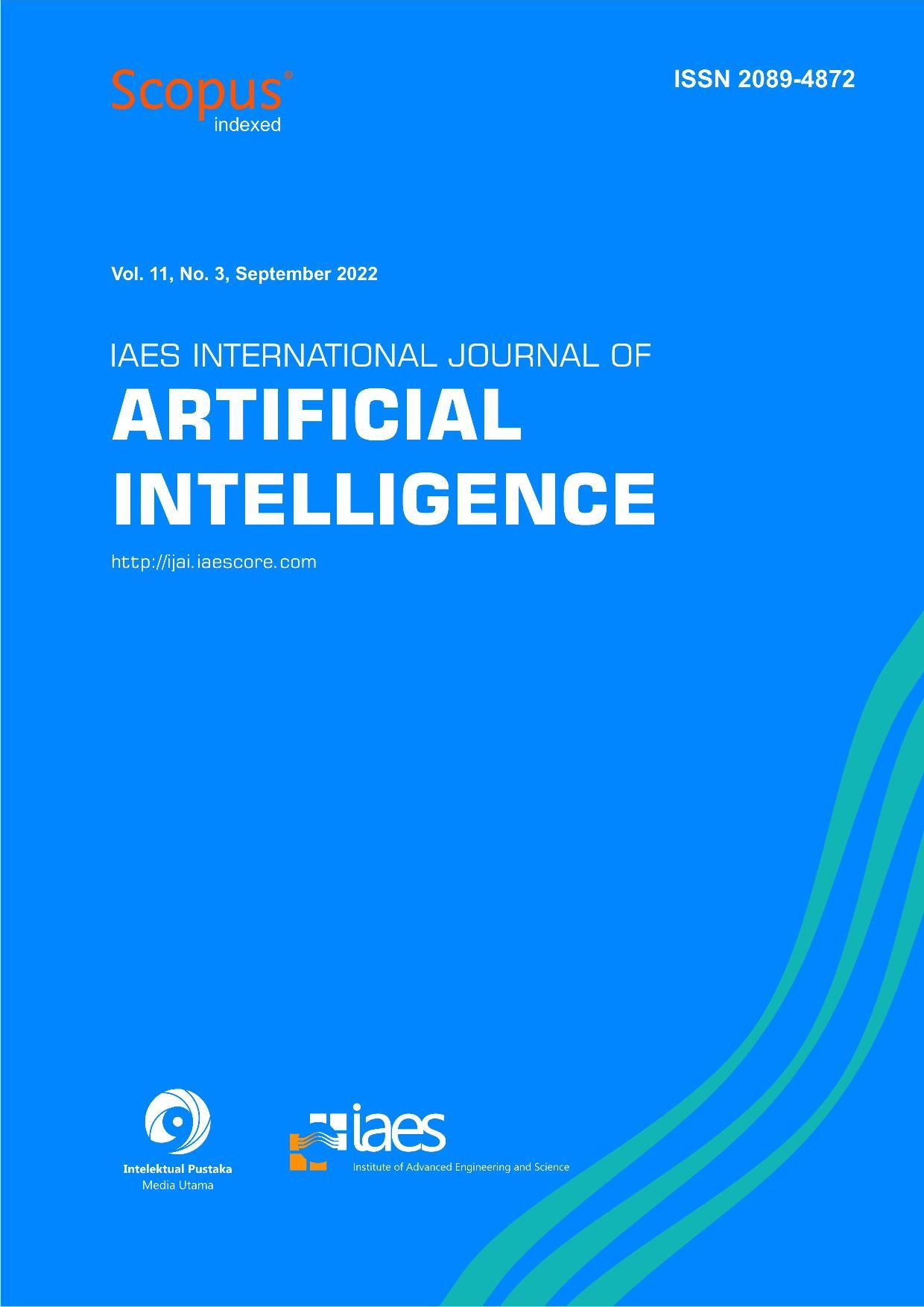
Journal of
Nurain Zulaikha Husin, Muhammad Zaini Ahmad, Mustafa Mamat 819 825
Deep learning based modeling of second hand ship prices in South Korea Changro Lee, Keyho Park 886 894
A smart traffic light using a microcontroller based on the fuzzy logic Desmira, Mustofa Abi Hamid, Norazhar Abu Bakar, Muhammad Nurtanto, Sunardi 809 818
Tiara Intana Sari, Zalfa Natania Ardilla, Nur Hayatin, Ruhaila Maskat 895 904
Bio inspired and deep learning approach for cerebral aneurysms prediction in healthcare environment Srividhya Srinivasa Raghavan, Arunachalam Arunachalam 872 877
Brainstorm on artificial intelligence applications and evaluation of their commercial impact Elvezia Maria Cepolina, Francesco Cepolina, Guido Ferla 799 808
Troop camouflage detection based on deep action learning
Four inputs one output fuzzy logic system for washing machine
Mohammed El Alaoui, Mohamed Ettaouil 916 922
(Continued on next page)
Microstrip antenna optimization using evolutionary algorithms Kalpa Ranjan Behera, Surender Reddy Salkuti 836 842
Graph transformer for cross lingual plagiarism detection Oumaima Hourrane, El Habib Benlahmar 905 915
Responsibility of the contents rests upon the authors and not upon the publisher or editors.
International IJ-AI Intelligence
Artificial
IJAI Vol. 11 No. 3 pp. 799-1196 September 2022 ISSN 2252-8938
Integrating singular spectrum analysis and nonlinear autoregressive neural network for stock price forecasting Asmaa Y. Fathi, Ihab A. El Khodary, Muhammad Saafan 851 858
Muslikhin, Aris Nasuha, Fatchul Arifin, Suprapto, Anggun Winursito 859 871
Abusive comment identification on Indonesian social media data using hybrid deep learning
Deep learning intrusion detection system for mobile ad hoc networks against flooding attacks Oussama Sbai, Mohamed Elboukhari 878 885
Optimization of agricultural product storage using real coded genetic algorithm based on sub population determination Wayan Firdaus Mahmudy, Nindynar Rikatsih, Syafrial 826 835
A novel evolutionary optimization algorithm based solution approach for portfolio selection problem Mohammad Shahid, Mohd Shamim, Zubair Ashraf, Mohd Shamim Ansari 843 850
A new approach to solve the of maximum constraint satisfaction problem
Youssef Mnaoui, Aouatif Najoua, Hassan Ouajji 986 994
Coastal forest cover change detection using satellite images and convolutional neural networks in Vietnam Khanh Nguyen Trong, Hoa Tran Xuan 930 938
The feature extraction for classifying words on social media with the Naïve Bayes algorithm
A deep learning approach based defect visualization in pulsed thermography
Machine learning modeling of power delivery networks with varying decoupling capacitors
Hypergraph convolutional neural network based clustering technique
Sethu Selvi Selvan, Sharath Delanthabettu, Menaka Murugesan, Venkatraman Balasubramaniam, Sathvik Udupa, Tanvi Khandelwal, Touqeer Mulla, Varun Ittigi 949 960
The prediction of the oxygen content of the flue gas in a gas fired boiler system using neural networks and random forest Nazrul Effendy, Eko David Kurniawan, Kenny Dwiantoro, Agus Arif, Nidlom Muddin 923 929
Erliza Yuniarti, Siti Nurmaini, Bhakti Yudho Suprapto 1026 1032
Pipe leakage detection system with artificial neural network
Binary spider monkey algorithm approach for optimal siting of the phasor measurement for power system state estimation Suresh Babu Palepu, Manubolu Damodar Reddy 1033 1040
Muhammad Iqmmal Rezzwan Radzman, Abd Kadir Mahamad, Siti Zarina Mohd Muji, Sharifah Saon, Mohd Anuaruddin Ahmadon, Shingo Yamaguchi, Muhammad Ikhsan Setiawan 977 985
Artificial intelligence in a communication system for air traffic controllers' emergency training
Smart power consumption forecast model with optimized weighted average ensemble Alexander N. Ndife, Wattanapong Rakwichian, Paisarn Muneesawang, Yodthong Mensin 1004 1018
Return on investment framework for profitable crop recommendation system by using optimized multilayer perceptron regressor Surekha Janrao, Deven Shah 969 976
Asraa Safaa Ahmed, Zainab Kadhm Obeas, Batool Abd Alhade, Refed Adnan Jaleel 939 948
Defense against adversarial attacks on deep convolutional neural networks through nonlocal denoising Sandhya Aneja, Nagender Aneja, Pg Emeroylariffion Abas, Abdul Ghani Naim 961 968
Yeong Kang Liew, Nur Syazreen Ahmad, Azniza Abd Aziz, Patrick Goh 1049 1056
A text mining and topic modeling based bibliometric exploration of information science research Tipawan Silwattananusarn, Pachisa Kulkanjanapiban 1057 1065
Hardware sales forecasting using clustering and machine learning approach Rani Puspita, Lili Ayu Wulandhari 1074 1084
A real time quantum conscious multimodal option mining framework using deep learning Jamuna S. Murthy, Siddesh Gaddadevara Matt, Sri Krishna H. Venkatesh, Kedarnath R. Gubbi 1019 1025 Indonesian load prediction estimation using long short term memory
Loc H. Tran, Nguyen Trinh, Linh H. Tran 995 1003
Arif Ridho Lubis, Mahyuddin Khairuddin Matyuso Nasution, Opim Salim Sitompul, Elviawaty Muisa Zamzami 1041 1048
Improving prediction of plant disease using k-efficient clustering and classification algorithms
An empirical study on machine learning algorithms for heart disease prediction Tsehay Admassu Assegie, Prasanna Kumar Rangarajan, Napa Komal Kumar, Dhamodaran Vigneswari 1066 1073
Machine learning of tax avoidance detection based on hybrid metaheuristics algorithms
Hamdani Hamdani, Heliza Rahmania Hatta, Novianti Puspitasari, Anindita Septiarini, Henderi 1119 1129
A new hybrid and optimized algorithm for drivers’ drowsiness detection Mouad Elmouzoun Elidrissi, Elmaati Essoukaki, Lhoucine Ben Taleb, Azeddine Mouhsen, Mohammed Harmouchi 1101 1107
Depression prediction using machine learning: a review Hanis Diyana Abdul Rahimapandi, Ruhaila Maskat, Ramli Musa, Norizah Ardi 1108 1118
Vigneshwaran Pandi, Prasath Nithiyanandam, Sindhuja Manickavasagam, Islabudeen Mohamed Meerasha, Ragaventhiran Jaganathan, Muthu Kumar Balasubramanian 1085 1093
Semi supervised approach for detecting distributed denial of service in SD honeypot network environment
A linear regression approach to predicting salaries with visualizations of job vacancies: a case study of Jobstreet Malaysia Khyrina Airin Fariza Abu Samah, Nurqueen Sayang Dinnie Wirakarnain, Raseeda Hamzah, Nor Aiza Moketar, Lala Septem Riza, Zainab Othman 1130 1142
Dengue classification method using support vector machines and cross validation techniques
A machine learning approach for Bengali handwritten vowel character recognition Shahrukh Ahsan, Shah Tarik Nawaz, Talha Bin Sarwar, M. Saef Ullah Miah, Abhijit Bhowmik 1143 1152
Fauzi Dwi Setiawan Sumadi, Christian Sri Kusuma Aditya, Ahmad Akbar Maulana, Syaifuddin, Vera Suryani 1094 1100
Multi objective optimization path planning with moving target Baraa M. Abed, Wesam M. Jasim 1184 1196
Suraya Masrom, Rahayu Abdul Rahman, Masurah Mohamad, Abdullah Sani Abd Rahman, Norhayati Baharun 1153 1163
A proposed model for diabetes mellitus classification using coyote optimization algorithm and least squares support vector machine Baydaa Sulaiman Bahnam, Suhair Abd Dawwod 1164 1174
Features analysis of internet traffic classification using interpretable machine learning models Erick A. Adje, Vinasetan Ratheil Houndji, Michel Dossou 1175 1183
A comprehensive analysis of consumer decisions on Twitter dataset using machine learning algorithms
1. INTRODUCTION
Brainstorm on artificial intelligence applications and evaluation of their commercial impact
Article history: Received Oct 26, 2021 Revised Apr 14, 2022 Accepted May 13, 2022 A countless number of artificial intelligence applications exist in a wide range of fields. The artificial intelligence (AI) technology is becoming mature, free powerful libraries enable programmers to generate new apps using a few lines of code. The study identifies the applications that are the most interesting for a developer as far as profit is concerned. Some AI applications related to trading, industry, sales, logistics, games, and personal services have been considered. To select the most promising AI applications, multi criteria methods have been adopted. This brainstorm may be useful to inspire new born start ups, willing to create viral apps/products. The paper wishes to be informative and light, for further information, a rich selection of publications and books is provided.
This is an open access article under the CC BY SA license. Corresponding Author: Elvezia Maria Cepolina Department of Political Sciences, University of Genoa Piazzale E. Brignole 3A 16123 Genoa (GE), Italy Email: elvezia.maria.cepolina@unige.it
John invented the first programming language for symbolic computation lisp. AI may be considered, in general, as a black box able to solve problems; it produces specific results from an input. The Antikythera Mechanism is probably the first computer: it is a 2000 year old mechanism that looks like a Swiss watch with gears, it is supposed to be a greek astrological mechanic calendar. It took several years of technology development before it was possible to use these architectures successfully. In an early period between the 1960s and 1970s, knowledge based systems (KBS) and artificial neural network (ANN) systems were developed as AI systems. The former proposes solutions by processing predefined rules that have been set by humans, based on their experience; examples of knowledge based systems include expert systems, which are so called because of their reliance on human expertise. The latter are black boxes that are trained with a large amount of input/output data. The limited availability of data made AI applications scarce in this period. Only since the 1980s did the use of AI start to grow: the increasing availability of data enabled the development of machine learning: the computer accesses large amounts of data and extracts knowledge from it to solve specific problems. Automatic machine learning is a subcategory of AI that differs significantly from previous techniques that saw humans as teachers of the computer. Today this technology is mature, at least for the following reasons: data storage systems are relatively inexpensive, millions of sensors are recording daily
Journal homepage: http://ijai.iaescore.com
Elvezia Maria Cepolina1, Francesco Cepolina2, Guido Ferla3 1Department of Political Sciences, University of Genoa, Genoa, Italy 2Department of Mechanical Engineering, University of Genoa, Genoa, Italy 3University of Camerino, Camerino, Italy
IAES International Journal of Artificial Intelligence (IJ AI) Vol. 11, No. 3, September 2022, pp. 799 808 ISSN: 2252 8938, DOI: 10.11591/ijai.v11.i3.pp799 808 799
Keywords: Artificial IndustrialmonetizingAcommercialAmethodapplicationsintelligenceclassificationrtificialintelligenceappsrtificialintelligenceartificialintelligence
The term artificial intelligence (AI) was coined by John McCarthy in the mid 1950s, with the following meaning the science and engineering of making intelligent machines. John worked on some of the world’s most innovative technologies such as: programming languages, the Internet, the web, and robots.
Article Info ABSTRACT
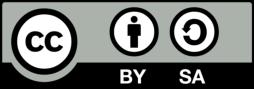
The potential value of AI in different sectors has been analysed and compared by a McKinsey study [3]. The study indicates that AI could potentially create from $3.5 trillion to $5.8 trillion in annual value in the global economy. To achieve the benefits, however, several limitations and barriers to the application of AI must be overcome. For instance, a large volume and variety of often labelled training data are needed. The value of AI is not in the models themselves, but in the ability of organisations to exploit them. Business leaders will need to prioritise and make careful choices about how, when, and where to implement them. The study by McKinsey identifies the sectors where AI could have the greatest impact on revenues. The work presented in the present article has a different point of view. The research wants to be of support for those who develop AI applications to choose the market and the applications where there is the highest potential.
ii) Definition of the judgement criteria: they represent the tool by which the achievement of a final objective is measured; identification of the weights of the judgement criteria in relation to the final objective; the goal is to maximise the profitability of application developers in the AI field.
Non compensative: they do not allow compensation between the effects of an alternative on different criteria of judgement. The methods of concordance analysis belong to this group and among them there are the methods of the electre family. The electre I method was applied in the research.
2. THE THEORETICAL BASIS
“big data” to analyse, in the World about 3.8 billion of people use smartphones (high end computers), almost all devices are interconnected (internet of things), computational power is fast (NVIDIA A100 Tensor Core GPU). Any calculator or computer is capable of AI. Python is one of the best solutions to create ambitious big size AI projects. There is a rich online python community ready to support the developers. Several works introduce the basic concepts of AI applications [1], [2].
The paper is structured: in section 2 the theoretical basis of multi criteria analysis is presented. In section 3, the process for the identification of the project alternatives is described. Section 4 refers to the definition of the judgement criteria. Section 5 presents the evaluation matrix and section 6 presents the results of the adopted multi criteria analysis. The results from the proposed methodology are compared with the results from the McKinsey study. Conclusions follows.
The aim of the research is to look for contexts in which AI application developers can achieve the greatest commercial impact. AI applications are born to solve different needs, from different fields: it is not trivial to compare and select the most promising ideas. When make it necessary to use criteria that are to a large extent non monetizable, as it is in the proposed research, it is necessary to resort to the set of procedures that go by the name of multi criteria analysis. The stages of the multi criteria analysis can be summarised as:
ISSN: 2252 8938 Int J Artif Intell, Vol. 11, No. 3, September 2022: 799 808 800creating
The AHP method structures the decision making process at several levels. In the present study, as shown in Figure 1, three levels have been considered: at the highest level we have the final objective, i.e., maximising the profit of developers of artificial intelligence applications, at the intermediate level the judgment criteria and at the lowest level the design alternatives.
Compensatory: they allow compensation between the effects of a project in relation to different criteria. To the first group belong the multi attribute utility theory (MAUT) and the analytic hierarchy process (AHP). The latter method was applied in the research.
The method aims to establish a weight for each alternative in relation to the final objective, and then a ranking of the alternatives. The weight (pko) of the alternative k in relation to the final objective o is given by the linear combination of the weights (eki) of the alternative k with respect to the judgment criteria i (for all the judgment criteria i=1..n) whose coefficients are, in turn, the weights (io ) of the judgment criteria i with respect to the final objective o
iii) Definition of the evaluation matrix whose elements represent the scores by which the effects of the alternatives are measured against each judgment criterion.
iv) Comparison of alternatives and final choice. Precisely, based on the way in which the alternatives are compared to assess their effect in relation to the final objective, the methods of multi criteria analysis are divided into two fundamental classes:
������ =∑������������ �� ��=1
i) Identification of the project alternatives.
In this way the column vector of weights of the alternatives with respect to the final objective [pA0, pBO,..,pKO] is obtained as the product of the evaluation matrix [eA1, eA2,..,eAn; eB1, eB2,..,eBn; ; eK1, eK2,..,eKn ] and the column vector of weights of the judgement criteria with respect to the final objective [10, 2o,.., no].
Brainstorm on artificial intelligence applications and evaluation of … (Elvezia Maria Cepolina) 801
g10 gn0 g20 ek1 ekn eki gi0 å = = n i kokiiope 1 g
Int J Artif Intell ISSN: 2252 8938
Finalobjective:O
i
n A B
The key aspect of concordance analysis is that alternatives are compared two by two. The comparison is based on the evaluation matrix the elements of which have been normalised and made directional and the weights of the judgment criteria in relation to the final objective. This pairwise comparison then allows the calculation of concordance and discordance indices. The first is a measure of the dominance of alternative x over alternative y, the second is an expression of the superiority of y over x. The two indices are calculated according to: ������ = ∑ �� ���� ∈������ ∑ �� ���� ; ������ = ������ ��∈������(����|������ ������|) ������ �� (����|������ ������|) where ������ e ������ are the sets defined as: ������ ={��|������ ≥������} ������ ={��|������ <������} and where ������is the generic, normalized, directional element of the evaluation matrix. It can be observed that the degree of concordance for each pair of alternative projects is a ratio between weights only, while the degree of discordance also considers the differences between the scores of the two compared pairs. From an interpretative point of view, it can be said that the degree of concordance expresses a weighted proportion of the criteria favourable to x with respect to y. The degree of discordance, on the other hand, expresses a proportion of the better propensity to implement y rather than to implement x. However, several other interpretations can be given. The electre I method aims at skimming the alternatives, trying to identify a subset of alternatives that are considered unacceptable because they are “inferior” to the others as show in Figure 2. The method does not, however, aim to rank the acceptable alternatives. In order to divide the set B of alternatives into the two complementary subsets (A and U in Figure 2), the analyst chooses two thresholds: and ; both values must be between 0 and 1; closer to zero, closer to 1. The indexes of concordance and discordance of the alternatives are compared with these threshold values: if, given a pair (x,y) of alternatives, it turns out at the same time (*){������ ≥�� ������ ≤�� there are no elements to assert that x is worse than y. Alternatives:A,B, K Judgmentcriteria:1 n1 2 4 K
Figure 1. Outline of the AHP methodology
3. METHOD: THE IDENTIFICATION OF THE PROJECT ALTERNATIVES
The process flow, used to define and select the AI applications, is subdivided in four main steps. The first phase is creative, free, open, and unconstrained; a pool of multidisciplinary researchers, thanks to different sessions of brainstorming, looks for potential customer needs. The customer satisfaction is the main goal. During this phase, any idea can be proposed and analysed. Then, one or more AI products are proposed to solve each customer need. This process is not always straight forward; some general customer needs, like “people like to have more spare time”, deserve more elaboration time to be fulfilled. The same need may be satisfied using a cluster of AI integrated applications. The brainstorming phase ends with a preliminary feasibility study; in this early stage, a first attempt is made to identify the potential of each application. This early feasibility study acts as a filter; only the applications that show a limited potential are rejected.
ISSN: 2252 8938 Int J Artif Intell, Vol. 11, No. 3, September 2022: 799 808 802 (**) {������ ≥�� ������ ≤��
It is somehow complex to create a method able to identify and select the best AI project alternative; while the brainstorming phase needs to be “free” to unlock the creativity, the evaluation phase avails of a “rigid” standardized judgment criteria, necessary to provide an objective evaluation. The evaluation is performed on a few general key parameters that can be applied on each idea proposed. For a better understanding, a flowchart of the methodology adopted is provided as shown in Figure 3.
It is now time to give a more refined shape to the ideas proposed. The second step of the process flow consists in classifying and cluster the customers by field of interest. The methodology allows to enucleate 6 fields of interest (in blue): trading, industry, sales, logistics, games, personal services. Each field of interest identifies a specific customer; the identification and the deep knowledge of each customer is crucial, to better serve him. Then, one or more specific applications are defined, tailored on the customer wishes: the active game application is born out of a desire to satisfy children’s need to play exciting games, while the virtual volunteer application is born out of a desire to satisfy elder people wish to share emotions.
If, on the contrary, both (*) are satisfied and at least one of the (**) is not verified, we can assert that project x is superior to project y. By examining in this way all the pairs of alternatives, it is possible to partition the set B into the two subsets: A is formed by the alternatives for which it is not possible to identify the superiority of one alternative over another of the same subset. The complementary subset U is formed by the alternatives for which at least one higher ranking alternative belonging to A, has been identified. The electre I method thus makes it possible to establish that the alternatives in A are better than the alternatives in U, but it does not make it possible to compare the alternatives in A with each other. If the set A consists of only one alternative, this is the best alternative. If, on the other hand, A contains several alternatives, it is possible to reduce the number of alternatives in A by modifying the weights attributed to the judgement criteria and/or by bringing the values of the thresholds and closer together. In the proposed research, the comparison of alternatives is carried out using the AHP methodology. Subsequently, the electre I method has been also implemented. The aim is to check whether it is possible to make only one alternative remain in the set A by varying the values of the and thresholds. If it is possible, then it can be stated that, all the other alternatives can be considered unacceptable because they are “inferior” to the one in the A set. The claim would be reliable as it has been shown that the electre method has lower sensitivity than the AHP method to changes in the weights [4]. The following paragraphs refer to the 4 stages of the multi criteria analysis and describe how each phase was approached in the study. Figure 2. The set B of alternatives is divided into two subsets: A and the complementary set U=B/A
Overall, 22 AI applications (in the green boxes of Figure 3) are proposed. AI may be applied to almost any field; the list of proposed applications is described in Table 1 (see in appendix) and does not want to be exhaustive.
B A U
4. METHOD: THE DEFINITION OF THE JUDGEMENT CRITERIA
The 2nd evaluation matrix is reported in Table 2. It has as many rows as there are the alternatives (AI projects) and as many columns as there are the judgement criteria (four). The generic element represents the normalised and directional weight of the alternative corresponding to the row, in relation to the judgement criteria corresponding to the column. In Figure 4 each alternative is represented by a column. The total height of the column represents the total weight assigned to the alternative and results from the sum of the weights as shown in Table 2 of the alternative corresponding to the four judgement criteria (the contributions of the weights to the four judgement criteria are represented with different shades of colour).
5. METHOD: THE DEFINITION OF THE EVALUATION MATRIX
Brainstorm on artificial intelligence applications and evaluation of … (Elvezia Maria Cepolina) 3. Shows the flowchart of the AI based models and experimental methods applied
A multidisciplinary panel of experts, from the trading, industry, sales, logistics, games, personal services macro areas, has been created. The experts have selected 4 criteria of judgement: Distribution, Profitability, Independence and Simplicity. Distribution refers to the number of clients that potentially may use the service and is measured as potential number of clients (N clients). Profitability is assessed as the potential earning per unit of product installed/sold (€/unit). Independence (from hardware) is the ability of the code to run, for example on mobile phones, without the need of dedicated hardware. Simplicity (of development) is inversely proportional to the time necessary to develop and maintain the code. These judgement criteria are represented as 4 blue spheres in the funnel in the fourth phase in Figure 3. As it concerns the identification of the weights (io) of the judgment criteria with respect to the final objective, it was assumed that all criteria have an impact to the same extent on maximising the profit of developers of AI applications. This assumption is affected by a degree of discretion. To reduce this discretion, it is planned to apply, in the future, the technique of indirect quantitative determination, using comparison matrices.
Int J Artif Intell ISSN: 2252 8938
803 Figure
The experts have filled in a 1st evaluation matrix, which has as many rows as are the alternative projects and as many columns as are the judgement criteria. Each value of the first matrix represents a measure of how well the project (corresponding to the row) meets the criterion (corresponding to the column). Each value of the 1st matrix is a score ranging from 1 to 5 (stars). The value is determined using the Delphi method: the experts have been iteratively interviewed, until they converge on a single value for each assessment.Each value is an ordinal measure, meaning that indicates the position of the project in the ranking list. The assessed values have been elaborated, to have a 2nd evaluation matrix containing cardinal, normalised and directional quantities. Given xij as the “raw” score that in the 1st evaluation matrix expresses the correspondence of alternative i to criterion j, the relative normalised value eij was obtained with: ������ = ������ ������ �� (������)
Table 2. The 2nd evaluation matrix of the proposed AI applications
ISSN: 2252 8938 Int J Artif Intell, Vol. 11, No. 3, September 2022: 799 808 804
AI Project Distribution(NClients) Profitability (€/unit) (fromIndependencehardware)
According to the AHP method, a final score is assessed for each project through the weighted summation method. The outputs of the AHP method show that the most promising ideas are: ‘personal gift’, ‘car sale’, ‘shop’, ‘online product orders’ (belonging to the sales macro area) and ‘elderly care’. Therefore, the sales macro area is the most profitable for AI application developers. Interestingly, according to Kinsey’s research [3], marketing and sales are one of the two macro areas where the potential impact of AI is greatest. The other macro area identified by the McKinsey researchers is the area of supply chain management and production. However, this macro area does not seem to be as profitable for AI developers as it is for AI users. The reason might be that (at least for Industry 4.0, considered in our study), the customisation of specific AI applications, required by different factories, and the difficult integration of sensors into complex systems, which provide the required big data, make the development of AI applications in this area time consuming and not so profitable. Each factory is particular
Plugin 0.4 1 0.4 0.4 Automated trading 0.2 0.8 0.6 0.2 Industry 4.0 0.4 0.8 0.4 0.4 Photo retouch 1 0.4 0.6 0.8 Car sales 1 0.6 0.8 0.8 Supermarket 1 0.8 0.6 0.6 Shop 1 0.8 0.8 0.6 Online orders 1 0.8 0.6 0.8 Personal gift 1 0.8 0.8 0.8 Warehouse 0.4 0.8 0.6 0.8 Transport 0.4 0.6 0.4 0.6 Delivery 0.6 0.6 0.8 0.8 Tales teller 0.8 0.4 0.6 0.6 Active games 0.8 0. 0.2 0.6 TV programs 1 0.4 0.8 0.6 Active calendar 0.6 0.4 0.8 0.6 News aggregator 0.6 0.4 0.4 0.2 PC archive 0.8 0.6 0.6 0.6 Smart watch 0.6 0.4 0.2 0.6 Elder care 0.8 0.6 0.8 0.8 Virtual volunteer 0.4 0.6 0.4 0.2 Medical file 0.8 0.8 0.6 0.2
Figure 4. A comparison, based on the AHP method, among the proposed AI applications
The brainstorming feasibility study, driven by the feelings/experience of the researchers, allowed to preliminary filter the ideas. The comparison of the applications, based on the 4 judgement criteria, allows now to select the most promising AI applications. This step is the last one in Figure 3 and it is represented as a funnel. The comparison of the applications has been performed first, by applying the AHP method secondly, by applying the electre I method for comparing the best projects resulting from the AHP analysis.
6. THE COMPARISON OF ALTERNATIVES: RESULTS AND DISCUSSION
Simplicity (of development)
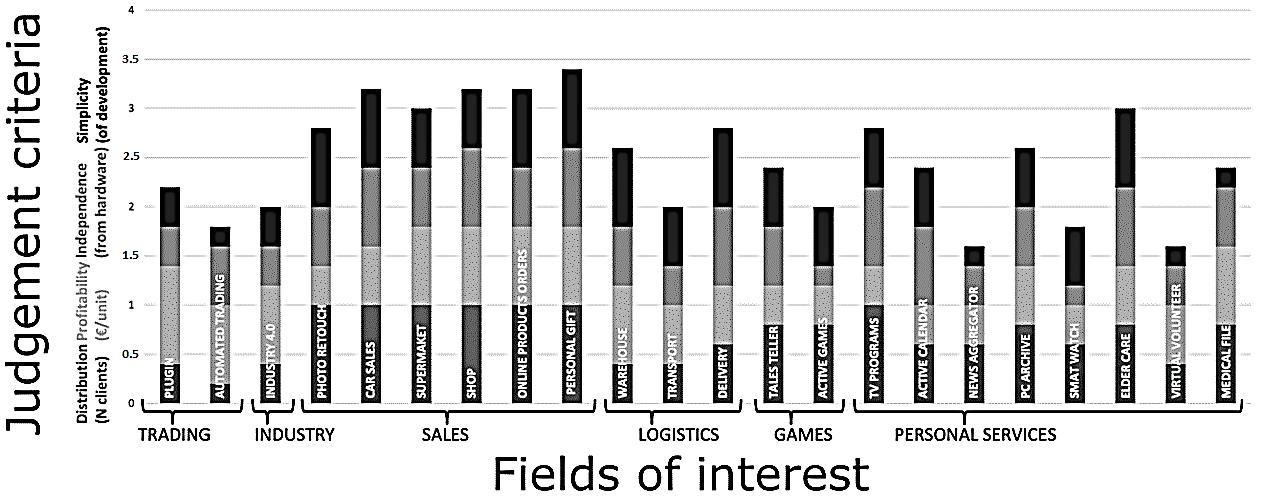
Int J Artif Intell ISSN: 2252 8938
The electre I method has been applied to compare the projects ‘personal gift’, ‘car sale’, ‘shop’, and ‘online product orders’. These projects are in the sales macro area that resulted the more promising one from the AHP analysis. Having fixed =0,5 and =0,55 as threshold values, the project ‘personal gift’ resulted the only one in the A set, where the set A is made up of the alternatives in respect of which no other project has been found to be superior as shown in Figure 2. All the other alternatives belong to the U set in Figure 2 and can therefore be considered “inferior” to the ‘personal gift’ project.
The ability of encapsulating bits of human intelligence inside a self standing “learning and thinking device” opens unlimited possibilities. Out of clichés and in the name of concreteness: data have value to the extent that they allow to improve the productivity of a company, when they allow it to become more efficient, to create and develop better products, to increase the satisfaction of own customers or even to enter new markets or develop new business models. AI allows you to increase these potentials and allows you to accelerate all the knowledge processes that lead to the achievement of these objectives and which are the basis of the self learning enterprise, a definition by which we mean those companies and organizations that decide to grow own knowledge potential and put it to value, available to the business. New software and hardware technology developments daily widen AI capabilities. A few examples of new AI applications have been described for the following fields: trading, industry, sales, logistics, games and personal services. The ideas proposed are only briefly described, with the aim of soliciting the imagination of the reader. Ideally, for any new ideas proposed, a start up may be created, able to financially exploit the specific “automated service”. Then, a methodology to compare the ideas has been introduced, based on four key parameters: distribution, profitability, independence and development. The best applications reach a wide public, have a high profitability, are independent from hardware and need limited coding time. An important goal of this brainstorm is to arouse the reader’s curiosity, leading him/her to find and solve existing problems, thanks to the AI powerful tools today available. On a daily basis, new start ups create AI apps that run on our smartphones Worldwide. The proposed brainstorm, on commercial AI applications, may help today’s CEO, selecting the next commercial digital products.
Figure 5. Shows the statistics distribution of the AI projects scores 7. CONCLUSION
Brainstorm on artificial intelligence applications and evaluation of … (Elvezia Maria Cepolina) 805 and relies on specific hardware, which is why the process of developing industrial applications offers only limited scalability.Thedatain the 2nd evaluation matrix can also be used to make some general considerations as shown in Figure 5. The highest scores were awarded in the criterion distribution: we live in a global connected world; the distribution of the AI applications is relatively easy. Profitability received lower scores: profitability in fact, depends heavily on the marketing strategy adopted; people often receive “almost free” professional digital services; hence profitability is not always straightforward. A persuasive marketing plan needs to be elaborated, to accomplish the “AI product sale”. Independence received slightly lower scores than profitability because not all the proposed AI applications can run directly on mobile applications; independence from hardware cannot always be guaranteed. The lowest scores were awarded in the simplicity criterion, because the time required for software development generally risks lowering the margins.
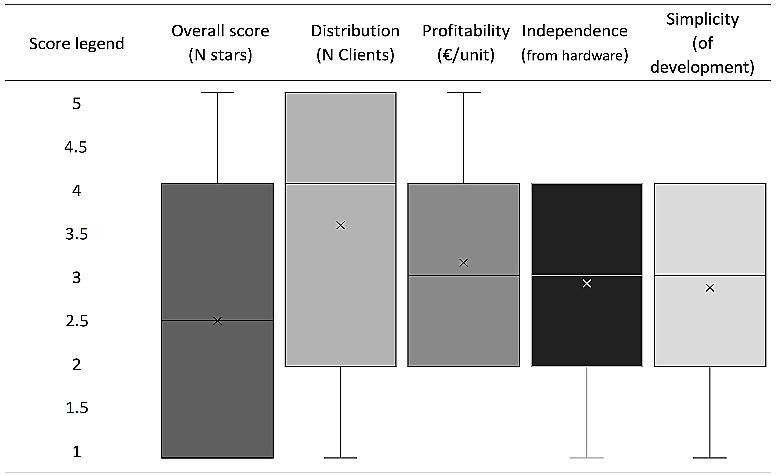
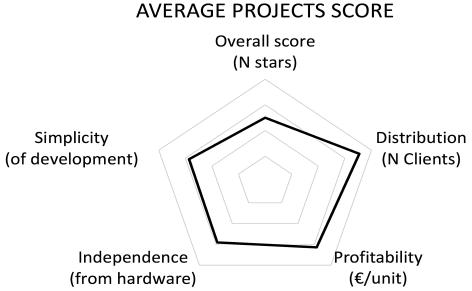
Table 1. The proposed applications: project alternatives
Field interestof alternativesProject Project description forecastsTrading Plugin The task is to give trading advice. For example, Metatrader® supports users giving real time forecasts and their probability to happen [5], [6] tradingAutomated AI platforms that are designed to work without human supervision [7]. For example, high frequency trading sends orders to the market with a milliseconds or microseconds frequency. Ethical problems issue: the AI, with the aim of chasing pure profit, may choose for us toxic financial strategies Industry Industry 4.0 An Industry 4.0 production environment allows a strong customization of products under the condition of high flexibility (mass) production [8] The sensors used for the production create a huge amount of data that needs to be evaluated. For example, Braincube® (braincube.com) is a commercial software that helps to select and tune the crucial production parameters. The machine learning creates a sort of mathematical model of the process, and sets the optimised production parameters, for the next batch of production. A predictive digital twin online process simulation is used to foresee and solve problems before they happen [9], [10] Sales retouchPhoto Modern fast communication is often spread using images, while the text is almost disappearing. Each photographer needs to give a special "appeal" to his photos, to beat the worldwide competition. AI may be used to create a self adaptive filter that first examines each photo, and then dynamically applies custom parameters [11] Car sales AI may be used to find an objective value for the cars on sale [12]. This service first asks all the characteristics of the car, and then provides a certified reference price for the sale in a specific country. This certification helps to gain confidence, both for the seller and for the buyer. Supermarket AI may help to drastically reduce food waste. AI can dynamically change the price according to deadlines/offers [13]. It can also predict all the goods that will never be sold in time and organise a charity donation to the poor people. Moreover, AI can help to optimise supplies, to eliminate unsold and expired products. Shop AI can also push the customer experience at the next level [14]. AI will analyse the purchases of each customer. Valuable customers will receive better discounts, oriented to the goods they buy more often. A mobile phone app may be the personal interface to build the loyalty between AI and the customer. AI may also help to easily find the goods inside a shop; two approaches can be followed: change of merchandise location or change the shop layout. AI may simulate and optimise the movements inside the shop. ordersOnline A commercial AI plugin may help to select products, according to customers' tastes; eBay browser will show for “sunglasses”, first the model of sunglasses you like most. giftPersonal The AI advisor needs to know the gender, age, and personal taste of the guest of honour. The advisor then, according to the given budget, will propose a shortlist of gifts [15] logistics Warehouse The task could be managing the space to optimise product picking [16]. AI automatic filling algorithm may save space inside a moulds warehouse [17] Transport AI can be integrated with traffic detection systems, for forecasting traffic volumes and warning conditions, that anticipate road accidents. AI can improve collective transport. Autonomous vehicles can be connected, with the aim of increasing the accessibility of rural areas, reducing the number of accidents. AI can simplify the displacements of those who cannot use collective transport, and who do not have a driving licence. The travel can be planned from peak hours to off peak hours, where possible [18] Delivery The context could be proximity e commerce where the target is to satisfy customer preferences, allowing for instance last minute changes, in order lists and/or delivery addresses, minimising the travelled distance [19], [20] The target is to improve the complex multi commodity pick up and delivery travelling salesman problem, reducing the execution time [21] Games Tale teller The AI storyteller tells goodnight stores to the children [22]. Each story is different and is based on random or custom subjects. gamesActive "Active games'' are classic video games with real life outcomes [23]. The concept of "active games" can be applied both for children and for elder people. Game activities include gymnastics, memory games and phone calls to friends. AI teaches to the children the balanced entertainment mix among digital, social, and physical life.
PC active Sometimes people have many documents placed unsorted on their PC desktop; there is the risk of losing them. The PC archive service may automatically archive and find documents. Also, logical maps may be created [26]
ISSN: 2252 8938 Int J Artif Intell, Vol. 11, No. 3, September 2022: 799 808 806
Personalservices programsTV The dilemma is: "Is there anything interesting to watch tonight?". Instead of doing an endless zapping, AI may suggest specific programs, tied for the audience in front of the television [24] calendarActive "What to do today?". The digital AI calendar best fits the daily activities to do, according to weather conditions, family needs, and working needs. The calendar is also able to find and manage conflicting conditions.
aggregatorNews Often the news, found on the web, seems to be polarised. It is difficult, for the user, to develop his own point of view. Fake news is also not easy to discover [25]. The news aggregator is a “research bot”, like Google, that shows and weighs together different points of view on the same topic.
APPENDIX
Smart watch An elder person, that lives alone inside a house, creates safety concerns. Smart watches already can keep track of biometrics data such as: blood pressure, oxygenation and physical activity done [27]. This data may be real time monitored and actively used by AI algorithms to save the elder people life [28] Elder care An elder care AI platform, like Alexa [29], may offer a rich list of customised services. volunteerVirtual AI platforms allow volunteers to speak remotely, using a video conference app, such as Skype. Elder people may use their television as a video interface. This solution helps to optimise the transport time. A further step is to virtualize the volunteer: when the volunteer is not available, a virtual volunteer may replace him [26]
Medical file The classification of diseases is a classic task [30], [31]: for example, the LYNA algorithm, analysing medical images, can help to find tissue problems. Surgical training simulation is another rich medical field. AI can correlate the data of the patient with the data of millions of patients having a similar disease, offering a valuable clinical decision support system.
[16] E. Bottani and B. Franchi, “Optimizing picking operations in a distribution center of the large scale retail trade,” in Proceedings of the 23rd International Conference on Harbor, Maritime and Multimodal Logistic Modeling and Simulation, 2021, pp. 60 69., doi: 10.46354/i3m.2021.hms.008.
[20] F. Cepolina, E. M. Cepolina, and G. Ferla, “Exact and heuristic static routing algorithms for improving online grocery shopping logistics,” in Proceedings of the 23rd International Conference on Harbor, Maritime and Multimodal Logistic Modeling and Simulation, 2021, pp. 17 26., doi: 10.46354/i3m.2021.hms.003.
[23] A. De Gloria, F. Bellotti, and R. Berta, “Serious games for education and training,” in International Journal of Serious Games, Feb. 2014, vol. 1, no. 1., doi: 10.17083/ijsg.v1i1.11.
[14] A. Bruzzone, K. Sinelshchikov, M. Massei, and W. Schmidt, “Machine learning and genetic algorithms to improve strategic retail management,” 2021., doi: 10.46354/i3m.2021.mas.023.
[26] A. De Mauro, Big data analytics. Analyzing and interpreting data with machine learning (in Italia). 2019.
[21] O. I. R. Farisi, B. Setiyono, and R. I. Danandjojo, “A hybrid approach to multi depot multiple traveling salesman problem based on firefly algorithm and ant colony optimization,” IAES Int. J. Artif. Intell., vol. 10, no. 4, pp. 910 918, Dec. 2021, doi: 10.11591/ijai.v10.i4.pp910 918. [22] L. Baiheng and Z. Wen, “Rethinking of artificial intelligence storytelling of digital media,” in 2020 International Conference on Innovation Design and Digital Technology (ICIDDT), Dec. 2020, pp. 112 115., doi: 10.1109/ICIDDT52279.2020.00029.
[18] R. Abduljabbar, H. Dia, S. Liyanage, and S. A. Bagloee, “Applications of artificial intelligence in transport: an overview,” Sustainability, vol. 11, no. 1, p. 189, Jan. 2019, doi: 10.3390/su11010189.
[17] F. Cepolina and E. M. Cepolina, “Space optimization in warehouses logistics,” in Proc. 16th International Conference on Harbor, Maritime and Multimodal Logistics Modelling and Simulation, HMS 2014, 2014, pp. 140 145.
[19] E. M. Cepolina, F. Cepolina, and G. Ferla, “On line shopping and logistics: a fast dynamic vehicle routing algorithm for dealing with information evolution,” in Proceedings of the 23rd International Conference on Harbor, Maritime and Multimodal Logistic Modeling and Simulation, 2021, pp. 27 36., doi: 10.46354/i3m.2021.hms.004.
[10] F. Longo, A. Padovano, L. Nicoletti, M. Elbasheer, and R. Diaz, “Digital twins for manufacturing and logistics systems: is simulation practice ready?,” in Proceedings of the 33rd European Modeling and Simulation Symposium (EMSS 2021), 2021, pp. 435 442., doi: 10.46354/i3m.2021.emss.062.
REFERENCES
[6] M. R. Pahlawan, E. Riksakomara, R. Tyasnurita, A. Muklason, F. Mahananto, and R. A. Vinarti, “Stock price forecast of macro economic factor using recurrent neural network,” IAES Int. J. Artif. Intell., vol. 10, no. 1, pp. 74 83, Mar. 2021, doi: 10.11591/ijai.v10.i1.pp74 83.
[8] F. Longo, L. Nicoletti, and A. Padovano, “Smart operators in industry 4.0: A human centered approach to enhance operators’ capabilities and competencies within the new smart factory context,” Comput. Ind. Eng., vol. 113, pp. 144 159, Nov. 2017, doi: 10.1016/j.cie.2017.09.016. [9] E. M. Cepolina and F. Cepolina, “Twin tools for intelligent manufacturing: a case study,” 2021., doi: 10.46354/i3m.2021.emss.059.
[12] X. Lu and X. Geng, “Car sales volume prediction based on particle swarm optimization algorithm and support vector regression,” in 2011 Fourth International Conference on Intelligent Computation Technology and Automation, Mar. 2011, pp. 71 74., doi: 10.1109/ICICTA.2011.25.
[4] M. E. Banihabib, F. S. Hashemi Madani, and A. Forghani, “Comparison of compensatory and non compensatory multi criteria decision making models in water resources strategic management,” Water Resour. Manag., vol. 31, no. 12, pp. 3745 3759, Sep. 2017, doi: 10.1007/s11269 017 1702 x. [5] T. Mohd, S. Jamil, and S. Masrom, “Machine learning building price prediction with green building determinant,” IAES Int. J. Artif. Intell., vol. 9, no. 3, pp. 379 386, Sep. 2020, doi: 10.11591/ijai.v9.i3.pp379 386.
[15] Z. Huang, D. Zeng, and H. Chen, “A comparison of collaborative filtering recommendation algorithms for e commerce,” IEEE Intell. Syst., vol. 22, no. 5, pp. 68 78, Sep. 2007, doi: 10.1109/MIS.2007.4338497.
[24] M. Rovira et al., “IndexTV: a MPEG 7 based personalized recommendation system for digital TV,” in 2004 IEEE International Conference on Multimedia and Expo (ICME) (IEEE Cat. No.04TH8763), 2004, pp. 823 826., doi: 10.1109/ICME.2004.1394327. [25] A. Jain, A. Shakya, H. Khatter, and A. K. Gupta, “A smart system for fake news detection using machine learning,” in 2019 International Conference on Issues and Challenges in Intelligent Computing Techniques (ICICT), Sep. 2019, pp. 1 4., doi: 10.1109/ICICT46931.2019.8977659.
[2] N. M. Mahfuz, M. Yusoff, and Z. Ahmad, “Review of single clustering methods,” IAES Int. J. Artif. Intell., vol. 8, no. 3, pp. 221 227, Dec. 2019, doi: 10.11591/ijai.v8.i3.pp221 227. [3] M. Chui et al., “Notes from the AI frontier: Insights from hundreds of use cases.” McKinsey Global Institute, pp. 1 30, 2018.
[27] A. J. Dahalan, T. R. Razak, M. H. Ismail, S. S. Mohd Fauzi, and R. A. JM Gining, “Heart rate events classification via explainable fuzzy logic systems,” IAES Int. J. Artif. Intell., vol. 10, no. 4, pp. 1036 1047, Dec. 2021, doi: 10.11591/ijai.v10.i4.pp1036 1047. [28] H. Mohd Nasir, N. M. A. Brahin, M. M. M. Aminuddin, M. S. Mispan, and M. F. Zulkifli, “Android based application for visually impaired using deep learning approach,” IAES Int. J. Artif. Intell., vol. 10, no. 4, pp. 879 888, Dec. 2021, doi: 10.11591/ijai.v10.i4.pp879 888. [29] V. Kepuska and G. Bohouta, “Next generation of virtual personal assistants (Microsoft Cortana, Apple Siri, Amazon Alexa and Google Home),” in 2018 IEEE 8th Annual Computing and Communication Workshop and Conference (CCWC), Jan. 2018, pp. 99 103., doi: 10.1109/CCWC.2018.8301638. [30] Z. Rustam, A. Purwanto, S. Hartini, and G. S. Saragih, “Lung cancer classification using fuzzy c means and fuzzy kernel C Means based on CT scan image,” IAES Int. J. Artif. Intell., vol. 10, no. 2, pp. 291 297, Jun. 2021, doi: 10.11591/ijai.v10.i2.pp291 297.
[7] N. Seman and N. A. Razmi, “Machine learning based technique for big data sentiments extraction,” IAES Int. J. Artif. Intell., vol. 9, no. 3, pp. 473 479, Sep. 2020, doi: 10.11591/ijai.v9.i3.pp473 479.
Brainstorm on artificial intelligence applications and evaluation of … (Elvezia Maria Cepolina) 807
Int J Artif Intell ISSN: 2252 8938
[11] A. Bharati, R. Singh, M. Vatsa, and K. W. Bowyer, “Detecting facial retouching using supervised deep learning,” IEEE Trans. Inf. Forensics Secur., vol. 11, no. 9, pp. 1903 1913, Sep. 2016, doi: 10.1109/TIFS.2016.2561898.
[1] V. S. Padala, K. Gandhi, and P. Dasari, “Machine learning: the new language for applications,” IAES Int. J. Artif. Intell., vol. 8, no. 4, pp. 411 412, Dec. 2019, doi: 10.11591/ijai.v8.i4.pp411 421.
[13] S. Singhal and P. Tanwar, “A prediction model for benefitting e commerce through usage of regional data: A new framework,” IAES Int. J. Artif. Intell., vol. 10, no. 4, pp. 1009 1018, Dec. 2021, doi: 10.11591/ijai.v10.i4.pp1009 1018.
ISSN: 2252 8938 Int J Artif Intell, Vol. 11, No. 3, September 2022: 799 808 808 [31] M. Z. N. AL Dabagh, “Automated tumor segmentation in MR brain image using fuzzy c means clustering and seeded region methodology,” IAES Int. J. Artif. Intell., vol. 10, no. 2, pp. 284 290, Jun. 2021, doi: 10.11591/ijai.v10.i2.pp284 290.
BIOGRAPHIES OF AUTHORS
Ph.D. Elvezia Maria Cepolina received her degree in Civil Engineering from the University of Genoa, Italy in 1998 and Ph.D degree in Engineering and Economics of Transport from the University of Genoa, Italy in 2021. She is currently Assistant Professor at Department of Political Sciences, University of Genoa; Member of the Italian Centre of Excellence on Logistics, Transport and Infrastructures (CIELI) and vice coordinator of the International PhD in Strategic Engineering. Her main research interests are: Strategic Engineering; Modelling and simulation of transport systems; Last mile freight distribution; Car sharing systems; Fleet optimization; Impact assessment. She can be contacted at email: elvezia.maria.cepolina@unige.it. Ph.D. Francesco Cepolina received four degrees: Ordinary degree of Bachelor of Engineering in Mechanical Engineering (1997, University of Leeds, UK), five years degree in Mechanical Engineering (1999, University of Genoa, IT), Ph.D in Mechanics and Design of Machines (2005, University of Genoa, IT) and Ph.D in Mechanical, Acoustic and Electronic Sciences (2006, University of Paris VI, FR). Francesco is deeply interested in: surgery and service robotics, logistics, integrated design and manufacturing, mechanical design and three dimensional modelling. Francesco is reviewer of the following journals: “IEEE Transactions on biomedical Engineering”, “Sensors and Actuators, Elsevier”. He can be contacted at email: francesco.cepolina@edu.unige.it.
Dr. Guido Ferla received the degree in Mechanical Engineering at the University of Genoa in 1979. Until August 2018 held the position of Technical Director (Italy) and Group Technology Manager (Italy and Germany) of the company Hager. Since August 2018 is an Industrial Consultant and collaborates with the School of Science and Technology University of Camerino. Areas of expertise: Design and industrialization of production plants/machinery/equipment; Production Qualty; Laboraties for chemical analysis of raw materials and mechanical analysis in accordance with European Standards; Process Control and Automation. He can be contacted at email: guido.ferla@yahoo.it.
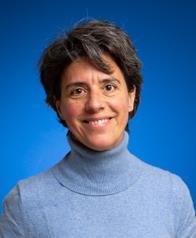



