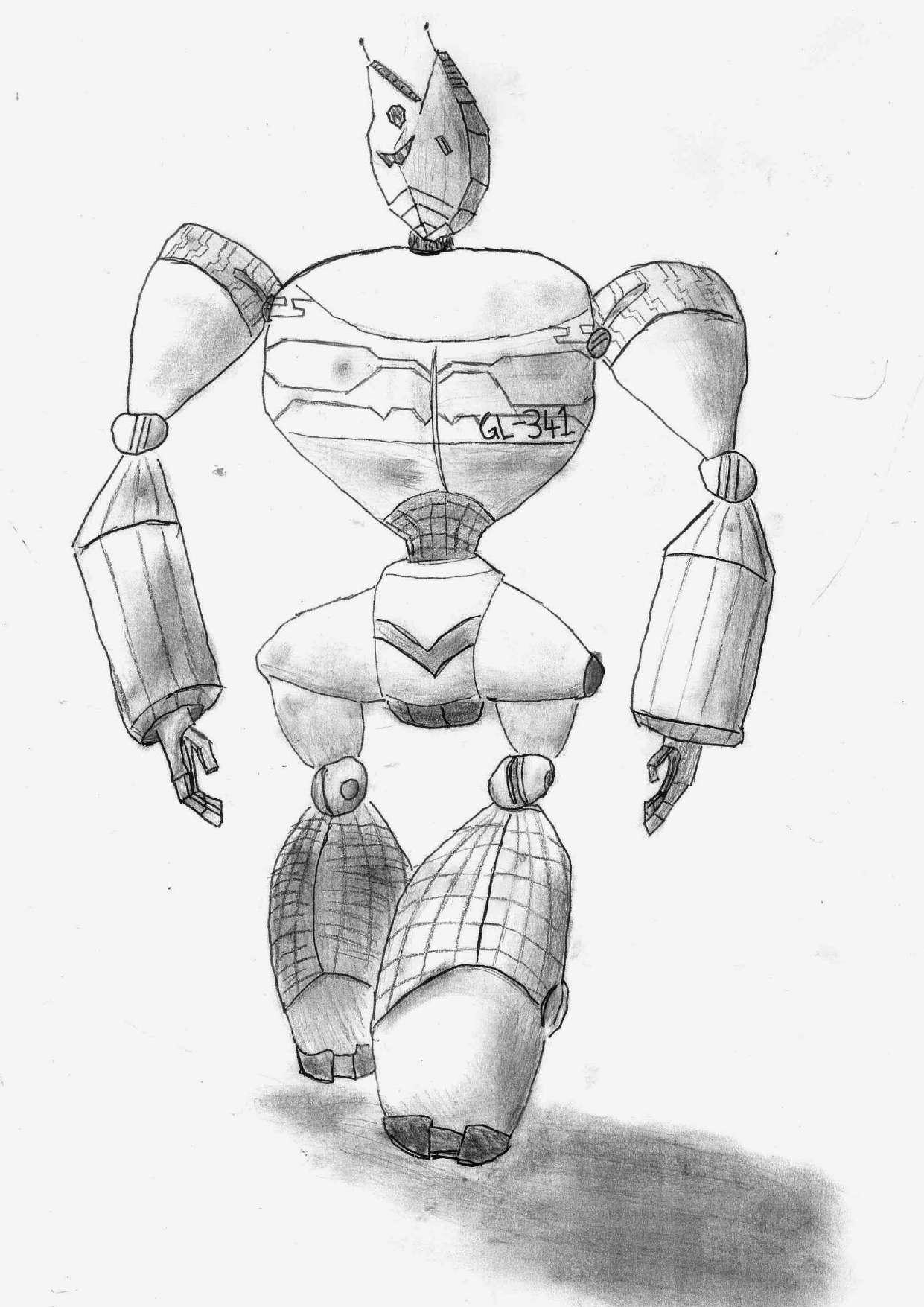
25 minute read
Robotics and Artificial Intelligence: How Far Can and Should AI Take Us? Jett Li
'ROBOTICS & AI' Callum Begbie (Year 6, Darwin)
Robotics and Artificial Intelligence: How Far Can and Should AI Take Us?
