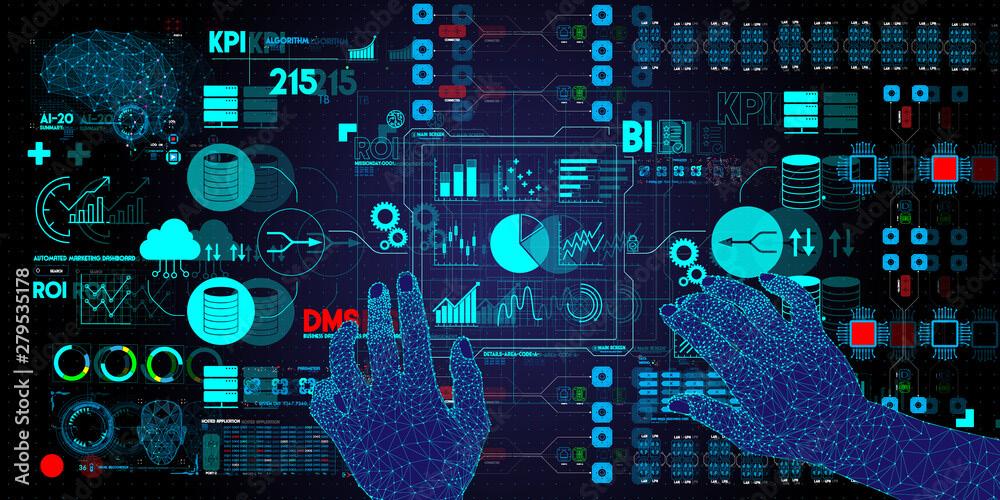
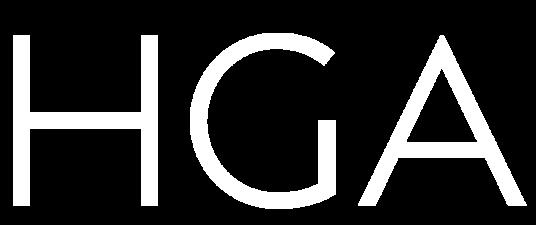
DESIGNING FOR DATA AND COMPUTATIONAL SCIENCES
Implications
Implications
Implications
Incorporating
Implications
Implications
Implications
Incorporating
Computer Science (CS) and the swarm of applied information technologies have a profound and rapid impact on all sectors of society, government, and business. Integrated not only in the everyday activities of modern society as consumables, but also in every academic discipline as a primary tool for analysis, computing has long existed outside labs of computer science departments. We are experiencing an unprecedented demand for graduates that are digitally literate with computing skills matched by a dramatic increase in computer science course enrollments.
By 2025 the amount of digital data generated each year is projected to reach 175 zettabytes (ZB is one billion terabytes) up from 33ZB in 2018. (Forbes)
Higher education institutions are investing heavily to address the ubiquity of computing and data, the need to broaden students’ digital literacy, and the infrastructure to support it.
The integration of computer science and engineering has been a natural path to take, giving rise to fields like self-governing robotics and quantum computing. Incorporating other sciences like biology and medicine was the next step, yielding smart prosthetics and machine-learning-based imaging analysis. But machine vision and sensors are only drops in the vast ocean of data generation.
Data is everywhere. Every academic discipline, from social science to the arts and humanities, is impacted. Every business decision, social media post, and financial transaction leaves a digital record that the world accumulates. These massive amounts of data can be converted into valuable insight using the expert skills acquired in computing and data analysis courses. Non-scientific domains like law and public policy have also been disrupted by Big Data, while leading edge technologies such as Machine Learning (ML), Artificial Intelligence (AI), and Extended Reality (XR) are used in business and creative writing, real-time consumer habit analysis, and interactive art installations.
The need for digital and computing-literate graduates is bringing a twopronged challenge to higher education institutions:
• Undergraduate computing courses are seeing a sharp increase in enrollment by both CS majors and non-majors. This creates demand for classroom space and faculty.
• Industry jobs are offering lucrative opportunities for both graduates and Ph.D. students, leading to a sharp reduction in the hiring pool for faculty.
The US Bureau of Labor Statistics projects that computer occupations will grow three times as fast as the average between 2019 and 2029 and will account for two-thirds of all new STEM jobs created.