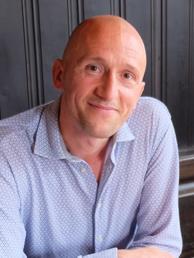



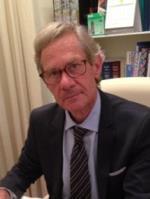



Department of Electrical Engineering Vocational Education, Universitas Sultan Ageng Tirtayasa, Serang, Indonesia
Department of Mechanical Engineering Vocational Education, Universitas Sultan Ageng Tirtayasa, Serang, Indonesia
IAES International Journal of Artificial Intelligence (IJ AI) Vol. 11, No. 3, September 2022, pp. 809 818 ISSN: 2252 8938, DOI: 10.11591/ijai.v11.i3.pp809 818 809
Corresponding Author: Norazhar Abu Bakar Department of Electrical Engineering, Faculty of Electrical Engineering, Universiti Teknikal Malaysia HangMelakaTuah Jaya, 76100 Durian Tunggal, Melaka, Malaysia Email: norazhar@utem.edu.my
A smart traffic light using a microcontroller based on the fuzzy logic Desmira1,2, Mustofa Abi Hamid1, Norazhar Abu Bakar2, Muhammad Nurtanto3, Sunardi4
4
1. INTRODUCTION
In recent years, traffic issues are an essential concern to be examined. The main problem is the frequent traffic congestions in many major cities in the world [1]. The constant time traffic control method is not optimal in regulating traffic, and the current densities of vehicles passing during peak hours may result in unavoidable congestions [2]. The increasing volume of vehicles is not comparable to the number of roads available [3]; this results in the buildup of vehicles on certain roads during peak hours, which causes congestions on the roads. This problem interferes with drivers and affects the local economy [2]. The impacts it may have on cost and time effectiveness may take the form of increased fuel consumption, traffic emissions, and noise. The congestion problem all over the world is caused by the growth of the number of vehicles that exceeds the available capacity [4], requiring a higher level of traffic efficiency in order to reduce the congestion. One way to increase traffic efficiency is to use an intelligent transportation system and real time signal control system [5] [7]. Using artificial intelligence, the traffic light system can be adjusted based on
Keywords: Fuzzy TrafficSmartSensorsMicrocontrollerlogictrafficlightsjam
Journal homepage: http://ijai.iaescore.com
Department of Electrical Engineering, Faculty of Electrical Engineering, Universiti Teknikal Malaysia Melaka, Melaka, Malaysia
Department of Railway Electrical Engineering, Politeknik Perkeretaapian Indonesia, Madiun, Indonesia
2
This is an open access article under the CC BY SA license.
Article history: Received Aug 14, 2021 Revised Apr 13, 2022 Accepted May 12, 2022 Traffic jam that is resulted from the buildup of vehicles on the road has become an important problem, which leads to an interference with drivers. The impacts it has on cost and time effectiveness may take the form of increased fuel consumption, traffic emissions, and noise. This paper offers a solution by creating a smart traffic light using a fuzzy logic based microcontroller for a greater adaptability of the traffic light to the dynamics of the vehicles that are to cross the intersection. The ATMega2560 microcontroller based smart traffic light is designed to create a breakthrough in the breakdown of congestions at road junctions, thereby optimizing the real time happenings in the road. Ultrasonic, infrared, and light sensors are used in this smart traffic light, resulting in the smart traffic light’s effectiveness in parsing jams. The four sets of sensors that are placed in four sections determine the traffic light timing process. When the length of vehicle queue reaches the sensor, a signal is sent as the microcontroller’s digital input. Ultrasonic and infrared sensors can reduce congestions at traffic lights by giving a green light time when one or all of the sensors are active so that the vehicle congestions can be relieved.
Article Info ABSTRACT
1
3
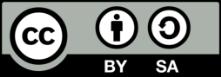
There are some studies offering solutions to the problem, one of which is by applying a fuzzy logic based controller to control the traffic at a four way intersection. The traffic light is applied to four junction sections with a fixed movement pattern; that is, when the east west traffic has a turn during the green light, then the north south traffic must not pass, and vice versa. To know the number of vehicles in each direction, sensors are used to provide fuzzy logic inputs. Fuzzy logic is applied to traffic lights and simulated with MATLAB tools with the Mamdani method [8]
ISSN: 2252 8938 Int J Artif Intell, Vol. 11, No. 3, September 2022: 809 818 810thelevel of vehicle density in the crossing path without ignoring the demand of the other line [8] [13]. The traffic density can be reduced by controlling the traffic light using a fuzzy logic based traffic light controller; this can reduce the stopping time at the traffic light because the controller can adjust to the traffic density [9]
The arrangement of the traffic flow at the intersection is primarily intended for the vehicles in each way to move in turn so as not to interfere with the flow of vehicles in the other way. There are different types of controls that are used for traffic lights. The controls used are based on the consideration of the situations and conditions of the intersection, including the traffic volume and the geometry of the junction [14] [21]
In an instance, researcher designed a traffic light control simulator with a fuzzy logic based microcontroller. The simulator was designed first for a trial using MATLAB with Sugeno’s method. In the simulator, a switch is used as a sensor that serves as a density counter on one track and a microcontroller is used as a light controller of the traffic [9], [22] [24]. In a separate study, researcher performed a simulation using adaptive neuro fuzzy inference system (ANFIS) on a six segment traffic light system to compare the average stopping time resulting from the use of the static method against that from the use of the dynamic method; ANFIS in the neuro fuzzy group was applied to an intersection of six sections [25], created an efficient ANFIS based fractional order PID (FOPID) controller for an electric vehicle (EV) speed tracking control driven by a direct current (DC) motor [25] Meanwhile, we offer a solution to the issue above by creating a smart traffic light with a microcontroller based on the fuzzy logic. This solution is very important to break down the increasingly severe congestions in major cities in Indonesia. The built in traffic light system can adapt to the intersection environment. If there is a road that has a long vehicle queue, then the green light time is longer on that road than on the other road that has only a shorter vehicle queue. Thus, the traffic light is more adaptive to the dynamics of vehicles that will cross the intersection. The traffic light can also communicate with neighboring traffic lights in two directions. This communication will generate information about the number of vehicles leaving the intersection toward each of the closest intersections. Using this information, apart from the information from the sensors, the traffic light will be able to recognize the number of vehicles coming in each direction.
Figure 1. Power
circuit
2. RESEARCH METHOD 2.1. Power supply planning A circuit of power supply is applied to the sensors, microcontroller, relay outputs, and light emitting diodes (LEDs). The input voltage is supplied by the State Electricity Company (Perusahaan Listrik Negara in Indonesian, PLN) at 220 Volts alternating current (AC), which is then lowered to 12 Volts AC and converted into DC voltage [26]. After that, the output voltage of power supply is set to 5 Volts according to the needs of the microcontroller. The power supply circuit can be seen in Figure 1. supply
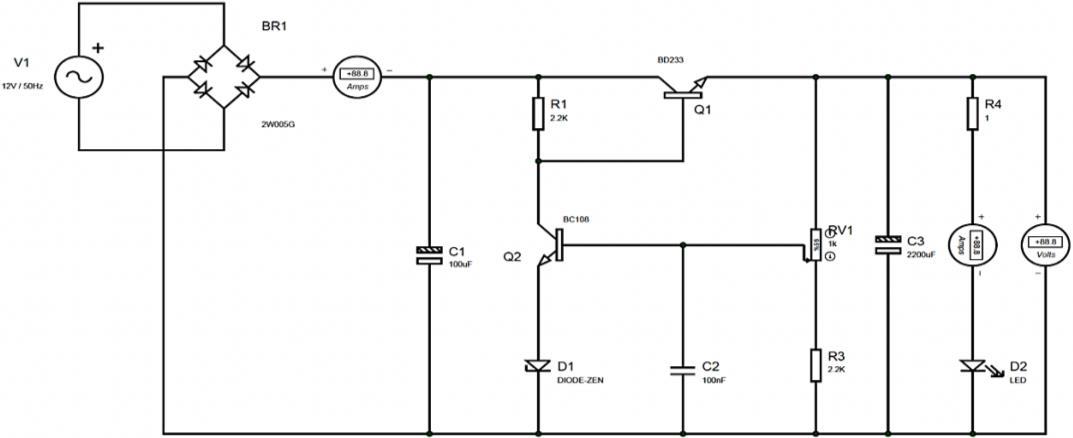
2.2.1. Sensors planning
Int J Artif Intell ISSN: 2252 8938 A smart traffic light using a microcontroller based on the fuzzy logic (Desmira) 811 2.2. Input planning
The ultrasonic sensor requires echo and trigger to communicate with the microcontroller, i.e., when a positive pulse (+) hits 0.58 millisecond to 23.26 milliseconds for an echo pin comparable to the distance required to propagate when a bottleneck occurs, while the trigger gets 4 milliseconds. The infrared sensor system mainly uses infrared as a medium for data communication [32], between the receiver and transmitter. The system will work if the infrared light emitted is obstructed by an object resulting in an infrared beam that cannot be detected by the receiver. The advantages or benefits of this system in its implementation include remote control, security alarms, and system automation. The transmitter on this system is self contained on an infrared LED equipped with a network capable of generating data to be transmitted through infrared light, while on the receiver there is a photodiode that serves to receive light. Infrared light is transmitted by the transmitter [33]. The infrared sensor set with photodiode can be seen in Figure 2. The light sensor serves as a street light sensor [34], so that the streetlight will be turned on automatically after sunset and turned off during daytime. A range of light sensors can be seen in Figure 3.
Figure 2. Infrared sensor circuit with photodiode Figure 3. Light sensor circuit
The sensors used are ultrasonic, infrared, and light sensors [27] [29]. The ultrasonic sensor detects the distance of a vehicle from an object in front of it [30], [31]. This sensor is capable of detecting a distance in the range of 3 cm to 3 m. Its working principle is that the ultrasonic sensor transmitter emits a frequency of 40 kHz that is generated by the microcontroller, which then is received by the ultrasonic sensor receiver. The distance set in the program is 5 cm, so that if there are objects that are censored at a distance of 5 cm, then the ultrasonic transmitter receives a surge reflection and will send signals on the microcontroller. The ultrasonic sensor works when it detects the vehicle density at one of the junction segments, whereas the microcontroller will adjust the time needed to reflect the signal back to the ultrasonic sensor receiver, which is comparable to 2 times the distance of the ultrasonic sensor and the density of the cars that occurs as shown in (1):
The input for this circuit is from the 3 sensors used as input tools on the microcontroller. It will then be processed into output. Each has two sensors placed at each section of the intersection. One light sensor works at night when the streetlight is lit. It will be active when there are objects on the line of reach. The sensor will then send the input signal on the microcontroller and the program that has been created will then execute it.
������������������������������������= (�� ��)��2(��) �� (1)
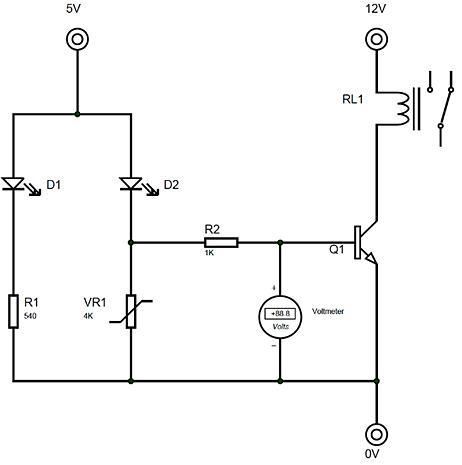
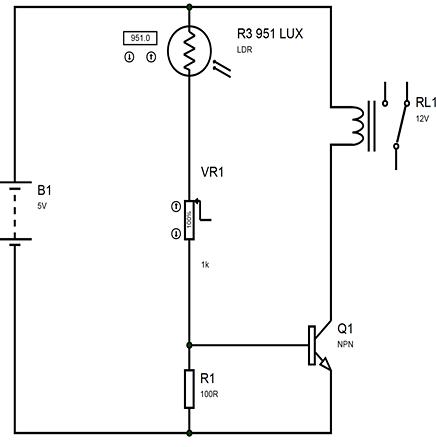
2.3.1. Microcontroller planning
2.4. Output planning
Figure 4. Flow chart of traffic light sensor
2.3. Process planning
ISSN: 2252 8938 Int J Artif Intell, Vol. 11, No. 3, September 2022: 809 818 812
The outputs are LEDs as traffic indication lights as the working markers of the traffic light program. The smart traffic light based on an ATMega2560 microcontroller is designed to make new breakthroughs in breaking down congestions at crossroads so as to optimize the actual situations in the field. This tool is designed as a control system that works according to the length of the vehicle queue utilizing 4 sets of sensors that are installed at each intersection. Time is added to traffic light when the length of the vehicle queue reaches the sonsor that has been installed. The sensor will send a signal as digital input on the microcontroller that will instruct the addition of time on the program. Meanwhile, if the length of queue on the road segment does not activate the sensor, then the time applied is normal in the program. In the design of this tool, the sensor is placed in the middle ofthe road to receive a signal from the vehicle queue if the number of vehicles in the queue exceeds the sensor limit. The workflow of the design can be seen in Figure 4.
If the relay is given a voltage of 5 Volts and ground on coil legs, then the relay will work. There are seven output relays used, which can be seen in Table 1. Supporting outputs in the form of LEDs are assembled into the crossroad traffic light. There are 12 LEDs consisting of LEDs in red, yellow, and green. The LEDs are placed in each section of the road intersection. The LEDs will work when the output pin of the microcontroller signals a high on the foot of the LED by 5 volt [35], [36]
The microcontroller used in this prototype is ATMega2560. The ATMega2560 microcontroller consists of 54 pins that can be used as inputs/outputs, of which 15 pins can be used as pulse width modulation (PWM), 16 pins as analog inputs, and four pins as universal asynchronous receiver/transmitter (UART). This prototype uses 23 inputs/outputs for the traffic light program.
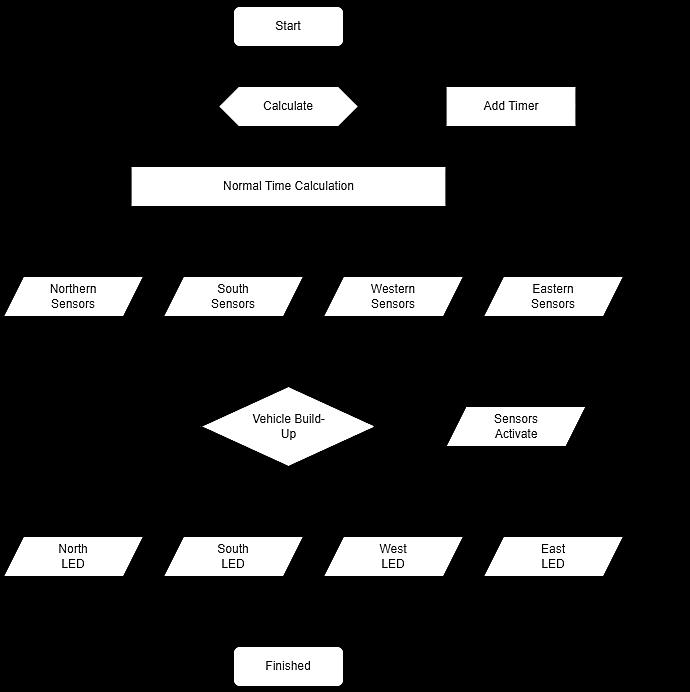
The flow chart of traffic light sensor in Figure 4 describes the details of how the signal prototype is made to determine the length of time of green and red light at an intersection and its effect on the vehicles buildup in one section of the intersection. This flow chart can help determine an efficient length of time of traffic light at a crossroads. After all the inputs from the sensors are examined, then the signal junction simulation will calculate the length of time of green and red light along with the buildup that occurs. After all the calculation process is complete, this prototype will show the best results and will run continuously to set the traffic light regularly in each section. The flow chart of the traffic light system in Figure 4 further describes the following traffic rules at the intersection: i) the lamp that is controlled using the fuzzification process is the green light of each traffic light, so that the red light of each traffic light will adjust to the green light that is active in the other traffic lights, and ii) the length of time the green light is lit in a traffic light will be limited to the absolute maximum and minimum time values, so even if the density level is very high, it will stillgeta stop turn following the red lightto give an opportunityforthe traffic from othersections to cross. The program will run with normal time alike to the traffic light in general: the green light will be active for 3 seconds, the yellow light for 1 second, and the red light in a normal cycle for 9 seconds, but if the sensor is activated, then the stopping time will be added with 3 seconds in each section. The digital input number 8 will be active if input from the real time counter (RTC) is received, which will instruct all flashing LEDs to be enabled for the night operating hours. In this study, the prototype time on traffic lights is made 1:10 against normal circumstances. The prototype is made using the software Arduino IDE to create a traffic light program based on an ATMega2560 microcontroller using C language. It will first determine the total number of digital pin inputs and digital outputs used.
Broadly, the processes that run in the smart traffic light based on ATMega2560 microcontroller include the programming process, program simulation process, instruction reading process, and sensor firing process according to instruction [37] [40]. The smart traffic light based on ATMega2560 microcontroller is designed to create breakthroughs in breaking down congestions at road junctions, optimizing the real time happenings in the field. This tool is designed as a control system that works according to the length of the vehicle queue utilizing four sets of sensors installed at each intersection. Time is added to a traffic light when the vehicle queue length reaches an appropriate limit according to the sensor that has been installed. The sensor will send a signal as digital input on the microcontroller that will instruct the addition of time on the program. If the length of vehicle queue in the road section does not activate the sensor, then the time calculation on the program runs normally. In the design of this tool, the sensor is placed in the middle of the road toreceive a signalfrom the vehicle queue when the numberofvehicles in the queue exceeds the sensor limit. 2.6. Program planning ATMega2560 has 54 digital inputs/outputs, of which 14 are used as PWM outputs, 16 as analog inputs, and 4 as UART. Additionally, it has 16 MHz crystal oscillator, universal serial bus (USB) connection, power jack, ICSP header, and reset button. This module has everything we need to program a microcontroller, such as a USB cable and a power source via an adaptor or a battery. The design details are presented in the flow chart in Figure 4.
The input and output test results, computed using (1), can bee have seen in Table 3. The distance calculation results can be seen in Table 4.
Int J Artif Intell ISSN: 2252 8938 A smart traffic light using a microcontroller based on the fuzzy logic (Desmira) 813 Table 1. Relay outputs No. Relay Description 1 Relay 1 Congestion Sensor Relay in the West 2 Relay 2 Congestion Sensor Relay in the East 3 Relay 3 Congestion Sensor Relay in the North 4 Relay 4 Congestion Sensor Relay in the South 5 Relay 5 Northern Sensor Relay 6 Relay 6 Western Sensor Relay 7 Relay 7 Light Sensor Relay 2.5. How does it work?
3. RESULTS AND DISCUSSION For the digital data, signaling requires a voltage source that has the “TTL” level, following the logic HIGH=+5 Volts and the logic LOW=0 Volt, so that the output of the light sensor system that will be fed to the parallel port must have a TTL voltage level. Table 2 describes the level of stability of the power supply.
Table 4. The distance calculation results No. Distance (s/m) Calculation Ultrasonic wave travel time 1 0 1 �� = 01x2 344m/s 0 58 ms 2 0 25 �� = 025x2 344m/s 1 45 ms 3 0 5 �� = 05x2 344m/s 2 91 ms 4 0 75 �� = 0.75x2 344m/s 4 36 ms 5 1 �� = 1x2 344m/s 5 81 ms 6 2 �� = 2x2 344m/s 11 63 ms 7 3 �� = 3x2 344m/s 17 44 ms 8 4 �� = 4x2 344m/s 23 26 ms
ISSN: 2252 8938 Int J Artif Intell, Vol. 11, No. 3, September 2022: 809 818 814
Table 2. Power supply testing Load Voltage (V) Without load 5.23 Ultrasonic load 1 5.15 Ultrasonic load 2 5.14 Infrared load 1 5.20 Infrared load 2 5.19 Light Sensor load 5.20 Table 3. Voltage testing results No. Reference voltage (V) Input voltage (V) Output voltage (V) 1 2 75 3 31 0 2 3 00 3 46 0 3 3 25 3 61 0 4 3 50 3 77 4 42 5 3 71 3 90 4 43 6 3 93 4 03 4 43 7 4 16 4 17 4 43 8 4 43 4 94 4 80
Using the existing data, the fuzzy logic method is undertaken in the following stages: i) data input; ii) fuzzification in which membership degrees are generated; and iii) rule base. The fuzzification process can be seen on Figure 5. According to the value data, we take a distance of 3.5 meters and a voltage of 4.5 Volts then insert these into the set. The fuzzification process can be seen in Figure 6. According the existing data, distance is a blurred value in the medium and small set positions. The distance for small set membership as shown in (2) ��(��)={1; 4 �� 4 3 0; ; �� ≤3 3 ≤�� ≤4 �� ≥4 (2) with the linear representation formula going down ������������(35)= 4 35 4 3 = 05 1 =05 (3) The distance for medium set membership as shown in (4): ��(��)={0; �� 3 4 3 1; ; �� ≤3 3 ≤�� ≤ 4 �� ≥4 (4)
Table 5. Rule base Rule Input set Sensor Travel timeDistance Voltage 1 Small Small Long 2 Small Medium Briefly 3 Small Big Briefly 4 Medium Small Long 5 Medium Briefly 6 Medium Big Briefly 7 Big Small Long Big Medium Long 9 Big Big Long
8
Int J Artif Intell ISSN: 2252 8938 A smart traffic light using a microcontroller based on the fuzzy logic (Desmira) 815 with the triangular linear representation formula ��������������(3.5)= 4 3 4 3 = 1 1 =1. (5)
Figure 5 Set membership Figure 6 Distance and voltage membership set From formulas (2) and (4), it can be concluded that the membership degree for the distance in small set is 0.5, in moderate set 1, and in large set 2. Then the membership degree for voltage is a blurred value in the medium and large set positions The voltage for medium set membership as shown in (6): ��(��)={1; 5 �� 5 4 0; ; �� ≤4 4 ≤�� ≤ 5 �� ≥5 (6) with the linear representation formula rising ��������������(4.5)= 5 45 5 4 = 05 1 =0.5 (7) The voltage for large set membership as shown in (8): ��(��)={0; �� 4 5 4 1; ; �� ≤4 4 ≤�� ≤ 5 �� ≥5 (8) with the triangular linear representation formula ��������(4.5)= 5 4 5 4 = 1 1 =1 (9) Then, the degree of membership for a voltage value of 4.5 is: small: 2, medium: 1, and large: 0.5. The rule base can be seen in Table 5.
Medium
According to the rule base in Table 5, when four rules rules 2, 3, 5 and 6 are entered into the program, then the following will apply: Rule 2: if distance=small and voltage=medium, then the sensor time is brief.
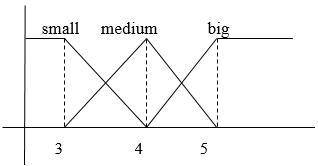
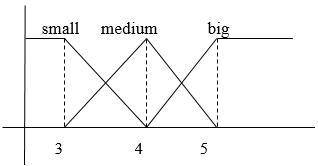
[11] K. Chatterjee, A. De, and F. T. S. Chan, “Real time traffic delay optimization using shadowed type 2 fuzzy rule base,” Appl. Soft Comput., vol. 74, pp. 226 241, Jan. 2019, doi: 10.1016/j.asoc.2018.10.008.
[12] M. Balta and İ. Özçeli̇k, “A 3 stage fuzzy decision tree model for traffic signal optimization in urban city via a SDN based VANET architecture,” Futur. Gener. Comput. Syst., vol. 104, pp. 142 158, Mar. 2020, doi: 10.1016/j.future.2019.10.020.
[13] Y. E. Hawas, M. Sherif, and M. D. Alam, “Optimized multistage fuzzy based model for incident detection and management on urban streets,” Fuzzy Sets Syst., vol. 381, pp. 78 104, Feb. 2020, doi: 10.1016/j.fss.2019.06.003.
[8] S. Komsiyah and E. Desvania, “Traffic lights analysis and simulation using fuzzy inference system of mamdani on three signaled intersections,” Procedia Comput. Sci., vol. 179, pp. 268 280, 2021, doi: 10.1016/j.procs.2021.01.006.
[14] Y. Zhang and R. Su, “An optimization model and traffic light control scheme for heterogeneous traffic systems,” Transp. Res. Part C Emerg. Technol., vol. 124, p. 102911, Mar. 2021, doi: 10.1016/j.trc.2020.102911.
α=min (µ small (x); µ medium (x)) α=min (1. 1)
[9] C. Karakuzu and O. Demirci, “Fuzzy logic based smart traffic light simulator design and hardware implementation,” Appl. Soft Comput., vol. 10, no. 1, pp. 66 73, Jan. 2010, doi: 10.1016/j.asoc.2009.06.002.
Rule 3: if distance=small and voltage=big, then the sensor time is brief. α=min (µ small (x); µ big (x)) α=min (1. 0.5)
[10] M. A. Hamid, S. A. Rahman, I. A. Darmawan, M. Fatkhurrokhman, and M. Nurtanto, “Performance efficiency of virtual laboratory based on Unity 3D and Blender during the Covid 19 pandemic,” J. Phys. Conf. Ser., vol. 2111, no. 1, p. 12054, Nov. 2021, doi: 10.1088/1742 6596/2111/1/012054.
[7] A. M. de Souza and L. A. Villas, “A new solution based on inter vehicle communication to reduce traffic jam in highway environment,” IEEE Lat. Am. Trans., vol. 13, no. 3, pp. 721 726, Mar. 2015, doi: 10.1109/TLA.2015.7069097.
[2] C. Vilarinho and J. P. Tavares, “Real time traffic signal settings at an isolated signal control intersection,” Transp. Res. Procedia, vol. 3, pp. 1021 1030, 2014, doi: 10.1016/j.trpro.2014.10.082. [3] L. W. Canter, Environmental impact of agricultural production activities. CRC Press, 2018. [4] H. Wang, K. Rudy, J. Li, and D. Ni, “Calculation of traffic flow breakdown probability to optimize link throughput,” Appl. Math. Model., vol. 34, no. 11, pp. 3376 3389, Nov. 2010, doi: 10.1016/j.apm.2010.02.027.
REFERENCES [1] H. He, C. Zhang, W. Wang, Y. Hao, and Y. Ding, “Feedback control scheme for traffic jam and energy consumption based on two lane traffic flow model,” Transp. Res. Part D Transp. Environ., vol. 60, pp. 76 84, May 2018, doi: 10.1016/j.trd.2015.11.005.
4. CONCLUSION The four sets of sensors placed in four sections determine the traffic light timing process. If the length of vehicle queue reaches the sensor, then the sensor is activated, a signal will be sent as digital input on the microcontroller, and the stopping time will be added with 9 seconds. The traffic light settings using fuzzy logic control still consider the interests of other traffic sections by providing a minimum of 9 seconds and a maximum of 27 seconds in 1 cycle of light traffic settings as the limits of the fuzzy logic control system. The ultrasonic and infrared sensors can reduce congestions at traffic lights by giving a green light time when one or all of the sensors are active, so that the vehicle congestions can be relieved. The automatic voltage regulator (AVR) ATMega2560 microcontroller requires some additional components such as capacitors, integrated circuit (IC) regulators, and resistors to be able to work or function as expected. Betterment for this research can be performed by adding input variables by adding multiple sensors at various intersections so as to produce real time outputs. It is also recommended to allow access for road users, so that when a congestion is detected, the road users can consider when to pass the road as indicated by the number of vehicles.
Rule 5: if distance=medium and voltage=medium, then the sensor time is brief. α=min (µ medium(x); µ medium(x)) α=min (0.4. 0.6)
Rule 6: if distance=medium and voltage=big, then the sensor time is brief. α=min (µ medium(x); µ big(x)) α=min (0.4. 0.4)
ISSN: 2252 8938 Int J Artif Intell, Vol. 11, No. 3, September 2022: 809 818 816
[5] J. Liu et al., “Secure intelligent traffic light control using fog computing,” Futur. Gener. Comput. Syst., vol. 78, pp. 817 824, Jan. 2018, doi: 10.1016/j.future.2017.02.017.
The smart traffic light that is based on the ATMega2560 microcontroler includes a fuzzy logic based system design, software with the C programming language, and hardware. The designed system forms a traffic light setting according to the vehicle queue. The smart traffic light is practical in the creation of tools, smooth in the assembly, capable of breaking down congestions and vehicles density, especially during peak hours, and of minimizing violations during crossing, and safe to use. Its drawbacks lie in its limited sensor range and its susceptibility to noise.
[6] C. Y. Cui, J. S. Shin, M. Miyazaki, and H. H. Lee, “Real time traffic signal control for optimization of traffic jam probability,” Electron. Commun. Japan, vol. 96, no. 1, pp. 1 13, Jan. 2013, doi: 10.1002/ecj.11436.
[31] J. Liu, J. Han, H. Lv, and B. Li, “An ultrasonic sensor system based on a two dimensional state method for highway vehicle violation detection applications,” Sensors, vol. 15, no. 4, pp. 9000 9021, Apr. 2015, doi: 10.3390/s150409000.
[32] J. M. Kahn and J. R. Barry, “Wireless infrared communications,” Proc. IEEE, vol. 85, no. 2, pp. 265 298, 1997, doi: 10.1109/5.554222.
[34] P. Elejoste et al., “An easy to deploy street light control system based on wireless communication and LED technology,” Sensors, vol. 13, no. 5, pp. 6492 6523, May 2013, doi: 10.3390/s130506492.
BIOGRAPHIES OF AUTHORS
[16] M. A. Hamid, E. Permata, D. Aribowo, I. A. Darmawan, M. Nurtanto, and S. Laraswati, “Development of cooperative learning based electric circuit kit trainer for basic electrical and electronics practice,” J. Phys. Conf. Ser., vol. 1456, no. 1, p. 12047, Jan. 2020, doi: 10.1088/1742 6596/1456/1/012047.
[25] M. A. George, D. V. Kamat, and C. P. Kurian, “Electric vehicle speed tracking control using an ANFIS based fractional order PID controller,” J. King Saud Univ. Sci., Jan. 2022, doi: 10.1016/j.jksues.2022.01.001.
[35] M. Jaanus, A. Udal, V. Kukk, and K. Umbleja, “Using microcontrollers for high accuracy analogue measurements,” Electron. Electr. Eng., vol. 19, no. 6, Jun. 2013, doi: 10.5755/j01.eee.19.6.4559.
[38] M. A. Hamid, D. Aditama, E. Permata, N. Kholifah, M. Nurtanto, and N. W. A. Majid, “Simulating the Covid 19 epidemic event and its prevention measures using python programming,” Indones. J. Electr. Eng. Comput. Sci., vol. 26, no. 1, 2022.
Desmira is currently a lecturer with Universitas Sultan Ageng Tirtayasa (Untirta), Indonesia. She is also an Assistant Professor with the Department of Electrical Engineering Vocational Education, Untirta. She has published more than 40 research articles, with more than 80 citations received in the Google Scholar and H index 3. His research interests in the areas of electrical engineering, sensors, and electronics. She can be contacted at email: desmira@untirta.ac.id
[27] S. Adarsh, S. M. Kaleemuddin, D. Bose, and K. I. Ramachandran, “Performance comparison of Infrared and Ultrasonic sensors for obstacles of different materials in vehicle/ robot navigation applications,” IOP Conf. Ser. Mater. Sci. Eng., vol. 149, p. 12141, Sep. 2016, doi: 10.1088/1757 899X/149/1/012141.
[18] L. Ramirez Polo, M. A. Jimenez Barros, V. V. Narváez, and C. P. Daza, “Simulation and optimization of traffic lights for vehicles flow in high traffic areas,” Procedia Comput. Sci., vol. 198, pp. 548 553, 2022, doi: 10.1016/j.procs.2021.12.284.
[36] B. Singh, Ed., Computational tools and techniques for biomedical signal processing. IGI Global, 2017.
[22] R. Rossi, M. Gastaldi, F. Orsini, G. De Cet, and C. Meneguzzer, “A comparative simulator study of reaction times to yellow traffic light under manual and automated driving,” Transp. Res. Procedia, vol. 52, pp. 276 283, 2021, doi: 10.1016/j.trpro.2021.01.032.
[37] M. M. Hilgart, L. M. Ritterband, F. P. Thorndike, and M. B. Kinzie, “Using instructional design process to improve design and development of internet interventions,” J. Med. Internet Res., vol. 14, no. 3, p. e89, Jun. 2012, doi: 10.2196/jmir.1890.
[39] D. Desmira, N. A. Bakar, R. Wiryadinata, and M. A. Hamid, “Comparison of PCA to improve the performance of ANFIS Models in predicting energy use in EEVE Laboratories,” Indones. J. Electr. Eng. Comput. Sci., vol. 27, no. 1, 2022.
[30] P. Kohler, C. Connette, and A. Verl, “Vehicle tracking using ultrasonic sensors & joined particle weighting,” in 2013 IEEE International Conference on Robotics and Automation, May 2013, pp. 2900 2905, doi: 10.1109/ICRA.2013.6630979.
[33] D. Liu, L. Wang, and K. C. Tan, Eds., Design and control of intelligent robotic systems, vol. 177. Berlin, Heidelberg: Springer Berlin Heidelberg, 2009.
[20] S. Ma and X. Yan, “Examining the efficacy of improved traffic signs and markings at flashing light controlled grade crossings based on driving simulation and eye tracking systems,” Transp. Res. Part F Traffic Psychol. Behav., vol. 81, pp. 173 189, Aug. 2021, doi: 10.1016/j.trf.2021.05.019.
[17] A. Rahmat, A. R. Nugroho, A. Saregar, M. A. Hamid, M. R. N. Prastyo, and A. Mutolib, “Small hydropower potential of rivers in Sukabumi Regency, West Java, Indonesia,” J. Phys. Conf. Ser., vol. 1155, p. 12041, Feb. 2019, doi: 10.1088/1742 6596/1155/1/012041.
[15] D. Desmira, M. A. Hamid, Irwanto, S. D. Ramdani, and T. Y. Pratama, “An ultrasonic and temperature sensor prototype using fuzzy method for guiding blind people,” J. Phys. Conf. Ser., vol. 1446, no. 1, p. 12045, Jan. 2020, doi: 10.1088/1742 6596/1446/1/012045.
[19] Y. Zhao and P. Ioannou, “A co simulation, optimization, control approach for traffic light control with truck priority,” Annu. Rev. Control, vol. 48, pp. 283 291, 2019, doi: 10.1016/j.arcontrol.2019.09.006.
[29] B. Mustapha, A. Zayegh, and R. K. Begg, “Ultrasonic and infrared sensors performance in a wireless obstacle detection system,” in 2013 1st International Conference on Artificial Intelligence, Modelling and Simulation, Dec. 2013, pp. 487 492, doi: 10.1109/AIMS.2013.89.
Int J Artif Intell ISSN: 2252 8938 A smart traffic light using a microcontroller based on the fuzzy logic (Desmira) 817
[21] Y. Perez and F. H. Pereira, “Simulation of traffic light disruptions in street networks,” Phys. A Stat. Mech. its Appl., vol. 582, p. 126225, Nov. 2021, doi: 10.1016/j.physa.2021.126225.
[28] M. S. Mohiuddin, “Performance comparison of conventional controller with fuzzy logic controller using chopper circuit and fuzzy tuned PID controller,” Indones. J. Electr. Eng. Informatics, vol. 2, no. 4, pp. 189 200, Dec. 2014, doi: 10.11591/ijeei.v2i4.120.
[23] U. Mittal, P. Chawla, and A. Kumar, “Smart traffic light management system for heavy vehicles,” in Autonomous and Connected Heavy Vehicle Technology, Elsevier, 2022, pp. 225 244. [24] C. A. Teixeira, E. R. L. Villarreal, M. E. Cintra, and N. W. B. Lima, “Proposal of a fuzzy control system for the management of traffic lights,” IFAC Proc. Vol., vol. 46, no. 7, pp. 456 461, May 2013, doi: 10.3182/20130522 3 BR 4036.00062.
[26] I. A. Darmawan et al., “Electricity course on vocational training centers: a contribution to unemployment management,” J. Phys. Conf. Ser., vol. 1456, no. 1, p. 12048, Jan. 2020, doi: 10.1088/1742 6596/1456/1/012048.
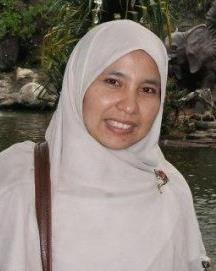




Sunardi is lecturer and researcher at the Department of Electrical Railway Engineering, Indonesia Railway Poytechnic, Madiun. Now a day, my research interest is Electric Vehicles, Power System, Sensors, Signal and System, and Earth and Planetary Sciences, Railway, TVET. He can be contacted at email: sunardi@ppi.ac.id
Mustofa Abi Hamid (Member, IEEE) has been the secretary of Department of Electrical Engineering Vocational Education, Universitas Sultan Ageng Tirtayasa (Untirta), Indonesia, since 2021. He is currently an Assistant Professor with Untirta. He is also a Assistant Professor with the Department of Electrical Engineering Vocational Education, Faculty of Teacher Training and Education, Untirta. He has published more than 40 research articles, with more than 620 citations received in the Google Scholar and Scopus H index 2.
He is on the Editor in Chief of the journal VOLT: Jurnal Ilmiah Pendidikan Teknik Elektro, Journal of Vocational and Technical Education and Training, and reviewer more than international journal. He can be contacted at email: abi.mustofa@untirta.ac.id
ISSN: 2252 8938 Int J Artif Intell, Vol. 11, No. 3, September 2022: 809 818 818
His research interests include in the areas of electrical engineering, electronics, technical and vocational education, curriculum design, learning strategies, learning media, ICT for learning.
Norazhar Abu Bakar (Member, IEEE) received his BEng. (Hons.) degree in electronics and electrical engineering from Leeds University, UK, MSc. (Eng.) in control systems from Sheffield University, UK, and Ph.D. degree in electrical and computer engineering from Curtin University, Australia. He started his career as an assembly engineer at Toshiba Electronics, Malaysia, and then moved to TNB as a SCADA/DA Project Engineer.
Currently, he is the Deputy Dean (Research and Postgraduate Studies) with the Faculty of Electrical Engineering, UTeM. He is a member of the High Voltage Engineering Research Laboratory in UTeM. His research interests are condition monitoring, asset management, and artificial intelligence. Since 2013, he has published several articles related to transformer condition monitoring and asset management in IEEE Transactions on Dielectrics and Electrical Insulation and IEEE Electrical Insulation Magazine. He can be contacted at email: norazhar@utem.edu.my Muhammad Nurtanto is an assistant professor in Department of Mechanical Engineering Education, Universitas Sultan Ageng Tirtayasa, Banten, Indonesia. Research interests in the fields of professional learning, Teacher Emotion, Teacher IdentityPhilosophy of education, STEM education, gamification, and teacher quality in vocational education. He can be contacted at email: mnurtanto23@untirta.ac.id.
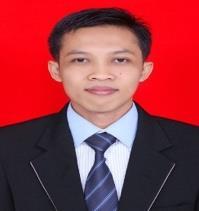