Jett Li (Year 12, Peel)
1 INTRODUCTION
In recent times, arguably the most exciting (as well as controversial) field of study is that of Artificial Intelligence (AI) and Robotics. As our world becomes increasingly dependent on machinery and automation as solutions to many of the issues we face, researchers all across the world have begun to search for more ways to integrate AI into our world.
The result of this research and experimentation is apparent. Breakthroughs are being made everywhere, from medicine to economics. We’ve all seen and heard of the potential machines have in the medical field, how they’re unmatched in efficiency and effectiveness and could possibly replace surgeons in the near future, as reported by Katrina Tse in the Scientific Harrovian (Issue IV, 2019). Similarly, many have seen the capabilities of machine learning in finance, especially the ability AI has to create perfect stock profiles and predict its growth to a reasonable degree of accuracy. The results we have seen have been staggering, and the only way is up. Even now, scientists are finding more ways to use AI in our daily lives, which begs the questions:
How did we get to this point? Where do we go from here? Where do we draw the line?
This article will explore these questions in depth and go into detail about the history of AI, what kind of breakthroughs are being made right now, and the ethical and moral issues that accompany the development of AI.
2.1 BEGINNINGS OF RESEARCH
The development of Artificial Intelligence began in the early 20th Century. Spurred on by fictional pieces of work that depicted fantastical forms of artificial beings and their uses, including the Wizard of Oz (1900), Metropolis (Figure 1), and even Frankenstein (1818), researchers began to explore different ways to create a sentient non-human being. This field of study truly began to take off in the 1950s, when an entire generation of scientists, philosophers and thinkers were exposed to widespread media coverage (both fictitious and real) on the possibilities of Artificial Intelligence.
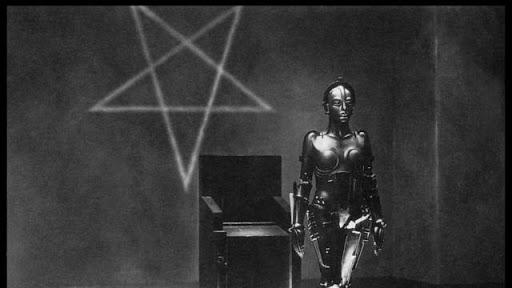
At this point in time, Alan Turing, the ‘father of AI and theoretical computer science’, theorised that machines could use reasoning and logic to solve problems much like humans do. He stated in his 1950 paper ‘Computing Machinery and Intelligence’ that if this were accomplished, it would be possible to build ‘intelligent’ machines and also test their level of intelligence relative to each other and to humans. However, extensive research based on this idea was not immediately purused, as computers were extremely expensive at the time and unobtainable for all but the most prestigious research facilities and universities [1].
However, five years later, a proof of concept - evidence that this theory was feasible - was created by researchers Allen Newell, Cliff Shaw, and Herbert Simon (Figure 2), through what is thought to be the first Artificial Intelligence programme in the world: Logic Theorist. This programme was created to mimic the problem-solving capabilities of a human being and was first presented to the world at the Dartmouth Summer Research Project on Artificial Intelligence (DSRPAI). Despite not making major scientific waves immediately, event attendees unanimously agreed that AI was a viable field of study and that the creation of a true sentient artificial intelligence would be possible in the future. On top of this, Logic Theorist would later be used in several other fields, most notably helping prove 38 of the 52 theorems in the renowned Principia Mathematica (Whitehead and Russell), and producing new and more elegant solutions for some, showing the viability and adaptability of AI. Figure 2: Herbert Simon (L) and Allan Newell (R), two of the developers of Logic Theorist Source: https://www.computerhistory.org
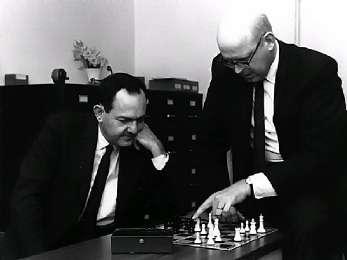
The Logic Theorist would then be the basis for the next two decades of AI-based research all across the globe. By 1974, breakthroughs in computer science had allowed researchers to create more intricate AI programmes such as Newell and Simon’s General Problem Solver (a follow-up programme to the Logic Theorist) and Joseph Weizenbaum’s ELIZA, one of the first computer programmes that was capable of understanding and interpreting human speech. Afterwards, government-funded projects such as the 1982 Fifth Generation Computer Project pushed the development of AI forwards, placing AI computing in the limelight and inspiring generations of programmers to help develop this technology further. By 1997, Deep Blue became the first AI programme to defeat a Grandmaster in Chess, acting as a benchmark for Artificial Intelligence development as machine had finally triumphed over humans in logical thinking and decision making - something unthinkable just a few decades prior.