According to a 2018 report by the National Academies of Sciences, Engineering, and Medicine (NASEM), computer science enrollments are at an all-time high, creating severe pressure and demand on college and university computer science departments. The report highlights that:
“... with more than half of new CS Ph.D.’s drawn to opportunities in industry, hiring and retaining CS faculty is currently an acute challenge that limits institutions’ abilities to respond to increasing enrollments.”
HGA Principal and Design Principal Samir Srouji and the Design Insights Group (DIG) led a research investigation to better understand the impact of the data and computational sciences revolution on architectural design. This study was triggered by our work on projects ranging from research university transdisciplinary science and engineering centers to liberal arts college digital innovation facilities.
The investigation sought to understand:
• Drivers of data and computational sciences programs.
• How these drivers inspire design solutions.
• What types of successful outcomes can be anticipated as a result.
The investigation team applied primary and secondary research methods, including literature review, building program and floor plan analysis. Data and computational science program directors at four national universities were also interviewed and asked about their recent experience participating in the planning and design process for their current facilities.
• “Computer Scientist Skills: Definition and Examples.” Indeed Career Guide, www.indeed.com/career-advice/resumes-cover-letters/ computer-scientist-skills
• National Academies of Sciences, Engineering, and Medicine (NASEM). Assessing and Responding to the Growth of Computer Science Undergraduate Enrollments. National Academies Press, 2018, https:// doi.org/10.17226/24926.
• Strack, Rainer, et al. What’s Trending in Jobs and Skills. Boston Consulting Group (BCG) x Burning Glass Technologies , 2019, webassets.bcg.com/8b/5c/9472831c43e885a14bd0a3fd5cf0/bcg-what-istrending-in-jobs-and-skills-september-2019.pdf.
• “The Future of Jobs Report 2023.” World Economic Forum, 30 Apr. 2023, www.weforum.org/publications/the-future-of-jobs-report-2023/in-full/.
• Tynan, Dan. “From Jobs to Skills: What the Future of Work Will Look Like.” Forbes, 28 June 2023, www.forbes.com/sites/guild/2023/06/28/fromjobs-to-skills-what-the-future-of-work-will-look-like/?sh=a10d4a4169eb
• U.S. Bureau of Labor Statistics. “Employment in STEM Occupations : U.S. Bureau of Labor Statistics.” Bls.gov, U.S. Bureau of Labor Statistics, 4 Sept. 2019, www.bls.gov/emp/tables/stem-employment.htm.
• Wood, Sarah, and Ilana Kowarski. “What Can You Do with a Computer Science Degree?” U.S. News & World Report, 9 May 2023, www.usnews. com/education/best-graduate-schools/articles/what-can-you-do-witha-computer-science-degree.
• Boston University | Center for Computing & Data Sciences
• Dartmouth College | The Class of 1982 Engineering and Computer Science Center
• Hamilton College | The Digital Gateway
• Michigan State University | Engineering and Digital Innovation Center
• University of Arkansas | Institute for Integrative and Innovative Research
• University College Dublin | O’Brien Centre for Science
• University of Maryland | Brendan Iribe Center for Computer Science and Engineering
• University of Washington | Bill & Melinda Gates Center for Computer Science & Engineering
• Amy Barrett, Assistant Provost, Academic Space Planning, Boston University
• Azer Bestavros, Associate Provost for Computing and Data Sciences and Founding Director of the Hariri Institute for Computing, Boston University
• David F. Kotz, Provost and Professor of Computer Science, Dartmouth College
• Hany Farid, Associate Dean and Head of School of Information; Professor for Electrical Engineering and Computer Sciences and the School of Information, University of California, Berkeley
• Munther A Dhleh, Founding Director of the Institute for Data, Systems, and Society (IDSS), Massachusetts Institute of Technology
It is difficult to overstate the impact of CS and technology on the world today: computing touches every aspect of our lives. Increasingly, humans experience life through CS-enabled devices. HGA’s research identified the following key findings:
A. The computing revolution has affected a vast array of disciplines and blurred the lines between tech and non-tech fields.
B. Amidst spiking CS enrollment, job growth outpaces worker creation.
C. Traditional workforce skillsets are being disrupted.
D. Faculty retention and recruitment are highly competitive.
E. There are seven key drivers in the planning of CS educational programs and facilities.
F. There are four key metrics to help establish the target mix of space types in new facilities.
Every CS student quickly learns that the field is astonishingly broad and deep, spanning technologies such as AI and ML, blockchain, cloud and distributed edge computing, data science, robotics, Internet of Things (IoT), and Extended Reality (XR).