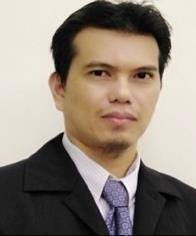




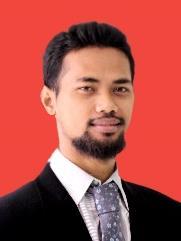




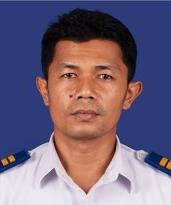




Nurain Zulaikha Husin1, Muhammad Zaini Ahmad1,2, Mustafa Mamat3
2Centre of Excellence for Social Innovation & Sustainability (CoESIS), Universiti Malaysia Perlis, Perlis, Malaysia
3Faculty of Informatics and Computing, Universiti Sultan Zainal Abidin, Terengganu, Malaysia
Corresponding Author: Muhammad Zaini Ahmad Institute of Engineering Mathematics, Faculty of Applied and Human Sciences, Universiti Malaysia Perlis Pauh Putra Main Campus, 02600 Arau, Perlis, Malaysia Email: mzaini@unimap.edu.my
1. INTRODUCTION
Fifty five years ago, the concept of fuzzy logic was introduced in [1]. Fuzzy logic was used as a part of artificial intelligence which resembles human behaviour that helps computer in making decisions. In recent years, the use of fuzzy logic techniques has increased significantly [2] The emergence of fuzzy logic can be seen in pattern recognition, controller design, economy management and decision making, communications and networking, aerospace applications and also in engineering sectors The researchers stated that there were a great number of researchers that interested in dealing with fuzzy logic to solve real world issues [3] This may lead to the innovation and industrialization in technologies, such as air conditioners, washing machine, antiskid braking systems and weather forecasting systems [4]. In 1974, the first study of fuzzy logic was conducted by using a model of a steam engine [5]. The fuzzy logic enables designers to control complex systemseffectivelybecauseit wasproven thatproportional, integral and differential (PID) controllers were less capable [6]Today,[8] the study of washing machine with fuzzy logic has gained attention among researchers. The implementation fuzzy logic in the washing machine will provide many benefits to the users, such as time saving, lowcostanditalsoresultsinbetterperformanceandproductivity.Thiswashingmachinealsopromotes‘one touch control’ to the users [9] Therefore, the researchers conducted the research on how the input variables affect the output variables by considering several factors of the variables. In 2013, a group of researchers introduced thedegreeofdirtandtype of dirt as input variables [10]. The authors described the concept of base ten minutes to decide the best washing time based on the input variables. Next, the amount of dirt, type of dirt, sensitivity of cloth and amount of cloth are studied to determine how these inputs will respond to different output
Article history: Received Dec 31, 2021 Revised May 24, 2022 Accepted Jun 2, 2022 The presence of a fuzzy logic system on the washing machines becomes a demand in every home as it simplifies human work. The uses of washing machine will facilitate the user, reduce electricity consumption, washing time, and water intake. Thus, in this paper, the fuzzy logic system is used to determine the washing time by considering four different inputs, which are type of fabric, type of dirt, dirtiness of fabric and weight of load. The possible rules from the input variables are developed by combining all the variables using fuzzy IF THEN rule. Referring to the Mamdani inference engine, a minimum membership function from input parts is truncated to the output for each of the rules. Next, the maximum membership function from the output is aggregated and the washing time can be calculated by using centroid method. The comparison is done by comparing the washing time of four input variables with three input variables.
IAES International Journal of Artificial Intelligence (IJ AI) Vol. 11, No. 3, September 2022, pp. 819 825 ISSN: 2252 8938, DOI: 10.11591/ijai.v11.i3.pp819 825 819
Four inputs-one output fuzzy logic system for washing machine
Article Info ABSTRACT
Keywords: Fuzzy logic system Mamdani method Washing time
1Institute of Engineering Mathematics, Faculty of Applied and Human Sciences, Universiti Malaysia Perlis, Perlis, Malaysia
Journal homepage: http://ijai.iaescore.com
This is an open access article under the CC BY SA license.
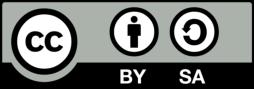
ISSN: 2252 8938 Int J Artif Intell, Vol. 11, No. 3, September 2022: 819 825
Figure 1. Fuzzy logic system process
2. METHOD In this paper, a simulation package in MATLAB which is fuzzy logic toolbox is used. This toolbox allows a few things to be done easily. Figure 1 shows the process of the fuzzy logic system [22] Several processes need to be considered before using a fuzzy logic toolbox. Fuzzy logic system is an alternative approach to control the process that involved a complex work that not easy to study manually [23]. The first step to build the fuzzy logic controller is to define the input and output variables. Since the boundaries of fuzzy sets may be vague, thus, there are no rules in generating the variables [24]. From Figure 2, four input variables are chosen, namely type of fabric, type of dirt, dirtiness of fabric and weight of load. As for the output variable, it is clear that washing time is the main component in developing a washing machine.
For each of the input and output variables, there exist a linguistic variable that allowed to take natural language as its values [25]. There are 14 linguistic variables for input and output variables, namely: silk (S), woolen (W), cotton (C), non greasy (NG), medium (M), greasy (G), small (Sm), large (La), light (Li), heavy (H), very short (VSh), short (Sh), long (Lo) and very long (VLo). The membership functions for each of the input and output variables are shown in Figure 3.
820variables
[11] Another studies presented the type of cloth, type of dirt and dirtiness of cloth as input variables in determining the washing time [12]. Besides, the fuzzy logic controllers use a Mamdani type to observe how five inputs and outputs variable responds to different conditions [13]. The input variables involved are type of dirt, turbidity of cloth, mass of cloth, sensitivity of cloth and water hardness while the output variables are washing time, wash speed, amount of water, amount of detergent and water hotness. In 2016, the fuzzy logic controller was designed for washing machine by incorporating five input and three output variables [14] A study on the washing time is also done when the amount of grease and dirtiness of cloth is put in the washing machine [15]. A relationship between four inputs and five outputs has been studied by other researchers [16] Other than that, a study was carried out by presenting four input variables which are type of cloths, dirtiness of cloths, amount of detergent and water [17]. This study proposed a neuro fuzzy controller where the controllers helped thesystem to take its own decisions. Wangand Ren [18] investigated another neural network fuzzy control. Hatagar and Halase provided the input variables of turbidity and turbidity change rate, as well as the output variable of washing time. Next, the spin time is studied as the output variable and the input variables are types of dirt, dirtiness of cloths and mass of cloths [19]. The fuzzy logic controller can be used to determine the washing speed when four inputs are considered [20]. Four input and output variables are studied in [21], where the inputs are dirtiness of cloths, type of fabric, type of dirt and volume of cloths. Whilst the outputs are water intake, water temperature, wash time and washing speed. Hence, to make the output of washing machine more efficient, the washing time is necessary to define as well as the users can estimate the total washing process. From the previous research, the authors used a few inputs related to the washing machine, but it can be seen that not many papers use weight of load as an input parameter in their study. Therefore, the main goal of this research is to present the fuzzy logic system for washing machine by considering four input variables and one output variable. Different from the previous research, one new parameter is considered, namely the weight of the load. The weight of the load is needed as the washer can weigh the cloths before starting the washing process in order to determine the best washing time. By considering the result of four input variables, we will make a time comparison with previous research. This new parameter will produce new results to identify the best washing time.
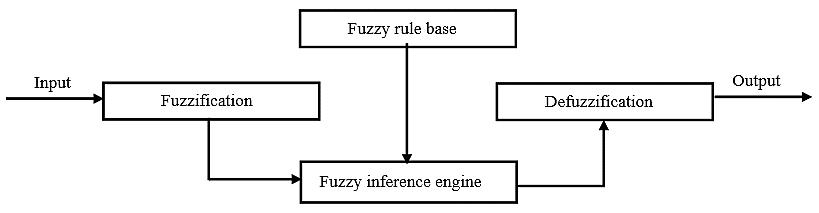
Figure 3. The membership functions of the input and output variables
Figure 2. The input and output variables in fuzzy logic system
Int J Artif Intell ISSN: 2252 8938 Four inputs one output fuzzy logic system for washing machine (Nurain Zulaikha Husin) 821
For type of fabric, it can be classified as silk, woolen and cotton. So, the user can choose from the selector whether it is a heavy or normal task, depending on the type of fabric they have. As for dirtiness of fabric, the sensor will determine it bylooking the water transparency. The sensor also will detect thetype ofdirt through the time saturation, wherethereisno change of wash waterat certain time. Besides,the load sensorthat has been installed in washing machine will weigh the load placed by the user. To ensure the clothes are washed thoroughly, the user can estimate the weight of load put into washing machine is less than the washer capacity. The second step in fuzzy logic system is fuzzification. Fuzzification is the process involving the transformation of crisp input into fuzzy input. The exact value of crisp inputs is measured by sensors in the machine and passed to the controller for processing. Figure 4 represents the crisp input (see red line) and the blue line represents the membership function of fuzzy input values for the input variables involved
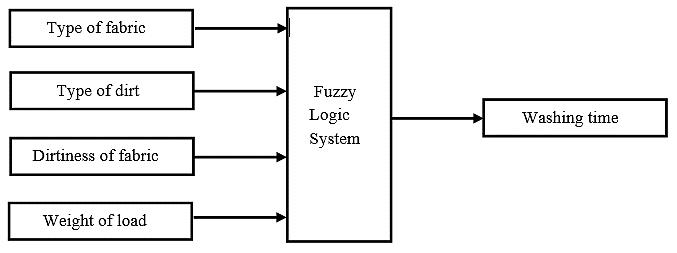
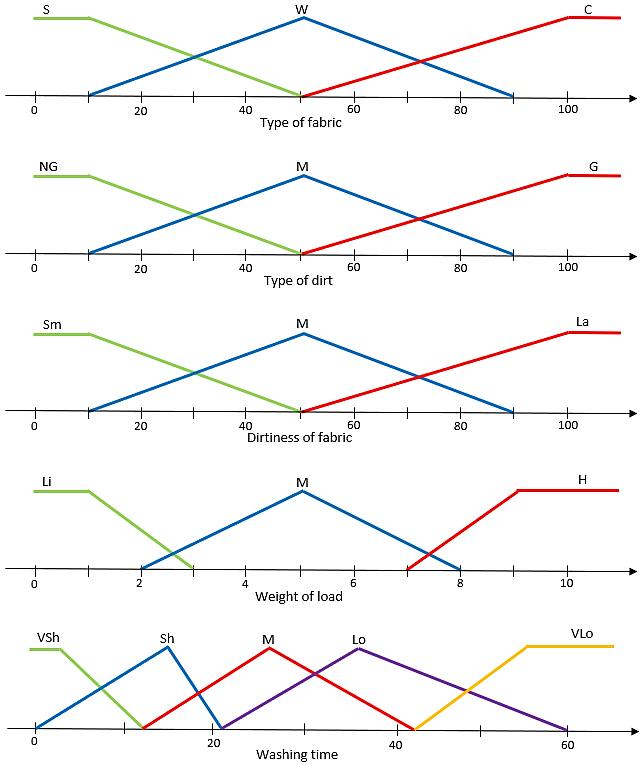
Then, the third step is to develop a fuzzy rule based on linguistic variables. A fuzzy rule base is a collection of linguistic statement that explain how the fuzzy inference system decides by classifying the input or controlling the output. Since we have the input and output variables, we then combine all the variables using fuzzy IF THEN rule. This rule can be obtained from the knowledge of experts. Table 1 shows fuzzy inference rule and the total number of rules involved is 81.
The rules declared above are not crisp but have fuzzy values. Therefore, the fuzzy inputs need to be convertedintoasinglefuzzyoutputbyemployingfuzzyimplicationwhichisMamdaniinferenceengine. Then, the AND operator is used to connect thefuzzyinput variablesfor each of therules. The function ofthis operator is to take a minimum value of membership functions from the fuzzy input variables. The fuzzy output variable is truncated using the value obtain from the input part. Thus, the entire truncated output is aggregated in one graph by taking the maximum value of membership degree and this will be used in the last step of fuzzy logic system. Thelast step infuzzylogicsystemis defuzzificationprocess.Defuzzificationis theprocess ofconverting the fuzzy output into crisp output. In order to find the crisp output, the centroid method is used where this method evaluates the center of area under the curve. The formula of this method is: Centroidmethod =��∗= ∫ ��(��)�������� ∫ ��(��)������ (1)
3. RESULTS AND DISCUSSION
81 C
No
In this section, the relationship between the input and output variables are analysed by using fuzzy logic system and the data will be run by fuzzy logic toolbox for the purpose of obtaining the washing time. In order to obtain the washing time, the crisp inputs need to be chosen first. In this study, the chosen crisp inputs for type of fabric, type of dirt,dirtiness of fabric and weight of load are 32,55, 48 and 7, respectively. The crisp inputs are imposed to the possible rules as shown in Figure 4. Then, the minimum value from fuzzy inputs is truncated to fuzzy output for each of the rules. Figure 5 shows how the maximum value of fuzzy outputs is aggerated in a single graph.
7
ISSN: 2252 8938 Int J Artif Intell, Vol. 11, No. 3, September 2022: 819 825 822
Table 1. Fuzzy logic rules for washing machine of rules Type of fabric Type of dirt Dirtiness of fabric Weight of load Washing time 1 S NG Sm Li VSh 2 S NG Sm M Sh 3 S NG Sm H M 4 S NG M Li Sh 5 S NG M M M 6 S NG M H M S NG La Li M La La H VLo
Figure 4. An illustration of fuzzification process
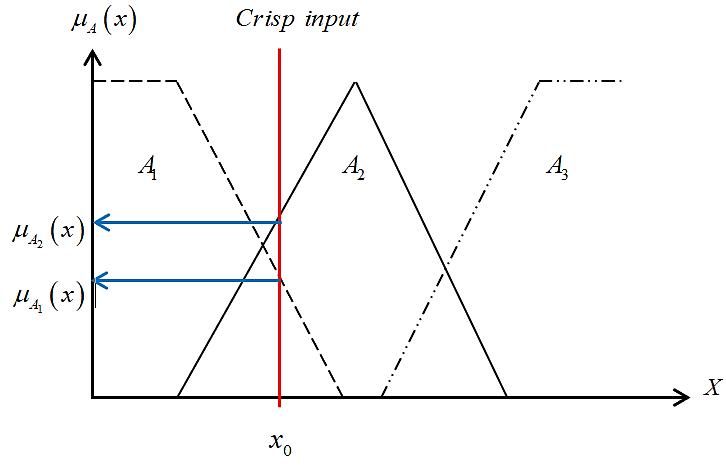
Int J Artif Intell ISSN: 2252 8938 Four inputs one output fuzzy logic system for washing machine (Nurain Zulaikha Husin) 823
Based on Figure 5, the membership function is obtained as: ��4 inputs(��) ={00733�� 08795,120≤�� <165 0.33,16.5≤�� <52.5 00440��+264,525≤�� ≤60 0,otherwise (2) By substituting (2) into (1), the washing time is calculated as in (3). The time required to wash the clothes for four input variables is 35.3 minutes This answer coincides with the answer obtained from fuzzy logic toolbox Washingtime = ∫��(��) ������ ∫��(��)���� = ∫ (00733�� 08795)165 12 ������+∫ 033������+∫ ( 00440��+264)������60 525 525 165 ∫ (00733�� 08795)165 12 ����+∫ 033����+∫ ( 00440��+264)����60 525 525 165 =35.3minutes (3)
Figure 5. An aggregation of output for four input variables
Next, the study of four input variables is compared with three input variables where the weight of load is excluded. Thus, the input variables involved are type of fabric, type of dirt and dirtiness of fabric. By using the same steps as stated in section 2, the crisp inputs are applied to each input involved and imposed on the possible rules. By considering the same process as in Figure 4, the minimum value of membership functions from fuzzy input variables is truncated onto a fuzzy output variable. Hence, the maximum value from fuzzy output is aggregated into one graph as shown in Figure 6. As shown in (4), the membership functions for three input variables are obtained by extracting all the information from Figure 6. ��3 inputs(��) = { 00647�� 07764,120≤�� <205 0.55,20.5≤�� <34.0 00667��+28179,340≤�� <355 045,355≤�� <490 0.0409��+2.454,49.0≤�� ≤60.0 0,otherwise . (4) Referring to (4), the washing time for three input variables is 34.45 minutes and details of the calculation are shown in (5). Washingtime=∫��(��).������ ∫��(��)����
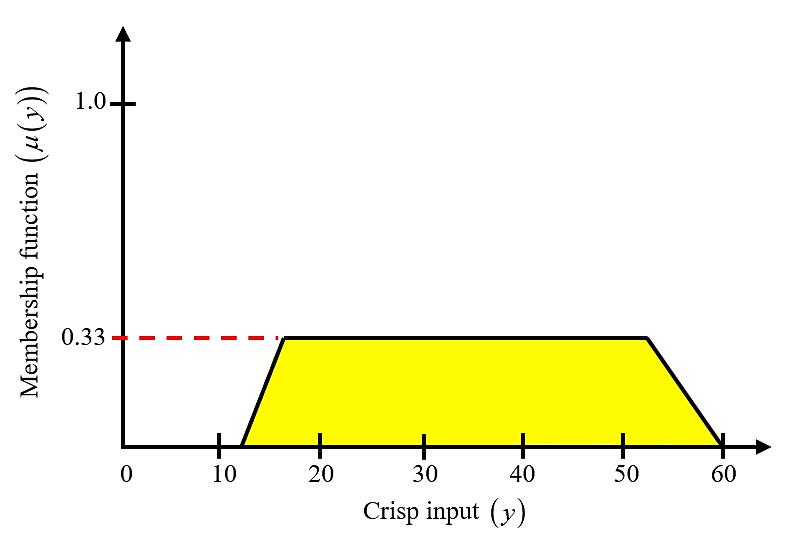
[6] M. M. Gouda, S. Danaher, and C. P. Underwood, “Fuzzy Logic Control Versus Conventional PID Control for Controlling Indoor Temperature of a Building Space,” IFAC Proc. Vol., vol. 33, no. 24, pp. 249 254, 2000, doi: 10.1016/s1474 6670(17)36900 8.
[7] M. J. Yusoff, N. F. N. Ismail, I. Musirin, N. Hashim, and D. Johari, “Comparative study of Fuzzy Logic controller and Proportional Integral Derivative controller on {DC} {DC} Buck Converter,” Jun. 2010, doi: 10.1109/peoco.2010.5559170.
ISSN: 2252 8938 Int J Artif Intell, Vol. 11, No. 3, September 2022: 819 825 824 = ∫ (00647�� 07764)������+∫ 055������+∫ ( 00667��+28179)������+355 34 34 205 205 12 ∫ 045������+∫ ( 00409��+2454)������60 49 49 355 ∫ (0.0647�� 0.7764)����+∫ 0.55����+∫ ( 0.0667��+2.8179)����+355 34 34 205 205 12 ∫ 0.45����+∫ ( 0.0409��+2.454)����60 49 49 355 =34.45minutes (5)
Figure 6. An aggregation of output for three input variables
REFERENCES [1] L. A. Zadeh, “Fuzzy sets,” Inf. Control, vol. 8, no. 3, pp. 338 353, 1965, doi: 10.1016/S0019 9958(65)90241 X.
[2] M. R. S. Emami, “Fuzzy Logic Applications In Chemical Processes,” J. Math. Comput. Sci., vol. 1, no. 4, pp. 339 348, 2010, doi: 10.22436/jmcs.001.04.11. [3] R. Kumari, V. K Sharma, and S. Kumar, “Design and Implementation of Modified Fuzzy based CPU Scheduling Algorithm,” Int. J. Comput. Appl., vol. 77, no. 17, pp. 1 6, 2013, doi: 10.5120/13612 1323. [4] H. Singh et al., “Real life Applications of Fuzzy Logic,” Adv. Fuzzy Syst., vol. 2013, pp. 1 3, 2013, doi: 10.1155/2013/581879.
[5] E. H. Mamdani, “Application of Fuzzy Algorithms for Control of Simple Dynamic Plant,” in Proceedings of the Institution of Electrical Engineers, 1974, vol. 121, no. 12, pp. 1585 1588, doi: 10.1049/piee.1974.0328.
4. CONCLUSION In this paper, the analysis clearly states the advantages of fuzzy logic in solving washing machine problem. By adding some of the intelligence, the users do not have to worry about their washing process. Four input variables were successfully studied in order to achieve optimal washing time. The result of four input variables was compared with three input variables and there is a slight time difference between these two studies. Therefore, the washing machine with four input variables can be classified as comparable controller with human behaviour as the washed clothes becomes cleaner and the time taken is suitable in accordance with the proposed input compared to washing machine with three inputs. It can be said that the washing machine with fuzzy logic controller is a great combination of technology and intelligence. For future work, to make the controller of washing machine works smoothly, more input variables can be implemented in washing machine in order to estimate the best washing time. Other than that, for future research, the researchers can use another method, namely the Sugeno method, to analyse the washing time and compare the result with existing research.
Thus, by comparing these two results, it can be concluded that there is a slightly difference in time between these two studies. The comparison shows that there is a superior timing between four and three inputs variable because the number of possible rules also varies. As the weight of the load is considered, the controller can function smoothly and efficiently in ensuring that the clothes are washed properly. If we change the value of the crisp input, then the result will also be different. To conclude this point, the washing time depends on the crisp input value as all the rules involved need to be considered.
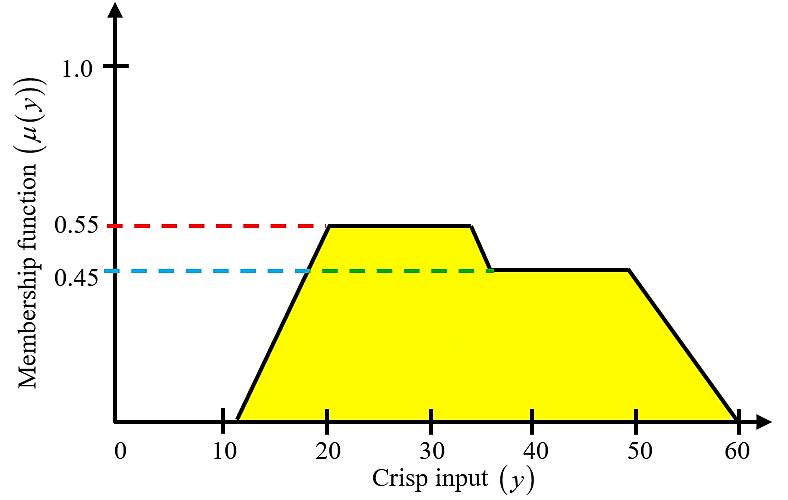
[14] T. Ahmed and A. Ahmad, “Fuzzy logic controller for washing machine with five input and three output,” Int. J. Latest Trends Eng. Technol., vol. 7, no. 2, pp. 136 143, 2016, doi: 10.21172/1.72.523. [15] M. A. Islam and M. S. Hossain, “Optimizing the wash time of the washing machine using several types of fuzzy numbers,” J. Bangladesh Acad. Sci., vol. 45, no. 1, pp. 105 116, 2021, doi: 10.3329/jbas.v45i1.54432.
[8] T. Ahmed and A. Toki, “A Review on Washing Machine Using Fuzzy Logic Controller,” vol. 4, no. 7, pp. 64 67, 2016. [9] K. Raja and S. Ramathilagam, “Washing machine using fuzzy logic controller to provide wash quality,” Soft Comput., vol. 25, no. 15, pp. 9957 9965, 2021, doi: 10.1007/s00500 020 05477 4. [10] M. Alhanjouri and A. A. Alhaddad, “Optimize wash time of washing machine using fuzzy logic,” in The 7th International Conference on Information and Communication Technology and Systems (ICTS 2013), 2013, pp. 77 80. [11] M. Demetgul, O. Ulkir, and T. Waqar, “Washing Machine Using Fuzzy Logic,” Autom. Control Intell. Syst., vol. 2, no. 3, pp. 27 32, 2014, doi: 10.11648/j.acis.20140203.11.
BIOGRAPHIES OF AUTHORS Nurain Zulaikha Husin completed her bachelor degree of Mathematics in 2017 from Universiti Teknologi MARA (UiTM) Kelantan, Malaysia. She received a master degree in Engineering Mathematics from Universiti Malaysia Perlis in 2018. She can be contacted at email: nuhazulaikha94@gmail.com.
[21] I. Iancu and M. Gabroveanu, “Fuzzy Logic Controller Based on Association Rules,” Math. Comput. Sci. Ser., vol. 37, no. 3, pp. 12 21, 2010. [22] S. S. Jamsandekar and R. R. Mudholkar, “Performance Evaluation by Fuzzy Inference Technique,” Int. J. Soft Comput. Eng., vol. 3, no. 2, pp. 158 164, 2013. [23] L. A. Q. Aranibar, “Learning fuzzy logic from examples,” Ohio University, 1994. [24] J. F. Silva and S. F. Pinto, Linear and Nonlinear Control of Switching Power Converters. 2018. [25] L. A. Zadeh, “The concept of a linguistic variable and its application to approximate reasoning I,” Inf. Sci , vol. 8, no. 3, pp. 199 249, 1975, doi: 10.1016/0020 0255(75)90036 5.
[16] K. A. Kareem and W. H. Ali, “Implementation of Washing Machine System Via Utilization of Fuzzy Logic Algorithms,” in Proceedings ISAMSR 2021: 4th International Symposium on Agents, Multi Agents Systems and Robotics, 2021, pp. 45 50, doi: 10.1109/ISAMSR53229.2021.9567796. [17] M. N. Virkhare and P. R. W. Jasutkar, “Neuro Fuzzy Controller Based Washing Machine,” Int. J. Eng. Sci. Invent , vol. 3, no. 1, pp. 48 51, 2014. [18] A.Z.Wang and G.F.Ren,“Thedesign ofneuralnetworkfuzzy controllerinwashing machine,”in Proceedings 2012 International Conference on Computing, Measurement, Control and Sensor Network, CMCSN 2012, 2012, pp. 136 139, doi: 10.1109/CMCSN.2012.35. [19] S. S. Hatagar and S. S. V Halase, “Simulating Matlab Rules in Fuzzy Controller Based Washing Machine,” Int. J. Emerg. Trends Sci. Technol., vol. 2, no. 7, pp. 2796 2802, 2015. [20] N. Wulandari and A. G. Abdullah, “Design and Simulation of Washing Machine using Fuzzy Logic Controller (FLC),” IOP Conf. Ser. Mater. Sci. Eng., vol. 384, pp. 1 8, 2018, doi: 10.1088/1757 899X/384/1/012044.
[12] S. Hatagar and S. V. Halase, “Three Input One Output Fuzzy logic control of Washing Machine,” Int. J. Sci. Res. Eng. Technol , vol. 4, no. 1, pp. 57 62, 2015.
Muhammad Zaini Ahmad is currently an associate professor at the Institute of Engineering Mathematics, Faculty of Applied and Human Sciences, Universiti Malaysia Perlis, from 2003 till date. He obtained Ph.D. from Universiti Kebangsaan Malaysia in 2012, specialising in the field of Industrial Computing. He has published research papers in various international journals. His research interest includes fuzzy set theory, differential equations and mathematics for decision making. He can be contacted at email: mzaini@unimap.edu.my.
Int J Artif Intell ISSN: 2252 8938 Four inputs one output fuzzy logic system for washing machine (Nurain Zulaikha Husin) 825
[13] M. Agarwal, A. Mishra, and A. Dixit, “Design of an Improved Fuzzy Logic based Control System for Washing Machines,” Int. J. Comput. Appl., vol. 151, no. 8, pp. 5 10, 2016.
Mustafa Mamat is currently a Professor in the Faculty of Informatics and Computing at the Universiti Sultan Zainal Abidin (UniSZA) Malaysia since 2013. He was first appointed as a Lecturer at the Universiti Malaysia Terengganu (UMT), in 1999. He obtained his Ph.D. from the UMT in 2007 with a specialization in optimization. He was appointed as a Senior Lecturer in 2008 and as an Associate Professor in 2010 also the UMT. To date, he has published more than 380 research papers in various international journals and conferences. His research interest in applied mathematics, with a field ofconcentration ofoptimization, includes conjugate gradient methods, steepest descent methods, Broyden’s family, and quasi Newton methods. He can be contacted at email: must@unisza.edu.my.
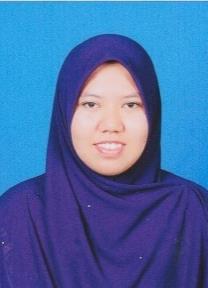



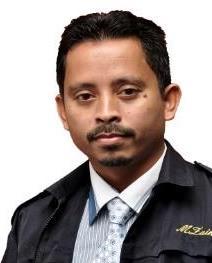



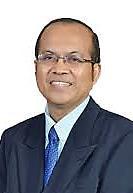



Journal homepage: http://ijai.iaescore.com
Department of Agricultural Socio Economic, Faculty of Agriculture, Universitas Brawijaya, Malang, Indonesia
IAES International Journal of Artificial Intelligence (IJ AI) Vol. 11, No. 3, September 2022, pp. 826 835 ISSN: 2252 8938, DOI: 10.11591/ijai.v11.i3.pp826 835 826
1Department of Informatics Engineering, Faculty of Computer Science, Universitas Brawijaya, Malang, Indonesia
Wayan Firdaus Mahmudy1, Nindynar Rikatsih1,2, Syafrial3
This is an open access article under the CC BY SA license.
3
Genetic SubMigrationKnapsackalgorithmproblempopulation
Corresponding Author: Wayan Firdaus Mahmudy Department of Informatics Engineering, Faculty of Computer Science, Brawijaya University Ketawanggede, Kec. Lowokwaru, Kota Malang, Jawa Timur 65145, Indonesia Email: wayanfm@ub.ac.id
Fresh agricultural products are a daily need for the community. The products are perishable and must be stored at the proper condition before being shipped to distributors or retailers [1]. In doing product storage, we need to pay attention to the product value and the profit that must be gained. For agricultural products that the stock needs to be always available with a good quality, trader has to maximize number of items in the storage and maximize the total profit without exceed the capacity of storage. Therefore, trader should do the right way of product storage to get maximum profit. The storage of fresh agricultural products problem could be addressed as a knapsack problem. Knapsack problem is a combinatorial and optimization problem that is often encountered in daily life and real industrial problems such as project selection, capital budgeting, cargo loading, and bin packing. [2], [3]. Knapsack problem also has a set of application for budgeting project, selection of items, material, and cost effective development [4] [6]. Optimization of knapsack problems is implemented to determine a number of items with a certain value that will be included into a container without exceeding the capacity of the container. The item selection is expected to provide maximum profit [7].
The storage of fresh agricultural products is a combinatorial problem that should be solved to to maximize number of items in the storage and also maximize the total profit without exceed the capacity of storage. The problem can be addressed as a knapsack problem that can be classified as NP hard problem. We propose a genetic algorithm (GA) based on sub population determination to address the problem. Sub population GA can naturally divide the population into a set of sub population with certain mechanism in order to obtain a better result. GA based on sub population is applied by generating a set of sub population which is happened in the process of initializing population. A special migration mechanism is developed to maintain population diversity. The experiment shows GA based on sub population determination provide better results comparable to those achieved by classical GA
1. INTRODUCTION
Agricultural
Article history: Received Sep 13, 2021 Revised Mar 17, 2022 Accepted Apr 15, 2022
2
Optimization of agricultural product storage using real-coded genetic algorithm based on sub-population determination
Article Info ABSTRACT
Various methods have been developed to solve knapsack problems such as local search, heuristics, meta heuristic, and hybridization methods. For example, a local search is modified to efficiently solve a large scale knapsack problem [8]. An evolutionary algorithm based approach is developed to solve hardware/software partitioning that is considered as a variant of knapsack problem [9]. A hybrid approaches are also developed to addres the complexity of the knapsack problem. For instance, ant colony optimization
Keywords: product
Department of Informatics, Institute of Technology, Science and Health of dr. Soepraoen Hospital, Malang, Indonesia
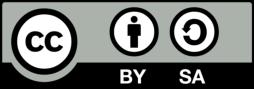
2.1. Problem statement
This section is divided into three parts that consists of problem statement, proposed fitness function to evaluate the quality of solutions, and mechanism of sub population GA (SPGA) with a special migration. First, we make the detail of statement problem to prepare the basic formulation to create fitness function. Then, we present fitness function before entering the main procedure of SPGA.
Optimization of agricultural product storage using real coded … (Wayan Firdaus Mahmudy) 827 and differential evolution algorithm are combined to explore strong characteristic of each method to solve different part the knapsack problem [10] In this research we propose genetic algorithm (GA) to solve the problem because it is simple, easy to use and has a wide search area. GA is one of the meta heuristic methods which has been proven that can be used to solve knapsack problems [11], [12]. GA is a meta heuristic search algorithm that can provide optimal solutions by adopting mechanism of biological evolution and natural selection [13], [14]. GA consists of several steps including population initialization, crossover and mutation as a reproductive process to produce new solutions and the last is selection to get the best solution [15], [16]. GA is proved to effectively solve the optimization problem [17] [19]. However, GA has weakness that is easy to be trapped in local optimum [20], [21]. It happens when the population of GA reaches a suboptimal state that the genetic operators produce offspring with a performance that can not be better than their parents [22]. The previous research uses dynamic genetic clustering algorithm and elitist technique to prevent premature convergence in GA [9]. A proper combination of crossover and mutation methods may be used to increase the GA performance [23]. The other research uses hybrid adaptive GA to overcome GA weakness. It combines GA with other algorithms to get better solutions [24] [28]. The other way to solve GA weakness is by giving random injection [29] To solve that weakness of GA, we propose GA based on subpopulation. This approach is adopting mechanism of parallel GA with the population that is naturally divided into a number of sub populations that evolve and converge with a significant independence level [30]. Parallel GA can improve computational efficiency over classical GA. It also facilitates parallel exploration of solution space to get the better solutions [31]. GA based on sub population determination solves GA weakness by keeping individual variety by setting the best individual of one sub population to another population. A special migration mechanism is developed to maintain population diversity [32]. It is applied to get out of local optimum that cause premature convergence and increase the quality of the solutions. Therefore, in this research we propose GA based on sub population determination to solve knapsack problem of agricultural product storage
Int J Artif Intell ISSN: 2252 8938
2. THE PROPOSED APPROACH
Table 1. Example of product quantity combination
No Product 1 Product 2 Product 3 Product 4 Product 5 Product 6 Product 7 Product 8 1 4,000 1,500 2,000 200 200 150 150 150 2 5,500 4,000 1,000 300 500 150 100 200 3 3,000 5,000 1,500 200 100 150 300 200 4 5,000 2,000 1,500 300 170 100 250 150 5 2,000 4,000 3,000 100 100 250 150 100
Based on Table 1 there are five combinations of the product quantity where the first one shows that the quantity of product 1 is 4,000 kg, product 2 is 1,500 kg, product 3 is 2,000 kg and the last product which is product 8 is 150 kg. Trader should find the appropriate quantity combination of the product because the quantity combination affects the profit. The calculation of gaining profit is showed in (1) to (5). =(∑ ����1������ �� ��=1 )+����2 (1) ���� =(∑ ����1������ �� ��=1 ) (2)
The problem addressed in this study is optimization of agricultural product storage that is conducted by traders. The agricultural product is stored in storage with certain quantity in kilograms without exceed the capacity and represented by product 1, product 2, product 3, and so on. In this study, it is assumed that the available capacity is 5,000 kg. Although storing more items will potentially get higher profits, but there are additional costs that must be incurred if the items exceed the storage capacity. If the agricultural product quantity exceeds the available capacity, it is necessary to rent a storage place to another party at a cost of IDR 100 per kg. Example of possible solutions are presented in Table 1.
����
ISSN: 2252 8938 Int J Artif Intell, Vol. 11, No. 3, September 2022: 826 835 828 ������������������ = ∑ (����1������1�� +�� ��=1 ����2������2��) (3) ������������ =���� ���� (4) ���������������������������� =������������������ ���� (5) ������ is the total profit which is gained from total revenue and total cost. Total revenue is represented by ���� while total cost is represented by ���� ���� is obtained from the purchase price per product (����1) multiplied by quantity (��) and summed by other expenses represented by ����2 ���� is the selling price per product represented by ����1 and multiplied by Q. Maximum profit represented by ������������. ������������ is obtained by all products that are stored as many as Q without exceeding the capacity. Those all products also should be sold out. However, not all products are sold out because the products are sold according to demand represented by ����1 with a selling price of ����1 so that there was a remaining stock of ����2 which experienced a decrease in the selling price of ����2. Therefore, the actual income obtained is the sales profit based on demand and stock sales with a decrease in price which is defined as the current total revenue ������������������. So that the profit is generated from total income minus expenses, namely as potential losses. An example of calculating profit is presented in Table 2.
Fitness value represents the quality of the solution produced by GA. The solution that provides maximum benefit is considered as a good solution. High fitness value represents high quality of solution which shows the high profit that can be provided. The determination of fitness function depends on calculating profit based on formulas (1) to (5). Hence, we consider the fitness function that is showed in (6).
Total revenue (TR) 25,240,000 Stock (kg) 1,000 500 500 40 20 50 30 50 Selling price of stock per kilogram 3,500 6,000 1,000 4,500 8,500 10,000 5,000 3,000 Selling price of stock 3,500,000 3,000,000 500,000 180,000 170,000 500,000 150,000 150,000 Total revenue (TR) of stock 8,150,000
Product Product 1 Product 2 Product 3 Product 4 Product 5 Product 6 Product 7 Product 8 Product quantity (kg) 4,000 1,500 2,000 200 200 150 150 150 Purchase cost per kilogram (Rp) 3,200 5,500 1,500 3,000 8,000 9,000 5,000 2,500 Purchase cost of each product (Rp) 12,800,000 8,250,000 3,000,000 600,000 1,600,000 1,350,000 750,000 375,000 Total purchase cost (Rp) 28,725,000 Expenses (Rp) 3,075,000 Excess capacity cost (Rp) 335,000 Total cost (TC) 32,135,000 Market demand (kg) 3,000 1,000 1,500 160 180 100 120 100 Selling price per kilogram 3,700 6,500 2,000 5,000 9,000 11,000 6,000 4,000 Selling price according to market demand 11,100,000 6,500,000 3,000,000 800,000 1,620,000 1,100,000 720,000 400,000
2.2. Proposed fitness function
Table 2. Profit calculation
2.3. Special migration on sub population genetic algorithm (GA) The GA is proposed by John Holand in 1975. GA is a heuristic method that mimic the mechanism of biological evolution and applies natural selection to obtain optimal solutions [5]. We apply classical GA with sub population to solve the problem in this study.
Current total revenue (TR current) 33,390,000
�������������� = 1000 ������������ ���������������������������� (6)
Maximum selling price 14,800,000 9,750,000 4,000,000 1,000,000 1,800,000 1,650,000 900,000 600,000 Maximum total revenue max (TR max) 34,500,000 Maximum total profit (TPF max) 2,365,000 Potential Loses 1,255,000
SPGA begins with population initialization and being continued by crossover, mutation, evaluation, migration between sub population and finally the selection. Based on the approaches in knapsack problems, we propose real coded chromosome representation as shown in Figure 1. Each number represents the quantity of product stored. The chromosomes which have been initialized are improved by a reproduction process consisting of one cut point crossover and random mutation. The crossover and mutation mechanisms are shown in Figure 2 and Figure 3. In the crossover process, each child inherits some of the genes from the parent. In the mutation process, some genes from the parent are shifted to produce a child. After reproduction process, then it goes into evaluation to combine the reproductive chromosomes with the existing population. The selected chromosomes will be passed to the next generation.
Optimization of agricultural product storage using real coded … (Wayan Firdaus Mahmudy) 829
3.1. General steps of genetic algorithm
Each subpopulation improves separately. There is one individual in each subpopulation that will be migrated to the next subpopulation in order to improve the variety of solutions. The migrations scheme is presented in Figure 4.
Sub population GA which is called SPGA in this research adopts mechanism of parallel GA with the population that is naturally divided into a number of sub populations [33]. Similarity of sub population GA and single population GA is both of them applies several steps that are preceded by initialization of the population containing chromosomes that represent the solution. Furthermore, chromosomes are developed to get new variations by using crossover mutation process and selection. However, there is the difference of Sub population GA with respect to single population GA that each sub population of SPGA iterates in parallel and share each other their individuals that are called migrant to improve the solutions. There are many ways to implement SPGA but this study uses a different SPGA from the other SPGA. We use Euclidean Distance in migrating the solutions, thus we call it Euclidean Distance sub population GA (EDSPGA). The Euclidean Distance will be explained in section of migration.
3.2. ED Migration of SPGA
Figure 1. Real coded chromosome representation Figure 2. One cut poin crossover Figure 3. Insertion mutation
3. METHODOLOGY
Int J Artif Intell ISSN: 2252 8938
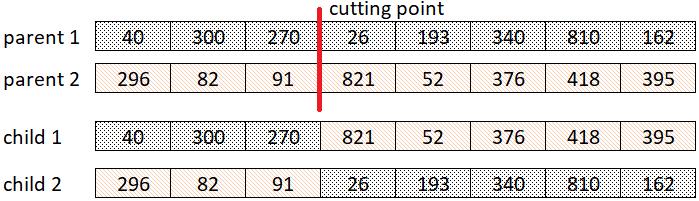
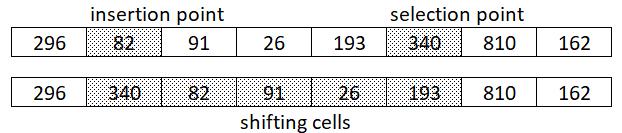
4. EXPERIMENTAL RESULT AND ANALYSIS
Ten individuals from first subpopulation and the best individual from the next population have been choosen. The ten individuals of first subpopulation are compared to the best individual of next subpopulation by using one dimention Euclidean Distance. The Euclidean Distance formulation is presented in (1).
Figure 4. Migration sceme
���� = √(��1 ��2)2 (7) ED means the distance between chromosome x1 and x2. x1 is a chromosome on the first sub population and x2 is a chromosome on the second sub populasi. By applying Euclidean Distance formulation, we cam found the chromosomes with the longest distance. The chromosome with the longest distance will replaces the best individual in the next subpopulation.
This section explains the experimental results of all three methods performance consists of classical GA, SPGA and EDSPGA. The results refer to parameter testing of classical GA as the basis SPGA and EDSPGA parameter testing. First, we evaluate the parameter of classical GA that consist of population size (popsize) testing showed in Figure 5 for fitness value and Figure 6 for computational time. Figure 5 shows that the convergence point is at popsize 600. Popsize is tested from 10 and stopped at 1,000 because after popsize 600 there is no significant increase in fitness value. While it is showed in Figure 6 that the computational time is continuously increasing as the popsize value increases.
ISSN: 2252 8938 Int J Artif Intell, Vol. 11, No. 3, September 2022: 826 835 830
Figure 5. Fitness value in population size testing