2.2 TYPES OF ARTIFICIAL INTELLIGENCE
Artificial Intelligence can be split into three smaller subsets: Artificial Narrow Intelligence (ANI), Artificial General Intelligence (AGI), and Artificial Superintelligence (ASI). ANI is the form of Artificial Intelligence that deals with specific functions, and is limited in scope. Many programmes we use today fall within this category as they are created to work in specific environments for specific purposes. AGI is the form of Artificial Intelligence that deals with several fields at once. Programmes like this include those that problem-solve and perform levels of abstract thinking. Lastly, ASI transcends human intelligence and performs on a higher level across all fields. This is ultimately what researchers are working towards [2].
For the majority of AI development, researchers have been creating differing forms of ANI, slowly closing in on the goal of creating an AGI programme on par with normal human intelligence. This is a goal that researchers are very close to completing, as it has been estimated that a fully functional AI on with this level of intelligence will be completed by 2020.
AI can also be classified based on whether it is ‘strong’ or ‘weak’. Weak artificial intelligence programmes are able to respond to inputs by identifying and matching a command to a task that is has already been programmed to do. For example, asking a smart home programme to turn on the lights works because the programme recognises the key terms ‘lights’ and ‘on’, thus allowing them to associate what is being said with a command to turn on the lights. However, this also means that weak AI programmes do not truly understand what they are being told and require a baseline programme to associate commands to in order to function.
On the other hand, strong AI programmes use what is known as clustering and association to process the data they are fed. Unlike weak AI programmes, strong AI doesn’t need programmed responses to fit the inputs they receive, instead they behave more like a human brain and are capable of creating a suitable response by themselves.
2.3 THE SCENE TODAY
Today, the most impactful subfield of AI research is that of ‘machine learning’, which is slowly being integrated into every aspect of our lives. Corporations like Amazon and Google, for example, are using machine learning and data processing AI programmes to sort through massive data sets to match users with the best advertisements and recommendations in order to keep us entertained.
Others are trying to branch out into different fields of study with the aim of improving our quality of life. Very soon we could see AI in the medical field, performing surgeries and prescribing medicine in place of human doctors and pharmacists. AI may also be able to educate children or act as an alternative to psychiatrists or psychologists in the near future with their enhanced cognitive abilities and adaptability to situations. While this seems similar to what we have with human psychologists at first, the fundamental difference lies in the ability of AI programmes to run through data sets too large for humans to manage and therefore they can make better, more informed choices when dealing with individual cases of psychological issues [3].
Besides improving the quality of human life, AI is also on track to improve several facets of the entertainment industry. AI programmes are now becoming even more important in the personalisation of a user’s experience on an entertainment platform and are key components of targeted marketing and advertising campaigns run by Entertainment and Media (E&M) companies. Many corporations are even looking into the creation and implementation of machine learning programmes that can help develop and create advertisements and trailers for upcoming projects [4].
Other branches of practical AI research that may become usable in the near future include space exploration, automating audio post production and self driving vehicles, as described by Ayuka Kitaura in the Scientific Harrovian (Issue IV, 2019).