A vast array of job categories exists within each technology, including software and hardware engineering; app, web, and game development; information management; and network architecture, to name a few. Often expertise in multiple technologies is needed for success.
MATH & STATISTICS
AI/ ML
DIGITAL INNOVATION
DATA SCIENCE
ENGINEERING & COMPUTER SCIENCE
SOFTWARE DEVELOPMENT
SCIENCE & HUMANITIES
A key component in the complex and rapidly changing computer science landscape is the dramatic shift in supply and demand for computing professionals. The NASEM aimed to improve understanding of the surge in higher education, computer science degree enrollment, and job growth in related fields. The NASEM published their findings in a 2018 report.
• 74% increase in CS majors at US colleges and universities between 2009 and 2015.
• Increased enrollment in CS courses for both CS and non-CS majors, with no indication of a decrease in demand.
• The number of jobs in computing technologies exceeds the number of CS graduates.
• Employment in computer occupations is expected to grow nearly twice as fast as the labor market as a whole between 2018 and 2028.
While many computer graduates aspire to work for the Silicon Valley giants, CS and technology jobs have dramatically increased in non-tech sectors of the economy— business, healthcare, education, entertainment, and government.
Analytics software company Burning Glass Technologies found the following trends among information technology job postings:
89% 65%
“For people who study computer science in their education, it’s a great, great time, and essentially the sky is the limit.”
(Salvatore Stolfo, Professor of Computer Science at Columbia University)
growth of job postings in the non-tech sector between 2013 and 2018.
40% of job postings in 2018 were in the non-tech sector.
growth of job postings in the tech sector between 2013 and 2018.
What are employers looking for in a computer scientist? According to Indeed.com, a successful candidate will have a combination of hard skills, i.e. technical proficiency and soft skills, like critical thinking, communication, teamwork, and work habits. While the former can be acquired in the solitude of one’s home via a plethora of online classes, the latter is developed through social networking, team projects, and purposefully collaborative transdisciplinary pursuits.
The skill-set requirements are rapidly evolving. The World Economic Forum (WEF), in collaboration with Forbes magazine, predicts that skillbased hiring will become increasingly important as AI machines start to automate repetitive tasks such as data entry and basic programming.
WEF’s 2023 Future of Jobs Report shows:
“44% of working skills will be disrupted over the next five years, with analytical skills, creative thinking, and technological literacy among those in greatest demand.”
Examples of this disruption can be seen even now. Burning Glass Technologies, in collaboration with Boston Consulting Group, analyzed 95 billion job listings over three years. The key finding was the increase of specialized or advanced skills in a wider range of positions. These increases occurred in both tech and non-tech industries, signaling the reshaping of skill requirements in the future, and driving business and humanities students to pursue enrollment in core CS courses.
• Natural Language Processing (NLP) is a requirement for some engineering jobs.
• AI and ML skills are appearing in job ads for marketing managers and business intelligence (BI) analysts.
• IoT skills are showing up in sales and marketing positions.
• Skills in cloud solutions and data visualization are being called for in marketing, analyst, and sales positions.
High Growth Skills are Associated with the Latest Technologies
Average growth in number of mentions in online postings, 2015-2018 (%)1
Experienced accelerating growth in the number of mentions from 2017-2018
Sources:
1 Average growth is the average year-over-year percentage change in the number of appearances in online
With the growing demand for graduates with computing skills and increased college enrollment, the competition to retain and recruit computer science faculty has increased exponentially. Lucrative business offers draw away a large portion of new Ph.Ds., and institutions are finding that fiscal compensation is not enough to recruit faculty into academia—a full package of user experience is desired.
We have learned that the quality of the facilities and the vibrancy it projects play a major role in attracting students and faculty. Buildings that project a sense of community, openness, and provide a variety of engagement modes seem to do this more successfully. Having state-ofthe-arts technical labs is not sufficient without the amenities that bring people together to foster collaboration and form community.
“This inspired and inspiring space will be an essential faculty recruitment tool. I am happy to say we have successfully recruited four computer science faculty this year, with two additional offers still pending. I can tell you that place and space make an enormous difference in successful recruitment of future faculty, especially in the sciences.”
(Elizabeth F. Smith, Ph.D., Dean of Faculty of Arts and Sciences, Dartmouth College)
HGA’s research uncovered common themes that drive higher education institutions into new territories, connecting the digital and physical sciences with the humanities and social sciences. It is paramount to foster these collaborations across multiple disciplines to educate graduates who have honed the diverse set of hard and soft skills needed in the workforce.