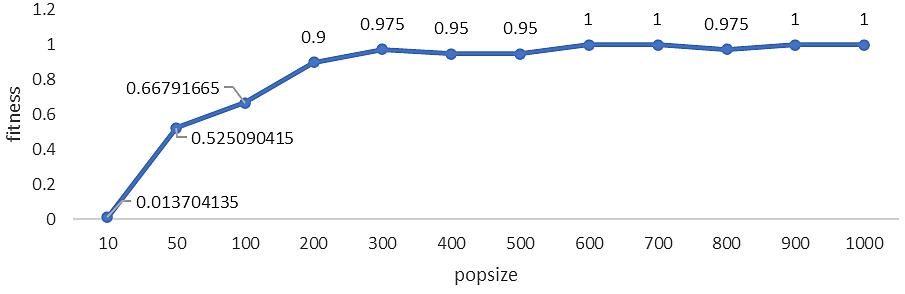
Figure 7. Fitness value in number of generations test
The next parameter test is number of generations testing showed in Figure 7 for fitness value and Figure 8 for computational time. Figure 7 shows that there is no significant change in the fitness value after 70 generations. Therefore, the best solution can be reached at 70 generations. Meanwhile, the computational time increase continuously as generation raises as shown in Figure 8. In this case, we found that small number of generations with the bigger population size can provide better result than small number of population size with bigger number of generations.
Good result is a good solution that is provided not only with a good fitness value but also a short computational time. Therefore, we set the number of generations as 70 wich is got from the test before with popsize 600. Last parameter testing of classical GA is crossover rate (cr) and mutation rate (mr) combination
Figure 8. Computational time in number of generations test
The test uses 600 populations and 70 generations that is showed in Figure 9 for fitness value and Figure 10 for computational time.
Figure 6. Computational time in population size testing
Int J Artif Intell ISSN: 2252 8938 Optimization of agricultural product storage using real coded … (Wayan Firdaus Mahmudy) 831
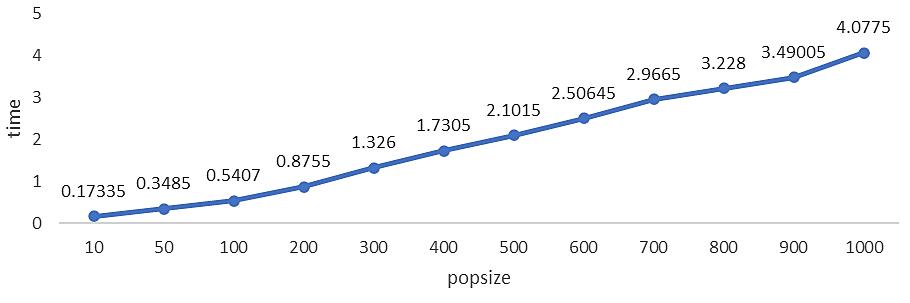
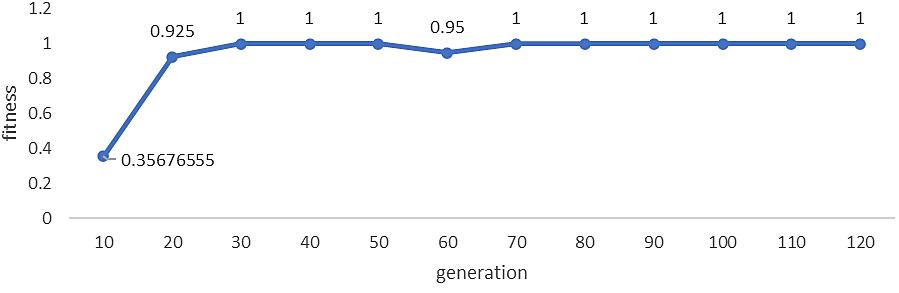
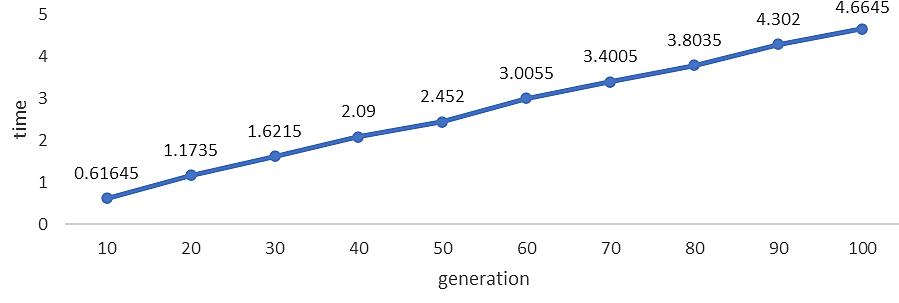
ISSN: 2252 8938 Int J Artif Intell, Vol. 11, No. 3, September 2022: 826 835 832
Test for cr and mr is carried out several times the best combination values of cr and mr. The values are used for population size and number of generation test. Figure 9 showed that the best value of cr and mr is 0.8 and 0.2 respectively. However, the computation time is lower in cr and mr of 0.9 and 0.1 with slighthy lower fitness value. Therefore, cr and mr used in this case are 0.9 and 0.1. The best values of each parameter of classical GA is used to discover the best number of subpopulations in SPGA and EDSPGA that is showed in Figure 11 for fitness value and Figure 12 for computational time
Figure 10. Computational time in cr and mr testing
Figure. 11 Fitness comparation of SPGA and EDSPGA
Crossover and mutation rates for SPGA and EDSPGA are 0.9 and 0.1, referring to the results of the classical GA parameter tests that were carried out previously. The generation is determined to be 20 because there are no significant changes in the 20th generation of SPGA. Population size and number of sub
Figure. 9 Fitness value in cr and mr testing
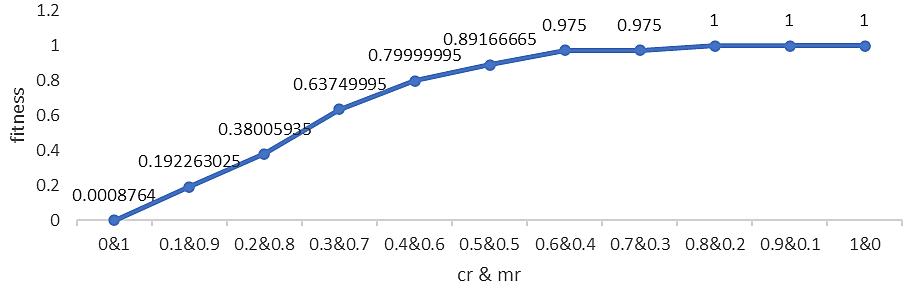
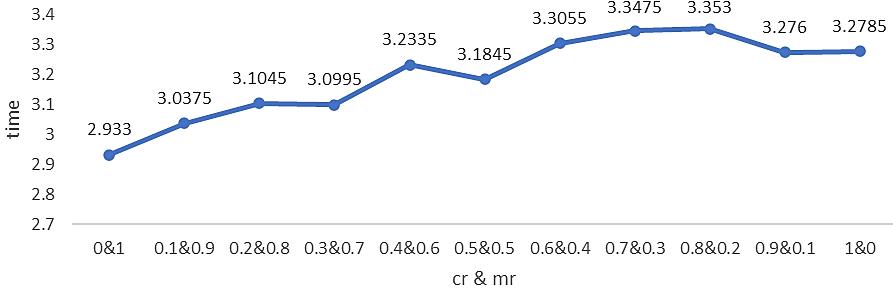
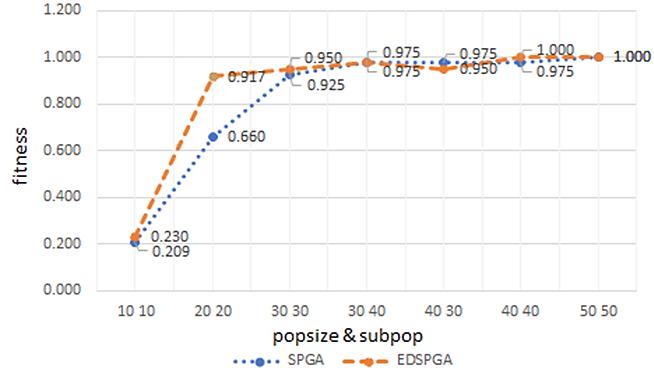
Figure 12. Computational time of SPGA and EDSPGA
12
Table 3. Test Result of GA, SPGA, and SPGA with eulidean distance Test GA SPGA EDSPGA Fitness Time Fitness Time Fitness Time 1 0.055555 0.158 1.0 2.81 1.0 2.1 2 0.142857 0.0624 1.0 2.62 1.0 2.17 3 0.058823 0.0625 1.0 2.56 1.0 1.04 4 0.076923 0.0625 1.0 2.63 1.0 2.08 5 0.166666 0.0817 1.0 2.51 1.0 2.04 6 0.333333 0.0625 0.5 2.67 1.0 2.1 7 0.25 0.0468 1.0 2.66 1.0 2.12 8 0.5 0.0781 1.0 2.45 1.0 2.06 0.333333 0.0683 1.0 2.71 1.0 2.02 10 0.02439 0.0575 1.0 2.77 1.0 2.13 0.071428 0.0625 1.0 2.57 1.0 2.24 0.05 0.0625 1.0 2.72 1.0 2.17 13 0.037037 0.0867 1.0 2.69 1.0 2.24 0.125 0.0539 1.0 2.53 1.0 2.16 15 0.5 0.0751 1.0 2.49 1.0 2.33 16 0.017543 0.0583 1.0 2.44 1.0 2.25 17 0.2 0.0697 1.0 2.62 1.0 2.27 18 0.166666 0.0625 1.0 2.76 1.0 2.1 0.111111 0.0708 1.0 2.84 1.0 2.27 20 0.25 0.0781 1.0 2.76 1.0 2.15 Average 0.173533 0.07102 0.975 2.6405 1.0 2.102
11
19
Optimization of agricultural product storage using real coded … (Wayan Firdaus Mahmudy) 833
9
populaton tests are carried out concurrently because high popsize does not always provide a better fitness value but provides a longer computational time referring to Figure 11. This is influenced by the generated number of sub population. In Figure 8 it can be seen that population size of 40 and sub population of 40 produce the highest fitness value and faster computation time than the larger number of popsize and subpop.
Int J Artif Intell ISSN: 2252 8938
14
Figure 11 shows that average fitness value of SPGA and EDSPGA are equally increase at population size of 30 and sub population of 40 which is 0.975. Although at population size of 40 and sub population of 30 EDSPGA decreased by 2.56% compared to SPGA, EDSPGA iss able to provide a higher increase than SPGA without much different of the computational time from the previous population size of 20 and sub population of 20. It shows that EDSPGA directly gives better fitness value not far from begining. Therefore, we can say that the performance of EDSPGA is better than SPGA for the same number of popsizes and subpops. However, in this case, we found that in EDSPGA, a low populationsize along with a higher sub population number provides better fitness value. This is indicated by an increase of fitness value on population size of 30 and sub population number of 40 from 0.95 to 0.975 and a decrease in the fitness value on population size 40 and sub population number of 30 from 0.975 to 0.95. Based on the experiments that have been done, we compare the performance of SPGA with ED respect to classical GA and SPGA without ED. The comparation of fitness average is showed in Table 3
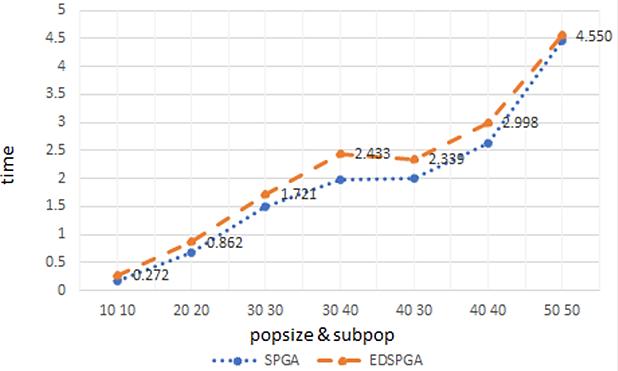
5. CONCLUSION
[13] A. Rahmi, W. F. Mahmudy, and M. Z. Sarwani, “Genetic algorithms for optimization of multi level product distribution,” Int. J. Artif. Intell., vol. 18, no. 1, pp. 135 147, 2020 [14] Q. Kotimah, W. F. Mahmudy, and V. N. Wijayaningrum, “Optimization of fuzzy Tsukamoto membership function using genetic algorithm to determine the river water,” Int. J. Electr. Comput. Eng., vol. 7, no. 5, pp. 2838 2846, Oct. 2017, doi: 10.11591/ijece.v7i5.pp2838 2846. [15] V. Meilia, B. D. Setiawan, and N. Santoso, “Extreme learning machine weights optimization using genetic algorithm in electrical load forecasting,” J. Inf. Technol. Comput. Sci., vol. 3, no. 1, pp. 77 87, 2018 [16] Z. A. Ali, S. A. Rasheed, and N. N. Ali, “An enhanced hybrid genetic algorithm for solving traveling salesman problem,” Indones. J. Electr. Eng. Comput. Sci., vol. 18, no. 2, pp. 1035 1039, 2020, doi: 10.11591/ijeecs.v18.i2.pp1035 1039.
REFERENCES
[17] S. D L, “Energy efficient intelligent routing in WSN using dominant genetic algorithm,” Int. J. Electr. Comput. Eng., vol. 10, no. 1, pp. 500 511, Feb. 2020, doi: 10.11591/ijece.v10i1.pp500 511.
Table 3 summarizes the computational time of classical GA is 0.07102 seconds, SPGA is 2.6405 seconds and SPGA with Euclidean Distance is 2.012 seconds. SPGA reaches the higher fitness value of 2.102, while classical GA is 0.173533 and basic SPGA 0.95. Even the computational time of EDSPGA is longer than classical GA and basic SPGA, we have found that EDSPGA giving the best result in fitness average among classical GA and basic SPGA. Thus, it proves the effectiness of the proposed migration mechanism to maintain population diversity and avoid an early convergence.
[18] A. M. Hemeida, O. M. Bakry, A. A. A. Mohamed, and E. A. Mahmoud, “Genetic algorithms and satin bowerbird optimization for optimal allocation of distributed generators in radial system,” Appl. Soft Comput., vol. 111, Nov. 2021, doi: 10.1016/j.asoc.2021.107727. [19] A. El Beqal, B. Benhala, and I. Zorkani, “A genetic algorithm for the optimal design of a multistage amplifier,” Int. J. Electr. Comput. Eng., vol. 10, no. 1, pp. 129 138, Feb. 2020, doi: 10.11591/ijece.v10i1.pp129 138. [20] W. F. Mahmudy, M. Z. Sarwani, A. Rahmi, and A. W. Widodo, “Optimization of multi stage distribution process using improved genetic algorithm,” Int. J. Intell. Eng. Syst., vol. 14, no. 2, pp. 211 219, 2021 [21] K. Kamil, K. H. Chong, H. Hashim, and S. A. Shaaya, “A multiple mitosis genetic algorithm,” IAES Int. J. Artif. Intell., vol. 8, no. 3, pp. 252 258, Dec. 2019, doi: 10.11591/ijai.v8.i3.pp252 258. [22] S. Malik and S. Wadhwa, “Preventing premature convergence in genetic algorithm using DGCA and elitist technique,” Int. J. Adv. Res. Comput. Sci. Softw. Eng., vol. 4, no. 6, pp. 410 418, 2014
[7] C. Changdar, G. S. Mahapatra, and R. K. Pal, “An improved genetic algorithm based approach to solve constrained knapsack problem in fuzzy environment,” Expert Syst. Appl., vol. 42, no. 4, pp. 2276 2286, Mar. 2015, doi: 10.1016/j.eswa.2014.09.006.
ISSN: 2252 8938 Int J Artif Intell, Vol. 11, No. 3, September 2022: 826 835 834
[9] Q. Zhai, Y. He, G. Wang, and X. Hao, “A general approach to solving hardware and software partitioning problem based on evolutionary algorithms,” Adv. Eng. Softw., vol. 159, Sep. 2021, doi: 10.1016/j.advengsoft.2021.102998.
[1] L. Shen et al., “Inventory optimization of fresh agricultural products supply chain based on agricultural superdocking,” J. Adv. Transp., pp. 1 13, Jan. 2020, doi: 10.1155/2020/2724164.
[2] F. D. Croce, F. Salassa, and R. Scatamacchia, “An exact approach for the 0 1 knapsack problem with setups,” Comput. Oper. Res., vol. 80, pp. 61 67, Apr. 2017, doi: 10.1016/j.cor.2016.11.015.
[5] I. M. Ali, D. Essam, and K. Kasmarik, “Novel binary differential evolution algorithm for knapsack problems,” Inf. Sci. (Ny)., vol. 542, pp. 177 194, Jan. 2021, doi: 10.1016/j.ins.2020.07.013.
[6] N. Thongsri, P. Warintarawej, S. Chotkaew, and W. Saetang, “Implementation of a personalized food recommendation system based on collaborative filtering and knapsack method,” Int. J. Electr. Comput. Eng., vol. 12, no. 1, pp. 630 638, Feb. 2022, doi: 10.11591/ijece.v12i1.pp630 638.
[10] X. Yang, Y. Zhou, A. Shen, J. Lin, and Y. Zhong, “A hybrid ant colony optimization algorithm for the knapsack problem with a single continuous variable,” in Proceedings of the Genetic and Evolutionary Computation Conference, Jun. 2021, pp. 57 65., doi: 10.1145/3449639.3459343.
[4] Y. He and X. Wang, “Group theory based optimization algorithm for solving knapsack problems,” Knowledge Based Syst., vol. 219, May 2021, doi: 10.1016/j.knosys.2018.07.045.
[11] O. Kabadurmus, M. F. Tasgetiren, H. Oztop, and M. S. Erdogan, “Solving 0 1 Bi objective multi dimensional knapsack problems using binary genetic algorithm,” in Studies in Computational Intelligence, vol. 906, 2021, pp. 51 67., doi: 10.1007/978 3 030 58930 1_4. [12] A. Syarif, D. Anggraini, K. Muludi, W. Wamiliana, and M. Gen, “Comparing various genetic algorithm approaches for multiple choice multi dimensional knapsack problem (mm KP),” Int. J. Intell. Eng. Syst., vol. 13, no. 5, pp. 455 462, Oct. 2020, doi: 10.22266/ijies2020.1031.40.
[3] Y. Feng and G. G. Wang, “A binary moth search algorithm based on self learning for multidimensional knapsack problems,” Futur. Gener. Comput. Syst., vol. 126, pp. 48 64, Jan. 2022, doi: 10.1016/j.future.2021.07.033.
[8] Y. Zhou, M. Zhao, M. Fan, Y. Wang, and J. Wang, “An efficient local search for large scale set union knapsack problem,” Data Technol. Appl., vol. 55, no. 2, pp. 233 250, Apr. 2021, doi: 10.1108/DTA 05 2020 0120.
The computational experiment proves that GA, SPGA and EDSPGA could effectively solve the problem the knapsack problem. However, based on the same parameters with SPGA, GA only reaches 17.8% of SPGA fitness value. On the other hand, although EDSPGA requires a longer computational time, the result of EDSPGA is increased by 2.56% from SPGA fitness value. The next research as future work can be considered as i) discovering SPGA and EDSPGA performance by not only test popsize and subpop but also the generation and adaptive changing of crossover rate and mutation rate and ii) exploring the complexity of the problem and applying the method to solve more complex problems.
[25] A. P. Rifai, P. A. Kusumastuti, S. T. W. Mara, R. Norcahyo, and S. Z. Md Dawal, “Multi operator hybrid genetic algorithm simulated annealing for reentrant permutation flow shop scheduling,” ASEAN Eng. J., vol. 11, no. 3, pp. 109 126, Apr. 2021, doi: 10.11113/aej.v11.16875.
[31] A. Marrero, E. Segredo, and C. Leon, “A parallel genetic algorithm to speed up the resolution of the algorithm selection problem,” in Proceedings of the Genetic and Evolutionary Computation Conference Companion, Jul. 2021, pp. 1978 1981., doi: 10.1145/3449726.3463160.
[32] W. N. Abdullah and S. A. Alagha, “A parallel adaptive genetic algorithm for job shop scheduling problem,” J. Phys. Conf. Ser., vol. 1879, no. 2, May 2021, doi: 10.1088/1742 6596/1879/2/022078.
[29] M. L. Seisarrina, I. Cholissodin, and H. Nurwarsito, “Invigilator examination scheduling using partial random injection and adaptive time variant genetic algorithm,” J. Inf. Technol. Comput. Sci., vol. 3, no. 2, pp. 113 119, Nov. 2018, doi: 10.25126/jitecs.20183250.
[28] A. A. K. Taher and S. M. Kadhim, “Improvement of genetic algorithm using artificial bee colony,” Bull. Electr. Eng. Informatics, vol. 9, no. 5, pp. 2125 2133, Oct. 2020, doi: 10.11591/eei.v9i5.2233.
[27] A. K. Ariyani, W. F. Mahmudy, and Y. P. Anggodo, “Hybrid genetic algorithms and simulated annealing for multi trip vehicle routing problem with time windows,” Int. J. Electr. Comput. Eng., vol. 8, no. 6, pp. 4713 4723, 2018, doi: 10.11591/ijece.v8i6.pp.4713 4723.
[30] F. Uysal, R. Sonmez, and S. K. Isleyen, “A graphical processing unit‐based parallel hybrid genetic algorithm for resource‐constrained multi‐project scheduling problem,” Concurr. Comput. Pract. Exp., vol. 33, no. 16, Aug. 2021, doi: 10.1002/cpe.6266.
[33] X. Shi, W. Long, Y. Li, and D. Deng, “Multi population genetic algorithm with ER network for solving flexible job shop scheduling problems,” PLoS One, vol. 15, no. 5, May 2020, doi: 10.1371/journal.pone.0233759.
BIOGRAPHIES OF AUTHORS Wayan Firdaus Mahmudy obtained a Bachelor of Science degree from the Mathematics Department, Brawijaya University in 1995. His Master in Informatics Engineering degree was obtained from the Sepuluh Nopember Institute of Technology, Surabaya in 1999 while a Ph.D. in Manufacturing Engineering was obtained from the University of South Australia in 2014. He is a Professor at Department of Computer Science, Brawijaya University (UB), Indonesia. His research interests include optimization of combinatorial problems and machine learning. He can be contacted at email: wayanfm@ub.ac.id.
Nindynar Rikatsih received Bachelor of Computer degree and her Master in Computer Science degree from Faculty of Computer Science, Brawijaya University (UB) in 2016 and 2020 respectively. She is currently a lecturer in Institut Technology, Science and Health of Hospital dr. Soepraoen, Malang. Her research interests include evolutionary algorithms, optimization problems, and data mining. She can be contacted at email: R.Nindynar@gmail.com Syafrial received Bachelor of Socio economic degree from Faculty of Agriculture, Brawijaya University (UB) in 1982 and received Master and Doctor degree in the field of Agricultural Economic Science, Bogor Agricultural University in 1986 and 2003 respectively. He is currently a senior lecturer of Agricultural Sosio Economic Department in Faculty of Agriculture, Universitas Brawijaya He can be contacted at email: syafrial.fp@ub.ac.id
Optimization of agricultural product storage using real coded … (Wayan Firdaus Mahmudy) 835
Int J Artif Intell ISSN: 2252 8938
[26] A. Iranmanesh and H. R. Naji, “DCHG TS: a deadline constrained and cost effective hybrid genetic algorithm for scientific workflow scheduling in cloud computing,” Cluster Comput., vol. 24, no. 2, pp. 667 681, Jun. 2021, doi: 10.1007/s10586 020 03145 8.
[23] S. Masrom, M. Mohamad, S. M. Hatim, N. Baharun, N. Omar, and A. S. Abd. Rahman, “Different mutation and crossover set of genetic programming in an automated machine learning,” IAES Int. J. Artif. Intell., vol. 9, no. 3, pp. 402 408, Sep. 2020, doi: 10.11591/ijai.v9.i3.pp402 408. [24] G. E. Yuliastuti, A. Mustika, W. Firdaus, and I. Pambudi, “Optimization of multi product aggregate production planning using hybrid simulated annealing and adaptive genetic algorithm,” Int. J. Adv. Comput. Sci. Appl., vol. 10, no. 11, pp. 484 489, 2019, doi: 10.14569/IJACSA.2019.0101167.
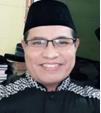
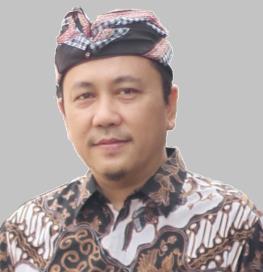



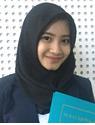






Microstrip antenna optimization using evolutionary algorithms
Kalpa Ranjan Behera, Surender Reddy Salkuti Department of Railroad and Electrical Engineering, Woosong University, Daejeon, Republic of Korea
Keywords: Artificial neural network Differential evolution E shape microstrip antenna Genetic TulipParticleMicrostripalgorithmantennaswarmoptimizationshapeantenna
Journal homepage: http://ijai.iaescore.com
Article Info ABSTRACT
IAES International Journal of Artificial Intelligence (IJ AI) Vol. 11, No. 3, September 2022, pp. 836 842 ISSN: 2252 8938, DOI: 10.11591/ijai.v11.i3.pp836 842 836
This is an open access article under the CC BY SA license. Corresponding Author: Surender Reddy Salkuti Department of Railroad and Electrical Engineering, 17 2, Woosong University Jayang dong, Dong gu, Daejeon 34606, Republic of Korea Email: surender@wsu.ac.kr NOMENCLATURE ���������� Effective permittivity of substrate ����,������������ Target cutoff frequency ���� Resonant frequency ���� Obtained bandwidth �� Speed of light ���������������� Target bandwidth �������� Effective length ���� �� Velocity of agent i iteration k ���������� Effective permittivity of the substrate �� Weight function �� Fitnessfunction �� Weight factor ��0 Desired resonant frequency �������� Random number between 0 and 1 ��0 Optimized resonant frequency ���� �� Current position of agent i and iteration k ���� Desired return loss ������������ Local best of agent i ��0 Return loss ���������� Global best ��, �� Biasing constants �� Total sampling points ���� Obtained cutoff frequency ���� Sampling frequency ��(����) Error found in one frequency 1. INTRODUCTION Wireless communication is broadly used in today’s communication technology. Antennas are an integral part of wireless communication. Microstrip antennas are popular due to their small size, lightweight, and efficiency. So it's used broadly in mobile phones, airplanes, satellites, and 5G communicatio [1] The
Article history: Received Aug 5, 2021 Revised May 22, 2022 Accepted May 31, 2022
Different structures of microstrip antenna optimization using different algorithms are the important field of wireless communications. Rectangular microstrip antenna, inverted E shaped antenna, tulip shaped antenna are some examples of microstrip antennas. The antenna dimensions are optimized by different algorithms. The operating frequencies for different antenna structures depend on antenna dimensions. The frequency of operation is 3 lS GHz for rectangular antenna, IMT 2000 for invelted E shaped antenna, 8 to 12 GHz for tulip shaped antenna, 2.16 GHz for miniaturized antenna structure. The dimensions of microstrip antennas are modified to get minimum reflection coefficient maximum gain and bandwidth. The dimensions are modified using different algorithms such as evolutionary algorithm, particle swarm optimization (PSO), artificial neural network (ANN), and genetic algorithms (GA)
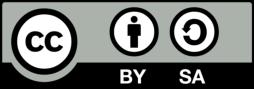
2. TYPES OF OPTIMIZATION FOR MICROSTRIP ANTENA
A rectangular microstrip antenna with an insert feed line has been chosen for optimization and it is shown in Figure 1. The length, width, and feed position are selected as antenna parameters. Return loss is a required parameter to optimize by adjusting the values of length, width, and feed position. The rectangular antenna is chosen due to its simple antenna parameters. The resonant frequency is calculated for rectangular microstrip antenna by the given formula. The effective length of the antenna is usually longer than the actual physical length. It is due to the fringing effect. Effective length is calculated by its formula. Effective
2.1. Differential evolution used in rectangular microstrip antenna
Microstrip antenna optimization using evolutionary algorithms (Kalpa Ranjan Behera) 837 antenna output such as bandwidth, return loss, the resonant frequency depends on antenna structure. So antenna dimensions are chosen carefully to get the required output. To choose the right dimension, it's required to optimize the antenna. Optimization is processed by applying different algorithms [2], [3]. The algorithms generate different solutions for different dimensions and different structures. The defective element recognition can take place using different algorithms [4] Differential evolution is an algorithm that uses mutation and recombination to generate a solution. The first population is go through mutation. So that a new solution is generated in this process. After mutation crossover is take place between the solution and the new solution generated. This process continues till the desired solution. The resonant frequency is optimized for rectangular microstrip antennas using an evolutionary algorithm for frequency band 3 to 18 GHz [5]. Particle swarm optimization (PSO) inspired by birds flocking and fish schooling. Each bird or particle represents the solution. Each particle search for a solution in its locality. The best solution found by each particle is replaced by the previous solution. Likewise, each group has the best solution which replaces by the next best solution. The solution obtained at last by the group is the best solution [6]. Bandwidth is improved for E shape microstrip antenna by optimizing antenna parameters using PSO [7]. The artificial neural network (ANN) is inspired by neurons of the human brain. Like human brains having neuron cells that process information from input to output, ANN has neurons that process information based on some weights. The ANN uses experience in the backpropagation algorithm and comes out with perfect results. Dimension optimization is another technique for modified tulip shaped microstrip antenna using an ANN in the X/Ku band [8] The genetic algorithm (GA) is inspired by Charles Darwin's theory of natural selection. natural selection is based on the fittest individual. The fitness function gives the fitness value. A crossover takes place between the pair having the best fitness value. The obtained offspring mutate some percentage and its fitness value is evaluated. The process takes place till the best solution. Using a GA for the rectangular antenna is a helpful technique to miniaturize antenna shape [9], [10]. The antenna parameters such as return loss, gain, bandwidth depend on antenna dimensions (length, width, height), substrate type, and ground plane dimension. Radiation patterns and impedance also vary with antenna dimensions and antenna type. A microstrip antenna is designed using boolean PSO and the method of moments [11] The geometry of the microstrip antenna depends on cross polarization, return loss, and bore sight directions. A circularly polarized stacked microstrip antenna has optimized feeding techniques. A c type feeding technique is used in the antenna structure [12]. The optimization technique is applied to feed along with return loss and bandwidth. A quasi planar surface planar short horn antenna is attached to the microstrip antenna for higher gain. The antenna is used in wideband circularly polarized and higher gain applications. A buried microstrip antenna optimized using GA and finite difference time domain. The antenna is optimized for maximum soil moisture sensing. The variation in performance due to soil moisture is minimized by design. The communication is performed between the buried antenna and receiver antenna [13]. A complementary particle swarm antenna is designed using the PSO technique. The PSO technique is used to place the parasitic sub patch in the proper place to get a minimum reflection coefficient. The substrate optimization technique is used to optimize antenna performance. Reciprocity theorem and integral equations are used as optimization tools in this process. Bandwidth and radiations are optimized for dipole and slot antenna. the optimized value is found out by varying substrate height and permittivity of substrate [14]. Two optimization techniques are used to optimize rectangular microstrip antenna. One is a global optimizer called central force optimization and another is local optimization called Nelder Mead optimization. the combination of global optimization followed by local optimization is called the hybrid central force optimization Nelder Mead approach. The optimization process performs better than another optimization process on given benchmark functions [15].
Int J Artif Intell ISSN: 2252 8938
In the next section, different types of microstrip antennas are described along with characteristic properties [16]. The characteristics vary with antenna dimensions, substrate types [17]. Optimized gain, bandwidth, the reflection coefficient is found out by applying optimization techniques. Rectangular, tulip, inverted E shaped antennas are taken for optimization. Optimization process use algorithms such as: i) differential evolution, ii) GA, iii) PSO, and iv) ANN in the subsections of 2nd section [18]. At the conclusion part we get, the optimized result may vary according to the algorithms applied to it.
ISSN: 2252 8938 Int J Artif Intell, Vol. 11, No. 3, September 2022: 836 842 838permittivity (����������) is a function of substrate permittivity (����). Substrate permittivity depends on the type of substrate in use. For example substrate permittivity �������� for FR4 substrate is 4.4 [5], [19]
A fitness function is a type of objective function that use to compare between design solution and desired solution. The fitness function gives a real number value that helps to improve our design. Fitness function minimization is used in the optimization process. For maximization purposes, we use negative signs and vice versa. �� =|��0 ��0|+|���� ��0| (2)
Fitness function called parameter estimation in statistics. It is used to get the perfect pair of the population of size 1×3. In (1×3) 1 is represent a number of iteration and 3 represents antenna parameters such as length, width, and feed position. A random population is generated for length, width, and feed position. In nest iteration population is updated using (3) ����,�� �� =�������� �� +��������(0,1)×(�������� �� �������� �� ) (3) �� is the randomly generated population. The population is mutated and crossover to get a new population in the differential evolution algorithm. The best fitness value is compared with the desired value and the antenna parameter is updated for the next generation population. The process continues till the desired solution is found. The solution parameters are fitted with Zeeland IE3D software and return loss is found out. The return loss is used in the fitness function to improve antenna parameters. 2.2. Inverted E shaped patch antenna
The inverted E shaped microstrip antenna fed with the probe is optimized for bandwidth maximization and it is depicted in Figure 2. The independent antenna parameters are length, width, slot length, and slot width. The aim is to maximize antenna bandwidth by varying antenna parameters and making center frequency constant at the IMT 2000 band.
Figure 1. Rectangular microstrip antenna Resonant frequency (����) is given by ���� = �� 2��������√���������� (1)
Figure 2. Inverted E shape microstrip antenna
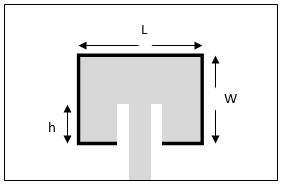
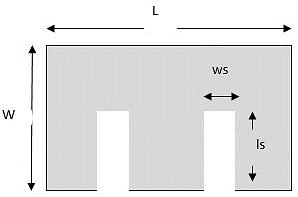
A new generation of the binary string is required to improve the result. So a new generation is formed by random single point crossover. The offspring obtained from crossover is mutated. A maximum of 5 bits are mutated in this process [31], [32]. The resonant frequency and return loss are found for a new generation and cost function is evaluated. The process crossover, mutation, fitness function evaluation continues till the desired result is found. The binary numbers contained in the final generation are our desired
Microstrip antenna optimization using evolutionary algorithms (Kalpa Ranjan Behera) 839
Modified leaf microstrip antenna has many more advantages over simple microstrip antenna, such as higher radiation, multi band operating mode, wider bandwidth, and small size. Tulip microstrip antenna is the combination of many modified leaf microstrip antennas and it is depicted in Figure 3. Here we need to operate a tulip microstrip antenna in X/Ku band. X band is in the range of 8 to 12 GHz and Ku band is in the range of 12 to 18 GHz. The antenna is fed with a coaxial probe [22]. The parameters for microstrip antenna are D, R1, R2, and W2. The proposed antenna is simulated through high frequency structure simulator (HFSS) simulation software. The output return loss and resonant frequency for the X/Ku band were found out. With the help of an ANN, a better value for return loss and the resonant frequency is found out.
To get the curve fit equation, the bandwidth is required by varying one independent variable and making other independent variables constant. 54 observations have been found out by varying antenna length and making antenna width, slot length, and slot width constant. The same procedure has been applied for antenna width, slot length, and slot width. Four tables are formed by varying four independent variables separately. For each table, graphmatica forms different equations. The graphmatica is curve fitting software to generate curve fitting equations.
Figure 4 depicts the view of miniaturized microstrip antenna A metal patch is divided into 10×10 number of square patches. A string of 20 random binary numbers is chosen to get the population in the process of GA optimization [26]. According to string, the binary one is represented with metal conducting materials, and binary 0 is replaced with nonconducting material on the antenna surface. The output for resonant frequency and return loss is calculated with the help of a software computer simulation technology (CST) [27], [28]. The cost function is calculated on the result obtained from CST with the help of MATLAB [29], [30] �������� =|1 ��∑ ��(����)�� ��=1 | (6)
2.2.1. Artificial neural network used in tulip-shaped microstrip antenna
Int J Artif Intell ISSN: 2252 8938
2.2.2. Microstrip antenna miniaturization using genetic algorithm Microstrip antenna size is decreasing due to the rapid reduction in the size of communication devices. The rectangular microstrip patch is divided into ���� small uniform rectangular cells. Each cell is either conducting material or nonconducting material. The cells are arranged in such a way that it gives the required output. The resonance frequency shift is the desired output for this optimization process [25]
Backpropagation is a method used to optimize the ANN. It is a multi layer perceptron network. Four dependent variables (����������, ������������, (��11)��������, (��11)����������) are the four input layers of neurons and four independent variables (��, ��1, ��2, ��2) are four output layers of the neuron [23], [24]. Number of hidden layers taken is 12 and the number of epochs is 2000. The ANN model is trained over different structures. It is observed the ANN trained model performs better with its past experience.
The fitness function is generated using root mean square error. The fitness function for our antenna is given below. M and N are biasing constants to control overall fitness. The PSO is optimized using MATLAB code. ��(��)=√��(���� ����,������������)2 +��(���� ����������������)2 (4) A number of random populations generated in the search space are called agents particles. Each particle represents a point in the search space. Each particle modifies its position by using information about current position, velocity, local best, and global best. The best coordinate fitness found by each particle is called local best. The best coordinate found by the group of particles is called global best. The distance between the current position and local best is called pbest and the distance between the current position and global best is called gbest [20], [21] The solution found after the termination of the process is the optimized solution. The solution found by PSO method is better than the conventional method. ���� ��+1 =������ �� +��1��������1(������������ ���� ��)+��2��������2(���������� ���� ��) (5)
ISSN: 2252 8938 Int J Artif Intell, Vol. 11, No. 3, September 2022: 836 842 840output.
The binary numbers are applied to the antenna structure to generate an optimized solution. The frequency shifts from 4.9 to 2.16 GHz with the miniaturization shape of a rectangular microstrip antenna [33]. The optimized antenna could be dual polarized and broadband. Different optimization process gives different optimized solutions that may be desired or not
Figure 4. Miniaturized microstrip antenna
The optimized value of microstrip antennas is efficient irrespective of structures. The return loss obtained in this optimization process is always better than the non optimize one. The optimized value of different algorithms is comparable. The result may vary and could be better for any other optimization algorithms. Optimization never limits the shape of antenna dimensions. Optimization modifies its dimension to get better results. The application of differential evolution, PSO, GA, and ANN to microstrip antenna is discussed in the section. Different microstrip antennas such as rectangular microstrip antenna, tulip antenna,
Figure 3. Tulip shaped microstrip antenna
3. CONCLUSION
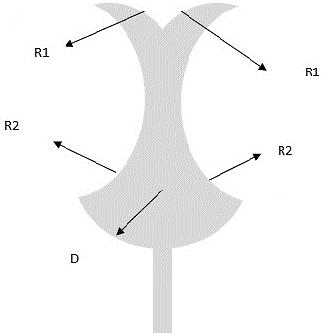
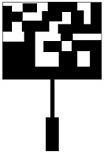
Int J Artif Intell ISSN: 2252 8938 Microstrip antenna optimization using evolutionary algorithms (Kalpa Ranjan Behera) 841 inverted E antenna are optimized using these algorithms. The area for future research includes the application of machine learning algorithms to the antenna design process. Some nature inspired optimization algorithms could be applied to the antennas to get optimized antenna characteristics. The algorithms could be applied to the different structures of microstrip antenna such as slot antenna, antenna array, and parasitic antennas.
[4] S. U. Khan, M. K. A. Rahim, M. Aminu Baba, A. E. K. Khalil, and S. Ali, “Diagnosis of faulty elements in array antenna using aature inspired cuckoo search algorithm,” International Journal of Electrical and Computer Engineering (IJECE), vol. 8, no. 3, p. 1870, Jun. 2018, doi: 10.11591/ijece.v8i3.pp1870 1874.
[16] C. A. Balanis, Antenna theory: analysis and design. John wiley & sons, 2016.
[9] J. Ayasinghe, J. A. Pros, and D. Uduwawala, “Genetic algorithm optimization of a high directivity microstrip patch antenna having a rectangular profile,” Radioengineering, vol. 22, no. 3, 2013.
[17] G. Kumar and K. P. Ray, Broadband microstrip antennas. Artech, 2002.
[20] A. A. Minasian and T. S. Bird, “Particle swarm optimization of microstrip antennas for wireless communication systems,” IEEE Transactions on Antennas and Propagation, vol. 61, no. 12, pp. 6214 6217, Dec. 2013, doi: 10.1109/tap.2013.2281517.
[6] A. K. Hamid and W. Obaid, “Hexa band MIMO CPW bow tie aperture antenna using particle swarm optimization,” International Journal of Electrical and Computer Engineering (IJECE), vol. 8, no. 5, p. 3118, Oct. 2018, doi: 10.11591/ijece.v8i5.pp3118 3128.
[2] A. Zaidi, A. Baghdad, A. Ballouk, and A. Badri, “Design and optimization of a high gain multiband patch antenna for millimeter wave application,” International Journal of Electrical and Computer Engineering (IJECE), vol. 8, no. 5, pp. 2942 2950, Oct. 2018, doi: 10.11591/ijece.v8i5.pp2942 2950.
[21] M. T. Islam, N. Misran, T. C. Take, and M. Moniruzzaman, “Optimization of microstrip patch antenna using Particle swarm optimization with curve fitting,” in 2009 International Conference on Electrical Engineering and Informatics, Aug. 2009, pp. 711 714, doi: 10.1109/iceei.2009.5254724.
[22] M. H. Misran, S. K. A. Rahim, M. A. M. Said, and M. A. Othman, “A systematic optimization procedure of antenna miniaturization for efficient wireless energy transfer,” International Journal of Electrical and Computer Engineering (IJECE), vol. 9, no. 4, p. 3159, Aug. 2019, doi: 10.11591/ijece.v9i4.pp3159 3166.
REFERENCES
[23] O. Ozgun, S. Mutlu, M. I. Aksun, and L. Alatan, “Design of dual frequency probe fed microstrip antennas with genetic optimization algorithm,” IEEE Transactions on Antennas and Propagation, vol. 51, no. 8, pp. 1947 1954, Aug. 2003, doi: 10.1109/tap.2003.814732.
[14] N. G. Alexopoulos, P. B. Katehi, and D. B. Rutledge, “Substrate optimization for integrated circuit antennas,” IEEE Transactions on Microwave Theory and Techniques, vol. 31, no. 7, pp. 550 557, 1983, doi: 10.1109/tmtt.1983.1131544. [15] K. R. Mahmoud, “Central force optimization: Nelder mead hybrid algorithm for rectangular microstrip antenna design,” Electromagnetics, vol. 31, no. 8, pp. 578 592, Nov. 2011, doi: 10.1080/02726343.2011.621110.
[19] S. V. R. S. Gollapudi et al., “Bacterial foraging optimization technique to calculate resonant frequency of rectangular microstrip antenna,” International Journal of RF and Microwave Computer Aided Engineering, vol. 18, no. 4, pp. 383 388, May 2008, doi: 10.1002/mmce.20296.
[3] O. O. Adedayo, M. O. Onibonoje, and O. M. Adegoke, “Optimetric analysis of 1x4 array of circular microwave patch antennas for mammographic applications using adaptive gradient descent algorithm,” International Journal of Electrical and Computer Engineering (IJECE), vol. 9, no. 6, p. 5159, Dec. 2019, doi: 10.11591/ijece.v9i6.pp5159 5164.
[5] M. Gangopadhyaya, P. Mukherjee, U. Sharma, B. Gupta, and S. Manna, “Design optimization of microstrip fed rectangular microstrip antenna using differential evolution algorithm,” in 2015 IEEE 2nd International Conference on Recent Trends in Information Systems (ReTIS), Jul. 2015, pp. 49 52, doi: 10.1109/retis.2015.7232851.
This research work was funded by “Woosong University’s Academic Research Funding 2022”
[10] J. W. Jayasinghe, J. Anguera, and D. N. Uduwawala, “A simple design of multi band microstrip patch antennas robust to fabrication tolerances for gsm, umts, lte, and bluetooth applications by using genetic algorithm optimization,” Progress In Electromagnetics Research, vol. 27, pp. 255 269, 2012, doi: 10.2528/pierm12102705.
[12] Nasimuddin, K. P. Esselle, and A. K. Verma, “Wideband high gain circularly polarized stacked microstrip antennas with an optimized c type feed and a short horn,” IEEE Transactions on Antennas and Propagation, vol. 56, no. 2, pp. 578 581, 2008, doi: 10.1109/tap.2007.915476.
[13] P. Soontornpipit, C. M. Furse, Y. C. Chung, and B. M. Lin, “Optimization of a buried microstrip antenna for simultaneous communication and sensing of soil moisture,” IEEE Transactions on Antennas and Propagation, vol. 54, no. 3, pp. 797 800, Mar. 2006, doi: 10.1109/tap.2006.869904.
[18] A. Deb, J. S. Roy, and B. Gupta, “Performance comparison of differential evolution, particle swarm optimization and genetic algorithm in the design of circularly polarized microstrip antennas,” IEEE Transactions on Antennas and Propagation, vol. 62, no. 8, pp. 3920 3928, Aug. 2014, doi: 10.1109/tap.2014.2322880.
ACKNOWLEDGEMENTS
[25] A. Singh and S. Singh, “Design and optimization of a modified sierpinski fractal antenna for broadband applications,” Applied
[11] F. Afshinmanesh, A. Marandi, and M. Shahabadi, “Design of a singlefeed dual band dual polarized printed microstrip antenna using a boolean particle swarm optimization,” IEEE Transactions on Antennas and Propagation, vol. 56, no. 7, pp. 1845 1852, Jul. 2008, doi: 10.1109/tap.2008.924684.
[7] B. K. Ang and B. K. Chung, “A wideband e shaped microstrip patch antenna for 5 6 ghz wireless communications,” Progress In Electromagnetics Research, vol. 75, pp. 397 407, 2007, doi: 10.2528/pier07061909.
[8] U. Ozkaya and L. Seyfi, “Dimension optimization of microstrip patch antenna in X/Ku band via artificial neural network,” Procedia Social and Behavioral Sciences, vol. 195, pp. 2520 2526, Jul. 2015, doi: 10.1016/j.sbspro.2015.06.434.
[1] H. M. Marhoon and N. Qasem, “Simulation and optimization of tuneable microstrip patch antenna for fifth generation applications based on graphene,” International Journal of Electrical and Computer Engineering (IJECE), vol. 10, no. 5, p. 5546, Oct. 2020, doi: 10.11591/ijece.v10i5.pp5546 5558.
[24] M. Lamsalli, A. El Hamichi, M. Boussouis, N. A. Touhami, and T. Elhamadi, “Genetic algorithm optimization for microstrip patch antenna miniaturization,” Progress In Electromagnetics Research Letters, vol. 60, pp. 113 120, 2016, doi: 10.2528/pierl16041907.
[29] A. Zaidi, A. Baghdad, A. Ballouk, and A. Badri, “Design and optimization of an inset fed circular microstrip patch antenna using DGS structure for applications in the millimeter wave band,” in 2016 International Conference on Wireless Networks and Mobile Communications (WINCOM), Oct. 2016, pp. 99 103, doi: 10.1109/wincom.2016.7777198.
BIOGRAPHIES OF AUTHORS Kalpa Ranjan Behera received the M.Tech degree in electronics and communication engineering from the college of engineering and technology, Bhubaneswar, India, in 2020. He got an internship from Woosong University, South Korea, in 2021. He is currently working toward software technology with a private company. His research interests include wireless communication, microstrip antenna, artificial intelligence, Internet of Things. He can be contacted at email: kalparanjanbehera@gmail.com
Surender Reddy Salkuti received the Ph.D. degree in electrical engineering from the Indian Institute of Technology, New Delhi, India, in 2013. He was a Postdoctoral Researcher with Howard University, Washington, DC, USA, from 2013 to 2014. He is currently an Associate Professor with the Department of Railroad and Electrical Engineering, Woosong University, Daejeon, South Korea. His current research interests include power system restructuring issues, ancillary service pricing, real and reactive power pricing, congestion management, and market clearing, including renewable energy sources, demand response, smart grid development with integration of wind and solar photovoltaic energy sources, artificial intelligence applications in power systems, and power system analysis and optimization. He can be contacted at email: surender@wsu.ac.kr
[27] R. C. Mahajan, V. Vyas, and M. S. Sutaone, “Performance prediction of electromagnetic band gap structure for microstrip antenna using fdtd pbc unit cell analysis and taguchi’s multi objective optimization method,” Microelectronic Engineering, vol. 219, p. 111156, Jan. 2020, doi: 10.1016/j.mee.2019.111156.
[31] R. J. Kavitha and H. S. Aravind, “An analytical approach for design of microstrip patch (MsP),” International Journal of Electrical and Computer Engineering (IJECE), vol. 8, no. 6, p. 4175, Dec. 2018, doi: 10.11591/ijece.v8i6.pp4175 4183.
ISSN: 2252 8938 Int J Artif Intell, Vol. 11, No. 3, September 2022: 836 842 842 Soft Computing, vol. 38, pp. 843 850, Jan. 2016, doi: 10.1016/j.asoc.2015.10.013
[33] P. Palai, D. P. Mishra, and S. R. Salkuti, “Biogeography in optimization algorithms: a closer look,” IAES International Journal of Artificial Intelligence (IJ AI), vol. 10, no. 4, pp. 982 989, Dec. 2021, doi: 10.11591/ijai.v10.i4.pp982 989.
[28] F. M. Monavar and N. Komjani, “Bandwidth enhancement of microstrip patch antenna using jerusalem cross shaped frequency selective surfaces by invasive weed optimization approach,” Progress In Electromagnetics Research, vol. 121, pp. 103 120, 2011, doi: 10.2528/pier11051305.
[32] D. P. Mishra, K. K. Rout, and S. R. Salkuti, “Compact MIMO antenna using dual band for fifth generation mobile communication system,” Indonesian Journal of Electrical Engineering and Computer Science, vol. 24, no. 2, pp. 921 929, Nov. 2021, doi: 10.11591/ijeecs.v24.i2.pp921 929.
[26] A. A. Al Azza, A. A. Al Jodah, and F. J. Harackiewicz, “Spider monkey optimization: A novel technique for antenna optimization,” IEEE Antennas and Wireless Propagation Letters, vol. 15, pp. 1016 1019, 2015, doi: 10.1109/lawp.2015.2490103.
[30] E. Hassan, E. Wadbro, and M. Berggren, “Topology optimization of metallic antennas,” IEEE Transactions on Antennas and Propagation, vol. 62, no. 5, pp. 2488 2500, May 2014, doi: 10.1109/tap.2014.2309112.
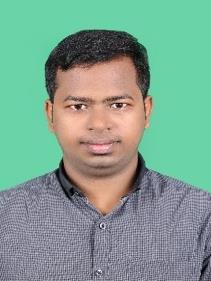