3 BREAKTHROUGHS AND NEW USES FOR ARTIFICIAL INTELLIGENCE
3.1 TREATING ASD WITH SOCIAL ROBOTS
Since the turn of the century, the frequency of children being diagnosed with ASD (Autism Spectrum Disorder) has shot up, particularly in countries such as the United States. Today, around 1 in 59 children in the United States have been identified as having ASD, according to the Centres for Disease Control and Prevention (CDC), compared to 1 in 150 just 20 years ago. With this explosive growth in individual cases of ASD, the demand for treatment or forms of therapy has similarly increased substantially.
If the ASD ‘epidemic’ was similar to any other form of pathogenic outbreak, dealing with the influx of cases would be costly and difficult to deal with at first, but still ultimately manageable. However
unlike treating diseases or physical ailments, treating a developmental disorder such as ASD is far more complex and therefore more difficult due to the nuances in each and every case. This means that treating the symptoms of this disorder, which include lack of body language, not understanding social cues, not recognising or giving eye contact, and lacking variance in their tone of voice, is something that needs to be targeted and tailor-made for each and every person in need of treatment.
For years, treatment has been done by therapists and doctors, human beings who could slowly adapt to and learn how to deal with their patients and improve their social skills one at a time. People were under the impression that treating developmental or psychological disorders is a uniquely ‘human’ job, since only human beings were capable of adapting their methods of treatment to suit the individual patient. Only humans can teach each other to act and socialise in a correct or proper way. However, soon this may not be the case.
One of the biggest issues with the status quo is that treatment is largely inaccessible due to the high demand but low supply of treatment (the limited number of psychiatrists or children’s doctors). As such, researchers such as Brian Scassellati, a robotics expert and cognitive scientist at Yale University, are in the process of finding a way to get around these shortcomings of ASD treatment. Scassellati has developed a number of social robots (Figure 3) to interact with children afflicted with ASD to see if an AI programme could be more effective than a human at teaching children to pick up social cues. The results he found were striking: after just one session with the robots, many of the children he had been working with were exhibiting forms of normal social behaviour: talking, laughing, and making eye contact during the Q & A session that followed the AI therapy. These milestones in the treatment of ASD, which would usually take some human therapists weeks or even months to accomplish, took the social robots just 30 minutes [5].
Figure 3: One of Brian Scassellati’s social robots. (Source: news.yale.edu)
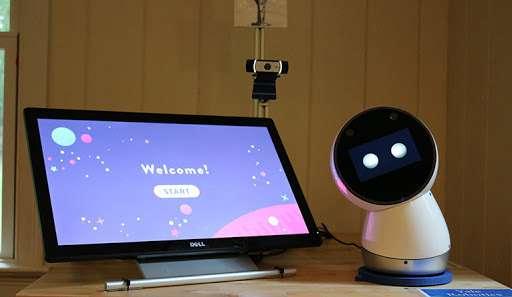
The reason behind the effectiveness of these robots is the subject of much debate. Scassellati, among many of his colleagues, believed that the human-like qualities of the robot the children were interacting with helped make children more responsive to its actions. However, after further testing, it appeared that there was no real difference between using a humanoid robot and a non-humanoid robot as the children responded in almost identical ways. On the other hand, there was a major difference in the quality of treatment between using robots and using a tablet/screen as a replacement. Children were shown to respond far worse to a simple screen projection, which suggested to Scassellati and his team that, while it did not matter how humanoid the robots were, it was the robot’s ability to respond to the child’s actions, without having the inherently ‘human’ qualities such as non-verbal communication, that were so overwhelming to the children in the first place, that made them so effective in their treatment [6].
Since then, Scassellati has conducted many more in-depth studies into how these newly programmed social robots would be able to impact and help the children and families they were loaned out to. During a one-month-long study, he provided 12 families with a tablet computer filled with social games and a new robot called ‘Jibo’ which would provide constant and immediate social feedback to the children during and after their games. Over the course of this month long study, all 12 subjects were shown to have made significant progress in their social development. Many of the children were starting to make prolonged eye contact when talking, initiating conversations, and responded better to communication. However, this form of robot/AI based therapy is still in its nascent stages, as there is no definitive proof that therapy sessions would provide any permanent change in the children’s social skills. Scassellati later stated that “We wouldn’t expect to see any permanent change after just 30 days.”; however, he also believes that this field of study is “very promising”. Many experts in the field feel the same way, and believe that since human-led therapy sessions are both scarce in quantity and expensive, robot-based therapy could be used to enhance the effectiveness of the therapy sessions. In Scassellati’s own words: “Most families can’t afford to have a therapist with them every day, but we can imagine having a robot that could be with the family every day, all the time; on demand, whenever they need it, whenever they want it” [7].
