtomultiple attributedecisionmaking.”AppliedMathematicalModelling38.2659-666.
[48]J.Ye.(2015)”Multiple-attributedecision-makingmethodunderasingle-valuedneutrosophic hesitantfuzzyenvironment.”JournalofIntelligentSystems24.123-36.
[49]L.A.Zadeh,(1965)”Fuzzysets.”Informationandcontrol8.3338-353.
[50]H.Y.Zhang,,J.Q.Wang&X.H.Chen(2014).Intervalneutrosophicsetsandtheirapplicationinmulticriteriadecisionmakingproblems.TheScientificWorldJournal.
[51]B.Zhu,Z.Xu,andM.XiaDualHesitantFuzzySets(2012),JournalofAppliedMathematics ArticleID879629,13pages.
[52]B.Zhua&Z.Xu(2014).Someresultsfordualhesitantfuzzysets.JournalofIntelligent& FuzzySystems,26,1657-1668.
TahirMahmood
DepartmentofMathematicsandStatistics,InternationalIslamicUniversity,44000Islamabad,Pakistan
E-mailaddress: tahirbakht@yahoo.com
JunYe
DepartmentofElectricalandInformationEngineering,ShoaxingUniversity,China
E-mailaddress: yehjun@aliyun.com
QaisarKhan
DepartmentofMathematicsandStatistics,InternationalIslamicUniversity,44000Islamabad,Pakistan
E-mailaddress: qaisarkhan421@gmail.com