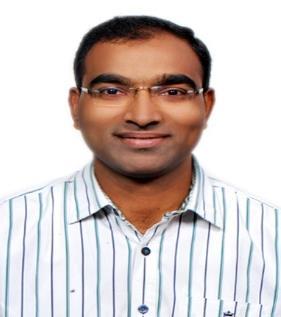



Mohammad Shahid1, Mohd Shamim1, Zubair Ashraf2, Mohd Shamim Ansari1 1Department of Commerce, Aligarh Muslim University, Aligarh, India 2Department of Computer Science, Aligarh Muslim University, Aligarh, India
1. INTRODUCTION
A novel evolutionary optimization algorithm based solution approach for portfolio selection problem
IAES International Journal of Artificial Intelligence (IJ AI) Vol. 11, No. 3, September 2022, pp. 843 850 ISSN: 2252 8938, DOI: 10.11591/ijai.v11.i3 pp843 850 843
Portfolio optimization Portfolio selection Sharpe Stochasticratiofractal search This is an open access article under the CC BY SA license.
Portfolio optimization is concerned with making a balance between risk and return of the selected securities in the security market. Thus, the different composition of securities in the portfolio will give varied results. Due care must be taken in selecting right combination of securities to make the optimal portfolio. The very basic principle of investment is diversification where investors have to commit their fund into different securities/assets based on their respective return and risk. The diversification of the portfolio is not as simple as people think by committing funds into different classes of assets. Systematic diversification requires a number of inputs for different securities like their expected return, standard deviation of the return, variance and covariance of the return, coefficient of correlation between the returns of different assets. The diversification will lead to optimize risk and return [1], [2] In the field of portfolio, the selection of best combination of securities out of the available in the security market has always been a tedious job for the experts of the field. Henry Markowitz has developed a model for portfolio optimization by emphasizing on selection of best securities in the portfolio. He emphasized on the computation of risk and the return of different securities and based on that optimal number of securities was selected to make the portfolio to its optimum level. In the real world, sometimes
Article history: Received Oct 11, 2021 Revised Apr 9, 2022 Accepted May 8, 2022
Journal homepage: http://ijai.iaescore.com
Keywords: Evolutionary algorithm
Corresponding Author: Mohd. Shamim Ansari Department of Commerce, Aligarh Muslim University Aligarh, India Email: drshamimansari@gmail.com
Article Info ABSTRACT
The portfolio selection problem is one of the most common problems which drawn the attention of experts of the field in recent decades. The mean variance portfolio optimization aims to minimize variance (risk) and maximize the expected return. In case of linear constraints, the problem can be solved by variants of Markowitz. But many constraints such as cardinality, and transaction cost, make the problem so vital that conventional techniques are not good enough in giving efficient solutions. Stochastic fractal search (SFS) is a strong population based meta heuristic approach that has derived from evolutionary computation (EC). In this paper, a novel portfolio selection model using SFS based optimization approach has been proposed to maximize Sharpe ratio. SFS is an evolutionary approach. This algorithm models the natural growth process using fractal theory. Performance evaluation has been conducted to determine the effectiveness of the model by making comparison with other state of art models such as genetic algorithm (GA) and simulated annealing (SA) on same objective and environment. The real datasets of the Bombay stock exchange (BSE) Sensex of Indian stock exchange have been taken in the study. Study reveals the superior performance of the SFS than GA and SA.
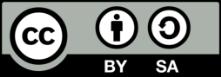
comparison has been done with state of art population based models (GA, SA) [6] from the Experimentaldomain.
Cheng et al. [9] proposed a novel approach for portfolio selection problems based GA to measures the three different risk such as semi variance, variance with skewness and mean absolute deviation. Jalota and Thakur [10] have designed to solve the portfolio selection problems by GA for handling the cardinality constraint, lower/upper bound and budget constraints. Li [11] presents a novel approach based on GA to solve the problems of investment and income for enterprises or individuals by mixing of operations research and finance. Chang and Hsu [12] proposed a particle swarm optimization (PSO) algorithm to select the top five portfolios of the stocks from the equity fund to optimize the ratio return and return rates. Jiang et al. [13] proposed PSO algorithm based on diffusion repulsion to portfolio selection problem to keep the faster convergence rate. Zhu et al. [14] proposed a novel approach using PSO for portfolio optimization problems to test the unrestricted and restricted risky portfolio investment. Reid and Malan [15] applied PSO algorithm to handle the two new constraints likes preserving feasibility and portfolio repair method. Deng and Lin [16] applied ant colony optimization (ACO) for mean variance portfolio optimization model to solve the cardinality constraints to effective low risk investment. Bacterial foraging optimization (BFO) [17] is another powerful approach for optimization problems. Niu et al. [18] proposed a BFO algorithm for portfolio selection problem to optimize liquidity risk by introducing the endogenous and exogenous liquidity risk. Kalayci et al. [19] proposed an effective solution approach based on an artificial bee colony (ABC) algorithm with infeasibility toleration procedures and feasibility enforcement for solving cardinality constrained portfolio selection model with the aim to optimize the return of investment. Chen et al. [20] reported an improved version of ABC algorithm for portfolio optimization to focus on balance the trade off between return and risk. Mazumdar et el. [21] proposed a novel approach for portfolio and unsystematic risk selection problem using grey wolf optimization to minimize the risk contributor that improve the diversification ratio. Shahid et el. [22] proposed a gradient based optimizer for unconstrained portfolio selection model. In another work [23], an invasive weed optimization has been applied for risk budgeted portfolio selection model optimizing Sharpe ratio.
ISSN: 2252 8938 Int J Artif Intell, Vol. 11, No. 3, September 2022: 843 850 of different securities are not symmetrically distributed but the model considers that the returns are normally distributed [3] [5]. There have been many models suggested for the same purpose, but the model discussed by Markowitz has been the core model and inspires many others in the domain [1]. However, the Markowitz model for portfolio optimization may be suitable if number of variables is few. But in case of large number of variables or constraints, this model may not give authentic and reliable result. Due to this limitation of the model suggested by Markowitz, some other techniques have been developed to give better solution by mixing the technique with quadratic programming. But attaining the optimum portfolio will be more difficult if the additional constraints are considered like boundary constraints, cardinality constraints which are in the form of non linear mixed integer programming problems. Solving such kinds of problems are extremely difficult than original problems. The available solutions are not enough to handle such types of problems. In such cases, the swarm intelligence (SI) and evolutionary computation (EC) approaches are being used in construct optimal portfolio by predicting the global optimum. Chang et al. [6] have been reported that genetic algorithm (GA) based solution for portfolio optimization problems suitable to approximate the unconstrained competent frontier. Kyong et al. [7] and Lin and Liu [8] presented the GA to optimize for the index fund management and transaction costs, respectively.
844returns
Some hybrid approaches are also reported to combine meta heuristics with exact algorithms or other meta heuristics to deal with complicated portfolio selection problem. Maringer and Kellere [24] used a hybrid local search algorithm that combines a meta heuristic namely simulated annealing (SA) and evolutionary algorithms (EA) for cardinality constrained portfolios. Tuba and Bacanin [25] proposed a hybrid approach combination with ABC and e firefly algorithm (FA) to optimize the mean variance return, Euclidean distance and retune error. Qin et al. [26] proposed a novel hybrid algorithm based PSO and ABC for conditional value at risk of portfolio optimization problem to optimize the mean and standard deviation. In this paper, authors proposed a portfolio selection model to maximize the Sharpe ratio by using stochastic fractal search (SFS) based evolutionary optimization approach. This approach is derived from natural growth process which is mathematically modeled by fractal theory to explore the solution space with a number of constraints. The major contributions of the work are listed as: To apply SFS based evolutionary optimization approach maximizing Sharpe ratio of the portfolio Performanceconstructed.
The rest of the paper is organized as: section 2 presents the mean variance model for Sharpe ratio with constraints handling procedures. Further it describes the SFS approach with algorithmic template. Section 3 reports the experimental results and their interpretations. Section 4 draws conclusion of the paper.
analysis has been conducted by using real datasets of the Bombay stock exchange (BSE).
2. RESEARCH METHOD
2.1. Problem formulation
In investment market, a portfolio (��) is designed with K assets from asset set, �� ={��1,��2,, ����} with weight set, �� ={��1,��2, ����}. Their respective expected returns are represented by using a return set, such as, ℛ ={��1,��2,,…����}. For the scenario, total portfolio risk and return can be expressed by using (1) and (2): ��risk =√∑ ∑ ���� ∗���� ∗������(��,��)�� �� �� �� (1) ��return = ∑ ���� �� ��=1 ∗���� (2) where ���� and ���� are the weights of ai and aj, respectively. ������(��,��) is the covariance matrix generated by return values of the assets in specified duration. In portfolio design, investors target to maximize Sharpe ratio (��ℛ��) of the portfolio under consideration. Risk free return is assumed here as zero as we are taken equity based assets. Now, the Sharpe ratio of the designed portfolio is estimated and can be written as (3).
In this section, the problem statement with a mathematical model of the portfolio selection model has been presented. Proposed evolutionary algorithm based solution approach has been discussed in detail. Further, an algorithmic template has been also given for better understanding of the proposed solution approach.
��(��)={��+∑ ����(��),�� ������ ∉�� ��(��), ������ ∈�� (4) where, �� and g are the solution and the constraints value of x in the feasible space. 2.2. Stochastic fractal search (SFS) In this section, solution approach portfolio selection using SFS has been presented to make effort for optimal weights to maximize Sharpe ratio. This algorithm has been proposed by Salimi [27] which is an evolutionary population based method with two phases, namely, the diffusion and updating. It is derived from the natural growth modeling by using concepts of fractal theory. In diffusion, diffused points create new points by moving around neighboring positions, also avoids premature convergence by avoiding local optima. The diffusion phase is presented by applying any of two Gaussian walks (��i): ��1 =Gaussian(μ��best,σ)+(δ∗��best δ′ ∗����) (7) ��2 =Gaussian(μ��,σ) (8) Here, ���� is the location of i th point, best point (��best) represents the best position of iteration, and δ,δ′ ∈ [0,1]. The parameters involved in the Gaussian function (μ��best and μ��) are same as |��best| and |����|. And, the standard deviation (σ) is estimated as σ=|log(g) g ∗(���� ��best)|.
Int J Artif Intell ISSN: 2252 8938 A novel evolutionary optimization algorithm based solution approach for portfolio … (Mohammad Shahid) 845
Max(��ℛ��)=max(��return ��risk ) (3) Subject to the constraints a) ∑ ���� =1K i=1 b) ���� ≥0 c) ai ≤���� ≤bi Here, (a) represents budget constraint. Further, (b) constraint restricts the short sell. Lastly, (c) constraint imposes lower and upper bounds for assets weights. Constraints listed above from (a) to (c) are repaired by using the constraint handling procedures given as follows: Moreover, as we see here, the above constraints from (a) to (c) are linear with convex feasible region. Therefore, a generalized penalty method used for constrain handling is also presented in this section. Now, consider, the search space of the decision variables is represented by X, then the penalty function �� may be as (4).
RESULTS AND DISCUSSION
ISSN: 2252 8938 Int J Artif Intell, Vol. 11, No. 3, September 2022: 843 850 846
Next, the updating phase alters every point’s positioning as per the most suitable position of a point in the population. Two update procedures are as: ��i ′(j)=Pr(j) ε∗(����(j) Yi(j)) (9) Pi ′′ ={Pi ′ δ ̂ ∗(Pg ′ ��best)if δ′ ≤05 Pi ′ +δ ̂ ∗(Pg ′ Pr ′) if δ′ >0.5 (10)
In (9), Pr and Pi are the two randomly generated distinct points obtained from diffusion phase. And, in (10), Pi ′ is the new point obtained from (9). And, δ&δ ̂ ∈[0,1], are random numbers. Finally, the proposed SFS algorithm to get the optimal weights for considered portfolio has been given as follows: Algorithm : SFS ( ) Begin 1. Initialize N points population 2. while (i < largest generation) do // i : number of iterations 3. for each Pi do // Pi : ith Points from N points 4. Diffusion () 5. { 6. q = dmax // maximum number of diffusions 7. for j = 1 : q do 8. if (��1 is selected) 9. generate a new point by using (7) 10. else if (��2 is selected) 11. generate a different point by (8) 12. end if 13. end for 14. } 15. end for 16. Update I () 17. { 18. Rank the points as per fitness values 19. for each Pi do 20. for each j →Pi do 21. if (rand [0, 1] ≥ Pai) 22. update jth component of Pi by (9) 23. else if 24. do nothing 25. end if 26. end for 27. end for 28. } 29. Update II () 30. { 31. Rank all points from update I on fitness values 32. for each new Pi do 33. if (rand [0, 1] ≥ Pai) 34. update the point by (10) 35. end if 36. end for 37. } 38. end while End 3.
In this section, a performance evaluation has been planned to evaluate the proposed model with comparative experimental analysis. This analysis has been done on an Intel (R) Core (TM) i7 CPU 3.20 GHz processor with 16 GB of RAM using MATLAB. Some well known meta heuristics namely genetic algorithm (GA), simulated annealing (SA), are also considered for comparative analysis. In the experimental analysis, datasets (BSE 30, BSE 100, BSE 200, and BSE 500) are extracted from S&P BSE Sensex of Indian stock exchange of monthly holding period returns from 1st April 2010 to 31st March 2020. The parameter setting is more challenging issue in meta heuristic approaches. In SFS, Size of initial population (��) and Maximum number of iteration (Itermax) control the rate of convergence. Itermax can be set as per the solution optimality requirement, a larger value of Itermax makes the method able to achieve a better result.
A novel evolutionary optimization algorithm based solution approach for portfolio … (Mohammad Shahid) 847
Table 1. Control parameters for GA, SA and proposed SFS algorithms
Figure 1. Convergence curve of GA, SA, and SFS on; (a) BSE 30, (b) BSE 100, (c) BSE 200, and (d) BSE 500
Algorithms Parameters Specifications Common parameters n = 100, Itermax= 100 SFS Random walk =1, d = 25, Pai = 1/2 GA Simulated crossover probability = 0.7 Polynomial mutation probability = 0.3 SA Neighbors =5, Mutation probability = 0.5
In this work, the best combination of parameters for the algorithm is used determined for experiments. Thus, the proposed single objective model optimizing Sharpe ratio is solved with best set of parameters on all considered datasets considering other parameters fixed. The parameters for proposed and other algorithms for comparative analysis are listed in Table 1.
We have performed 20 different runs to obtain the best optimal value of weight vector maximizing the Sharpe ratio for all data sets in the formulated optimization model. And the Max (best), Min (worst) and average values of Sharpe ratio of obtained solutions by various models are reported in Table 2. The best values among the considered models are shown in bold in the tables. The results of GA, SA, SFS algorithms of various runs for Sharpe ratio of all datasets are presented in Box Plots in Figures 2(a) to (d) for better presentation and graphical self interpretation.
Int J Artif Intell ISSN: 2252 8938
Here, Figures 1(a) to (d) demonstrates a convergence comparison of GA, SA and SFS algorithms over objective fitness function. The bench mark datasets from Bombay Stock Exchange namely BSE 30, BSE, 100, BSE 200 and BSE 500 has been used for the study. For performance comparison, simulation experiments of each algorithm Viz. GA, SA, and SFS has been taken in the study. (a) (b) (c) (d)
Table 2. Sharpe ratio GA SA SFS K=30 Max 0.390334 0.396953 0.400857 Min 0.365241 0.394756 0.400597 Avg. 0.372709 0.395917 0.400829 K=100 Max 0.471465 0.439110 0.486310 Min 0.399370 0.425896 0.471068 Avg. 0.440248 0.430771 0.482106 K=200 Max 0.556202 0.477881 0.604232 Min 0.450313 0.435389 0.544238 Avg. 0.522603 0.456614 0.575661 K=500 Max 0.608987 0.378147 0.636084 Min 0.462989 0.298987 0.542441 Avg. 0.535208 0.351277 0.590286
The portfolio selection problem is one of the core problems in investment management which drawn the attention of investors in the recent decades. Due to constraints need to be managed in portfolio construction, the conventional techniques are not good enough in giving solutions. Therefore, recent optimization methods are used to find optimum solution in complex scenario. In this work, a portfolio selection model based on an evolutionary algorithm namely SFS has been proposed. The natural growth has been mathematically modeled to explore the search space for optimum solution. For performance evaluation, an experimental analysis has been conducted to determine the effectiveness of the proposed model by doing
Figure 2. Box plots of all four algorithms on (a) BSE 30, (b) BSE 100, (c) BSE 200, and (d) BSE 500 SFS algorithm performs better in account of both the convergence rate and fitness value than GA and SA as shown in Figures 1(a) to (d). Further, SFS performs remarkably good compared to others and delivers higher values of Sharpe ratio (on all measures i.e., Max., Min., and Avg.) for all considered benchmark datasets such as BSE 30, BSE, 100, BSE 200 and BSE 500. The performance order on objective parameter of remaining algorithms is GA and SA. Thus, we can argue that the proposed approach greatly contributes robust portfolio optimization with satisfied desired constraints.
ISSN: 2252 8938 Int J Artif Intell, Vol. 11, No. 3, September 2022: 843 850 848
(a) (b) (c) (d)
4. CONCLUSION
[8] C. C. Lin and Y. T. Liu, “Genetic algorithms for portfolio selection problems with minimum transaction lots,” European Journal of Operational Research, vol. 185, no. 1, pp. 393 404, Feb. 2008, doi: 10.1016/j.ejor.2006.12.024.
A novel evolutionary optimization algorithm based solution approach for portfolio … (Mohammad Shahid) 849 performance comparison with state of art models from the domain such as GA and SA. The real datasets of the S&P BSE Sensex of Indian stock exchange have been taken for performance evaluation. Study shows the superior performance of SFS on objective parameter among its peers for all data sets in the study.
[6] T. J. Chang, N. Meade, J. E. Beasley, and Y. M. Sharaiha, “Heuristics for cardinality constrained portfolio optimisation,” Computers & Operations Research, vol. 27, no. 13, pp. 1271 1302, Nov. 2000, doi: 10.1016/S0305 0548(99)00074 X.
[17] K. M. Passino, “Biomimicry of bacterial foraging for distributed optimization and control,” IEEE Control Systems, vol. 22, no. 3, pp. 52 67, Jun. 2002, doi: 10.1109/MCS.2002.1004010.
This work is supported by the major research project funded by ICSSR with sanction No. F.No. 02/47/2019 20/MJ/RP.
[5] P. Chunhachinda, K. Dandapani, S. Hamid, and A. J. Prakash, “Portfolio selection and skewness: Evidence from international stock markets,” Journal of Banking & Finance, vol. 21, no. 2, pp. 143 167, Feb. 1997, doi: 10.1016/S0378 4266(96)00032 5.
[4] M. S. Young and R. A. Graff, “Real estate is not normal: A fresh look at real estate return distributions,” The Journal of Real Estate Finance and Economics, vol. 10, no. 3, pp. 225 259, May 1995, doi: 10.1007/BF01096940.
[7] K. J. Oh, T. Y. Kim, and S. Min, “Using genetic algorithm to support portfolio optimization for index fund management,” Expert Systems with Applications, vol. 28, no. 2, pp. 371 379, Feb. 2005, doi: 10.1016/j.eswa.2004.10.014.
[10] H. Jalota and M. Thakur, “Genetic algorithm designed for solving portfolio optimization problems subjected to cardinality constraint,” International Journal of System Assurance Engineering and Management, vol. 9, no. 1, pp. 294 305, Feb. 2018, doi: 10.1007/s13198 017 0574 z.
ACKNOWLEDGEMENTS
[12] J. F. Chang and S. W. Hsu, “The construction of stock_s portfolios by using particle swarm optimization,” in Second International Conference on Innovative Computing, Informatio and Control (ICICIC 2007), Sep. 2007, pp. 390 390, doi: 10.1109/ICICIC.2007.568.
[1] H. Markowitz, “Portfolio selection,” The Journal of Finance, vol. 7, no. 1, pp. 77 91, Mar. 1952, doi: 10.1111/j.1540 6261.1952.tb01525.x. [2] H. M. Markowitz, Portfolio selection: Efficient diversification of investment. New York: John Wiley & Sons, 1959.
[9] T. J. Chang, S. C. Yang, and K. J. Chang, “Portfolio optimization problems in different risk measures using genetic algorithm,” Expert Systems with Applications, vol. 36, no. 7, pp. 10529 10537, Sep. 2009, doi: 10.1016/j.eswa.2009.02.062.
[13] Weigang Jiang, Yuanbiao Zhang, and Jianwen Xie, “A particle swarm optimization algorithm based on diffusion repulsion and application to portfolio selection,” in 2008 International Symposium on Information Science and Engineering, Dec. 2008, pp. 498 501, doi: 10.1109/ISISE.2008.248.
[14] H. Zhu, Y. Wang, K. Wang, and Y. Chen, “Particle swarm optimization (PSO) for the constrained portfolio optimization problem,” Expert Systems with Applications, vol. 38, no. 8, pp. 10161 10169, Aug. 2011, doi: 10.1016/j.eswa.2011.02.075.
[18] B. Niu, Y. Fan, H. Xiao, and B. Xue, “Bacterial foraging based approaches to portfolio optimization with liquidity risk,” Neurocomputing, vol. 98, pp. 90 100, Dec. 2012, doi: 10.1016/j.neucom.2011.05.048.
[20] A. H. L. Chen, Y. C. Liang, and C. C. Liu, “Portfolio optimization using improved artificial bee colony approach,” in 2013 IEEE Conference on Computational Intelligence for Financial Engineering & Economics (CIFEr), Apr. 2013, pp. 60 67, doi: 10.1109/CIFEr.2013.6611698. [21] K. Mazumdar, D. Zhang, and Y. Guo, “Portfolio selection and unsystematic risk optimisation using swarm intelligence,” Journal of Banking and Financial Technology, vol. 4, no. 1, pp. 1 14, Apr. 2020, doi: 10.1007/s42786 019 00013 x.
[22] M. Shahid, Z. Ashraf, M. Shamim, and M. S. Ansari, “A novel portfolio selection strategy using gradient based optimizer,” in Proceedings of International Conference on Data Science and Applications. Lecture Notes in Networks and Systems, 2022, pp. 287 297. [23] M. Shahid, M. S. Ansari, M. Shamim, and Z. Ashraf, “A risk budgeted portfolio selection strategy using invasive weed optimization,” in International Conference on Computational Intelligence, Algorithms for Intelligent Systems, 2022, pp. 363 371. [24] D. Maringer and H. Kellerer, “Optimization of cardinality constrained portfolios with a hybrid local search algorithm,” OR Spectrum, vol. 25, no. 4, pp. 481 495, Oct. 2003, doi: 10.1007/s00291 003 0139 1. [25] M. Tuba and N. Bacanin, “Artificial bee colony algorithm hybridized with firefly algorithm for cardinality constrained mean variance portfolio selection problem,” Applied Mathematics & Information Sciences, vol. 8, no. 6, pp. 2831 2844, Nov. 2014, doi: 10.12785/amis/080619.
REFERENCES
Int J Artif Intell ISSN: 2252 8938
[3] H. Grootveld and W. Hallerbach, “Variance vs downside risk: Is there really that much difference?,” European Journal of Operational Research, vol. 114, no. 2, pp. 304 319, Apr. 1999, doi: 10.1016/S0377 2217(98)00258 6.
[11] B. Li, “Research on optimal portfolio of financial investment based on genetic algorithm,” in 2019 International Conference on Economic Management and Model Engineering (ICEMME), Dec. 2019, pp. 497 500, doi: 10.1109/ICEMME49371.2019.00104.
[15] S. G. Reid and K. M. Malan, “Constraint handling methods for portfolio optimization using particle swarm optimization,” in 2015 IEEE Symposium Series on Computational Intelligence, Dec. 2015, pp. 1766 1773, doi: 10.1109/SSCI.2015.246.
[16] G. F. Deng and W. T. Lin, “Ant colony optimization for markowitz mean variance portfolio model,” in International Conference on Swarm, Evolutionary, and Memetic Computing, 2010, pp. 238 245.
[19] C. B. Kalayci, O. Ertenlice, H. Akyer, and H. Aygoren, “An artificial bee colony algorithm with feasibility enforcement and infeasibility toleration procedures for cardinality constrained portfolio optimization,” Expert Systems with Applications, vol. 85, pp. 61 75, Nov. 2017, doi: 10.1016/j.eswa.2017.05.018.
[26] Q. Qin, L. Li, and S. Cheng, “A novel hybrid algorithm for mean CVaR portfolio selection with real world constraints,” in International Conference in Swarm Intelligence, 2014, pp. 319 327. [27] H. Salimi, “Stochastic fractal search: A powerful metaheuristic algorithm,” Knowledge Based Systems, vol. 75, pp. 1 18, Feb. 2015, doi: 10.1016/j.knosys.2014.07.025.
Mohammad Shahid is an Assistant Professor in the Department of Commerce, Aligarh Muslim University, Aligarh, India. He has received his Ph.D. from School of Computer and Systems Sciences, Jawaharlal Nehru University, New Delhi, India. He earned his M.Tech. in Computer Science and Technology from the School of Computer and Systems Sciences, Jawaharlal Nehru University, New Delhi and MCA from Department of Computer Science, Aligarh Muslim University, Aligarh, India. His areas of research interest are Cloud computing, Workflow Scheduling, Computational Intelligence, Portfolio Optimization. He is member of IEEE. You can contact him at: Department of Commerce, AMU, Aligarh 202002, India. He can be contacted at email: mdshahid.cs@gmail.com
ISSN: 2252 8938 Int J Artif Intell, Vol. 11, No. 3, September 2022: 843 850 850
BIOGRAPHIES OF AUTHORS
Mohd Shamim is professor at Department of Commerce, Aligarh Muslim University, India. He earned master’s degree and Ph.D. from AMU Aligarh. He has over 21 years of experience in teaching and research in the field of corporate finance and accounting. He got published around 45 research paper in reputed of National and international Journals. He has successfully guided 11 research scholars and more than 60 dissertations of the professional courses of the department. He has delivered more than 80 invited lectures in different institutes, colleges, and universities. He is also editor and reviewer of many international journals. He can be contacted at email: shamim1234@gmail.com Zubair Ashraf received the Ph.D. degree in computer science from the Department of Computer Science at South Asian University, New Delhi, India, in 2020. He is currently an Assistant Professor in the Department of Computer Science, Aligarh Muslim University, Aligarh, India. His current research interests include cloud computing, machine learning, artificial intelligence, fuzzy sets and systems, and evolutionary algorithms. He can be contacted at email: ashrafzubair786@gmail.com Mohd. Shamim Ansari is an Associate Professor at the Department of Commerce, Aligarh Muslim University (AMU), India. He has over 19 years of experience in teaching and research in the area of banking and finance. He has to his credit over 25 research papers, one book and presented over three dozen papers in national and international conferences and seminars. He has also completed a major research project funded by ICCSR, Government of India, and is presently working on the second project as a co director. He has also successfully guided four doctoral theses. He is also an editorial board member in the reputed Journal. He can be contacted at email: drshamimansari@gmail.com.
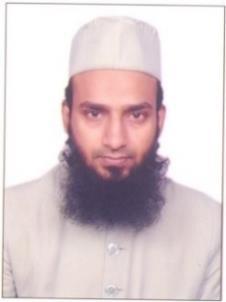




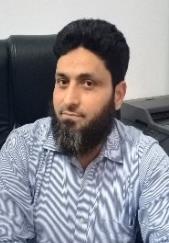




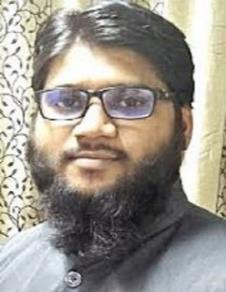




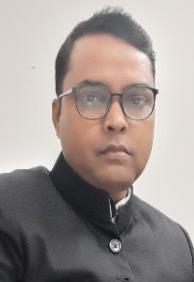




Article Info ABSTRACT
Journal homepage: http://ijai.iaescore.com Integrating singular spectrum analysis and nonlinear autoregressive neural network for stock price forecasting
This is an open access article under the CC BY SA license. Corresponding Author: Asmaa Y. DepartmentFathiofOperations Research and Decision Support, Faculty of Computers and Information, Cairo 12613UniversityOrman, Giza, Egypt Email: a.fathi@fci cu.edu.eg 1. INTRODUCTION
IAES International Journal of Artificial Intelligence (IJ AI) Vol. 11, No. 3, September 2022, pp. 851 858 ISSN: 2252 8938, DOI: 10.11591/ijai.v11.i3 pp851 858 851
Asmaa Y. Fathi1, Ihab A. El Khodary1, Muhammad Saafan2 1Department of Operations Research and Decision Support, Faculty of Computers and Information, Cairo University, Giza, Egypt 2Department of Petroleum Engineering, Universiti Teknologi PETRONAS, Seri Iskandar, Malaysia
Article history: Received Sep 11, 2021 Revised Mar 10, 2022 Accepted Apr 6, 2022
Keywords: Data preprocessing Nonlinear autoregressive neural Snetworkingular spectrum analysis Stock market Stock price prediction
A stock exchange is a legitimate organization that provides chances for investing in firms by purchasing or selling their listed shares [1]. The stock market functions similar to other economic markets, i.e., buyers aim to pay the lowest possible price for the stock, while sellers aim for higher prices. Investing in stock markets yields considerable gains, making it more appealing than low yielding assets such as government bonds. Moreover, the high liquidity present in the stock market allows the investors to transfer the assets into cash quickly [2] Nevertheless, only a few people engage in stock trading due to the challenges of forecasting stock prices, which increases investment risk. During the last decade, analytical and computational methods have advanced and given rise to several innovative new approaches to analysis financial time series based on nonlinear and nonstationary models [3]. Machine learning models have been effectively employed in various sectors, including the stock market. According to the literature, artificial neural network models (ANNs) are the main machine learning method used in forecasting various financial markets [4] [7]. ANN is a proper tool in forecasting stock prices because it does not imply any prior assumptions and can grasp nonlinear functions regarding the data properties [6]. ANNs are classified as static or dynamic networks. For instance, dynamic neural networks, e.g., the nonlinear autoregressive neural network (NARNN), assess the output utilizing a number of its
The main objective of stock market investors is to maximize their gains. As a result, stock price forecasting has not lost interest in recent decades. Nevertheless, stock prices are influenced by news, rumor, and various economic factors. Moreover, the characteristics of specific stock markets can differ significantly between countries and regions, based on size, liquidity, and regulations. Accordingly, it is difficult to predict stock prices that are volatile and noisy. This paper presents a hybrid model combining singular spectrum analysis (SSA) and nonlinear autoregressive neural network (NARNN) to forecast close prices of stocks. The model starts by applying the SSA to decompose the price series into various components. Each component is then used to train a NARNN for future price forecasting. In comparison to the autoregressive integrated moving average (ARIMA) and NARNN models, the SSA NARNN model performs better, demonstrating the effectiveness of SSA in extracting hidden information and reducing the noise of price series.
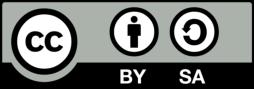
The SSA decomposes signals using singular value decomposition (SVD) and generates singular values containing information regarding the original time series [23]. The SSA approach is divided into two stages, decomposition and reconstruction, each of which consists of two steps [24]. This section outlines the SSA's stages.
2. RESEARCH METHODS
2.1.1. Decomposition
2.1. Singular spectrum analysis (SSA)
The singular spectrum analysis (SSA) has a variety of applications, including signal denoising, trend extraction, and forecasting [16]. Hassani et al. [17] employed the SSA to forecast the GBP/USD exchange rates. The authors found that the SSA model performed better than the random walk model. Fenghua et al. [18] employed the SSA for decomposing stock prices into a trend, fluctuation, and noise terms. Then, the support vector machine (SVM) is used to predict each term. Afterward, Abdollahzade et al [19] proposed a model integrating the neuro fuzzy with SSA to predict nonlinear chaotic time series. The authors concluded that the SSA improved the prediction performance due to noise reduction in the original time series. Lahmiri [20] presented a hybrid forecasting that integrates SSA and SVM optimized by particle swarm. The performance of the proposed model was evaluated with intraday stock prices, and results indicated its promise for predicting noisy time series. Later, Xiao et al. [21] forecasted the Shanghai composite index using the SSA SVM model established by [18]. The authors compared the SSA with the empirical mode decomposition and found that the former yields better forecasts. Recently, Sulandari et al. [22] integrated the SSA and ANN to forecast time series and found that the SSA ANN model outperformed the ANN model. This paper presents a hybrid model combining SSA and NARNN for stock price forecasting. First, the model divides the weekly stock closing prices into training and testing sets. Second, the SSA decomposes the training set into various components to extract hidden features and decrease the noise. Third, a NARNN is constructed and trained for each decomposed component. Forth, the model predicts the future values of various components by decomposing the preceding available prices. Finally, the SSA NARNN model aggregates the predicted values to obtain the final output. Therefore, these procedures simulate the real trading process and avoid inserting any information regarding the stock future performance in the training process. The suggested model's reliability is demonstrated using the weekly closing prices of twenty four stocks listed on the Egyptian Exchange. Additionally, the superiority of the SSA NARNN model is demonstrated by comparison to the autoregressive integrated moving average (ARIMA) and the single NARNN model.
The decomposition is performed in two steps: embedding and SVD. Embedding is the first step in which the price series is converted to a lagged trajectory matrix [24]. If ���� =[��1,��2, ,���� ]�� is a time series of length N, the mapped trajectory matrix, M, is defined as [25], [26]: �� =[��1 ��2 ���� ]=(��1 ��2 ⋯ ���� ��2 ��3 ⋯ ����+1 ⋮ ⋮ ⋱ ⋮ ���� ����+1 ⋯ ���� ) (1) where L is the embedding dimension and satisfies 2≤L≤N. M is a Hankel matrix. For instance, a trajectory matrix of a price series, St=[1.90, 1.62, 1.55, 1.60, 1.56, 1.47, 1.51, 1.48, 1.66], for L=5 is formulated as: �� = ( 1.90 1.62 1.55 1.60 1.56 1.62 1.55 1.60 1.56 1.47 1.55 1.60 1.56 1.47 1.51 1.60 1.56 1.47 1.51 1.48 156 147 151 148 166) The SVD is used after the embedding step for factorizing the trajectory matrix into biorthogonal elementary matrices [24]. This procedure is denoted by [27]:
ISSN: 2252 8938 Int J Artif Intell, Vol. 11, No. 3, September 2022: 851 858 852preceding inputs. Thus, dynamic networks possess a memory that supports recognizing time varying characteristics [8]. Unfortunately, the high noise level and interaction between hidden features in the price series decrease the ANNs prediction efficiency [9] [11]. Therefore, a data preprocessing technique, e.g., singular spectrum analysis or multicomponent amplitude and frequency modulated (AM FM) models [12] [15], is needed to improve the prediction accuracy via noise reduction and extracting underlying information hidden in the price series.
2.2. Nonlinear autoregressive neural network (NARNN)
Figure 1.
The NARNN is a feed forward dynamic network that forecasts future values of a time series by using its previous d values [29]. Hence, the NARNN utilizes the time series’ past behavior to predict its future behavior. Figure 1 illustrates the NARNN's structure utilized in our work. The network includes an input layer with a time delay line (TDL), two hidden layers, and an output layer. The TDL is configured with an eight week feedback delay, which means that the previous eight closing prices are utilized to forecast the ninth closing price. The first and second hidden layers' activation functions are tan sigmoid and log sigmoid, respectively. Additionally, the output layer has a single neuron with linear activation. The levenberg marquardt backpropagation (LMBP) algorithm is used in the network learning process. A typical NARNN structure
Int J Artif Intell ISSN: 2252 8938 Integrating singular spectrum analysis and nonlinear autoregressive neural … (Asmaa Y. Fathi) 853 �� =∑ ���� �� ��=1 =�������� =∑ ���� ���� ���� ���� ��=1 (2) where ���� is the rth elementary matrix, U and V are orthonormal system, Σ is a diagonal matrix whose diagonal elements, ����, are the singular values of ������ 2.1.2. Reconstruction The second stage of the SSA is reconstruction, which involves projecting the time series onto data adaptive eigenvectors to decrease the dataset dimensionality and express it in an optimum subspace [28]. The reconstruction stage includes two processes: grouping and diagonal averaging. Grouping is the process of categorizing elementary matrices into groups based on their eigentriples. Then, the matrices within each group are then added together [16], [24]. Let g={��1 ��2 ����} represents a group of n chosen eigentriples; then, the matrix Mg for group g is written: ��g =����1 +����2 +⋯+������ (3) Splitting of indices set, r = 1, …, R, into m subsets, g1, g2,…, gm, renders the original mapped trajectory matrix as: �� =Mg1 +��g2 +⋯+��g�� (4) The contribution of a component, Mg, in the trajectory matrix is represented by the ratio of its eigenvalues as ∑ �� ���� ∈g /∑ ���� �� ��=1 . The second step in the reconstruction process is the diagonal averaging along the N antidiagonal of the matrix Mg, also known as the Hankelization process H(Mg). This process converts the matrix into a time series that is a component of the original series st. If �������� is an element of Mg, then the kth term of the reconstructed time series is obtained through averaging the elements �������� that satisfies i+j=k+1, where 1≤k ≤N. The reconstructed time series will have a length N. The application of the diagonal averaging process to all the terms of (4) renders the decomposed components of the original series as: ���� =��(��g1)+��(��g2)+⋯+��(��g��) (5)
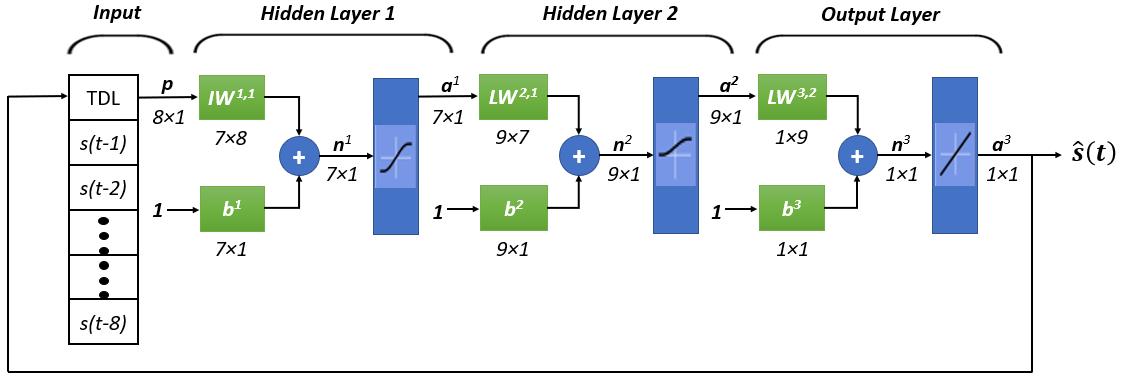
Figure 2. Sensitivity analysis of the model performance to the number of neurons in NARNN hidden layers
3. RESULTS AND DISCUSSION
ISSN: 2252 8938 Int J Artif Intell, Vol. 11, No. 3, September 2022: 851 858 854 2.3. The hybrid SSA-NARNN model
∆�� = ( ���� ∑ ���� �� ��=1 )������( ���� ∑ ���� �� ��=1 ) (6)
The covariance and standard deviation, σ, of two components ��g1 and ��g2, is utilized to calculate the correlation coefficient, ρ, as: �� = ������(��g1 ��g2) ��1 ��2 (7) components with ρ≥0.4 are considered linearly dependent and aggregated The hybrid SSA NARNN model's steps are summarized: a) Divide the stock prices into a training and testing sets, 70% and 30%, respectively.
For demonstration, the SSA decomposition process of the COMI, the heaviest constituent of the EGX 30, is detailed. The first 70% of the available 260 trading weeks are chosen for training. The training data is decomposed into 14 elementary matrices, then the increment of singular entropy is determined. As
c) Calculate ∆�� for all singular values.
d) Determine the number of orders at which ∆�� attains an asymptotic value, then group the following elementary matrices into the noise term. e) Reconstruct the different components. f) Aggregate components with ρ ≥ 0.4 g) Create and train a NARNN for each with the structure illustrated in Figure 1. h) Predict the price of each point in the testing dataset: Decompose the previous prices in the manner described in steps 2 7. Predict one step of each component. Add the predicted values to obtain the final price.
The SSA NARNN model combines the SSA and NARNN models to forecast stock prices. First, the model employs the SSA to decompose the price series into separate components, as discussed in section 2.1. Then, the increment of singular entropy, ΔE represented by (6), is utilized to separate the noise component. When ΔE reaches an asymptotic value, the information in the time series is extracted, and the remaining components represents the noise term.
b) Decompose the training set using SSA with L=14 [30]
The stock market data used in this research involves twenty four stocks listed on the EGX 30 index with five year historical data from January 2016 to December 2020. The following are the seven economic sectors of the twenty four stocks involved in our analysis: i) basic resources, ii) non bank financial services, iii) banks, iv) textile and durables, v) real estate, vi) food, beverages a d tobacco, and vii) industrial goods, services & automobiles. The proposed SSA NARNN model is utilized to forecast the weekly closing prices of the twenty four stocks involved in our analysis. First, a sensitivity analysis is carried to determine the optimum number of neurons in hidden layers of the NARNN. Figure 2 shows the average calculated mean absolute error (MAPE) of the predicted stocks versus the number of neurons in NARNN hidden layers. The lowest average MAPE is obtained using seven and nine neurons in the first and second hidden layers.
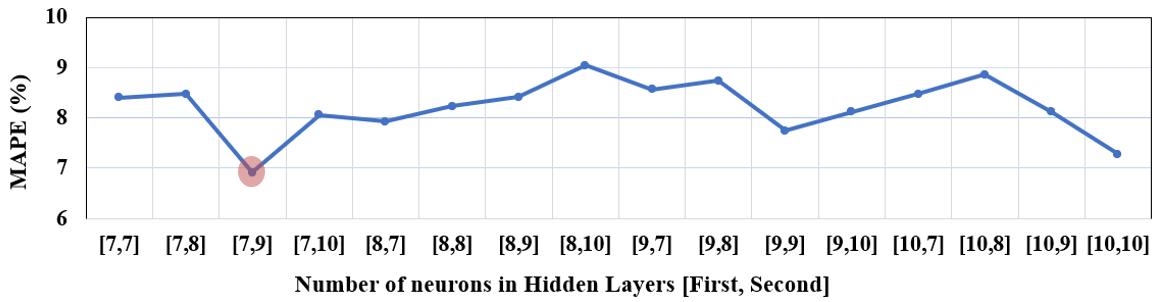
Figure 4 shows the reconstructed components, COMI's training set. RC1, RC2, and RC3 represent the market trend, fluctuation, and noise, respectively.
Integrating singular spectrum analysis and nonlinear autoregressive neural … (Asmaa Y. Fathi) 855
Int J Artif Intell ISSN: 2252 8938
Figure 4. COMI training prices and the three reconstructed components using SSA
illustrated in Figure 3, the increment of singular entropy saturates at the sixth order. Thus, the noise term is formed by combining elementary matrices from 7 to 14. The next step is to reconstruct the various matrices and calculate the correlation coefficient, as indicated in Table 1. The first and second reconstructed components have a correlation coefficient of 0.298, showing that they are separable. Likewise, components six and seven are separable. On the other hand, components two through six have higher correlation coefficients, ρ≥0.4, and are thus integrated into the one component.
Figure 3. Increment of the singular entropy Table 1. Correlation coefficients matrix of the seven reconstructed matrices Reconstructed Matrix 1 2 3 4 5 6 7 1 1 0.298 0.025 0.014 0.001 0.026 0.012 2 0.298 1 0.421 0.057 0.067 0.009 0.005 3 0.025 0.421 1 0.549 0.092 0.125 0.032 4 0.014 0.057 0.549 1 0.552 0.146 0.024 5 0.001 0.067 0.092 0.552 1 0.489 0.090 6 0.026 0.009 0.125 0.146 0.489 1 0.289 7 0.012 0.005 0.032 0.024 0.090 0.289 1
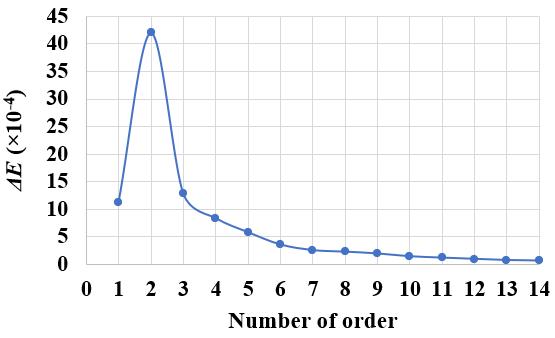
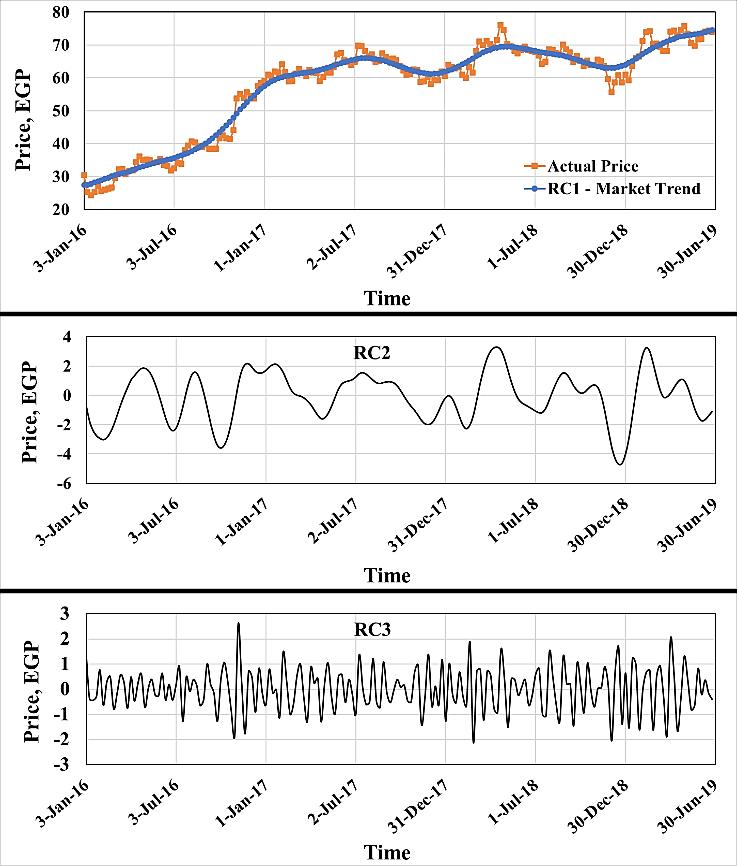
Each reconstructed component is utilized for training a NARNN. Then, the latest eight decomposed points are then supplied to the trained NARNNs in order to forecast the components of the first point in the testing dataset. To predict the components of the second point, the SSA is used to decompose all previous prices, i.e., the training set plus the first testing point. The NARNNs are then fed the most recent eight weeks' decomposed data. This procedure is repeated until all of the points in the testing set have been predicted.
Figure 5. SSA NARNN model's predicted weekly closing prices of the COMI stock Table 2. Evaluation of SSA NARNN and NARNN performance for the twenty four stocks Stock RMSE MAPE ARIMA NARNN SSA NARNN ARIMA NARNN SSA NARNN ABUK 2.45 2.95 1.51 10.2 12.3 6.8 AMOC 0.52 0.43 0.29 13.2 10.4 7.5 ESRS 0.97 1.92 0.89 8.8 19.7 8 SKPC 1.34 3.47 1.04 12.2 43.5 10.1 CCAP 0.29 0.36 0.22 12.1 15.5 8.8 EKHO 0.07 0.06 0.05 4.3 3.6 3.1 HRHO 1.26 1.21 0.94 6.8 6.5 5.2 OIH 0.08 0.13 0.06 14.1 23.1 10.5 PIOH 0.53 1.92 0.41 11.8 44.3 8.5 COMI 5.21 6.25 3.73 5.2 6.2 3.6 CIEB 2.73 4.12 2.31 6.3 11.1 4.8 EXPA 0.75 0.75 0.73 5.8 5.8 4.8 ORWE 0.41 0.52 0.33 4.8 6.8 3.4 EMFD 0.18 0.12 0.11 4.3 3.3 3.2 HELI 0.56 0.46 0.45 7.8 6 5.9 MNHD 1.12 1.09 0.73 27.6 27 18.5 OCDI 1.23 1 0.92 7.7 6.5 5.9 ORHD 0.59 0.51 0.5 12.5 9.4 9.3 PHDC 0.31 0.79 0.19 16.6 52.1 11.4 TMGH 0.63 0.52 0.44 6.9 6 5.1 EAST 0.86 0.79 0.73 5.9 4.9 4 EFID 0.73 0.68 0.62 5.6 5 4.1 AUTO 0.37 0.33 0.26 10.4 9.3 7.7 SWDY 0.81 0.74 0.66 7.3 5.9 5.7
Finally, the predicted decomposed components are then aggregated to obtain the weekly closing price using the SSA NARNN model. Figure 5 compares the predicted versus actual weekly closing prices of the COMI's testing dataset.Inorder to analyze the new SSA NARNN model's performance and prove its effectiveness, we compared it with the ARIMA and single NARNN models. Table 2 shows each model's measured evaluation criteria, with the best results bolded. The results demonstrated that the suggested SSA NARNN outperforms the ARIMA model and the single NARNN without data preprocessing. Owing to noise and nonstationary reduction in the price data, the SSA increased the system's learning and generalization abilities. From this analysis, the proposed SSA NARNN model proved its ability to predict stock prices in financial markets.
4. CONCLUSION With regards to the importance of stock market prediction and the difficulties associated with it, researchers are constantly attempting new methods to examine these markets. NARNN is a machine learning model that contains a TDL, which lessens short term volatility. Also, the SSA is among the effective data
ISSN: 2252 8938 Int J Artif Intell, Vol. 11, No. 3, September 2022: 851 858 856
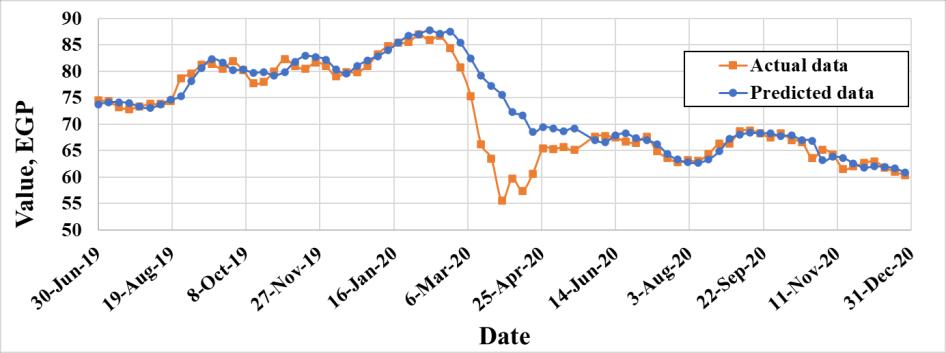
[11] L. Y. Wei, “A hybrid ANFIS model based on empirical mode decomposition for stock time series forecasting,” Applied Soft Computing, vol. 42, pp. 368 376, May 2016, doi: 10.1016/j.asoc.2016.01.027.
REFERENCES
[1] M. Göçken, M. Özçalıcı, A. Boru, and A. T. Dosdoğru, “Integrating metaheuristics and artificial neural networks for improved stock price prediction,” Expert Systems with Applications, vol. 44, pp. 320 331, Feb. 2016, doi: 10.1016/j.eswa.2015.09.029.
[2] P. Sugunsil and S. Somhom, “Short term stock prediction using SOM,” in Lecture Notes in Business Information Processing, vol. 20, 2009, pp. 262 267., doi: 10.1007/978 3 642 01112 2_27.
[7] M. R. Senapati, S. Das, and S. Mishra, “A novel model for stock price prediction using hybrid neural network,” Journal of The Institution of Engineers (India): Series B, vol. 99, no. 6, pp. 555 563, Dec. 2018, doi: 10.1007/s40031 018 0343 7. [8] A. Safari and M. Davallou, “Oil price forecasting using a hybrid model,” Energy, vol. 148, pp. 49 58, Apr. 2018, doi: 10.1016/j.energy.2018.01.007.
[18] W. Fenghua, X. Jihong, H. Zhifang, and G. Xu, “Stock price prediction based on SSA and SVM,” Procedia Computer Science, vol. 31, pp. 625 631, 2014, doi: 10.1016/j.procs.2014.05.309.
[23] A. Y. Fathi, I. A. El Khodary, and M. Saafan, “A hybrid model integrating singular spectrum analysis and backpropagation neural network for stock price forecasting,” Revue d’Intelligence Artificielle, vol. 35, no. 6, pp. 483 488, Dec. 2021, doi: 10.18280/ria.350606.
[6] X. Lin, Z. Yang, and Y. Song, “Short term stock price prediction based on echo state networks,” Expert Systems with Applications, vol. 36, no. 3, pp. 7313 7317, Apr. 2009, doi: 10.1016/j.eswa.2008.09.049.
[4] Y. Wang and H. Xing, “Time interval analysis on price prediction in stock market based on general regression neural networks,” in Communications in Computer and Information Science, 2011, vol. 144, no. 2, pp. 160 166., doi: 10.1007/978 3 642 20370 1_27. [5] J. F. Jerier, V. Richefeu, D. Imbault, and F. V. Donzé, “Packing spherical discrete elements for large scale simulations,” Computer Methods in Applied Mechanics and Engineering, vol. 199, no. 25 28, pp. 1668 1676, May 2010, doi: 10.1016/j.cma.2010.01.016.
[21] J. Xiao, X. Zhu, C. Huang, X. Yang, F. Wen, and M. Zhong, “A new approach for stock price analysis and prediction based on SSA and SVM,” International Journal of Information Technology and Decision Making, vol. 18, no. 1, pp. 35 63, Jan. 2019, doi: 10.1142/S021962201841002X.
[24] H. Hassani, “Singular spectrum analysis: methodology and comparison,” Journal of Data Science, vol. 5, no. 2, pp. 239 257, Jul. 2021, doi: 10.6339/JDS.2007.05(2).396.
Int J Artif Intell ISSN: 2252 8938
[25] C. M. Rocco S, “Singular spectrum analysis and forecasting of failure time series,” Reliability Engineering and System Safety,
[10] F. Zhou, H. Zhou, Z. Yang, and L. Yang, “EMD2FNN: a strategy combining empirical mode decomposition and factorization machine based neural network for stock market trend prediction,” Expert Systems with Applications, vol. 115, pp. 136 151, Jan. 2019, doi: 10.1016/j.eswa.2018.07.065.
[15] F. Gianfelici, G. Biagetti, P. Crippa, and C. Turchetti, “AM FM decomposition of speech signals: an asymptotically exact approach based on the iterated hilbert transform,” in IEEE/SP 13th Workshop on Statistical Signal Processing, 2005, 2005, no. 2, pp. 333 338., doi: 10.1109/SSP.2005.1628616.
[12] F. Gianfelici, C. Turchetti, and P. Crippa, “Multicomponent AM FM demodulation: the state of the art after the development of the iterated hilbert transform,” in 2007 IEEE International Conference on Signal Processing and Communications, 2007, pp. 1471 1474., doi: 10.1109/ICSPC.2007.4728608.
[16] Y. Lin, B. W. K. Ling, N. Xu, R. W. K. Lam, and C. Y. F. Ho, “Effectiveness analysis of bio electronic stimulation therapy to Parkinson’s diseases via joint singular spectrum analysis and discrete fourier transform approach,” Biomedical Signal Processing and Control, vol. 62, p. 102131, Sep. 2020, doi: 10.1016/j.bspc.2020.102131.
[22] W. Sulandari, S. Subanar, M. H. Lee, and P. C. Rodrigues, “Time series forecasting using singular spectrum analysis, fuzzy systems and neural networks,” MethodsX, vol. 7, 2020, doi: 10.1016/j.mex.2020.101015.
[17] H. Hassani, A. S. Soofi, and A. A. Zhigljavsky, “Predicting daily exchange rate with singular spectrum analysis,” Nonlinear Analysis: Real World Applications, vol. 11, no. 3, pp. 2023 2034, Jun. 2010, doi: 10.1016/j.nonrwa.2009.05.008.
[3] C. H. Cheng and L. Y. Wei, “A novel time series model based on empirical mode decomposition for forecasting TAIEX,” Economic Modelling, vol. 36, pp. 136 141, Jan. 2014, doi: 10.1016/j.econmod.2013.09.033.
[9] H. Rezaei, H. Faaljou, and G. Mansourfar, “Stock price prediction using deep learning and frequency decomposition,” Expert Systems with Applications, vol. 169, p. 114332, May 2021, doi: 10.1016/j.eswa.2020.114332.
[19] M. Abdollahzade, A. Miranian, H. Hassani, and H. Iranmanesh, “A new hybrid enhanced local linear neuro fuzzy model based on the optimized singular spectrum analysis and its application for nonlinear and chaotic time series forecasting,” Information Sciences, vol. 295, no. 65, pp. 107 125, Feb. 2015, doi: 10.1016/j.ins.2014.09.002.
Integrating singular spectrum analysis and nonlinear autoregressive neural … (Asmaa Y. Fathi) 857 preprocessing techniques that have recently been considered to reduce the noise and extract hidden information from time series. Therefore, this paper introduces a new model, i.e., the SSA NARNN model, to forecast stock prices. Using SSA, the model first decomposes the financial time series into various components. The decomposed components are then supplied into the NARNN, which fades the short term volatility using the eight preceding timesteps. The model performance is validated with twenty four stocks listed on the Egyptian Exchange. Results indicate that the SSA increased the NARNN's learning and generalization, and the SSA NARNN model outperforms the ARIMA and NARNN models. This study recommends the following for future research: i) developing a decision based trading strategy using the proposed SSA NARNN model to provide buy and sell signals and ii) integrating the price prediction model with other intelligent models to build portfolios with higher returns.
[20] S. Lahmiri, “Minute ahead stock price forecasting based on singular spectrum analysis and support vector regression,” Applied Mathematics and Computation, vol. 320, pp. 444 451, Mar. 2018, doi: 10.1016/j.amc.2017.09.049.
[14] S. C. Pei and K. W. Chang, “The mystery curve: a signal processing point of view,” IEEE Signal Processing Magazine, vol. 34, no. 6, pp. 158 163, Nov. 2017, doi: 10.1109/MSP.2017.2740457.
[13] M. Feldman, “Analytical basics of the EMD: Two harmonics decomposition,” Mechanical Systems and Signal Processing, vol. 23, no. 7, pp. 2059 2071, Oct. 2009, doi: 10.1016/j.ymssp.2009.04.002.
BIOGRAPHIES OF AUTHORS Asmaa Y. Fathi is a demonstrator at the Department of Operations Research and Decision Support, Faculty of Computers and Information, Cairo University, Giza, Egypt. She received her B.Sc. degree in Operations Research and Decision Support from Cairo University, Egypt, in 2015. She has been intensively involved in artificial intelligence research like neural networks, genetic algorithms, and hybrid intelligent systems. Recently, she has focused on applications of AI in predicting price behavior in financial markets. She can be contacted at email: a.fathi@fci cu.edu.eg Ihab A. El Khodary is a professor of Operations Research and Decision Support (Data Analysis) at the Faculty of Computers and Artificial Intelligence, Cairo University. He obtained his bachelor’s degree in Civil Engineering from Kuwait University, and his masters and Ph D degrees in Transportation Planning from the University of Waterloo, Canada. His research interests include mathematical modeling, decision support, and data analytics. His research activities have resulted in over 50 peer reviewed articles in academic journals and conference proceedings. He has supervised over 20 Ph D and M Sc theses in mathematical modeling with applications in many disciplines including finance, medicine, sports, and archeology. He has over 25 years of teaching experience in Canada and Egypt, teaching courses in mathematical modeling, forecasting, statistics, software applications in operations research, analytics, and transportation engineering at all program levels. He can be contacted at email: e.elkhodary@fci cu.edu.eg.
[27] M. C. R. Leles, J. P. H. Sansão, L. A. Mozelli, and H. N. Guimarães, “Improving reconstruction of time series based in singular spectrum analysis: a segmentation approach,” Digital Signal Processing, vol. 77, pp. 63 76, Jun. 2018, doi: 10.1016/j.dsp.2017.10.025.
[30] H. Hassani, Z. Ghodsi, E. S. Silva, and S. Heravi, “From nature to maths: Improving forecasting performance in subspace based methods using genetics colonial theory,” Digital Signal Processing, vol. 51, pp. 101 109, Apr. 2016, doi: 10.1016/j.dsp.2016.01.002.
[29] S. Lahmiri, “Wavelet low and high frequency components as features for predicting stock prices with backpropagation neural networks,” Journal of King Saud University Computer and Information Sciences, vol. 26, no. 2, pp. 218 227, Jul. 2014, doi: 10.1016/j.jksuci.2013.12.001.
ISSN: 2252 8938 Int J Artif Intell, Vol. 11, No. 3, September 2022: 851 858 858 vol. 114, no. 1, pp. 126 136, Jun. 2013, doi: 10.1016/j.ress.2013.01.007.
Muhammad Saafan is a Ph D candidate at the Department of Petroleum Engineering, Universiti Teknologi PETRONAS (UTP), Perak Darul Ridzuan, Malaysia. He holds a master's degree in petroleum engineering in the area (production history matching using intelligent algorithms). Besides his main work in petroleum engineering, he is interested in applying artificial intelligence in predicting financial markets and portfolio optimization. He can be contacted at email: muhammad.saafan@yahoo.com
[28] A. Groth and M. Ghil, “Monte carlo singular spectrum analysis (SSA) revisited: detecting oscillator clusters in multivariate datasets,” Journal of Climate, vol. 28, no. 19, pp. 7873 7893, Oct. 2015, doi: 10.1175/JCLI D 15 0100.1.
[26] M. de Carvalho and A. Rua, “Real time nowcasting the US output gap: singular spectrum analysis at work,” International Journal of Forecasting, vol. 33, no. 1, pp. 185 198, Jan. 2017, doi: 10.1016/j.ijforecast.2015.09.004.
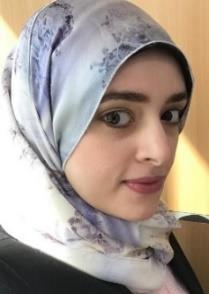