3.2 ALGORITHMS TO AID FUSION TECHNOLOGY RESEARCH
One of the most pressing issues of the modern world is that of energy consumption. As nations develop, the human race as a whole has become more and more dependent on resources used to power our homes and industries. For the past two centuries or so, this has meant using large amounts of fossil fuels such as oil, natural gas, and coal. However, by the mid to late 1900s, alternative forms of energy production began to surface, including solar, wind, hydroelectric, and nuclear power.
Currently, 14% of Earth’s electricity is generated by nuclear power plants. However this figure varies heavily depending on the country, as LEDCs (Less Economically Developed Countries) may only use nuclear power for less than 1% of their energy requirements while MEDCs (More Economically Developed Countries) such as France may use it for up to 75% of its electricity. The reasons why richer countries tend to gravitate towards nuclear power are obvious: nuclear power plants are far more efficient than conventional, fossil fuel-based power plants, and nuclear power is considerably more sustainable and generally less damaging to the environment. In this modern day and age, efficient and clean energy is the way forward for many of the world’s leading nations as they look for ways to maintain energy production or even increase it without contributing even more to the growing issue of global warming.
However, the nuclear power stations used today are still ‘fission’ power plants, meaning they operate by splitting unstable atomic nuclei - typically Uranium-235 - into smaller ‘daughter’ nuclei (releasing energy while doing so). As nuclear fission is based on splitting large atomic nuclei into smaller parts, the byproducts of nuclear reactors are mainly radioactive in nature and can be deadly to living organisms if they are left exposed to the radioactive substances for too long. On top of this, the nuclear waste produced in such reactors have an incredibly long half-life, meaning disposal of this waste is both difficult and expensive. This, coupled with the violently reactive nature of the substances involved in nuclear fission, is what prevents many nations from fully embracing and adopting nuclear fission as a major form of energy provision.
As a result, scientists have been attempting to develop an industrially viable form of nuclear fusion technology, which generates energy by fusing small, harmless nuclei together in the same way that stars in the universe do, providing a clean and just as efficient alternative to replace current fission technology. This would solve the biggest issue people currently have with nuclear energy: that it is dangerous and potentially disastrous, with historical evidence such as the Chernobyl Disaster and Fukushima Meltdown.
However, there have been several setbacks in the development of fusion technology. Research into fusion reactors has been going on since as early as the 1940s, but technical difficulties, such as the sudden loss of confinement of plasma particles and energy during the reaction, have held researchers back for decades. ITER, the leading authority on fusion technology and a collaborative project between China, the EU, India, Japan, South Korea, Russia and the USA, estimates that the first commercially viable fusion reactor will be available sometime after 2054. However, with the help of AI and deep learning programmes, this may actually be accomplished much sooner than expected. In the US Department of Energy’s Princeton Plasma Physics Laboratory (PPPL) and Princeton University, scientists are beginning to use AI programmes to forecast and prevent sudden and unexpected nuclear fusion reactions that may damage the tokamaks, the devices that confine them (Figure 4) [8] [9].

Figure 4: Depiction of fusion research on a doughnut-shaped tokamak enhanced by artificial intelligence [8]
The deep learning code, the Fusion Recurrent Neural Network (FRNN), is built upon the massive databases provided by decades of research from the DIII-D National Fusion Facility and the Joint European Torus, two of the largest fusion facilities in the world. From the new data, FRNN is able to train itself to identify and predict disruptions on tokamaks outside of its original dataset (from Princeton’s facilities) using ‘neural networks’, which use mathematical algorithms to weigh the input data and decide whether a disruption will occur, or how bad the disruption will be, adjusting itself after every session for mistakes made. A disruption, caused by a rapid loss in stored thermal and magnetic energy due to growing instability in the tokamak plasma, can lead to the melting of the first wall in the tokamak and leaks in the water cooling circuits. Soon, FRNN will reach the 95% accuracy threshold within the 30 millisecond time frame required by researchers in the field of study, which means it will soon be a practical and critical part of fusion research in the near future. This new way of understanding and predicting disruptions, one of the key issues that have plagued fusion development for decades, may also evolve into an understanding of how to control them [10].