The team identified seven key drivers for decision-making during the planning of computer science education programs and facilities.
Expand programs and faculty to respond to the growing number of students seeking careers requiring computing skills.
Provide flexible spaces that accommodate a variety of teaching styles through evolving technologies, interactive, team-based learning, and hybrid learning models.
Create warm, welcoming, vibrant, technology-rich environments for social interaction and virtual and in-person engagement.
Connect traditional academics with external stakeholders. Provide space for industry engagement and embed entrepreneurship centers within the program.
Develop linkages and networks within the building and across campus. Establish open-access hacker and maker spaces to extend data and technology reach beyond STEM fields.
Foster soft skill development by embedding ideation and work environments that support planned or spontaneous connections and can adapt to dynamic team sizes.
Locate groundbreaking research in central nodes to celebrate the success of the institution. Provide a mix of standard and specialized laboratories to further advancements in theoretical and experimental research.
The research team compared area and population metrics across six case study buildings and extracted key ratios that can inform the programming and planning of these project types. Some of the data points collected indicate building efficiency impacts, average research team size, ratios of laboratory to write-up space, and many more.
HGA is continuously adding to the data set beyond the completion of the research project. This searchable comparative data set enables us to advise our clients on best practices to achieve their academic goals.
The team saw an increase in the number of larger, flat-floor, bring-your-own device interactive classrooms. Rising enrollment and faculty shortages are driving up CS introductory class sizes (400-500 students and growing) and forcing pedagogies that rely on multiple teaching assistants to support a single faculty member, paired with online participation. This enlarged class size brings unique challenges to the architectural design of the room, including acoustic and line-of-sight issues, monitor content distribution, voice lift, and two-way streaming technologies to enable each student to be heard—physically present or online— power to each table, and robust WIFI systems.
The family of Technology-Enabled Active Learning (TEAL) classrooms is large, and each institution has a specific flavor of space and technology arrangements that best reflects their needs.
Computing education and research primarily occurs in two major building typologies—the “basic” computer science building, focusing on maximizing office, computational and instructional spaces, and the transdisciplinary Engineering++ center that focuses on providing specialized facilities for research and teaching that cannot be easily accommodated elsewhere on campus. Understanding the high-level research and teaching program components and methodologies, as well as how the new facility fits within the campus mission, will help frame decision-making around the space types and utility intensity mix.
The general trend is away from planning dedicated computer science buildings, in favor of the transdisciplinary centers.
In higher education research, we are also seeing the disruptive effect of computing. Physical and life sciences teams increasingly have permanent “non-lab” members and are shifting wet lab programming metrics toward greater ratios of office and collaboration space to lab components. Fields like computational biology and theoretical physics are placing CS graduates in the lab environments, counteracting the previous trend.
With emerging technologies like computer vision, robotics, and XR, a substantial portion of computing is shifting away from the graduate student desk and towards a hybrid compute-and-build environment, the size of which is driven not by the student desk allocation, but by the size and function of the equipment and experimental procedures.
Key measures for planning research buildings include the average team size and the area per team member, calculated as the sum of per-person allocations for research offices and labs, and core labs. Both measures are directly related to the nature of the research methodology and experimental needs. As illustrated below, the CS building typology clusters around a team size of five and 90 SF/ person, while the Engineering++ center exhibits a much wider distribution of space needs.
Amenities and community spaces are key components to the success of any building. Both types average in the 13-15% range of the user program. An important divergence is the percent open to enclosed community space, respectively 1:8 in the computer science building vs 1:1 in the engineering ++ building type.
In a CS building, the primary research space is often an open office environment, driving the need for a larger portion of enclosed group workspaces. In a transdisciplinary building, much of the population’s primary workspace is a laboratory, which, in turn, necessitates the availability of large, open, warm spaces to take a respite from the edges of hard science.
Emerging trends show the reduction of faculty office sizes. Typically, the number of guest chairs is reduced, and the meeting table is removed. This is offset by providing more shared conference rooms in the office cluster. In correlation, we see a rise in the overall percentage of community space in the building. Balancing the dedicated versus general access collaboration spaces becomes a cultural preference. Both CS and Engineering ++ buildings are following this trend.
It is hard to make predictions beyond the next few years about the future of computing and data science. Artificial intelligence (AI) and machine learning (ML) fields are expected to grow exponentially; new disruptive technologies often come as a big surprise and take hold quickly.