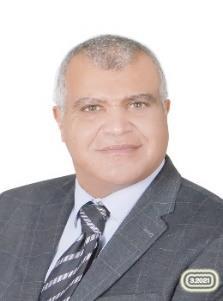



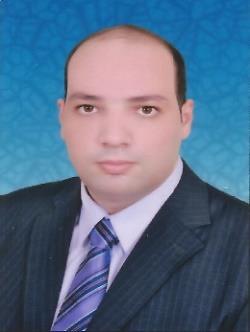



Keywords: Action learning Deep learning Deep action learning
Muslikhin1, Aris Nasuha2, Fatchul Arifin3, Suprapto2, Anggun Winursito2
2Department of Electronics Engineering, Universitas Negeri Yogyakarta, Yogyakarta, Indonesia
Article history: Received Dec 8, 2021 Revised May 22, 2022 Accepted Jun 2, 2022 Detecting troop camouflage on the battlefield is crucial to beat or decide in critical situations to survive. This paper proposed a hybrid model based on deep action learning for camouflage recognition and detection. To involve deep action learning in this proposed system, deep learning based on you only look once (YOLOv3) with SquezeeNet and the fourth steps on action learning were engaged. Following the successful formulation of the learning cycle, an instrument examinestheenvironment and performancein action learning with qualitative weightings; specific target detection experiments with view angle, target localization, and the firing point procedure were performed. For each deep action learning cycle, the complete process is divided into planning, acting, observing, and reflecting. If the results do not meet the minimal passing grade after the first cycle, the cycle will be repeated until the system succeeds in the firing point. Furthermore, this study found that deep action learning could enhance intelligence over earlier camouflage detection methods, while maintaining acceptable error rates. As a result, deep action learning could be used in armament systems if the environment is properly identified.
YOLOv3TroopSquezeeNetcamouflage
Troop camouflage detection based on deep action learning
Journal homepage: http://ijai.iaescore.com
1Department of Electronics Engineering Education, Universitas Negeri Yogyakarta, Yogyakarta, Indonesia
3Department of Electronics and Informatic Engineering Education, Universitas Negeri Yogyakarta, Yogyakarta, Indonesia
Article Info ABSTRACT
Troop camouflage in military operations is indispensable to trick and move as close as possible to the opponent, while from the opponent's side constantly trying to extract field conditions from possible enemy camouflage. Separately, the development of artificial intelligence has provided many benefits for recognition and detection purposes [1]. However, the problem of camouflage detection is considered to be difficult to overcome because distinguishing between objects and the same background requires a different strategy [2] The camouflage subdomains recognition includes segmentation, distance measurement, and troop recognition. The use of several approaches is required and even relatively new methods. Previous work by Shen et al. [3] introduced rapid camouflage detection using polarization and deep education. Unfortunately, the testing of this work uses artificial targets. It is similar with using deep learning; you only look once (YOLOv3) with an average accuracy of 91.55% [1]. Furthermore, it turns out that Xiao et al. [1] used a camouflage dataset that was not considered as a vague, for example, a fighter plane with a sky background or a frigate with an ocean background. However, the interesting point lies within how to camouflage the detected object according to the background, even though it is not necessary for the attacking troops in battle. A recent study introduced deep learning using camouflaged object detection with cascade and feedback fusion (CODCEF) [4] which can detect within 37 ms using an NVIDIA Jetson Nano device. Another
IAES International Journal of Artificial Intelligence (IJ AI) Vol. 11, No. 3, September 2022, pp. 859 871 ISSN: 2252 8938, DOI: 10.11591/ijai.v11.i3.pp859 871 859
This is an open access article under the CC BY SA license. Corresponding Author: DepartmentMuslikhin of Electronics Engineering Education, Universitas Negeri Yogyakarta 1st Colombo Street, Karangmalang Campus, Yogyakarta 55281, Indonesia Email: muslikhin@uny.ac.id 1. INTRODUCTION
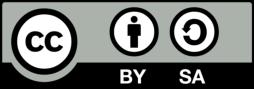
The principle of action learning imitates human learning, where the learner will try to achieve the passing grade, and if he failed to achieve it and try to repeat [23], [25], [26]. For every effort to achieve passing grade, an evaluation is carried out by the instructor, which resulted to a need of an assessment instrument in action learning [27], [28] In this light, action learning will have a repeated cycle and updates the evaluation of vision at different angles until it meets a certain level of passing grade. Besides the deep learning primary intelligence, it also learns to improve its capabilities by introducing the action learning. In practice, we will apply to troop camouflage for recognition and detection. Therefore, we expect that our system driven by deep action learning will be more accurate in detecting. Specifically, we propose to develop a rapid detection system using action learning that is robust in camouflage, which are frequently confronted while identifying troops for battlefield. This paper contains the following information: A deep action learning is employed to estimate troop camouflage, which is a pretty homogonous pattern, vary in battlefield environment, also various military uniform.
Current works by [11], [18] [20] showed the use of deep learning followed by generative adversarial networks (GAN). Although GAN has gained popularity after being combined with other techniques in the camouflage concern, there is no improvement in its intelligence because the environment is examined from each perspective separately, and the system is trained with static data, which is insufficient for upgrading the knowledge itself [21], [22]. We are trying to decipher the weakness of GAN or deep learning, which is commonly used in camouflage problems. The key idea offered in this study is the ability to upgrade the intelligence of a deep learning for satisfactory detection. The concept was adopted from the educational world where action learning has long been applied, but for the fields of artificial intelligence, image processing or robotics, it has not been widely reported in scientific publications [14], [23] [25]
We strive to be accurate in recognizing and detecting troop camouflage with deep action learning as basic Wedetection.occupied an action learning to update system knowledge independently. Self correction for troop camouflage detection in the battlefield was given and assessed; it might contribute as alternative routes to military devices. Insection2ofthisrestarticle,weintroducedourproposedsystem,andweexaminedtheresearchmethod in section 3. Results and discussion on deep action learning applied to troop camouflage detection will be presented in section 4. Finally, in section 5, we conclude up the work and provide suggestions for future projects.
2. PROPOSED SYSTEM
Figure 1 depicts our entire system; the dashed line box represents the deep learning process in which YOLOv3 is combined with SquezeeNet. The image outside the dashed line is a chart of action learning. The combination of both is called a deep action learning, while the system is without preprocessing step on targets detection. Since we donotuse preprocessing, weareworried aboutthedetectionprecision.Forthis reason, action learning in camouflage detection optimizes the self correction method; if the detection precision does not match the specified passing grade, the system will see the input image from a different alternative point of view. The red green blue (RGB) images with a resolution of 275×183 pixels ~ 640×480 pixels are used as inputs. The detectionprocessinactionlearningoccursintheactingphase, wherepreviously,theoutputof YOLOv3wasused as input in the planning phase. After the detection process is known, it will continue with the observation process; the detected target is observed again and its perspective is checked from a certain angle as a reflection discussion.
According to [9], which offers the MirrorNet, it is effective for camouflage patterns detection with an accuracy up to 87%. Basically, deep learning that has been implemented in general still has some flaws. Examples of merging deep learning with several scenarios are becoming more common and have been widely applied, such as Shi et al. [10] and Tsai et al. [11], who used reinforcement learning (RL) and deep convolutional neural network (DCNN) for mobile robots, respectively [12]. Chen et al. [13] combined recurrent neural network (RNN), deep reinforcement learning (DRL), and long short term memory (LSTM) in a comparable way; however, their capability was only around 47%. Visible weaknesses, the level of accuracy is determined during previous training. Where the number of datasets, learning rate, and epoch greatly affect performance. Another weakness is due to the nature of camouflage, which tends to have the same texture or pattern between objects against the background. Therefore, it is presumed that the current use of deep learning is no longer able to overcome the problem of camouflage detection. For this reason, other deep learning methods are needed, either as supervised or unsupervised learning [10], [14] [17]
ISSN: 2252 8938 Int J Artif Intell, Vol. 11, No. 3, September 2022: 859 871 860study [5] used data augmentation to perform camouflage detection. This method is considered adequate with 99% accuracy, superior in a lightweight but does not specify the computer specifications used. Although research [6], [7] had used many datasets, the detection results were not exceptionally good. Experiments conducted by [8] claimed that as the rapid camouflage detection using deep learning, this work still needs 0.82 seconds for a single detection process. A quite unique method is tried by Yan et al [9].
3. METHOD 3.1. Proposed approach in deep action learning Deep action learning was developed based on a combination of deep learning and action learning. YOLOv3 was selected as the method for deep learning using SqueezeNet as a feature extractor. The rectified linear unit (ReLU) functionused in fire modules, kept the original SqueezeNetactivation settings [19],[29] The fully connected (FC) layers will follow the leaky ReLU function. Leaky ReLU is a modified version of ReLU with a bit of slope in the function output for negative data. As a result, the derivative is never zero; it can limit the appearance of silent neurons, resolving the issue of ReLU failing to learn when negative intervals are encountered. As (1), the term of Leaky ReLU is explained in (1).
ϕ(x)=f(x) ={ x, x>0 01x, x<0 (1)
Figure 1. Overall architecture diagram
Int J Artif Intell ISSN: 2252 8938 Troop camouflage detection based on deep action learning (Muslikhin) 861
In action learning theory, there is no limit to when the cycle will end, and it depends on the performance of the ability to reach the passing grade. Therefore, in our proposed deep action learning may depend on the hardware specification that our proposed system is running on. The system built for deep action learning is ultimately run on a central processing unit (CPU) with a Core i5 6200 CPU@ 2.4 GHz (4 CPUs) processor speed and random access memory (RAM) of 16 GB capacity with onboard high definition (HD) Graphics 520, 4 GB of memory. Meanwhile, the deep action learning algorithm was developed using MATLAB.The deep action learning offered in this study was purely intended for detecting troops camouflage on the battlefield without the help of preprocessing or digital image processing intervention. In other words, the output of this system is the actual result of detection with a confidence value, then compared with various optimizers. There are three optimizers used in this study, stochastic gradient descent with momentum (SGDM), root mean square propagation (RMSProp), and adaptive moment optimization (ADAM). The findings were presented as a confidence level with a bounding box. The target will be determined using the square shape of the bounding box as a reference. Hence, detection utilizing deep action learning is a hybrid method, so it can be easy to describe separately in section 3.
The categorical cross entropy loss function in (2) will be used to tune our design during training. While training, we would utilize the categorical cross entropy loss function in (2) to improve our model: loss= ∑ y ̂ i1 logyi1 +y ̂ i2 logyi2 + +y ̂ im logyim n i=1 (2) where the numbers n and m denote the number of samples and categories, respectively. The real value is represented by y, whereas the estimated value is represented by y ̂ .
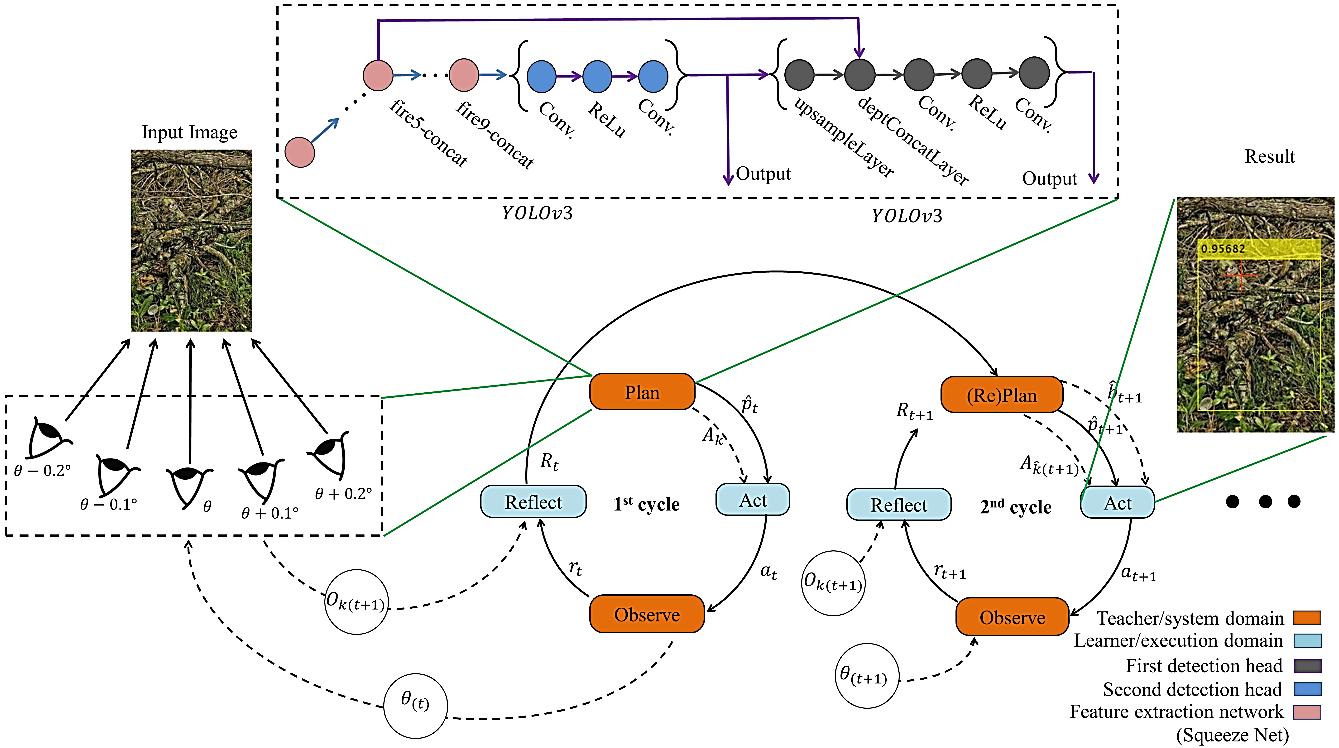
If ���� =1, the cycle will come to a halt; if ���� =0, it will scroll to the next cycle to evaluate ��(t+1) and return its value. When observation ���� receives inputs from value β∧δ in (8), deep action learning works a second time to assess if the camouflaged target has been detected or not, and the cycle continues.
���� ≠0⟹ ���� (7) We can write (7) from (8), and the reflection value result is determined by ���� with binary properties, {���� =��∧�� ⟹����; ��,�� ≠0 ���� =����, ���� ∈{0,1} (8)
In practice, it is crucial to pay more attention to the categories with small samples when having the loss function, since it aims to resolve the problem of sample imbalance. We add loss components to the loss function, as shown in (3), to let the model training proceed smoothly and avoid overfitting. loss= ∑ λ1y ̂ i1 logyi1 +λ2y ̂ i2 logyi2 + +λmy ̂ im logyim n i=1 (3) For each target category, the values of loss factor �� have been computed as presented in (4). ���� = ���� ������ (4) where the total number of samples is represented by ����. The number of target categories is n, and ���� denotes the sample amount of class I Although action learning was presented, RL was a source of inspiration. RL used the Bellman equation to calculate adiscounted valuefromthegoal point; the multiplepaths were trainedto achieve supreme value. The value of each state in RL was defined in advance. On the other hand, with action learning, the value was eliminated and substituted with a real time assessment based on the instrument, also known as a passing grade for students (system). At the same time, the passing grade is expressed by ����. The assessment of the environmental value is derived from the eight evaluation indicators in Table 1. Table 1 Assessment of the environment on deep action learning Aspect (Indicators) Scale/Probability (n) ω0 1 2 3 Planning and action Measure the YOLOv3 detection on an image �� (%) >50 ≤80 81~95 ≥96 12 Assess the current passing grade �� ≤75 76~90 ≥91 3 Adjust view an input image �� 9 Suspect the appearance of bounding box �� No Yes 3 Observing and reflecting Confirm on previous �� camouflage detection No Yes 8 Ensure firing point is visible No Yes 5 Compare the ���� versus �� ≤���� �� =���� ≥���� 5 Compare the result of �� versus ��0 (%) ≤��0 �� =��0 ≥��0 5
ISSN: 2252 8938 Int J Artif Intell, Vol. 11, No. 3, September 2022: 859 871 862
To verify self correction algorithm using deep action learning, the targeted images were performed with the following details. Where the output of YOLOv3 detection is �� and �� is the result of bounding box, and ��, �� ∈ ℝ. In addition to using scales or probabilities, we used weighting to ascertain the influence composition in deep action learning. The weights were intuitive in their settings, with a maximum total score of 50 The assessment indicators result in Table 1 can be represented as (5). β= ∑ niωi 8 i=1 + ni+1ωi+1 +⋯+ni+6ωi+6 (5) As a function, the first cycle's plan can be stated as (6), where the planning is denoted by ����, acting by ����, observing by ����, and reflecting by ����. ���� =��∧��∧�� ⟹���� (6) If the ���� has satisfied the states by ��,��,��, it will proceed to the ���� procedure, which will include conditions such as (7).
Int J Artif Intell ISSN: 2252 8938
The detailed deep action learning for troop camouflage is limited to detecting for the trained classes of 1249, and the cycle number episodes in deep action learning cannot be predicted. In this paper, the number of cycles is natural (unlimited) and depends on deep action learning performance. We fulfilled this because it does not involve hardware such as rifles, cannons, or other battle equipment, but there will be a limit on the cycles number if later apply hardware. Additional explanations are discussed in section 3.
4. RESULTS AND DISCUSSION
4.1.1.ResultsCamouflage targets detection
The testing was conducted separately, and Figure 2 shows the results of camouflage detection with the SGDM optimizer in various viewing angles. Figure 2(a) rotating target 0.2° can detect three targets with confidencevalues of 0.804, 0.785, and0.807,respectively. The next confidencevalue whenthe target is rotated 0.1° experiences a significant increase as presented in Figure 2(b); for example, from 0.804 as shown in Figure 2(c), it increases to 0.899. However, as shown in Figure 2(d), there is a downward trend and increases again when rotated +0.2°, as can be seen in Figure 2(e). Through a series of experiments, the optimal rotation limit was 1°<θ <1°, over this limit, the recall was not quite perfect, as shown in Figure 2(f). (a) (b) (c) (d) (e) (f)
Figure 2. Camouflage detection results (a) rotated image �� 02°, (b) rotated image �� 01°, (c) original image �� 0°, (d) rotated image ��+01°, (e) rotated image ��+02°, and (f) rotated image ��+15°
As seen in the value of >��±1°, the detection results did not show a significant improvement. However, itdoes notmeanthatthe value of(��+��)≈0° was the best. Thisassumption needed to beconfirmed with various approaches, as manifested in Figure 2(c), the second bounding box toward Figure 2(d). Let us observe the differences in inconsistent perspectives; for instance, for a certain angle, the value of �� >(��+��), and the result is not always better. It means that through this stage, a decision maker needs another method to adjust image rotation to get an optimal result, and action learning will work for this purpose. Another argument is that the optimizer of a detector has a significant role as well; and it needs to be disclosed. Figure 3 shows a comparison of the detection results of the three optimizers; SGDM, RMSProp, and ADAM are shown in red, green, and blue, respectively. Prior to that phase, the three optimizers were trained to generate each detector with SquezeeNet. Parameter details during training using initial learning rate 0.0001, mini batch size of 16, maximum epochs of 200, and verbose frequency of 30. After being tested on one of the same image inputs, the results are presented in Figure 3. Figure 3(a) shows an original image with �� 0° detected by RMSProp optimizer, while Figure 3(b) shows rotated image �� 01° detected by the same optimizer. Figures 3(c) and 3(d) are detected by ADAM optimizer rotated image �� 0°, �� 01° with confidence values of 0.980 and 0.971, respectively. If compared between Figure 3(e) original image �� 0° detected by SGDM optimizer and Figure 3(f) rotated �� 01°, Figure 3(f) is significantly better with a confidence value of 0.960.
Troop camouflage detection based on deep action learning (Muslikhin) 863 3.2. The system limitation
Testing the detection results of troops camouflaged in the forest battlefield using a deep action learningapproachwasconducted separately.Thisseparatetestcanbeunderstoodcomprehensivelyconsidering that the process combines detection using deep learning YOLOv3 while self correction used action learning. Three results will be presented: first, evaluating the detection results with a comparison of the optimizer, then evaluating the performance of action learning, and evaluating deep action learning.
4.1.
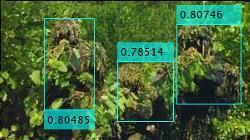
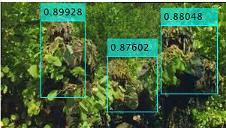
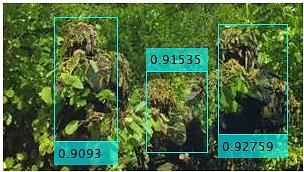
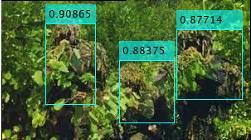
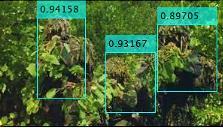
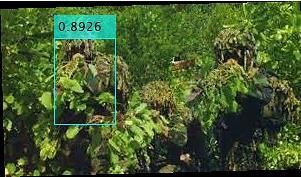
Figure 3 Troops camouflage detection results with different optimizers without rotating image. (a) original image �� 0° detected by RMSProp optimizer, (b) rotated image �� 0.1° detected by RMSProp, (c) original image �� 0° detected by ADAM optimizer, (d) rotated image �� 0.1° detected by ADAM optimizer, (e) original image �� 0° detected by SGDM optimizer, and (f) rotated image �� 01° detected by SGDM optimizer
Figure 3 shows that the ADAM optimizer has a reasonably good detection result for images that fused with the background. On the other hand, the SGDM optimizer has the lowest. The detection results presented in Figure 3 are pure detection results from a YOLOv3 detector on an image repeated three times without any interference from other methods such as rotating, zooming in, and zooming out the image. Several tests, including those in Figures 3(c) and 3(d), have a saturation detection where the test value will stagnate at a particularvalueafterseveralrepetitionsofthetestascanbeseen inTable2.Thecomparisonbetween optimizer performance is significantly different for each optimizer as we have italicized. It means that the probability of testing remains to be valid in this case, although sometimes it is not significant. Table 2 Bechmarking of optimizer’s performance Optimizers Parameters Confidence Accuracy Precission Recall F1 mAP Time (s) RMSProp 0.80 0.97 0.99 0.99 0.99 0.99 0.40 SGDM 0.86 0.92 1 0.92 0.96 0.88 0.39 ADAM 0.82 0.94 1 0.94 0.97 0.93 0.41
Now, we focus on the test results; Table 2 shows 25% of the tested samples from the dataset or about 288 of 1,153 images. The SGDM optimizer in terms of confidence value is 0.86 or superior to other optimizers. However, in terms of accuracy, precision, recall, performance, and mean average precision (mAP), RMSProp is superior while the SGDM detection time is 0.01 seconds or 0.39 seconds faster. On the other hand, the ADAM and SGDM optimizers have perfect precision of 1. 4.1.2. Deep action learning for self correction detecting It is critical to understand the principles of the existing general learning approaches to adopt deep action learning in artificial intelligence, and it must be stressed that action learning is different from other learning approaches. While there are several syntactical similarities between RL, active learning, experimental learning, andmetacognitivelearning,theprocessforinstantplanning,acting,assessing,reflecting,evaluating,orreviewing are different. Dick et al. and Altricther et al were the first to introduce action learning [26], [27], [30] in general, while Aldridge, Bell, Norton, Mc Niff, Stringer et al. Whitehead, and others modified it and called it classroom action research [28], [31] [33]. It resulted in no scholarly articles in engineering about the development of deep action learning in machine learning, and its application remains restricted to the educational field [28], [31] [33].
Deep action learning consists of deep learning and action learning; let us go into detail about action learning separately. The proposed action learning is similar with that offered by Dick et al. and Altricther et al. consisted of four cycles. The first cycle is the planning step, where the input image is observed from the perception of =0° to be detected using YOLOv3. In this status, the output of YOLOv3 is provided as input for planning. Next on the acting step, the possible value of the highest confidence level will be observed by using
ISSN: 2252 8938 Int J Artif Intell, Vol. 11, No. 3, September 2022: 859 871 864 (a) (b) (c) (d) (e) (f)
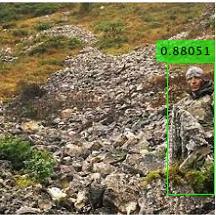
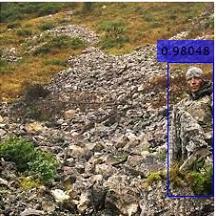
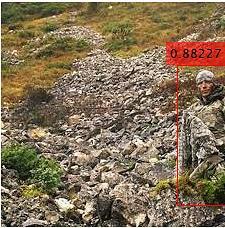
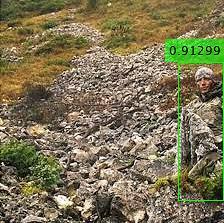
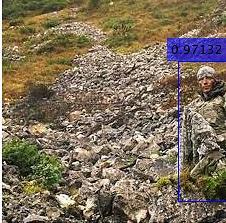
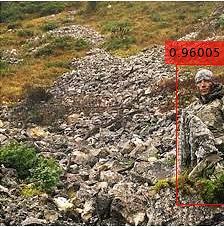
4.2. 4.2.1.DiscussionDetection
As shown in Figure 4, the results of deep action learning showed a variable increase that cannot be predicted on the way to reaching the specified passing grade = 0.9. From 0.64 to 0.91, it took seven cycles, and detection speed took 1.14 seconds. Optimizer settings and rotation angle selection were purely made by the system that had been built, which will be discussed in the discussion subsection. (a) (b) (c) (d) (e) (f) (g) (h)
Troop camouflage detection based on deep action learning (Muslikhin) 865 image playback, selecting the type of optimizer, and providing alternative shooting points from the detection results. Followed by the third step, observing, in which this section compares the interim detection results to the passing grade. The results of the comparison at the observing step are delivered to the reflecting section to make decisions. If the detection value or confidence level being compared has not reached the passing grade, the cycle is repeated until it meets the passing grade value. The overview ofthe entire series of deep action learning procedures is presented in Figure 4. We try to evaluate the same image in Figure 4(a) with an original input ��+0° After deep action learning was run for the first cycle with the detection results using the ADAM optimizer as can be seen in Figure 4(b), the result was unable to detect. Deep action learning rolled to the second cycle with the SGDM optimizer �� 1° as shown in Figure 4(c) with a confidence value of 0.6441; Figure 4(d) showed a slight increase when using the RMSProp optimizer, and the input was rotated �� 0.2° When we tried to detect in the fourth cycle of Figure 4(e) with the ADAM optimizer and the angle at �� 0.1°, the result dropped to 0.6051, whereas when the SGDM optimizer was used again with the angle at ��+0.2°, as can be seen in Figure 4(f), the confidence level increased and continued to do so as presented in Figure 4(g) using RMSProp optimizer at �� 01°. Finally, Figure 4(h) with RMSProp optimizer and angle ��+01° was saturated at 0.9148 was considered as the best at that time.
Figure 4. Sequence of camouflage detection using deep action learning (a) an original input ��+0°, result of detecting with, (b) ADAM optimizer, (c) SGDM optimizer �� 0.1°, (d) RMSProp optimizer �� 0.2°, (e) ADAM optimizer �� 0.1°, (f) SGDM optimizer ��+0.2°, (g) RMSProp optimizer �� 0.1°, (h) RMSProp optimizer ��+01° targets in camouflaged object (CAMO) dataset
Int J Artif Intell ISSN: 2252 8938
The detection results for the experiments to detect objects was similar to the background from CAMO dataset with some extended data. After being observed, significant differences were found in the detection results, for example, bright and dark images. There is a tendency for dark images to have low confidence values. Figure 5 depicts the confidence value of the histogram. Therefore, the bounding box alone is not enough, and it is necessary to add the centroid of the target for target aiming purposes. If only the results of the confidence value are pure, the detection results with deep learning are less valuable. Calculating the centroids from the bounding box, as presented in (9), is the simplest method. (9)
�������� = [ ��11 ��12 ��13 ��14 ��21 ��22 ��23 ��24 ��31 ��32 ��33 ��34 ⋮ ⋮ ⋮ ⋮ ����1 ����2 ����3 ����4]
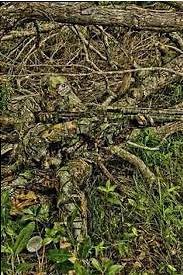
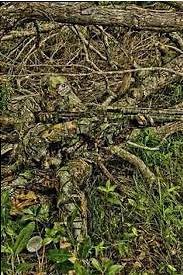
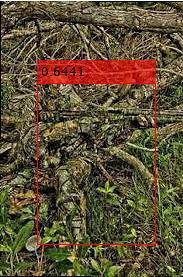
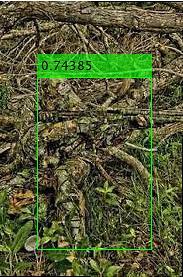
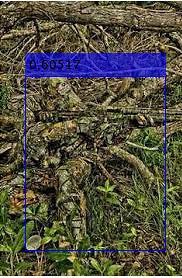
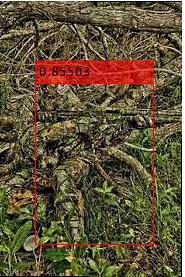
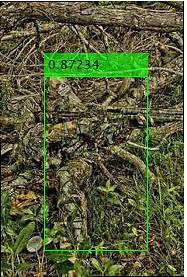
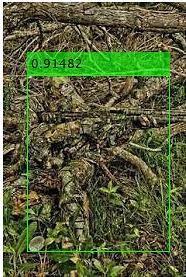
So, we could find the centroids (��������,��������) from (9) as follows {�������� = ���� + �� 2 �������� = ���� + �� 2 (10) The centroid can be determined using (10) and serves as the target's firing reference point. The centroid point in this state was still in the 2D, as for the detection results obtained using (9) and (10) can be seen in Figure 5. Figures 5(a) and 5(b) show troop camouflage with more than one target, while Figures 5(c) and 5(d) show one target camouflage only. Observed from the histogram side, the top two images represented in Figures 5(e) and 5(f) are images with dark dominance, while the second bottom two images shown in Figures 5(g) and 5(h) had a relatively normal histogram distribution. The two groups of images could show empirical evidence that the tendency for the normal curve to dominate was lower in detection accuracy even with the same optimizer.
Figure 5 Shooting targets based on ADAM optimizer; (a) (b) a dark input images, (c) (d) a balance input image with each image hitogram distribution. The dark image (e) (f) tends to have a left skewed histogram while the balanced image (g) (h) has a normal curve and the detection rate results tend to be stable
ISSN: 2252 8938 Int J Artif Intell, Vol. 11, No. 3, September 2022: 859 871 866The first line in the (9) matrix shows the first bounding box and the second line is the second bounding box. If ��11, ��12, are denoted for ����,���� while �� = ��13 and �� = ��14. The bounding box matrix �������� comprises of four columns a[1 ,4] and the number of rows depends on the number of detected targets ��[��,4] on each coordinate.
(a) (b) (c) (d) (e) (f) (g) (h)
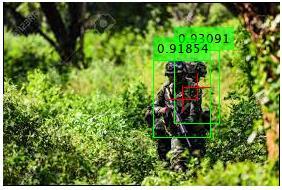
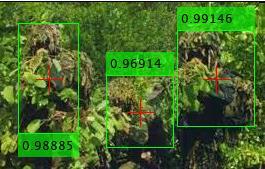
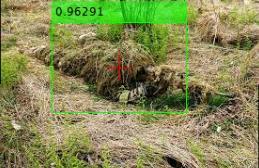
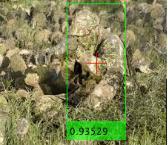
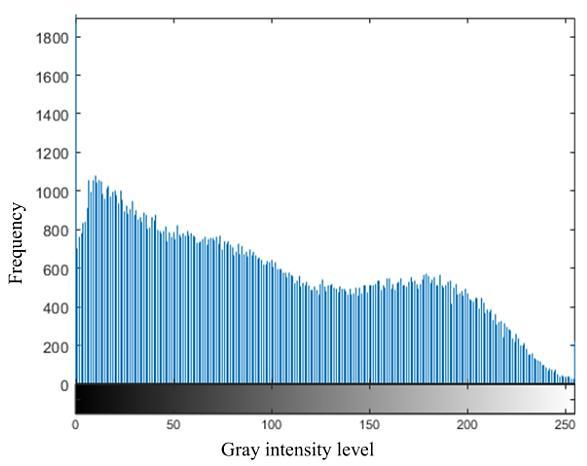
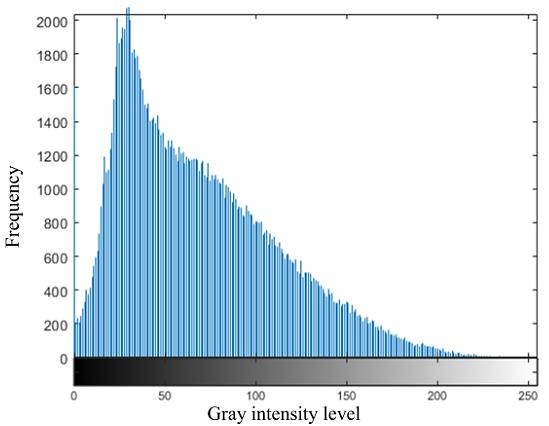
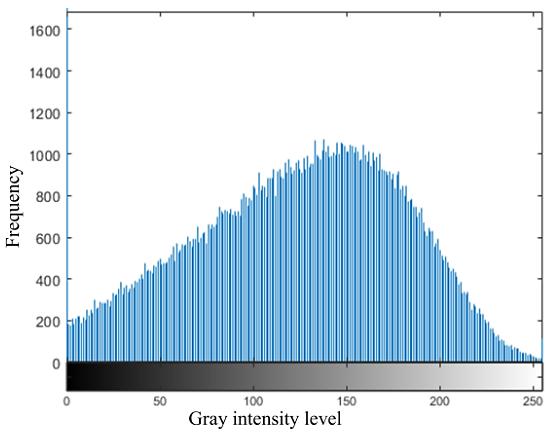
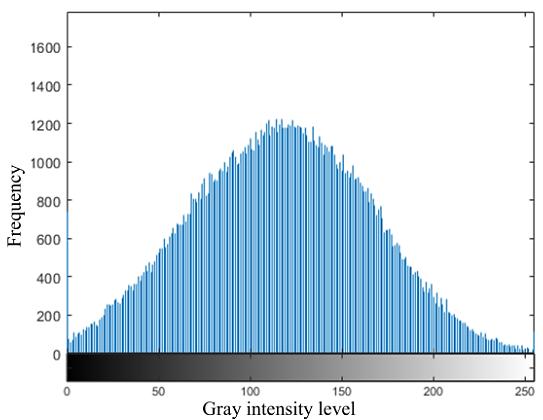
Troop camouflage detection based on deep action learning (Muslikhin) 867
Deep action learning evaluation
Before starting with the target detection technique and localization for firing target, the parameter settings for YOLOv3 as a deep learning approach should be understood. The results of deep learning were undoubtedly influenced by the differences in parameters. The starting mini batch size, learning rate, and the maximum epoch used will all hold a major impact on detection accuracy and training duration. The training results on the three optimizers (SGDM, ADAM, and RMSProp) are presented in Figure 6. If the learning rate is too low, forexample,training may takea longer process. Ifthe learningrate is too high,however, the training may provide a suboptimal or diverge output. The centroid can be determined using (10) and serves as the target's firing reference point. A detector constructed during training can be seen in general performance based on the training loss over iteration numbers. The training loss of the YOLOv3 detector is depicted in Figure 6 using the SGDM, ADAM, and RMSProp optimizers. In contrast to the other two, RMSProp was found to be the best, as seen in Figure 6. The value of training loss was virtually nil in the 500th iteration and tended to stagnate until the 600th iteration, while the ADAM and SGDM optimizers were close to each other in the 800th iteration. This detector's precision was critical for overall system testing verification. At all recall levels, the precision should ideally be one.Figure 6. RMSEs of training loss during iteration in a YOLOv3 detector; training loss for SGDM optimizer (solid line), ADAM optimizer (dashed cross line), and RMSProp optimizer (dashed line) Comparisons were also made to compare deep action learning with other methods. As presented in Table 3, the system can perform self correction to achieve a minimum passing grade ��0. The duration of passing grade achievement varies according to the cycles, and of course, the more cycles, the longer the time required. We set ��0 = 0.88 with the developed algorithm and were able to detect as seen in the second row of Table 3 quickly; the detection result was 0.97, meant that it did not require self correction using deep action learning. Meanwhile, self correction dynamics occurred in the first, third, fourth, and fifth rows with each detection result. Focusing on the first row, shows in Table 3 with �� = 0.2° was unable to detect the target in the first cycle, and in the second cycle, the target was detected at 0.79 and 0.92. Deep action learning tried to find another optimizer alternative using RMSProp, and the result was able to exceed ��0 with a result of 0.94. The fourth line was almost similar with the first line, while the last line had two cycles with the same optimizer in detecting improvements with 0.58 seconds for both cycles. In this paper, RMSProp was found as the best optimizer, as shown in Figure 6, but we only use it as a single option. The selection of detectors was completely determined by deep action learning through the assessment mechanism in Table 1. Due to this reason, we need to compare the results of camouflage detection using deep action learning with other methods such as Unet++, CPD, SINet, and MirrorNet, which at least use CAMO dataset.Obviously, the deep action learning annotation procedure for determining the firing target will be more accurate than the existing methods, as presented in Table 4. We compare SqueezeNet's performance to state of the arts stated in [34] to establish a fair comparison. Table 4 compares the E measure (Eφ) [35], S measure (Sα) [36], weighted F measure (���� ��) [37], and MAE performance of several approaches [38] As can be seen, the strategies introduced recently tend to produce superior results. Deep action learning with SqueezeNet, our suggested method, achieved the greatest results in terms of Eφ, Sα, ���� ��, and MAE. In every metric, deep action learning with the SqueezeNet backbone outperformed state of the art approaches by a significant margin.
Int J Artif Intell ISSN: 2252 8938
4.2.2.
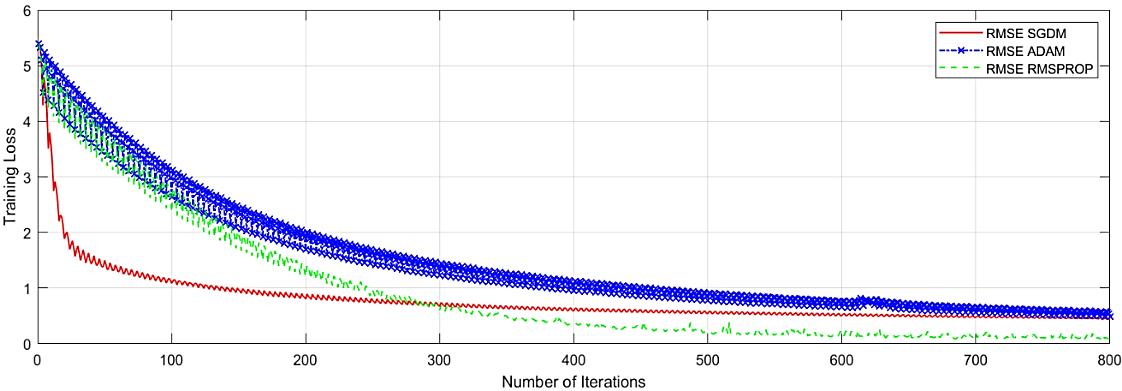
ISSN: 2252 8938 Int J Artif Intell, Vol. 11, No. 3, September 2022: 859 871 868 Table 3 Self correction process sequence on camouflage detection using deep action learning Input (��=��°) ����+�� ����+�� ����+�� �� ����| ���� (s)T 02° n/a 1.03 01° 0.790.92| 01° 0.960.94| na na 01° na 0.350.97 0° na 1.12 01° na 02° 0.76 01° 0.87 1.0400° 0.67 01° 0.88 na 02° 0.88 0.58 01° 0.98 Table 4 Comparison of methods performance on CAMO dataset Method Year Training Setting Evaluation Metrics ���� ⇑ ��∅ ⇑ ���� �� ⇑ ������ ⇓ Unet++ [39] 2018 CAMO [38] + COD [34] 0.599 0.653 0.392 0.149 CPD [34] 2019 CAMO [38] + COD [34] 0.726 0.729 0.550 0.115 SINet [34] 2020 CAMO [38] 0.708 0.706 0.476 0.131 MirrorNet [9] 2020 CAMO [38] 0.741 0.804 0.652 0.100 SquezeeNet 2021 CAMO [38] + extended 0.782 0.856 0.657 0.083 4.2.3. Experiments of self-correction on deep action learning In this subsection, we will discuss the self correction process using deep action learning. As presented in the preceeding subsection, in Figure 5, the detection results held values that were upgraded by the system. Self correction uses deep action learning, while the passing grade was set at ��0=0.95 in Figure 7(a) at the beginning of the system detected the target using SGDM optimizer which resulted in 0.89, 0.86, 0.92, and 0.90 at �� + 02°, because it failed to reach ��0=0.95, the system wastried to be updated this time using the ADAM optimizer at ��+0° as shown in Figure 7(b), and the detection results were 0.92, 0.90, 0.90, and 0.90. The cycle was added from cycle two to cycle three, where this time the input image was set at �� 01° and back to SGDM optimizer and now successfully passed ��0 as shown in Figure 7(c), which was set with detection results of 0.97, 0.95, 0.95, and 0.95 for each target.
Figure 7. A sequence of self correction in deep action learning, firstly, detection using SGDM optimizer (a), changed to ADAM optimizer (b) and switched back to SGDM optimizer (c) with internal assessment (a) (b) (c)
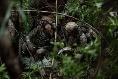
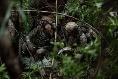
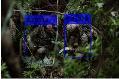
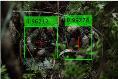
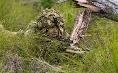
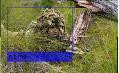
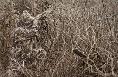
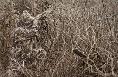
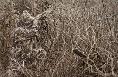
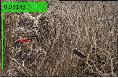
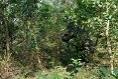
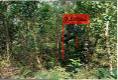
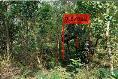
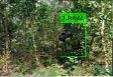
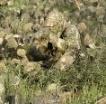
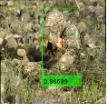
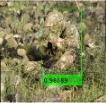
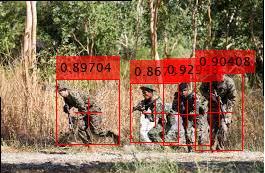
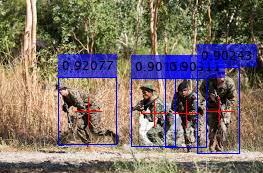
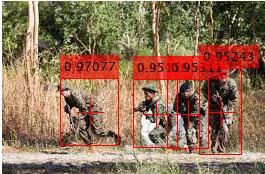
ACKNOWLEDGEMENTS
[19] Y. Ibrahim et al., “Soft errors in DNN accelerators: A comprehensive review,” Microelectron. Reliab., vol. 115, Dec. 2020, doi: 10.1016/j.microrel.2020.113969.
[7] S. Levine, P. Pastor, A. Krizhevsky, and D. Quillen, “Learning hand eye coordination for robotic grasping with deep learning and large scale data collection,” ArXiv160302199, Mar. 2016, [Online]. Available: http://arxiv.org/abs/1603.02199.
[14] H. T. L.Chiang, A. Faust, M. Fiser, and A. Francis,“Learningnavigation behaviors end to end with AutoRL,” IEEE Robot. Autom. Lett., vol. 4, no. 2, pp. 2007 2014, Apr. 2019, doi: 10.1109/LRA.2019.2899918.
[13] S. Chen, M. Wang, W. Song, Y. Yang, Y. Li, and M. Fu, “Stabilization approaches for reinforcement learning based end to end autonomous driving,” IEEE Trans. Veh. Technol., vol. 69, no. 5, pp. 4740 4750, May 2020, doi: 10.1109/TVT.2020.2979493.
5. CONCLUSION
[17] Y. Zou and R. Lan, “An end to end calibration method for welding robot laser vision systems With deep reinforcement learning,” IEEE Trans. Instrum. Meas., vol. 69, no. 7, pp. 4270 4280, Jul. 2020, doi: 10.1109/TIM.2019.2942533.
[15] G. Yang, R. Zhu, Z. Fang, C. Y. Chen, and C. Zhang, “Kinematic design of a 2R1T robotic end effector with flexure joints,” IEEE Access, vol. 8, pp. 57204 57213, 2020, doi: 10.1109/ACCESS.2020.2982185.
REFERENCES
[6] W. Yu, X. Wang, P. Calyam, D. Xuan, and W. Zhao, “Modeling and detection of camouflaging worm,” IEEE Trans. Dependable Secur. Comput., vol. 8, no. 3, pp. 377 390, May 2011, doi: 10.1109/TDSC.2010.13.
Authors thank Biomedic and Artificial Intelligence Laboratory of Engineering Faculty, Universitas Negeri Yogyakarta for financial support.
The exciting notion about self correction using deep action learning is that the shift in the bounding box localization results is insignificant. The target firing point is approximately the same if compared to the optimizer and viewing angle variations. In fact, Figure 7 structurally has a histogram pattern like Figures5(a)to(c)witharelativelyevendistributionofhistograms.Aquarteroftheapproximately 1249images trained in deep action learningutilizing CAMO datasets was used for testing in this study. However, in addition to the testing of CAMO dataset, accuracy is beyond the scope of this paper.
Int J Artif Intell ISSN: 2252 8938
[8] Y. Shen et al., “Rapid detection of camouflaged artificial target based on polarization imaging and deep learning,” IEEE Photonics J., vol. 13, no. 4, pp. 1 9, Aug. 2021, doi: 10.1109/JPHOT.2021.3103866.
[11] C. Y. Tsai, Y. S. Chou, C. C. Wong, Y. C. Lai, and C. C. Huang, “Visually guided picking control of an omnidirectional mobile manipulator based on end to end multi task imitation learning,” IEEE Access, vol. 8, pp. 1882 1891, 2020, doi: 10.1109/ACCESS.2019.2962335.
[12] A. Caglayan and A.B.Can, “Volumetricobject recognition using3 D CNNson depth data,” IEEE Access,vol.6,pp.20058 20066, 2018, doi: 10.1109/ACCESS.2018.2820840.
[3] Y. Shen, J. Li, W. Lin, L. Chen, F. Huang, and S. Wang, “Camouflaged target detection based on snapshot multispectral imaging,” Remote Sens., vol. 13, no. 19, Oct. 2021, doi: 10.3390/rs13193949.
[10] H. Shi, L. Shi, M. Xu, and K. S. Hwang, “End to end navigation strategy with deep reinforcement learning for mobile robots,” IEEE Trans. Ind. Informatics, vol. 16, no. 4, pp. 2393 2402, Apr. 2020, doi: 10.1109/TII.2019.2936167.
[4] K. Huang, C. Li, J. Zhang, and B. Wang, “Cascade and fusion: A deep learning approach for camouflaged object sensing,” Sensors, vol. 21, no. 16, Aug. 2021, doi: 10.3390/s21165455.
Thisstudyhasestablishedan effectivedeepactionlearningfortroopcamouflagerecognitiondetection in the CAMO dataset. A deep action learning designed with deep learning (YOLOv3) and action learning can detect and make firing points camouflaged in 2D image workspace. Inside, the YOLOv3 is equipped with SquezeeNet and modified the view angle on the input image driven by deep action learning. The processes of detecting troops in deep action learning include planning, acting, observing, and reflecting on whole steps without preprocessing. However, the results showed a value at 0.97 and 0.99 for accuracy and recall, respectively. Within a passing grade of 0.88, this evaluation mechanism calculated with an indefinite cycle in deep action learning. In the future, we intend to investigate the problem of camouflaged instance segmentation. For the experiment, we will improve video based detection using YOLOv4 or YOLOv5 with a preprocessing approach.
[5] J. Yu, G. Zhou, S. Zhou, and J. Yin, “A lightweight fully convolutional neural network for SAR automatic target recognition,” Remote Sens., vol. 13, no. 15, Aug. 2021, doi: 10.3390/rs13153029.
Troop camouflage detection based on deep action learning (Muslikhin) 869
[1] H. Xiao, Z. Qu, M. Lv, Y. Jiang, C. Wang, and R. Qin, “Fast self adaptive digital camouflage design method based on deep learning,” Appl. Sci., vol. 10, no. 15, Jul. 2020, doi: 10.3390/app10155284.
[2] H. Lu, X. Wang, S. Liu, M. Shi, and A. Guo, “The possible mechanism underlying visual anti camouflage: a model and its real time simulation,” IEEE Trans. Syst. Man, Cybern. Part A Syst. Humans, vol. 29, no. 3, pp. 314 318, May 1999, doi: 10.1109/3468.759290.
[9] J. Yan, T. N. Le, K. D. Nguyen, M. T. Tran, T. T. Do, and T. V Nguyen, “MirrorNet: Bio inspired camouflaged object segmentation,” IEEE Access, vol. 9, pp. 43290 43300, 2021, doi: 10.1109/ACCESS.2021.3064443.
[16] M. Yasin, B. Mazumdar, O. Sinanoglu, and J. Rajendran, “Removal attacks on logic locking and camouflaging techniques,” IEEE Trans. Emerg. Top. Comput., vol. 8, no. 2, pp. 517 532, Apr. 2020, doi: 10.1109/TETC.2017.2740364.
[18] V. Digani, L. Sabattini, and C. Secchi, “A probabilistic eulerian traffic model for the coordination of multiple AGVs in automatic warehouses,” IEEE Robot. Autom. Lett., vol. 1, no. 1, pp. 26 32, Jan. 2016, doi: 10.1109/LRA.2015.2505646.
[39] Z. Zhou, M. M. Rahman Siddiquee, N. Tajbakhsh, and J. Liang, “UNet++: A nested U net architecture for medical image segmentation,” in DLMIA 2018, ML CDS 2018: Deep Learning in Medical Image Analysis and Multimodal Learning for Clinical Decision Support, 2018, pp. 3 11. BIOGRAPHIES OF AUTHORS Muslikhin is robotics and artificial intelligent researcher who expertreceived. His B.S. and M.S. degrees in electronics engineering education, and technology and vocational education from Universitas Negeri Yogyakarta, Yogyakarta, Indonesia in 2011 and 2013, respectively. In 2021, he received his Ph.D. in electrical engineering from Southern Taiwan University of Sciences and Technology in Tainan, Taiwan. Robotics, machine vision, and artificial intelligence are among his research interests. In addition, he took first place in the TIRT International Innovative Robotics Festival in Taoyuan City, Taiwan,in2020.Theteamused an AIoTrobotictohelpquarantineCOVID 19patientsin thiscompetition. He can be contacted at email: muslikhin@uny.ac.id Aris Nasuha is a machine learning researcher with a focus on research and development. He has received a B.Sc. (physics) from Universitas Gadjah Mada, M.Eng. and Dr. (electrical engineering) from Institut Teknologi Sepuluh Nopember, Indonesia respectively. His research interest includes digital signal processing, digital image processing and machine learning. Now he is head of Electronics Engineering Departmentat Vocational School Program, Universitas NegeriYogyakarta,Indonesia.Hecan be contacted at email: arisnasuha@uny.ac.id
[35] D. P. Fan, C. Gong, Y. Cao, B. Ren, M. M. Cheng, and A. Borji, “Enhanced alignment measure for binary foreground map evaluation,” in Proceedings of the Twenty Seventh International Joint Conference on Artificial Intelligence, Jul. 2018, pp. 698 704, doi: 10.24963/ijcai.2018/97.
[38] T. N. Le, T. V Nguyen, Z. Nie, M. T. Tran, and A. Sugimoto, “Anabranch network for camouflaged object segmentation,” Comput. Vis. Image Underst., vol. 184, pp. 45 56, Jul. 2019, doi: 10.1016/j.cviu.2019.04.006.
[25] O. Serrat, “Action learning,” in Knowledge Solutions, Singapore: Springer Singapore, 2017, pp. 589 594.
[22] P. V. Mohan, S. Dixit, A. Gyaneshwar, U. Chadha, K. Srinivasan, and J. T. Seo, “Leveraging computational intelligence techniques for defensive deception: A review, recent advances, open problems and future directions,” Sensors, vol. 22, no. 6, Mar. 2022, doi: 10.3390/s22062194.
[23] C. Brook and M. Pedler, “Action learning in academic management education: A state of the field review,” Int. J. Manag. Educ., vol. 18, no. 3, Nov. 2020, doi: 10.1016/j.ijme.2020.100415.
[37] R. Margolin, L. Zelnik Manor, and A. Tal, “How to evaluate foreground maps,” in 2014 IEEE Conference on Computer Vision and Pattern Recognition, Jun. 2014, pp. 248 255, doi: 10.1109/CVPR.2014.39.
[27] H. Altrichter, S. Kemmis, R. McTaggart, and O. Zuber‐Skerritt, “The concept of action research,” Learn. Organ., vol. 9, no. 3, pp. 125 131, Aug. 2002, doi: 10.1108/09696470210428840. [28] L. M. Bell and J. M. Aldridge, Student voice, teacher action research and classroom improvement. Rotterdam: SensePublishers, 2014. [29] W. Fang, L. Wang, and P. Ren, “Tinier YOLO: A real time object detection method for constrained environments,” IEEE Access, vol. 8, pp. 1935 1944, 2020, doi: 10.1109/ACCESS.2019.2961959. [30] B. Dick, “Action research literature 2006 2008,” Action Res., vol. 7, no. 4, pp. 423 441, Dec. 2009, doi: 10.1177/1476750309350701. [31] J. Whitehead and J. McNiff, Action research living theory. London: Thousand Oaks: SAGE Publications, 2006.
[33] E. T. Stringer, L. M. Christensen, and S. C. Baldwin, Integrating teaching, learning, and action research: enhancing instruction in the K 12 classroom. Thousand Oaks, Calif: Sage, 2010. [34] D. P. Fan, G. P. Ji, G. Sun, M. M. Cheng, J. Shen, and L. Shao, “Camouflaged object detection,” in 2020 IEEE/CVF Conference on Computer Vision and Pattern Recognition (CVPR), Jun. 2020, pp. 2774 2784, doi: 10.1109/CVPR42600.2020.00285.
[21] H. Li, A. Abdelhadi,R. Shi, J. Zhang,and Q. Liu, “Adversarial hardware with functional and topological camouflage,” IEEE Trans. Circuits Syst. II Express Briefs, vol. 68, no. 5, pp. 1685 1689, May 2021, doi: 10.1109/TCSII.2021.3065292.
[26] B. Dick, E. Stringer, and C. Huxham, “Theory in action research,” Action Res., vol. 7, no. 1, pp. 5 12, Mar. 2009, doi: 10.1177/1476750308099594.
[32] L. Norton, “Action research in teaching and learning: a practical guide to conducting pedagogical research in universities.” 2019, Accessed: Jun. 11, 2021. [Online]. Available: http://search.ebscohost.com/login.aspx?direct=true&scope=site&db=nlebk&db=nlabk&AN=1926353.
ISSN: 2252 8938 Int J Artif Intell, Vol. 11, No. 3, September 2022: 859 871 870 [20] Y. Kim, H. Kang, N. Suryanto, H. T. Larasati, A. Mukaroh, and H. Kim, “Extended spatially localized perturbation GAN (eSLP GAN) for robust adversarial camouflage Patches,” Sensors, vol. 21, no. 16, Aug. 2021, doi: 10.3390/s21165323.
[24] R. Van Gasse, K. Vanlommel, J. Vanhoof, and P. Van Petegem, “Teacher interactions in taking action upon pupil learning outcome data: A matter of attitude and self efficacy?,” Teach. Teach. Educ., vol. 89, Mar. 2020, doi: 10.1016/j.tate.2019.102989.
[36] D. P. Fan, M. M. Cheng, Y. Liu, T. Li, and A. Borji, “Structure measure: A New Way to Evaluate Foreground Maps,” ArXiv:170800786, Aug. 2017, [Online]. Available: http://arxiv.org/abs/1708.00786.
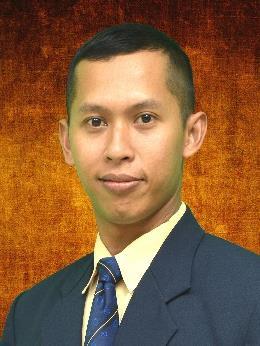