Julien Kates-Harbeck, a Harvard physics graduate and a collaborator of the FRNN project, stated that although FRNN is currently able to predict and help mitigate the devastating impacts of disruptions, the end goal is to “use future deep learning models to gently steer the plasma away from regions of instability with the goal of avoiding most disruptions in the first place.” However, moving from an AI-based predictive programme to one that can effectively control plasma would require much more work and comprehensive code which encompasses the first principles in physics. While this seems like yet another setback in a century-old quest for the so-called ‘holy grail’ of energy, Bill Tang, a principal research physicist in PPPL, states that controlling plasma is just “knowing which knobs to turn on a tokamak to change conditions to prevent disruptions” and that it is “in our sights and it’s where we [PPPL team] are heading” [11].
The main issue with the way surgeries are conducted in the modern world is how they can easily be impacted by human error. Operations are complex and convoluted procedures that require the operators to have a specific skill set for each and every patient they come across. Many of these surgical procedures involve making extremely precise cuts and incisions, leaving little to no room for error. As a result, the possibility of human mistakes impacting the success of a procedure is extremely high. The possibility of mistakes are further exacerbated by the high-stress environments surgeons and doctors work under as well as the long shifts they typically take. As a result, surgeries are less efficient and more timeconsuming than they otherwise should be.
This is where AI-assisted surgeries come in. Even though these programmes are nowhere near sophisticated enough to complete operations on their own, they are more than capable of helping reduce a surgeon’s variations that could affect the outcome of the procedure and the patient’s recovery. Doctor John Birkmeyer, chief clinical officer at Sound Physicians, stated that “a surgeon’s skill, particularly with new or difficult procedures, varies widely, with huge implications for patient outcomes and cost. AI can both reduce that variation, and help all surgeons improve – even the best ones.” Programmes are able to help surgeons determine what is happening during complex surgical procedures by providing real-time data about the movements and cuts the surgeons make. This allows surgeons to work with a greater degree of precision and accuracy as they are able to adjust and adapt to the information they are given on the fly. According to a study, AI-assisted surgeries also result in fewer complications compared to human surgeons working by themselves [12].
Figure 3: An artistic depiction of the role of AI in surgery. (Source: Robotics Business Review)
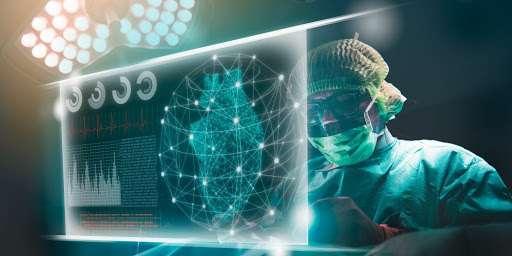
Even though this kind of technology is still in its early stages, it’s already seeing great success around the world as an aid to conventional forms of surgery and, when paired with robotic systems, can perform tasks such as suturing small blood vessels (as thin as 0.03mm-0.08mm). The possibilities of AI-assisted surgeries are seemingly endless. However, AI can also benefit the medical field in ways beyond just this.
Another key development in the medical AI field is the creation of virtual nursing assistants. A significant expense of any hospital or healthcare facility is the money spent on nurses and caretakers of patients. From interacting with patients to directing patients to the most effective care setting, these workers deal with many aspects of patient treatment by giving physical support through treatments, as well as emotional support through basic interactions and care-taking duties. It has been estimated that nurses account for close to $20 billion USD annually in the healthcare industry, a figure that could be drastically reduced after the introduction of AI-based, virtual nursing assistants.