A few big ideas are likely to remain important: Data and computing will continue to transform every discipline and sector of society, making transdisciplinary collaboration and research paramount.
• Rapidly changing technologies will require adaptable and flexible teaching and research spaces.
• A significant investment by academic institutions in quantum research will transform the field dramatically.
As architects, we recognize that we must design spaces that are flexible, accommodate evolving technologies, and provide research and learning environments that allow for harnessing of data and emerging technologies.
“We are essentially designing a new building type that is the equivalent of a Swiss army knife with multiple capabilities for research and learning. These facilities must be capable of re-generating themselves over time.”
(SamirSrouji, HGA Design Principal)
Interactive team-based learning and research are integral to computer science education. CS teaching spaces also need to accommodate a range of learning and teaching styles. Considerations to keep in mind:
• Classrooms with flexible seating to accommodate small group work, student-led discussions, and blended learning.
• Multi-purpose furniture that supports learning activities ranging from heads-down work to collaborative co-creation.
• Maker and hacker spaces to facilitate hands-on learning and prototyping of project ideas.
• Traditional lecture spaces with robust online learning capabilities to accommodate hybrid teaching.
• Technology-rich spaces to support learning and group work throughout the building.
Computational research takes place in two broad categories of space— the office-like “computer lab” and the specialized “electronics lab.” Research topics and their instrumentation have a fast-paced turnover and often dynamic team size over the life of the project. Consequently, flexibility of research and lab spaces is key: it is vital to be able to easily adapt space to accommodate new research initiatives.
Creating this adaptable environment in the computer lab incorporates features like plug-and-play power with floor and ceiling distribution, robust data connectivity—hardwired or wireless, and open ceilings with overhead pipe grids for equipment mounting. Dartmouth College Engineering and Computer Science Center features a 2” raised floor for power and data cable distribution to allow for reconfiguration of the space as the research changes and uses the overheard pipe grid. While the former solution has been a long-standing staple in commercial office buildings, we are now seeing it used more in educational computational facilities. Similarly, while the latter solution has been typically employed in engineering research labs, we see it enter the computational office environment to aid in its transformation to sensor- and projection-rich space for immersive reality research.
Some recently benchmarked computational facilities are limiting the amount of dedicated, highly-specialized spaces like server farms, electronics shops, and wet labs constructed within new buildings. Many campuses are leveraging their existing data centers and encouraging research collaborations between the computer science departments with engineering and humanities to bolster the use of shared facilities like prototyping, robotics, and virtual and augmented reality labs. As an example, Boston University’s Center for Computing and Data Sciences (programmed by HGA) purposefully delivers just that—a “basic CS building.”
On the opposite end of the spectrum is the idea that new buildings should incorporate unique spaces that cannot be accommodated elsewhere on campus through renovation, and they should foster thematic research with select faculty from multiple departments. A prime example is ultra-quiet high-performance basement space for quantum computing research, typically done in collaboration with physics and material science faculty, as part of the design for Michigan State University’s Engineering and Digital Innovation Center.
As computing and data sciences become more embedded across disciplines and projects, it is increasingly important to consider spaces that facilitate collaboration in all its modes: planned and unplanned encounters, team meetings, building events, campus-wide and public seminars, job fairs and industry collaborations.
One approach to creating transdisciplinary learning and research communities is to co-locate education and administrative spaces with collaboration and research spaces for thematic research—for example, Smart Prosthetics—bringing together undergraduate students and graduate researchers from biology, medicine, engineering, and CS. Vibrant collaborative hubs facilitate connections and collaboration on projects across disciplines for both students and faculty.
Many institutions have been implementing a “hub and spoke” concept between computer and data science buildings and computing nodes in other buildings on campus—physically and virtually. Faculty and researchers that may primarily reside in the main hub often work closely with research teams in each node and need an inviting homeaway-from-home for day visits.
It is paramount to foster micro and macro connections within each building and across campus to maximize the impact of computer science on the mission of the institution.
Design features intended to increase collaboration include:
• A central atrium with a series of terraced platforms, which accommodates both public events and intimate interactions.
• Whiteboard walls that encourage doodling and provide space to turn casual conversation into project ideas.
• Main circulation paths dotted with small gathering spaces to encourage spontaneous meetups.
• Outdoor spaces to meet, exhibit work, and connect with nature.