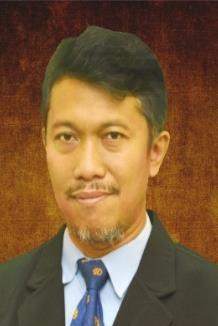



Int J Artif Intell ISSN: 2252 8938
Troop camouflage detection based on deep action learning (Muslikhin)
871
Suprapto received B.S. degree from the department of electrical engineering education, Universitas Negeri Yogyakarta in 2001. Afterward, he received M.S. degree from the department of electrical engineering, Gadjah Mada University in 2005. He received Ph.D. degree in control theory, electrical engineering, National University of Science and Technology, Taiwan in January 2018. Since 2005, he has been a lecturer at the Departement of Electronics Engineering, Yogyakarta State University, Yogyakarta, Indonesia. His current research interest is related to control theory, signal processing, and computational intelligence. He can be contacted at email: suprapto@uny.ac.id Anggun Winursito is a researcher with a focus on signal processing and instrumentation. He has received M.Eng. from Gadjah Mada University and B.Ed. from Universitas Negeri Yogyakarta in 2014 and 2018. His areas of expertise are speech recognition, image recognition, analog and digital signal processing, and instrumentation. He has participated in several local and national research projects including speech pattern recognition and radar telecommunications systems. He was awarded the best paper at International Conference on Smart Computing and Electronic Enterprise (ICSCEE) held in Malaysia for his research on the compression of speech features to improve the accuracy of speech recognition systems. He can be contacted at email: anggunwinursito@uny.ac.id
Fatchul Arifin is received a B.Sc. in electric engineering at Universitas Diponegoro in 1996. Afterward,He received master’sdegree fromthedepartment ofelectrical engineering, Institutut Teknologi Bandung, Indonesia in 2003 and doctoral degree in electric engineering from Institut Teknologi Sepuluh November, Surabaya, Indonesia in 2014. Currently he is the lecturer at both undergraduate and postgraduateelectronicsandinformaticprogramatUniversitasNegeriYogyakarta,Indonesia.Hisresearch interests include but not limited to artificial intelligent systems, machine learning, fuzzy logic, and biomedical engineering system. He can be contacted at email: fatchul@uny.ac.id
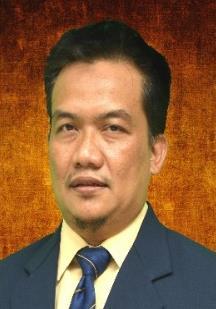



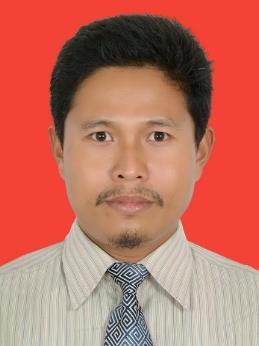



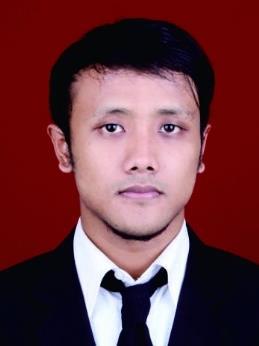



This is an open access article under the CC BY SA license. Corresponding Author: Srividhya Srinivasa Raghavan Department of Computer science Engineering, Bharath Institute of Higher Education and Research Bharath University Men’s Hostel, Tiruvanchery, Selaiyur, Chennai, Tamil Nadu 600126, India Email: vidhyasrinivasan1890@gmail.com
Article Info ABSTRACT
Article history: Received Sep 4, 2021 Revised Mar 30, 2022 Accepted Apr 28, 2022 Diagnosis is being used in a variety of fields, including treatments, scientific knowledge, technology, industry, and many deals. A diagnosis begins with the person’s complaints and understanding something about the condition of the patient dynamically while in a question and answer session, as well as by taking measurements, like blood pressure or skin temperature, among other things. The prognosis is then calculated by considering the obtainable patient information. The adequate intervention is then prescribed, and the method may be repeated. In the medical field, humans, sometimes, have constraints when diagnosing diagnosis, primarily because this procedure is arbitrary and heavily relies on the assessor’s memories and perception of patient transmissions. The work is primarily concerned with the investigation of cerebellar aneurysm diagnosing. In the meantime, it’s become evident even during literature reviews research that a much more basis of theoretical research of a number of existing learning methods was required. As a result, this paper is to provide a comparison of classification techniques like tree structure, random trees, and regression. At about the same time, another important goal is to have a decision making framework based on biomimetic elephant whale enhancement for a great deal of consideration of cerebral aneurysm variables, providing a quick, accurate, and dependable clinical medicine remedy.
Journal homepage: http://ijai.iaescore.com and deep learning approach for cerebral aneurysms prediction in healthcare environment
Srividhya Srinivasa Raghavan1, Arunachalam Arunachalam2 1Department of Computer Science, Biher, India 2Dr. M. G. R. Educational and Research Institute (Phase II), Tamil Nadu, India
Bio-inspired
1. INTRODUCTION
IAES International Journal of Artificial Intelligence (IJ AI) Vol. 11, No. 3, September 2022, pp. 872 877 ISSN: 2252 8938, DOI: 10.11591/ijai.v11.i3.pp872 877 872
Since about the 1970s, the use of computer programs that rely on artificial intelligence (AI) techniques in the medical field has increased in number. AI is the study of how to replicate human intellect in computers. There have been computer programs that can assist physicians in diagnostics, and they’ve been used routinely in many medical settings. AI technologies can also be used to create features that enable therapy making plans, information gathering, alert creation, and patient monitoring, among other things. Medical decision support processes (MDSP) are computerized intelligent systems that use relevant information to create patient specific guidance or perception. A lot of scientists have expressed awareness of the potential of AI in treatments, which is summarized: i) offers a lab for medical training examination, organization, recognition, and cataloging; ii) creates new tools to aid in appropriate medical, education, and research; iii) combines activities in medicine, computer programming, cognitive neuroscience, and other fields; iv) offers valuable content self control for modern research medical specialization.
Keywords: Bio inspired algorithm Computed LinearElephantDecisionangiographytomographymakingframeworkwhaleoptimizationregression
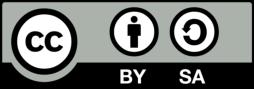
Medical clinical decisions (like treatment decisions) for a specific case is a complicated process that requires a variety of factors like various illnesses and illness creates, expense, a patient’s physical and mental state, and so on. Problems include obtaining, collecting, and collecting information that will be used to train the network. It becomes a major concern, particularly when the process needs large amounts of data over long durations, which are frequently unavailable given the lack of effective recording systems and information privacy concerns. Because of the complexity (or lack of advisability) of developing better designs from small datasets while considering so many factors, most intelligent processes in the medical environment have concentrated on designing for specific software testing, also known as classification tasks.
Int J Artif Intell ISSN: 2252 8938 Bio inspired and deep learning approach for cerebral aneurysms … (Srividhya Srinivasa Raghavan) 873
Artificial neural systems (ANSs) as well as kernel based designs possess powerful learning capacity and can typically achieve higher correctness in classifier than previous white box designers; however, the designer’s perception is difficult, and it is typically called a black box design.
In the initial periods of biomedical processes, the information technology community predicted the imminent emergence of powerful machines for trying to deal with the field of biomedical information. Pattern matching methods were used in one initial approach. Recognition system is now more frequently affiliated with image analysis [1], but in the beginning, it started referring to methodologies that permitted the computer to browse for structures. Images or organizations of variables related to specific ailments could be used to create these trends. The latter implementation has become more commonly referred to as pattern recognition [2]. Various approaches get their own set of advantages and disadvantages. Decision tree algorithms and guideline structures, for example, are easily understandable for humans and are commonly known as white box designs; even so, they are ineffective when communicating with continuous data.
The creation of a clever healthcare system is a hard process. The phase of data gathering presents challenges, such as how to incorporate heterogeneous patient records varying from patient’s condition to physical examination, and from alcohol prescribing to lab tests. It is also difficult to execute a solution that matches the path clinicians’ purpose, assume, and operate. There are numerous issues to consider when implementing different concepts for biomedical devices, with the health files posing the most difficulty.
The following goals were set in order to reach the aims of successfully implementing a decision making framework for medical diagnosing: i) investigate various disorder large datasets available online, as well as standardize data sources in the Indian context. As a result, data points for cerebellar aneurysms predicting the presence must be gathered from various sources of data; ii) there is a problem with data collection and measurement for computation, particularly in India. One of the goals is to gather information from Indian hospitals and standardize the given dataset. An effective strategy must be developed using the obtainable health experts’ information inputs and hospital information to recompense for the omitted information in the data set. Thus, information must be preprocessed and analyzed (extracting features
Figure 1. Computed tomography angiography image of brain with cerebral aneurysm
The theory only addresses the medicine classification task, which is critical in treatment decisions. The section that follows attempts to provide a brief overview of the role of computer vision in medical uses, as well as the advantages and challenges of clinical information systems.
The concept augmentation of cerebellar aneurysm explanation is depicted in Figure 1. The dark spot in the figure represents the punctured region of the disorder for doctors to identify. Viewers have seen a semi opaque red layer on the axisymmetric, transverse, and corona discharge sequence at every coordinate where the theory testing a likelihood greater than 0.5. Even before trying to read with design expansion, clinicians were given the model’s conjectures in the shape of return on investment (ROI) categories that were straight layered on the upper edge of computed tomographic angiography (CTA) tests. To make sure that all physicians were familiar with the enhanced image interaction.
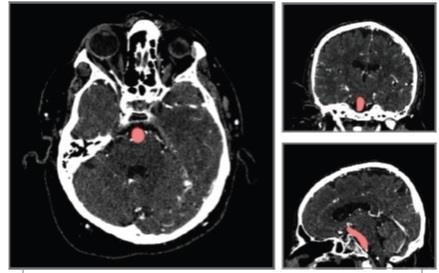
ELEPHANT-WHALE OPTIMIZATION (EWO)
2.
Step 1: The hunt procedure iteratively refines the answer to get the ideal arrangement.
Step 2: Boss female elephants lead concentrated neighborhood look at spots, where a higher likelihood of finding the best arrangement is normal.
In this segment, a novel method for predicting efficient variables to integrate into the diagnosis of cerebral aneurysm disorder is discussed. The elephant whale optimization (EWO) method was created predicated on their behavior in determining the best communication for obtaining food as a goal. EWO has a place with the gathering of contemporary met heuristic inquiry improvement algorithms. This imitates the conduct and attributes of an elephant, and its system depends on a double inquiry instrument, or the pursuit operators can be separated into two gatherings. In this situation, EWO has three fundamental qualities as powerful inquiry streamlining algorithm;
One of the main goals of this research was to automatically classify cerebellar aneurysms based on their rupture prestige using various cutting edge data mining methods. Classification models [5] were created and implemented with a chosen range of attributes in accordance with the variables shown in Table 1, and the correctness of the classifiers was evaluated with fresh aneurysm situations. Because they are generated from diverse sources, the prospective selection of features is incredibly large and innately multisensory. Clinical information [6] is made up of data that has traditionally been used in clinical practice, like demographic trends (e.g., age, gender), style of living (e.g., smoker status), and aneurysm anatomy size and type. The Table 1 shows the medical features and their specification. Using these parameters, cerebral aneurysm can be diagnosed.
3.1. Support vector machines (SVM) SVM [7] is a set of methods for classifying both linear and nonlinear information. It converts the actual information into high dimensional storage, from which a hyper plane is calculated to split the data based on every instance’s category. New input can be categorized by tracing it into a spot in a similar dimensional that the hyper plane has already split (categorized).
ISSN: 2252 8938 Int J Artif Intell, Vol. 11, No. 3, September 2022: 872 877 874evaluation); iii) using an innovative bio inspired wildlife optimization technique, develop a strategic recommendation for parameter estimation; iv) determine the optimal prototype and conduct a comparative analysis of the various methodologies. Machine learning algorithms [3] appropriate for computer aided clinical diagnosis are inclusive of diagnostic understanding disclosure and elaboration ability. Even though medical interventions have advanced and become more effective and sophisticated in recent years as a result of technological advancements (e.g., CAT/MRI scanner and microscopy), the fact still remains that these techniques have not attained many states (both establishing and created) at the same stage. This was a significant disadvantage that hampered the investigation because data gathering, digitization, and planning take around a year a priori. Furthermore, experts discuss and debate if the symbolic design (knowledge based systems with expert input) or the numerical method (model recognition and neural systems with data derived awareness) is the best way to accommodate profiles. As a result, it is essential to investigate medical decision support systems and propose a technique for accurate assessment of cerebral aneurysms [4]
Step 3: The male elephants have obligations of investigations out of the nearby optima. Whales are the greatest mammals among all creatures and they are excessive creatures. There are some significant principle parts of this creature, for example, humpback, executioner, blue, and finback. Whales never rest on the grounds that they have to inhale more often than not from the oceans and seas. Besides, half of the minds can just rest. Whales live alone or in gatherings. A portion of their part, for example, the executioner whales can live in a family for a large portion of their life. The humpback whales are considered the greatest whales, and their preferred prey is little fish and krill species. The exceptional chasing method for humpback whales is considered as the primary intriguing purpose of these whales which can be characterized as a bubble net feeding strategy. This strategy is used to find out the best pre processing for data analytics.
3. RESEARCH METHODOLOGY
3.2. The decision tree A decision tree is a classification algorithm [8] that subdivides the computer course iteratively until every partition corresponds to (primarily) one class. The precision of the classification model is determined by which feature is used at every partitioning stage. In this context, gain ratio initiatives are used.
1 LinearModelRegression 0.608 0.04 0.1647 87%
2 Random Forest 0.567 0.04 0.0764 78% 3 Decision Tree 0.45 0.09 0.0024 77%
Fuzzy rules [10] method creates rules in higher dimensional areas that are fuzzy pauses. For every dimension, trapezoid fuzzified features describe such hyper rectangles. Every rule is made up of one fuzzified intermission for every dimension, as well as which includes columns and an amount of rule readings nodes.
4. RESULTS AND DISCUSSION
To use the posterior distribution calculated from the observed cases, this adoption of data is the highest posterior distribution class [9]. This method usually works well in exercise, despite the fact that it is based on presumptions that are not always true. To begin with, all characteristics are considered to be distributed. Second, for every feature, quantities are presumed to test the normality.
3.4. The fuzzy rules
Int J Artif Intell ISSN: 2252 8938 Bio inspired and deep learning approach for cerebral aneurysms … (Srividhya Srinivasa Raghavan) 875 Table 1. Medical features Parameter Specification
3.5. Networks of neurons
3.3. Bayesian classifier
From the above table and the graph, it explains that linear regression shows the maximum accuracy of 87% comparatively, whereas random forest and decision tree yields 78% and 77% respectively. The above graph shows the accuracies [23] of the models, which will be useful [24] when tracing out the cerebral aneurysm at the earlier stage as a result the mortality rate [25] can be reduced.
Table 2 Comparison results S. No Algorithm/Model Correlationcoefficient absoluteMeanerror Root squaredmeanerror (AfterAccuracyCrossValidation)
Neuter Feminine and Masculine Age Positively From 22 To 84 locality of Aneurysm Values categorized up to 24 dominance of Aneurysm 3 ways Aneurysm Type Bifurification, Two ways of dissecting sidewalls
The data sets consist of 20 samples with respect to the parameters as shown in Table 1. The software tool used for implementation is weka 3.9.2 which is one of the important data mining tools [17]. Table 2 and Figure 2 show the comparison results of the prediction [18] of the cerebral aneurysm from the training datasets. The table also shows the accuracy of prediction where linear regression [19] and decision tree [20] and random forest [21]. The accuracy [22] is calculated using (1) �� =����+�� (1)
For forecasting to estimate the number, neural pathways [11] are frequently used. The systems are made up of non linear technological sides that are organized in patterns similar to biological neural systems. Weights that are adjusted during the training process are used to arrange computational components in several stacks [12]. In our experimentations, we used probability based neural network models, which do not involve the system architecture to be defined and understood, generate more portable interpretations, and outperform a traditional number of layers conceptions. Predictive affiliation principles. Categorization predictive associative rules (CPAR) [13] is an inference method that manages to avoid producing larger data sets compilations, as is the instance of more conventional cluster analysis methodologies like FP growth [14]. Every one of the samples in the training collection has its own set of regulations. These regulations are built incrementally by potentially suitable to the precursor [15]. In order to determine which, accredit to add to the predictors of a principle, an indicator of 'benefit' in the classifier’s precision [16] is calculated.
REFERENCES
[1] M. L. Antonie, O. R. Zaïane, and A. Coman, “Application of data mining techniques for medical image classification,” in Proceedings of the Second International Conference on Multimedia Data Mining, 2001, pp. 94 101.
5. CONCLUSION
[4] A. Chien, M. A. Castro, S. Tateshima, J. Sayre, J. Cebral, and F. Viñuela, “Quantitative hemodynamic analysis of brain aneurysms at different locations,” Am. J. Neuroradiol., vol. 30, no. 8, pp. 1507 1512, Sep. 2009, doi: 10.3174/ajnr.A1600.
[5] U. Kurkure, D. R. Chittajallu, G. Brunner, Y. H. Le, and I. A. Kakadiaris, “A supervised classification based method for coronary calcium detection in non contrast CT,” Int. J. Cardiovasc. Imaging, vol. 26, no. 7, pp. 817 828, Oct. 2010, doi: 10.1007/s10554 010 9607 2. [6] A. Frangi, “The @neurIST project: towards understanding cerebral aneurysms,” SPIE Newsroom, 2007, doi: 10.1117/2.1200706.0782. [7] K. P. Bennett and C. Campbell, “Support vector machines,” ACM SIGKDD Explor. Newsl., vol. 2, no. 2, pp. 1 13, Dec. 2000, doi: 10.1145/380995.380999. [8] Y. B. W. E. M. Roos et al., “Direct costs of modern treatment of aneurysmal subarachnoid hemorrhage in the first year after diagnosis,” Stroke, vol. 33, no. 6, pp. 1595 1599, Jun. 2002, doi: 10.1161/01.STR.0000016401.49688.2F.
[9] A. Abdehkakha, A. L. Hammond, T. R. Patel, A. H. Siddiqui, G. F. Dargush, and H. Meng, “Cerebral aneurysm flow diverter modeled as a thin inhomogeneous porous medium in hemodynamic simulations,” Comput. Biol. Med., vol. 139, p. 104988, Dec.
The most common brain disease is a cerebral aneurysm. It occurs when the vein in the brain bulges and hemorrhage happens. In the work carried out, the detailed study on cerebral aneurysm disease, machine learning concepts are employed. The proposed method provides a novel methodology inspired by a bio inspired algorithm, Also, the classification techniques have been discussed with a simple analysis. The proposed technique will provide reports proximately after clinical outcome with lesser cost. It is helpful for patients who are suffering from cerebral aneurysms. The technique will help to reduce the mortality rate by diagnosing the cerebral aneurysm at an early stage. As a result, the purpose of this paper is to make a comparison of classification techniques like random trees, and regression analysis. At about the same time, an evenly important goal is to have a decision making framework based on biomimetic elephant whale enhancement for a great deal of consideration of cerebral aneurysm variables, providing a quick, accurate, and dependable clinical medicine remedy.
ISSN: 2252 8938 Int J Artif Intell, Vol. 11, No. 3, September 2022: 872 877 876 Figure 2. Prediction of cerebral aneurysm
[2] X. Tan, H. Pan, Q. Han, and J. Ni, “Domain knowledge driven association pattern mining algorithm on medical images,” in 2009 Fourth International Conference on Internet Computing for Science and Engineering, Dec. 2009, pp. 30 35, doi: 10.1109/ICICSE.2009.65. [3] S. R. S. Vidhya and A. R. Arunachalam, “Machine learning techniques for morphologic and clinical features extraction of cerebral aneurysm,” in 2021 10th IEEE International Conference on Communication Systems and Network Technologies (CSNT), Jun. 2021, pp. 617 621, doi: 10.1109/CSNT51715.2021.9509565.
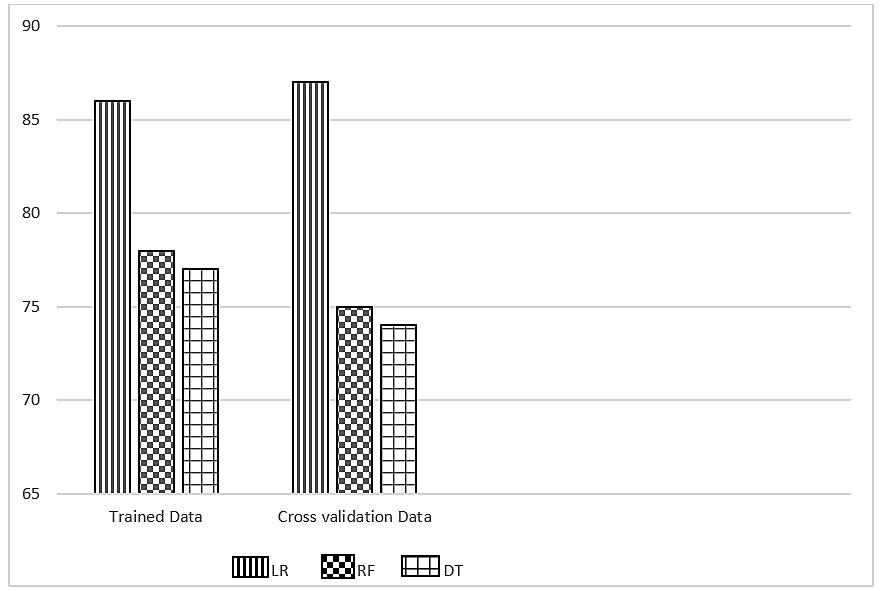
[19] J. R. Cebral, F. Mut, J. Weir, and C. M. Putman, “Association of hemodynamic characteristics and cerebral aneurysm rupture,” Am. J. Neuroradiol., vol. 32, no. 2, pp. 264 270, Feb. 2011, doi: 10.3174/ajnr.A2274.
[25] S. Kimura et al., “Subarachnoid hemorrhage due to ruptured cerebral aneurysm at the distal part of anterior inferior cerebellar artery posterior inferior cerebellar artery variant after $γ$ knife irradiation,” Interdiscip. Neurosurg., vol. 29, p. 101533, Sep. 2022, doi: 10.1016/j.inat.2022.101533.
[10] N. Etminan et al., “Cerebral aneurysms: formation, progression, and developmental chronology,” Transl. Stroke Res., vol. 5, no. 2, pp. 167 173, Apr. 2014, doi: 10.1007/s12975 013 0294 x. [11] L. B. Stam, R. Aquarius, G. A. de Jong, C. H. Slump, F. J. A. Meijer, and H. D. Boogaarts, “A review on imaging techniques and quantitative measurements for dynamic imaging of cerebral aneurysm pulsations,” Sci. Rep., vol. 11, no. 1, p. 2175, Dec. 2021, doi: 10.1038/s41598 021 81753 z. [12] G. Toth and R. Cerejo, “Intracranial aneurysms: Review of current science and management,” Vasc. Med., vol. 23, no. 3, pp. 276 288, Jun. 2018, doi: 10.1177/1358863X18754693.
[13] S. Kim, K. W. Nowicki, B. A. Gross, and W. R. Wagner, “Injectable hydrogels for vascular embolization and cell delivery: The potential for advances in cerebral aneurysm treatment,” Biomaterials, vol. 277, p. 121109, Oct. 2021, doi: 10.1016/j.biomaterials.2021.121109.
[14] S. Parthasarathy and C. C. Aggarwal, “On the use of conceptual reconstruction for mining massively incomplete data sets,” IEEE Trans. Knowl. Data Eng., vol. 15, no. 6, pp. 1512 1521, Nov. 2003, doi: 10.1109/TKDE.2003.1245289.
[15] M. X. Ribeiro, A. G. R. Balan, J. C. Felipe, A. J. M. Traina, and C. Traina, “Mining statistical association rules to select the most relevant medical image features,” in Studies in Computational Intelligence, Springer Berlin Heidelberg, 2009, pp. 113 131. [16] M. X. Ribeiro, A. J. M. Traina, C. T. Jr, N. A. Rosa, and P. M. de A. Marques, “How to improve medical image diagnosis through association rules: The IDEA method,” in 2008 21st IEEE International Symposium on Computer Based Medical Systems, Jun. 2008, pp. 266 271, doi: 10.1109/CBMS.2008.55. [17] S. C. Johnston et al., “Endovascular and surgical treatment of unruptured cerebral aneurysms: Comparison of risks,” Ann. Neurol., vol. 48, no. 1, pp. 11 19, Jul. 2000, doi: 10.1002/1531 8249(200007)48:1<11::AID ANA4>3.0.CO;2 V. [18] H. G. Lee, K. Y. Noh, and K. H. Ryu, “A data mining approach for coronary heart disease prediction using HRV features and carotid arterial wall thickness,” in 2008 International Conference on BioMedical Engineering and Informatics, May 2008, pp. 200 206, doi: 10.1109/BMEI.2008.189.
[20] S. Benkner et al., “@neurIST: Infrastructure for advanced disease management through integration of heterogeneous data, computing, and complex processing services,” IEEE Trans. Inf. Technol. Biomed., vol. 14, no. 6, pp. 1365 1377, Nov. 2010, doi: 10.1109/TITB.2010.2049268. [21] M. R. Berthold and J. Diamond, “Constructive training of probabilistic neural networks,” Neurocomputing, vol. 19, no. 1 3, pp. 167 183, Apr. 1998, doi: 10.1016/S0925 2312(97)00063 5. [22] J. Iavindrasana, A. Depeursinge, P. Ruch, S. Spahni, A. Geissbuhler, and H. Müller, “Design of a decentralized reusable research database architecture to support data acquisition in large research projects,” Stud. Heal. Technol. Informatics, vol. 129, pp. 325 329, 2007. [23] M. R. Berthold, “Mixed fuzzy rule formation,” Int. J. Approx. Reason., vol. 32, no. 2 3, pp. 67 84, Feb. 2003, doi: 10.1016/S0888 613X(02)00077 4. [24] R. J. A. Little and D. B. Rubin, Statistical analysis with missing data. Hoboken, NJ, USA: John Wiley & Sons, Inc., 2002.
BIOGRAPHIES OF AUTHORS Srividhya Srinivasa Raghavan has graduated from SCSVMV University with Bachelor’s Degree in Information Technology she has received her M.E degree in Computer Science and Engineering from AnnaUniversity, Chennai in 2015. She has more than five years of teaching experience in the Department of Computer Science and Engineering in various colleges. She is currently serving as Assistant Professor at the School of Computing, Sathyabama Institute of Science and Technology, Chennai. She is currently pursuing her research work in medical image processing and deep learning. Her research interests are AI, image processing, and Big Data. She has presented her research findings in various reputed national/international journals and also in the proceedings of national/ international conferences. She can be contacted at email: vidhyasrinivasan1890@gmail.com
Dr. Arunachalam Arunachalam is currently working as Dean (Academic) of Dr.M.G.R. Educational and Research Institute (Deemed to be University) Adayalampattu Phase II Campus, Chennai, India. He has received his graduation from Madras University with Bachelor’s Degree in Computer Science and Engineering in the year 2002. He has received his M. Tech degree in Computer Science and Engineering from Bharath Institute of Higher Education and Research, Chennai in the year 2007 and a Ph.D. degree in Computer Science and Engineering from Bharath Institute of Higher Education and Research, Chennai, India in the year 2017. Some of his research findings are published in top cited journals. His research areas of interest include Computer Networks, Artificial Intelligent, Image Processing and Big Data. He can be contacted at email: arunachalam.cse@drmgrdu.ac.in
Int J Artif Intell ISSN: 2252 8938 Bio inspired and deep learning approach for cerebral aneurysms … (Srividhya Srinivasa Raghavan) 877 2021, doi: 10.1016/j.compbiomed.2021.104988.
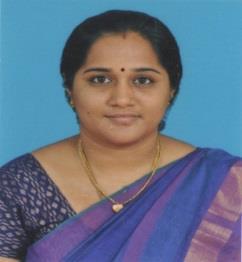