Virtual nursing assistants would resolve many of the issues that the current healthcare industry has to contend with. Similar to how mental health robots and surgical-assisting AI help pre-existing doctors and psychiatrists deal with their workload more efficiently, virtual nursing assistants (which operate 24/7) can monitor patients, interact with them, and help dispel worries patients may have about their own well-being. On top of their ability to interact directly with patients, these programmes are also able to help with administrative tasks such as scheduling meetings between patients and doctors and even prescribe medication for patients leaving the hospital. All things considered, the potential of these virtual nursing assistants are immense and could save the industry billions of dollars in the long run, as less money is needed to pay the nurses and care-takers, which used to be distinctively ‘human’ jobs.
4 SHORTCOMINGS OF AI AND ROBOTICS
4.1 LACK OF EMOTIONAL INTELLIGENCE
One major area of concern in the development and use of AI is their perceived lack of humanity and human-like qualities. Many experts in fields that deal with interpersonal relationships or social interaction (including psychologists, therapists and doctors) believe that AI or social robots will be incapable of ever fully replacing humans in these fields. The gap between a programme designed to mimic humanity and true social interactions is seemingly insurmountable, which leads many to believe that AI programmes, no matter how comprehensive or well-made, will only be able to play a supporting role to humans [13].
However, this shortcoming of artificial intelligence may soon be dealt with. While it is true that current programmes cannot effectively identify human emotions or social cues in the same way humans can, breakthroughs in the research of ‘emotional AI’ with programmes that can detect and recognise emotions (a process called facial coding in market research) then react accordingly, may one day help artificial intelligence programmes step into these previously ‘untouchable’ fields. Experts believe that emotional AI could be used as a medical tool to help diagnose mental ailments such as depression and dementia, or be used in medical facilities as ‘nurse bots’ to monitor and support patients [14].
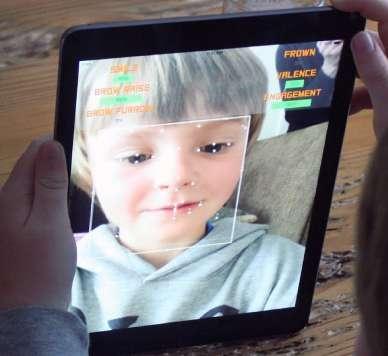
Figure 5: example of emotional detection software [13] 4.2 SENTIENT PROGRAMMES
Perhaps the biggest worry people have about the development of Artificial Intelligence is the possible rise of sentient AI programmes. While our current level of technology is still several decades away from being able to create programmes powerful enough to pose the problem the general public envisions, the potential issues of sentient AI are still food for thought.
For a programme to be classified as ‘sentient’, it must first fulfil two requirements: first, the programme must be capable of being self-aware, and secondly, the programme must be able to be classified as ‘conscious’. As of right now, humanity has already created several programmes capable of rudimentary self-awareness, many of which are capable of analysing and adapting to their surroundings without prompts from code. However, although humanity is capable of creating robots that can mimic a human’s consciousness and understanding of themselves/the outside world, we are still unable to make programmes capable of this without using data from the internet, or by simply learning and understanding consciousness by themselves [15].
As humanity has just entered the AGI phase, it is unlikely that we will experience anything like what we see in films such as The Terminator or Ghost in the Shell (Figure 6). A survey conducted in 2016 revealed that the majority of experts in the field of AI research and computer science feel that it will take at least 50 more years for technology to develop to the point where AI will be able to replace skilled human jobs. Right now, most programmes operate based on memorising data, identifying patterns within them, and slowly learning to match new pieces of input data to the pattern they have identified, which is very different from how human minds operate (based on prediction rather than memorisation). Facebook’s head of AI development, Yann Lecun, stated that in terms of unfocused intelligence, AI programmes are currently behind rats. As a result of this, it seems more reasonable to see the rise of sentient AI as a potential long term issue after humanity reaches the ASI research stage; however, until then, no one is in danger of being overtaken or undermined by these programmes [16].