Public art installations can link physical and digital science in inspiring ways, engage the larger community, and showcase innovation and student success throughout campus. Incorporating science, art, and architecture strengthens the vision for the building, and highlights the activities within at multiple levels.
We often participate in the selection of the artist and work with them to integrate their art. Responsive, sensory, or web-interaction-enabled pieces draw public engagement to the building and often showcase the science within.
“GeNeSiS - A Primordial Alphabet Soup” by Erwin Redl at the University of Rhode Island’s Center for Chemical and Forensic Science consists of 480 LED light panels suspended in three layers. It uses the symbols of the periodic table as building blocks for interactive poetry. Users can submit their pieces through the project’s website.
The COVID-19 pandemic demonstrated that remote and asynchronous teaching and learning are not only possible but may be a way to meet the overwhelming demand for computer science courses and degrees. UC Berkeley is considering making CS courses within their core curriculum fully automated, online, and scalable for 1,000 to 10,000 students. A Dartmouth College CS professor added:
“Undergraduate education will likely change [after COVID]. Online instruction has no physical space limitations. Class enrollment can be increased, allowing more students to access popular courses instead of being turned away because of classroom size. There is a growing interest in online options for all classes. The challenge will be how to effectively teach in a hybrid mode. This has big implications for space and classroom technology.”
Many faculty expressed interest in continuing to work from home a few days per week. This prompted a discussion about eliminating private offices to avoid underutilization when there is a rising concern about revenue and real estate costs in higher education.
By offering online learning opportunities, institutions can expand their offerings and reach more potential students, addressing the growing needs of the workforce for CS graduates. However, it calls into question how to provide opportunities for collaboration and hands-on learning. Balancing online participation with on-campus presence will be critical for transdisciplinary programs.
Predictions about the future of computing and data science are challenging to make beyond the next few years. Data analytics, selfgoverning machines, extended reality, and other computing fields all change the skill set needed in the workforce in tech and non-tech sectors.
Computer and data sciences are embedded in all aspects of academia, government, and industry. Their impact will continue to transform every discipline and sector of society and highlight the need to equip the future workforce with hard and soft skills for success.
For higher education institutions, it is important to continue to invest in technology-rich, flexible spaces that can adapt to rapidly evolving teaching methodologies and student enrollment numbers. Parallel investment in the growth of transdisciplinary research, both in the computer laboratory and the high-performance specialty labs for quantum and material science, is needed to recruit and retain faculty in cutting-edge research inquiries. Finally, providing warm and inviting collaboration spaces of various scales is key to the maturation of a close-knit network of innovative minds and the success of the program and institution.
Artificial Intelligence (AI):
The study of “intelligent agents” – devices capable of perceiving their environment and taking actions that maximize the chance of successfully achieving their goals.
Augmented Reality (AR):
Technology that superimposes a computer-generated image on a user’s view of the real world, thus providing a composite view.
Computational Science:
Encompasses the invention, implementation, testing, and application of algorithms and software used to solve large-scale scientific and engineering problems.
Computer Science (CS):
The study of computation, information, and automation.
Computing:
Any goal-oriented activity requiring, benefiting from, or creating computing machinery. It includes the study of algorithmic processes and development of both hardware and software. It has scientific, engineering, mathematical, technological, and social aspects. Major computing fields include computer engineering, computer science, cybersecurity, data science information systems, information technology, and software engineering.
Data Science:
An interdisciplinary field that uses algorithms, procedures, and processes to examine large amounts of data in order to uncover hidden patterns, generate insights, and direct decision-making. Data scientists use advanced machine learning algorithms to sort through, organize, and learn from structured and unstructured data.
Extended Reality (XR):
Collective term that refers to immersive technologies including virtual reality (VR), augmented reality (AR), and mixed reality (MR).
Internet of Things (IoT):
Devices with sensors, processing ability, and software technologies that connect and exchange data with other devices and systems over the internet or other communication networks
Machine Learning (ML):
The use and development of computer systems that are able to learn and adapt without following explicit instructions, by using algorithms and statistical models to analyze and draw inferences from patterns in data.
Mixed Reality (MR):
The merging of real-world and computer-generated environments. Physical and virtual objects may co-exist in mixed reality environments and interact in real time.
Transdisciplinary:
Transdisciplinarity integrates the natural, social and health sciences in a humanities context, and transcends their traditional boundaries.
Virtual Reality (VR):
A simulated experience that employs pose tracking and 3D near-eye displays to give the user an immersive feel of a virtual world.