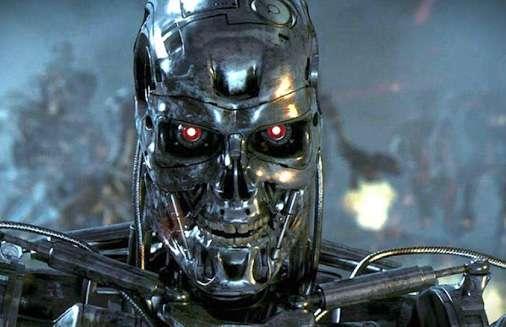
Figure 6: An example of a currently unachievable robot from The Terminator franchise
(Source: Film Stories)
5 CONCLUSION
To conclude, the potential of Artificial Intelligence programmes are limitless. As technology and our understanding of computer science develops, humanity will inevitably become increasingly dependent on the abilities of AI programmes. In the short term, we will see developments and improvements in industries such as healthcare and energy, which will help propel the human race forward. Currently, fears of AI becoming too influential or powerful, or even developing ‘sentience’ are unfounded, as humanity is still decades away from being capable of developing Artificial Superintelligence (ASI) programmes, which are truly sentient or self-aware.
In the foreseeable future, the development and growth of AI-based fields of research should be monitored, but not restricted. The only real harm AI can do to humanity in the next 20-30 years or so is lowering the demand for certain jobs, which would become increasingly automated. The upsides to this are explosive economic, educational and healthcare developments. AI programmes can be a powerful and useful tool during this period of time, however upon reaching the Artificial General Intelligence phase (AGI), humanity needs to become more wary of these programmes and begin to place restrictions and limitations on their usage. Until that point, we should enjoy the benefits brought by these programmes to the fullest.
BIBLIOGRAPHY [1] “The History of Artificial Intelligence” - Harvard http://sitn.hms.harvard.edu/flash/2017/history-artificial-intelligence/
[2] “The Evolution of Artificial Intelligence” - UBS https://www.ubs.com/microsites/artificial-intelligence/en/new-dawn.html
[3] “AI in Movies, Entertainment, Visual Media”- Emerj https://emerj.com/ai-sector-overviews/ai-in-movies-entertainment-visual-media/
[4] “The State of AI in 2019”-The Verge https://www.theverge.com/2019/1/28/18197520/ai-artificial-intelligence-machine-learningcomputational-science
[5] “How 30 Days with an in home robot could help children with ASD”-ScienceMag https://www.sciencemag.org/news/2018/08/how-30-days-home-robot-could-help-children-autism
[6] “Artificial Intelligence and Autism”-Towards Data Science https://towardsdatascience.com/artificial-intelligence-and-autism-743e67ce0ee4
[7] “Improving social skills in children with ASD using a long term, in home, social robot” - ScienceMag https://robotics.sciencemag.org/content/3/21/eaat7544
[8] “Artificial Intelligence accelerates development of limitless fusion energy”- SciTechDaily https://scitechdaily.com/artificial-intelligence-accelerates-development-of-limitless-fusion-energy/
[9] “A Nuclear Powered World” -NPR https://www.npr.org/2011/05/16/136288669/a-nuclear-powered-world
[10] “Fusion Energy Pushed back beyond 2050” -BBC https://www.bbc.com/news/science-environment-40558758
[11] “Plasma Disruptions : A task force to face the challenge” - Iter https://www.iter.org/newsline/-/3183
[12] “How AI assisted surgery is improving surgical outcomes” - Robotics Business Review https://www.roboticsbusinessreview.com/health-medical/ai-assisted-surgery-improves-patientoutcomes/
[13] “Emotion AI overview” - Affectiva
[14] “13 Surprising Uses for Emotion AI technology”-Gartner https://www.gartner.com/smarterwithgartner/13-surprising-uses-for-emotion-ai-technology/
[15] “Researchers are already building the foundation for sentient AI” - VentureBeat https://venturebeat.com/2018/03/03/researchers-are-already-building-the-foundation-for-sentient-ai/
[16] “How far are we from truly human like AI?” - Forbes https://www.forbes.com/sites/forbestechcouncil/2018/08/28/how-far-are-we-from-truly-human-likeai/#3b494a2031ac