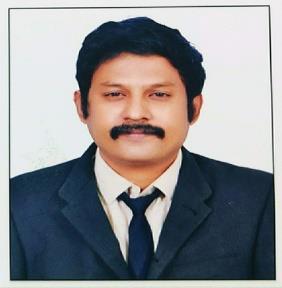



IAES International Journal of Artificial Intelligence (IJ AI) Vol. 11, No. 3, September 2022, pp. 878 885 ISSN: 2252 8938, DOI: 10.11591/ijai.v11.i3 pp878 885 878
Keywords: CICDDoS2019 dataset Data flooding attack Deep learning Deep neural network Intrusion detection system SYNMANETsflooding attack
MANETs have a number of advantages over classical networks, in that they can straightforwardly be implement and disassemble, as well as the flexibility provided by the fact that the nodes are not attached.
Oussama Sbai, Mohamed Elboukhari Department of Applied Engineering, ESTO (Higher School of Technology) Mohammed 1st University, Oujda, Morocco
Deep learning intrusion detection system for mobile ad hoc networks against flooding attacks
UDP flooding attack
MANET’s applications are in continuous development and cover a variety of areas, like vehicular ad hoc network (VANET) [1] in smart road traffic [2], smart cities and smart home, in general smart environment [3]. Furthermore, flay ad hoc network (FANET) in smart air traffic [4]. Besides being operable as a stand alone network, ad hoc networks can also be attached to the Internet [5], such as the paradigm of internet of things (IoT) [6] and internet of vehicle (IoV) [7] Intrusion detection system (IDS) is the mechanism used by the network’s nodes for monitoring and analyzing the network traffics, for which of these last represent a breach of security policy and standards,
This is an open access article under the CC BY SA license. Corresponding Author: Oussama DepartmentSbaiof Applied Engineering, ESTO (Higher School of Technology) Mohammed 1st University Oujda, Morocco Email: o.sbai@ump.ac.ma 1. INTRODUCTION A mobile ad hoc network (MANET) is a self organizing group, self connected of mobile nodes without using central administration and fixed infrastructure. When a node wants to create a connection with another node outside of its communication range, its node’s neighbors collaborate with it and transmit the messages. Therefore, the nodes of MANETs behave as a router as well as a host. The network’s topology is temporary and constantly changing. Added to that, nodes can leave the network and new ones can join it.
Article Info ABSTRACT
Journal homepage: http://ijai.iaescore.com
Article history: Received Apr 16, 2021 Revised May 21, 2022 Accepted Jun 2, 2022 Mobile ad hoc networks (MANETs) are infrastructure less, dynamic wireless networks and self configuring, in which the nodes are resource constrained. With the exponential evolution of the paradigm of smart homes, smart cities, smart logistics, internet of things (IoT) and internet of vehicle (IoV), MANETs and their networks family, such as flying ad hoc networks (FANETs), vehicular ad hoc networks (VANETs), and wireless sensor network (WSN), are the backbone of the whole networks. Because of their multitude use, MANETs are vulnerable to various attacks, so intrusion detection systems (IDS) are used in MANETs to keep an eye on activities in order to spot any intrusions into networks. In this paper, we propose a knowledge based intrusion detection system (KBIDS) to secure MANETs from two classes of distributed denial of service (DDoS) attacks, which are UDP/data and SYN flooding attacks. We use the approach of deep learning exactly deep neural network (DNN) with CICDDoS2019 dataset. Simulation results obtained show that the proposed architecture model can attain very interesting and encouraging performance and results (Accuracy, Precision, Recall and F1 score).
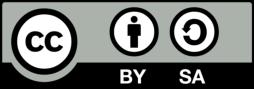
ABID: Anomaly based or behavior based intrusion detection. KBID: Knowledge based, also known as Misuse or Signature intrusion detection.
2. RELATED WORKS
SBID: Specification based intrusion detection. Hybrid or compound IDS, it is a combination and fusion of the different precedent detection techniques. This work represents a continuation of our previous ones, where we studied the attacks in MANETs [11], and an extension and improvement of [12] and [13]. In this paper, we present a deep neural network IDS (DNN IDS) for MANETs against both Distributed UDP/data and SYN flooding attacks. The presented models exhibit good results, according to the result of our experiments. The paper’s organization is: section 2 presents some related works. The description of the proposed work is presented in section 3, with definition of the context of this work, the grid search to develop an adequate DNN model, the utilized dataset, plus the selected features. Section 4 discusses the experimental results obtained. At the end, we closed this work by a conclusion.
3. WORK DESCRIPTION
3.1. Context of proposed work UDP or data flooding attack as her name defines it when the attackers nodes inject in MANETs a great volume of nugatory UDP packets, is also a type of DDoS attacks. As a result, the unnecessary packets overload the network and decrease its bandwidth. Besides, consume the battery of intermediate nodes [11]. In the previous works [22] and [12], where we used the ns 3 platform [23] to study the MANET’s reaction with AODV [24] and OLSR [25] protocols when a data flooding malicious nodes exist in network, the results showed that the network’s normalized routing load (NRL) increases and the network’s packet delivery ration PDR decreases by a significant values. Another type of DDoS and flooding attack that MANETs suffer from is SYN flooding attack, this attack works by making use of the TCP connection’s three way handshake process [11]Among the solutions to detect these types of attacks, there is the method of Knowledge based intrusion detection systems (KBIDS). The Figure 1 describe the architecture of KBIDS: the IDS save a knowledge or an internal database that contains signatures or patterns of already known threats and looks if any user’s activity matches with stored patterns/signatures, then an alarm will trigger. In knowledge based intrusion detection (KBID) mechanism, an event is proclaimed as non intrusive or acceptable, if is not formally acknowledged as a threat based on existing internal database. However, if an event that has reduced network performance is detected as an unknown attack because it does not match the saving rules, the IDS add a new rule to the existing knowledge database.
Int J Artif Intell ISSN: 2252 8938
Deep learning intrusion detection system for mobile ad hoc networks against … (Oussama Sbai) 879 thus report any illegal or malicious activity [8]. Based on the detection methodologies used, the IDS are divided into four categories [9], [10]:
This section is considerate to present a works, which they have employed deep learning approach in IDS for MANETs and they derivate like VANETs. In the paper [14], the authors propose a protection mechanism based on the artificial neural network algorithm together with the swarm based artificial bee colony optimization technique, against blackhole and grayhole attacks for MANETs using Ad hoc On demand Distance Vector (AODV) protocol. In [15], Feng et al. suggest an IDS installed in plug and play device to detect denial of service (DoS), XSS and SQL attacks for ad hoc network on using deep learning model. The author uses KDD99 dataset plus the XSS and SQL attack sample collected from waf log. In the work [16], Zeng et al. present a deep learning IDS to detect blackhole, wormhole, sybil and distributed denial of service (DDoS) attacks in VANETs. In experimental phase, they use ISCX 2012 IDS dataset [17] and simulated dataset on using ns 3 simulator [18] Sowah et al. [19] advance an artificial neural network IDS to detect the man in the middle (MITM) attack and identify the malicious nodes for MANETs using AODV protocol. The paper use dataset generated by ns 2 simulator to describe the performance of developed IDS. In the work [20], Alheeti and McDonald Maier develop an intelligent hybrid IDS by combining knowledge and anomaly detection methods for VANETs. The IDS is based on proportional overlapping scores method (POS), multilayer perceptron (MLP) and fuzzy system to detect DoS attack. The authors use the Kyoto dataset for the performance tests. In this paper [21], Vimala et al. combine neural network algorithm, support vector machine and fuzzy system in their proposed IDS for MANETs. For the test phase, the authors use the KDD99 dataset. In the anterior works [12] and [13], we proposed two IDSs for MANETs, one to detect UDP flooding attack and the other to detect SYN flooding attack, on using DNN. The CICDDoS2019 dataset is used to test the proposed IDS.
ISSN: 2252 8938 Int J Artif Intell, Vol. 11, No. 3, September 2022: 878 885 880
Table 2. Features used in the proposed DNN model
Protocol Type of the protocol used Fwd IAT Min Packets flow inter arrival time Min. Fwd IAT Max Packets flow inter arrival time Max. Packet Length Std Standard deviation of the packet length Fwd Packet Length Std Standard deviation of a packet in the forward direction
Fwd IAT Total Packets flow inter arrival total time. Flow Duration Length of connection in seconds Destination port Port receiving packets
The CICDDoS2019 Dataset has been defined in [27], has 80 network traffic features collected from principal component analysis of proteomics (PCAP) files by the CICFlowMeter software, which is freely available on the Canadian Institute for Cybersecurity website [28]. The dataset contains 12 types of DDoS attack, each attack is delivered in his specific file. In our case, we use file of UDP and SYN attack. In the precedent work [11], we studied the existed attacks that suffer from MANETs, and we find UDP and SYN flooding attack are a part of them. For the other attacks presented in this dataset are not considered for MANETs, due to use applications and the nature of all system MANETs.
Table 1. Hyper parameters configured for grid search Hyper parameter Values Number of layers 3; 4 Number of nodes 37 75 Weight initialization random_normal; he_uniform Optimization rmspop Loss function categorical_crossentropy Learning rate 0 01; 0.001; 0 0001
Figure 1. Knowledge based intrusion detection (KBID) [26]
Feature Description
ACK Flag Count Number of packets with ACK Init Win bytes forward The total number of bytes sent in initial window in the forward direction min seg size forward Minimum segment size observed in the forward direction
3.4. Statistical measures To select the best and adequate DNN model, we use accuracy, recall, F1 score, and precision as performance metrics. In the mathematical equation shown (1) (4), the true positive (TP) and the true negative (TN) define the number of samples that were correctly classified as Benign and Attack class respectively.
3.2. CICDDoS2019 dataset
3.3. Proposed methodology To insure the scalability of our proposed IDS, we use a Standalone based scheme in MANETs and nodes share detection results with their neighbors, with a privacy process [29] to secure the network transactions between them. Because we are concentrating on intrusion detection, the intricacies of these processes are outside the scope of this paper. Table 1 presents the grid search of network structure and hyper parameters used to develop an optimal neural network topology. In our proposed solution for detecting UDP and SYN flooding attacks in MANETs, we have selected 11 features to use in the proposed DNN model, where Table 2 presents their definitions. The step involved in the DNN IDS is shown in Figure 2.
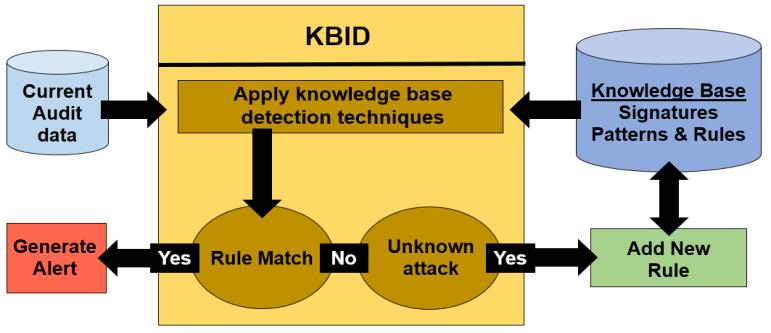
4. EXPERIMENTAL RESULTS AND DISCUSSION
Accuracy
Precision+Recall
Int J Artif Intell ISSN: 2252 8938 Deep learning intrusion detection system for mobile ad hoc networks against … (Oussama Sbai) 881
Figure
The false positive (FP) and the false negative (FN) are the number of Benign and Attack samples respectively, that have been incorrectly identified as Attack samples. = TP+TN TP+TN+FP+FN (1) = TP TP+FP (2) Recall = TP TP+FN (3) Score = 2 × Precision×Recall (4) 2. Block diagram of proposed DNN IDS
Precision
F1
Table 3. Different classifications in the training and testing sets
Class Number of training samples Number of testing samples Benign 37 947 3 526 SYN 4284751 1582289 UDP 3134645 3754680
In our experiment, we combined the different possibilities of hyper parameters values presented in Table 1, in order to obtain the best optimal results and suitable for the case of MANETs, and we constructed the training set and testing set from CICDDoS2019 dataset according to the paper. Table 3 describe in detail the training and testing sets. In Table 4, we present the different configuration of DNN architecture, who gave us the results presented in Figures 3 to 7. We remark that we executed the DNN a maximum of 4 layers and between 37 and 75 of total hidden nodes. This choice is made by taking into consideration the weak points of MANET’s nodes (power limitation, limiting memory and calculation consumption); The learning rate parameter is fixed in 0 001 value, because in the test phase other value do not give us a good result. Briefly, in this table, we present the configuration of the promoting DNN models.
The experimental results are presented in Figures 3 to 7. In terms of accuracy as shown in Figure 3, the Model 3 by 99.94% outperforms Model 5, Model 7, and Model 8 by 0.19%, 1.34% and 0.02% respectively. For the precision as shown in Figure 4, the Model 8 by 99% outperforms Model 3 by 1% and other models by 32%. Recall as shown in Figure 5 of the Model 11 by 97% outperforms Model 6 and Model 2 by 1%, Model 2 and Model 4 by 2%, Model 7 and Model 9 by 3%, Model 12 by 5%, Model 1 and Model
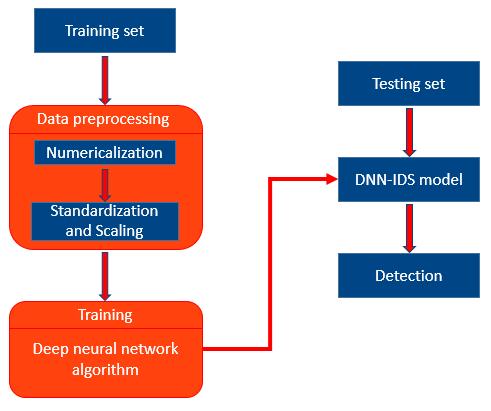
Table 4. DNN models Layers Nodes Weight initialization Learning
Table 5. Confusion matrix of Model 3 Benign SYN UDP Benign 1304 408 1814 SYN 88 3754503 89 UDP 1 553 1581735 Figure 3. Accuracy results of DNN models
ISSN: 2252 8938 Int J Artif Intell, Vol. 11, No. 3, September 2022: 878 885 88210by 7%, Model 5 by 16%, Model 3 by 17% and Model 8 by 29%. F1 score as shown in Figure 7 of the Model 3 by 84% outperforms Model 5 by 11%, Model 8 by 14%, Model 7 by 15%, Model 11 by 16%, Model 9 by 17%, Model 2, Model 4, Model 6, and Model 12 by 18%, and Model 1 by 20%. In terms of Loss as shown in Figure 6, we remark the best performance are those of Model 3, Model 5, and Model 8. The Model 8 by 1.2% outperforms Model 5 (Loss = 1.3%) by 0.1%, Model 3 (Loss = 2.6%) by 1.4%.
1 3
On analyzing the confusion matrix of the Model 3 presented in Table 5, and by making a comparison of all the parameters, we find the Model 3 (yellow row in Table 4) has the best results: with a lead of +0.19% of the Model 8 which is the most efficient of the other models in term of accuracy. A difference of 1% of the best result (Model 8) in term of precision, and with a lead of +0 11% of the Model 5 which is the most efficient of the other models in term of F1 score. For the Loss scalar, there is a difference of 1.4% of the best results offered by Model 8. Taking into consideration the use cases of the MANETs, we choose the model who has the minimum number of layers and hidden nodes, because more nodes imply power and calculation consumption. rate Model 37 random_normal 0.001 Model 2 3 39 random_normal 0.001 Model 3 3 39 he_uniform 0.001 Model 4 3 40 random_normal 0.001 Model 5 3 42 he_uniform 0.001 Model 6 3 48 he_uniform 0.001 Model 7 3 48 random_normal 0.001 Model 8 3 53 he_uniform 0.001 Model 9 3 55 he_uniform 0.001 Model 10 4 52 random_normal 0.001 Model 11 4 71 random_normal 0.001 Model 12 4 75 random_normal 0.001
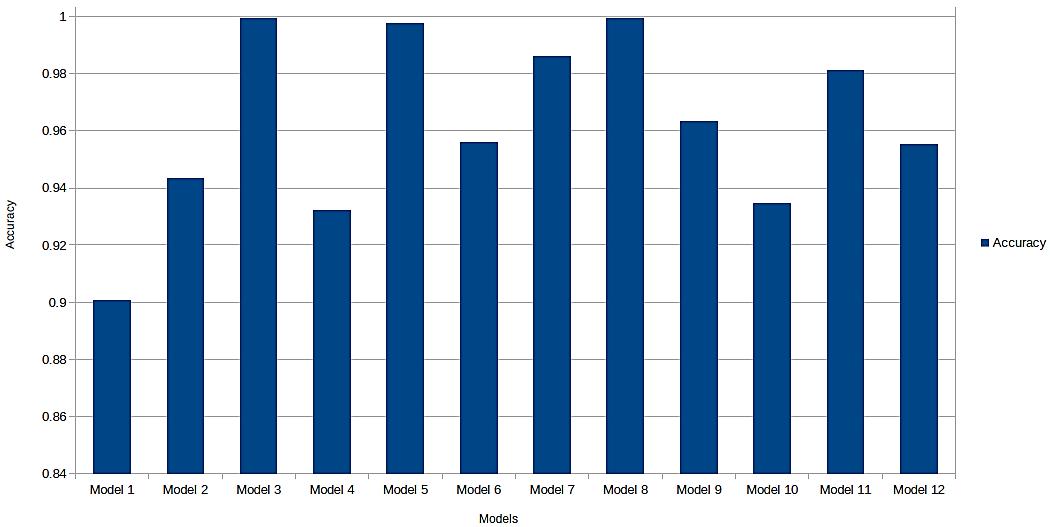
Int J Artif Intell ISSN: 2252 8938 Deep learning intrusion detection system for mobile ad hoc networks against … (Oussama Sbai) 883 Figure 4. Precision results of DNN models Figure 5. Recall results of DNN models Figure 6. Loss results of DNN models
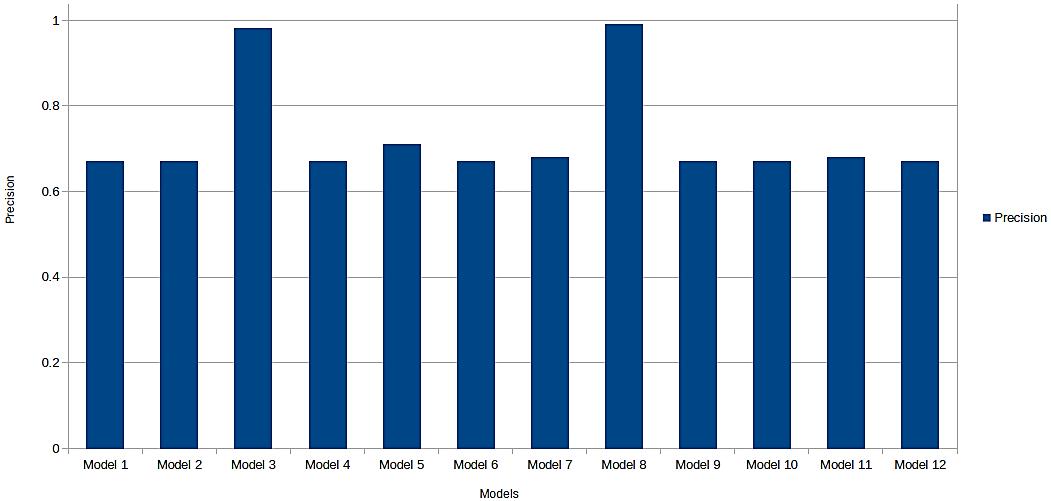
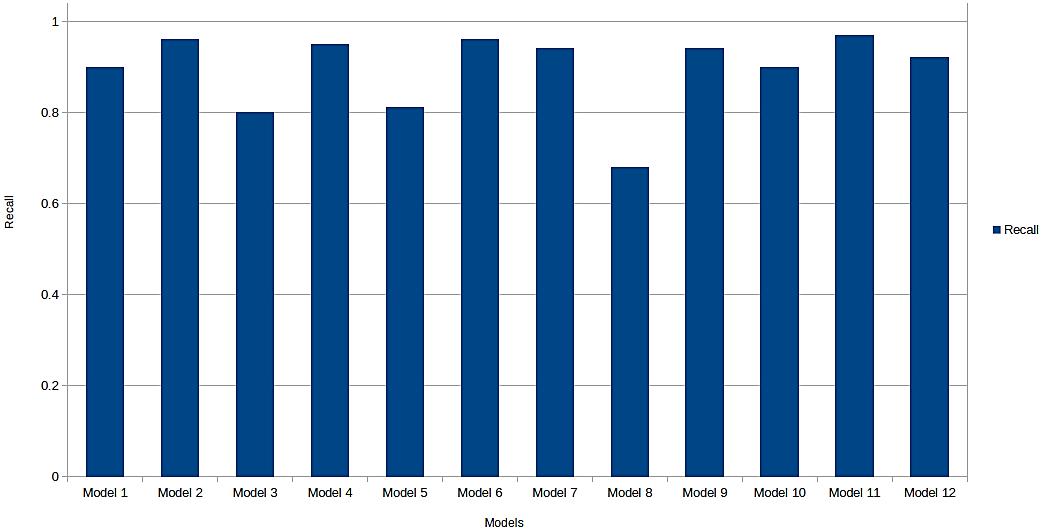
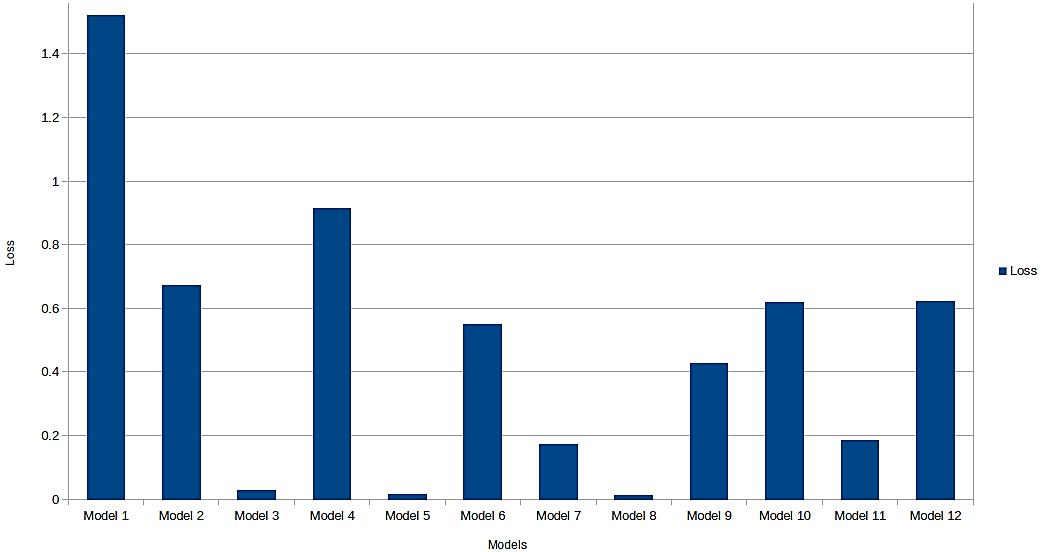
REFERENCES
[5] R. Datta and N. Marchang, “Security for mobile ad hoc networks,” in Handbook on Securing Cyber Physical Critical Infrastructure, Elsevier Inc., 2012, pp. 147 190. [6] J. Marietta and B. C. Mohan, “A review on routing in internet of things,” Wirel. Pers. Commun., vol. 111, no. 1, pp. 209 233, Mar. 2020, doi: 10.1007/s11277 019 06853 6. [7] X. Shen, R. Fantacci, and S. Chen, “Internet of vehicles [scanning the Issue],” Proc. IEEE, vol. 108, no. 2, pp. 242 245, Feb. 2020, doi: 10.1109/JPROC.2020.2964107.
[12] O. Sbai and M. Elboukhari, “Data flooding intrusion detection System for MANETs using deep learning approach,” in Proceedings of the 13th International Conference on Intelligent Systems: Theories and Applications, Sep. 2020, pp. 1 5, doi: 10.1145/3419604.3419777.
[13] O. Sbai and M. Elboukhari, “Intrusion detection system for manets using deep learning approach,” Int. J. Comput. Sci. Appl., vol. 18, no. 1, pp. 85 101, 2021.
[2] A. Lamssaggad, N. Benamar, A. S. Hafid, and M. Msahli, “A survey on the current security landscape of intelligent transportation systems,” IEEE Access, vol. 9, pp. 9180 9208, 2021, doi: 10.1109/ACCESS.2021.3050038.
[4] D. S. Lakew, U. Sa’ad, N. N. Dao, W. Na, and S. Cho, “Routing in flying Ad Hoc networks: A comprehensive survey,” IEEE Commun. Surv. Tutorials, vol. 22, no. 2, pp. 1071 1120, 2020, doi: 10.1109/COMST.2020.2982452.
[10] K. Khan, A. Mehmood, S. Khan, M. A. Khan, Z. Iqbal, and W. K. Mashwani, “A survey on intrusion detection and prevention in wireless ad hoc networks,” J. Syst. Archit., vol. 105, May 2020, doi: 10.1016/j.sysarc.2019.101701.
In this paper, we have applied DNN algorithm in KBID to detect two of important members of the several DDoS attack categories: data/UDP flooding and SYN flooding attacks in MANETs. Our model was trained and evaluated with CICDDoS2019 dataset, it is purely dedicated to DDoS attacks, with a large number of transaction network records. According to the environment of MANETs, the obtained results with DNN of maximum three deep hidden layers with 39 hidden nodes, learning rate 0 001 and he_uniform function for Weight initialization, are so promoting. As a perspective, we will continue this research by upgrading the proposed IDS to identify other attacks in MANETs using a deep learning method and find a solution to solve the problem of detection of zero day attacks.
[14] P. Rani, Kavita, S. Verma, and G. N. Nguyen, “Mitigation of black hole and gray hole attack using swarm inspired algorithm with artificial neural network,” IEEE Access, vol. 8, pp. 121755 121764, 2020, doi: 10.1109/ACCESS.2020.3004692.
[3] B. K. Tripathy, S. K. Jena, V. Reddy, S. Das, and S. K. Panda, “A novel communication framework between MANET and WSN in IoT based smart environment,” Int. J. Inf. Technol., vol. 13, no. 3, pp. 921 931, Jun. 2021, doi: 10.1007/s41870 020 00520 x.
[15] F. Feng, X. Liu, B. Yong, R. Zhou, and Q. Zhou, “Anomaly detection in ad hoc networks based on deep learning model: A plug and play device,” Ad Hoc Networks, vol. 84, pp. 82 89, Mar. 2019, doi: 10.1016/j.adhoc.2018.09.014.
Figure
[9] S. Kumar and K. Dutta, “Intrusion detection in mobile ad hoc networks: techniques, systems, and future challenges,” Secur. Commun. Networks, vol. 9, no. 14, pp. 2484 2556, Sep. 2016, doi: 10.1002/sec.1484.
[11] O. Sbai and M. Elboukhari, “Classification of mobile Ad Hoc networks attacks,” in 2018 IEEE 5th International Congress on Information Science and Technology (CiSt), Oct. 2018, vol. 2018, pp. 618 624, doi: 10.1109/CIST.2018.8596391.
[8] K. Scarfone and P. Mell, “Guide to intrusion detection and prevention systems (IDPS) (Draft),” 2012.
5. CONCLUSION
[1] G. Li, Q. Sun, L. Boukhatem, J. Wu, and J. Yang, “Intelligent vehicle to vehicle charging navigation for Mobile electric vehicles via VANET based communication,” IEEE Access, vol. 7, pp. 170888 170906, 2019, doi: 10.1109/ACCESS.2019.2955927.
ISSN: 2252 8938 Int J Artif Intell, Vol. 11, No. 3, September 2022: 878 885 884 7. F1 score results of DNN models
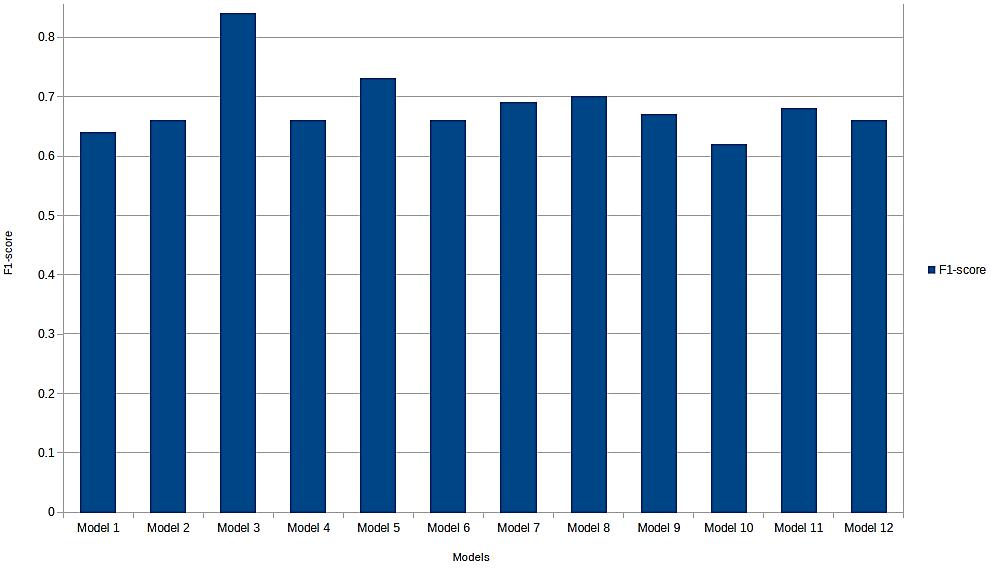
Int J Artif Intell ISSN: 2252 8938 Deep learning intrusion detection system for mobile ad hoc networks against … (Oussama Sbai) 885
[17] A. Shiravi, H. Shiravi, M. Tavallaee, and A. A. Ghorbani, “Toward developing a systematic approach to generate benchmark datasets for intrusion detection,” Comput. Secur., vol. 31, no. 3, pp. 357 374, May 2012, doi: 10.1016/j.cose.2011.12.012.
[19] R. A. Sowah, K. B. Ofori Amanfo, G. A. Mills, and K. M. Koumadi, “Detection and prevention of man in the middle spoofing attacks in MANETs using predictive techniques in artificial neural networks (ANN),” J. Comput. Networks Commun., vol. 2019, pp. 1 14, Jan. 2019, doi: 10.1155/2019/4683982.
[18] G. F. Riley and T. R. Henderson, “The ns 3 network simulator,” in Modeling and Tools for Network Simulation, Berlin: Springer Berlin Heidelberg, 2010, pp. 15 34.
[22] O. Sbai and M. Elboukhari, “A simulation analyses of MANET’s attacks against OLSR protocol with ns 3,” in Innovations in Smart Cities Applications Edition 3, M. Ben Ahmed, A. A. Boudhir, D. Santos, M. El Aroussi, and \.Ismail Rak\ip Karas, Eds. Cham: Springer International Publishing, 2020, pp. 605 618.
NS 3 Tutorial 2011 Oslo slides.pdf. [24] C. Perkins, E. Belding Royer, and S. Das, “RFC3561: Ad hoc on demand distance vector (AODV) routing,” RFC Editor, Jul. 2003. doi: 10.17487/rfc3561. [25] T. Clausen and P. Jacquet, “RFC3626: Optimized link state routing protocol (OLSR),” RFC Editor, Oct. 2003. doi: 10.17487/rfc3626. [26] A. Nadeem and M. P. Howarth, “A survey of MANET intrusion detection & prevention approaches for network layer attacks,” IEEE Commun. Surv. Tutorials, vol. 15, no. 4, pp. 2027 2045, 2013, doi: 10.1109/SURV.2013.030713.00201. [27] I. Sharafaldin, A. H. Lashkari, S. Hakak, and A. A. Ghorbani, “Developing realistic distributed denial of service (DDoS) attack dataset and taxonomy,” in 2019 International Carnahan Conference on Security Technology (ICCST), Oct. 2019, pp. 1 8, doi: 10.1109/CCST.2019.8888419. [28] A. H. Lashkari, G. D. Gil, M. S. I. Mamun, and A. A. Ghorbani, “Characterization of tor traffic using time based features,” in Proceedings of the 3rd International Conference on Information Systems Security and Privacy, 2017, vol. 2017, pp. 253 262, doi: 10.5220/0006105602530262. [29] Y. Cai, H. Zhang, and Y. Fang, “A conditional privacy protection scheme based on ring signcryption for vehicular Ad Hoc networks,” IEEE Internet Things J., vol. 8, no. 1, pp. 647 656, Jan. 2021, doi: 10.1109/JIOT.2020.3037252.
BIOGRAPHIES OF AUTHORS Oussama Sbai is a PhD candidate in computer science at Mohammed 1st University, Oujda, Morocco. His research interests include network security and network IDS using machine learning and deep learning. He can be contacted at email: o.sbai@ump.ac.ma.
[16] Y. Zeng, M. Qiu, D. Zhu, Z. Xue, J. Xiong, and M. Liu, “DeepVCM: A deep learning based intrusion detection method in VANET,” in 2019 IEEE 5th Intl Conference on Big Data Security on Cloud (BigDataSecurity), IEEE Intl Conference on High Performance and Smart Computing, (HPSC) and IEEE Intl Conference on Intelligent Data and Security (IDS), May 2019, pp. 288 293, doi: 10.1109/BigDataSecurity HPSC IDS.2019.00060.
[20] K. M. A. Alheeti and K. McDonald Maier, “Intelligent intrusion detection in external communication systems for autonomous vehicles,” Syst. Sci. Control Eng., vol. 6, no. 1, pp. 48 56, Jan. 2018, doi: 10.1080/21642583.2018.1440260.
[23] S. Kristiansen, “Ns 3 tutorial,” pp. 1 48, 2010, [Online]. Available: https://www.uio.no/studier/emner/matnat/ifi/INF5090/v11/undervisningsmateriale/INF5090
Mohamed Elboukhari received the DESA (diploma of high study) degree in numerical analysis, computer science and treatment of signal in 2005 from the faculty of Science, Mohammed 1st University, Oujda, Morocco. He is currently professor, department of Applied Engineering, ESTO, Mohammed 1st University, Oujda, Morocco. His research interests include cryptography, quantum cryptography and wireless network security, Mobile Ad Hoc Networks (MANETs). He can be contacted at email: elboukharimohamed@gmail.com.
[21] S. Vimala, V. Khanaa, and C. Nalini, “A study on supervised machine learning algorithm to improvise intrusion detection systems for mobile ad hoc networks,” Cluster Comput., vol. 22, no. S2, pp. 4065 4074, Mar. 2019, doi: 10.1007/s10586 018 2686 x.
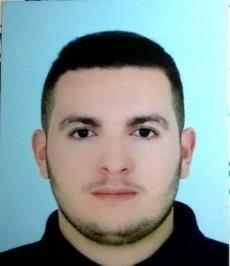



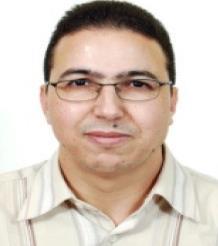



Accurate ship valuation can encourage transparency and reliability in the shipping industry. In this age driven by artificial intelligence; however, deep learning approaches have not yet taken root in ship valuation. Despite the significant achievements of deep learning algorithms in the field of unstructured data such as computer vision, the same cannot be said for the structured data dominant areas, including the shipping industry. Neural networks (NNs), the most common algorithms for implementing deep learning, are known not to have a relative advantage in handling structured data, particularly in processing categorical data. The inefficiency of NNs for processing categorical data significantly degrades their performance when categorical data occupy a significant portion of a dataset. In this study, we employed a NN to estimate second hand ship prices. Its architecture was specified using entity embedding layers to enhance the performance of the network when categorical variables were highly cardinal. Experimental results demonstrated that the information contained in categorical data can be efficiently extracted and fed into a NN using the entity embedding technique, thereby improving the prediction accuracy for ship valuation. The network architecture specified in this study can be applied in wider valuation areas where categorical data are prevalent.
Corresponding Author: Changro DepartmentLeeof Real Estate, Kangwon National University 1 Kangwondaehak gil, Chuncheon si, Gangwon do, 24341, South Korea Email: spatialstat@naver.com
This is an open access article under the CC BY SA license.
Article history: Received Sep 15, 2021 Revised Mar 8, 2022 Accepted Apr 6, 2022
IAES International Journal of Artificial Intelligence (IJ AI) Vol. 11, No. 3, September 2022, pp. 886 894 ISSN: 2252 8938, DOI: 10.11591/ijai.v11.i3 pp886 894 886
Keywords: Categorical data Entity StructuredShipNeuralembeddingnetworkvaluationdata
Article Info ABSTRACT
Journal homepage: http://ijai.iaescore.com
1. INTRODUCTION Ship prices are notorious for volatility due to peaks and valleys in the shipping industry, and there are always rent seekers attempting to gain financial returns by exploiting the unpredictability of ship prices. This situation has become more apparent during the coronavirus disease (COVID 19) pandemic. Although the ship sale and purchase market thrives on price volatility [1], it also indicates difficulties that valuation practitioners experience in estimating ship prices. Deep learning algorithms such as neural networks (NNs) have been appearing in the valuation literature since the 1990s [2], [3] to boost the predictive accuracy of asset valuation and have now started to replace the conventional models in the real estate valuation [4], [5] However, in contrast to the active application of NNs to real estate valuation, few such studies have been conducted in the domain of ship valuation. In this study, we attempt to apply a NN for estimating ship prices. First, we specify an appropriate NN architecture for application to tax returns of ship acquisition to estimate second hand ship prices. We then compare the NN’s prediction accuracy to that of a few baseline models. Finally, we interpret the result produced by NN training to provide useful insights for ship valuation stakeholders. Ship valuation plays a
Changro Lee1, Keyho Park2 1Department of Real Estate, Kangwon National University, Chuncheon, South Korea 2Department of Geography, Seoul National University, Seoul, South Korea
Deep learning-based modeling of second-hand ship prices in South Korea
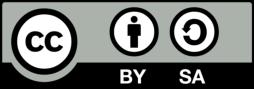
Additionally, the prices of second hand ships vary more drastically than those of new building prices depending on vessel specific characteristics such as shipbuilding materials, engine manufacturers, and age. This individual heterogeneity cannot be effectively captured by the income approach or cost approach. In contrast, the sales comparison approach can capture heterogeneity; thus, it was adopted for estimating second hand ship prices in this study.
Deep learning based modeling of second hand ship prices in South Korea (Changro Lee) 887 key role in the shipping market, such as investment decisions of shipping investors, and ship loan approvals by financial institutions. In contrast to the new building price of a ship, which is essentially a forward contract for the delivery of an age zero vessel in the future, the price of second hand ships is remarkably unpredictable, and thus more difficult to estimate to a reasonable degree, as explained above. This study aims to estimate second hand ship prices.
It is well known that deep learning has been disproportionately applied in unstructured data laden sectors such as computer vision and natural language processing industries. The use of the NN algorithm in this study is expected to significantly promote the adoption of deep learning tools in structured data dominant sectors including the shipping industry. Additionally, the empirical findings identified in the results of the NN may provide useful insights for ship valuation practitioners. This paper is constructed. Section 2 describes background information on ship valuation and the entity embedding technique. Section 3 explaines the data used and the NN architecture selected for estimating ship prices. Results and implications are interpreted in section 4. Lastly, conclusions are presented in section 5.
Structured data comprise continuous variables and categorical variables. Categorical variables assume values that are names or labels such as vessel body color and engine type, which can be represented by fixed numbers. These numbers are called levels or elements and provide no meaningful information directly. Categorical variables are observed frequently in the shipping industry. For example, ships are often classified as passenger ships, fishing boats, or cargo ships. Ship valuation agents provide price estimates by considering key factors such as ship type and the presence/absence of specific features (with or without automatic radar plotting aid, and with or without lifeboats). These valuation factors are types of categorical variables. In the ship valuation literature, most studies have paid little attention to employing categorical
Int J Artif Intell ISSN: 2252 8938
The literature on ship valuation has largely focused on the analysis of the time series properties of ship prices, such as analyzing co integrating relationships between ship prices and the time charter rate, or market trends in the new building prices and second hand prices of ships [6] [8]. Although this approach can provide an estimate of the value of a standardized generic ship based on a constructed time series of ship values, it cannot produce a price estimate for a specific ship. To provide price estimates at a vessel specific level, valuation must be performed based on micro scale cross sectional data such as individual ship sales records. A few studies have been conducted based on cross sectional data to generate ship specific price estimates [1], [9], [10]. In this line of research, one of three valuation methods, namely the income approach, sales comparison approach, and cost approach, is typically employed. The income approach estimates ship prices using discounted cash flow analysis or Monte Carlo simulation techniques based on the freight rate or time charter rate. Because the income approach is based on cash flow generated in the future, it is typically favored by financial institutions when approving loan applications for ships. In the sales comparison approach, actual transactions between buyers and sellers are collected and utilized for estimating ship prices. Because this method directly relies on market evidence, its estimates are generally accepted as convincing estimates by market participants. The cost approach estimates ship prices by subtracting depreciation from replacement costs and is widely adopted in tax assessment because it is relatively simple and easy to implement. However, estimates from the cost approach tend to deviate from the market value as a ship becomes obsolete. In an ideal market, price estimates from all three valuation approaches tend to converge. In this study, the sales comparison approach was used for ship valuation. Its estimates are based on market evidence; thus, they are considered reliable and convincing by stakeholders, as explained earlier.
2.2. Neural network (NN) with entity embedding Deep learning has achieved excellent performance in terms of utilizing unstructured data such as images, audio, video, and free form text. For example, it intensively exploits imagery data to operate self driving cars [11], [12] or detect concrete building defects [13], [14]. In contrast to the unstructured data that are commonly used in deep learning areas, the dominant data type found in the shipping industry is spreadsheet like structured data. Ship transaction records, ship inspection certificates, and safety construction certificates are specific examples of structured data observed in shipping businesses. These structured data were utilized thoroughly in this study.
2. LITERATURE REVIEW 2.1. Ship valuation approach
ISSN: 2252 8938 Int J Artif Intell, Vol. 11, No. 3, September 2022: 886 894 or have been reluctant to use them because efficient tools for processing such variables were unavailable. In [1] estimated ship prices using a multivariate density estimation method and the employed explanatory variables were deadweight tonnage, age, and the time charter rate. All these are continuous variables that are convenient to use for density estimation. In [10] estimated second hand ship prices using NNs, but their study also had limitations in that only continuous variables (age, time charter rate, new building price, and scrap value) were utilized for price estimation. One reason for using only continuous variables is that NNs are natively well suited to processing continuous data but inefficient at handling categoricalCategoricaldata. variables need to be converted to numerical representations to allow a quantitative model to process them. Several methods have been developed for this purpose. The simplest method, namely the one hot encoding approach, converts each element in a categorical variable into a new categorical column and assigns a binary value of one or zero to those columns. Although this method has been used commonly in the valuation literature [15], it has two disadvantages. First, when there are high cardinality variables such as ship types or ZIP codes, one hot encoding incurs excessive computational demand. Second, it treats different elements of categorical variables completely independently of each other and does not account for their informative interrelations.
888variables
Figure 1 presents an example of categorical data embedding methods. If there is a categorical variable, day of week, each element in it is converted into a separate binary variable under the one hot encoding approach. Figure 1(a) shows an example of this approach. The problem with it is that the informative relationship between each element is lost during conversion. In contrast, an embedding is a vector representation of a categorical variable, and the example can be represented with four numbers for each element. Figure 1(b) shows this method, and the number four is referred to as the embedding dimension. Figure 1. Example of categorical data encoding methods (a) one hot encoding and (b) entity embedding
If there is a clear ordering of the elements in a categorical variable, they can be translated into a set of numerical scores based on domain specific knowledge. For example, ship agents can rank the grade of ship maintenance on an integer scale. A ship in a poor maintenance state may be scored as five, whereas an optimally maintained ship could be given a score of one. This method can be used efficiently in processing ordinal categorical variables [16], but has the drawbacks of demanding domain knowledge in the form of expert advice or consultation. The entity embedding technique has been proposed as an alternative for extracting meaningful information from categorical variables more efficiently. Entity embedding is a technique for mapping categorical values into a multi dimensional space with fewer dimensions than the original number of levels. In this space, values with similar function outputs are close to each other [17]
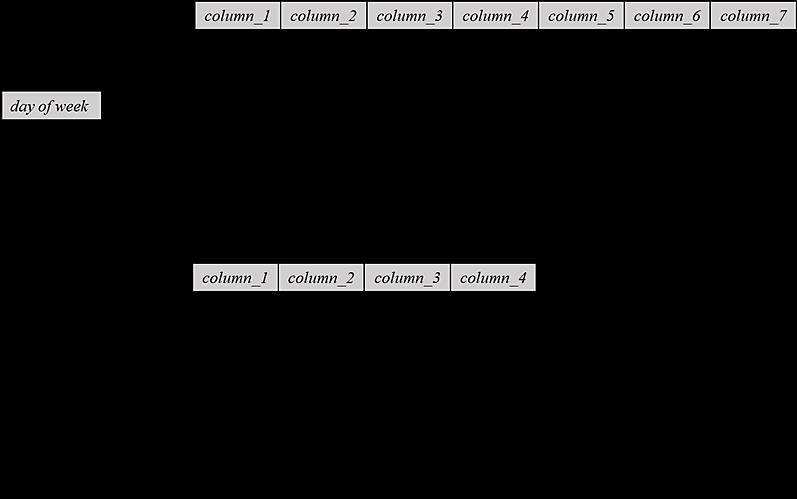
The dataset used in this study contained tax returns for property acquisitions of ships traded in 2018. The ship attributes available in the tax returns include the deadweight tonnage, age, and acquisition price, as reported by taxpayers. Table 1 presents descriptive statistics for the 3,475 ships used in our analysis
The initial dataset included over 4,000 ships and the following records were removed during data preprocessing: records with missing values, redundant records, and implausible records such as an acquisition price reported as less than 10,000,000 KRW (approximately 9,000 USD). The median acquisition price for all ships in the dataset is 38,000,000 KRW (approximately 34,000 USD). The median deadweight tonnage is 5 tons and 99.4% of the ships (3,453 of 3,475) are less than 50,000 tons. Overall, this table indicates that small and medium sized ships are typically traded and reported for the purposes of property acquisition taxes. Ships with deadweight tonnage values of up to 50,000 tons are generally referred to as “handy size” vessels and are mainly constructed in shipyards in South Korea, China, Japan, and Vietnam.
3.2. Architecture of a neural network (NN) Ship prices are affected by various factors, and selecting relevant variables involves a trade off between valuation theory and data availability. Nine inputs were employed to estimate ship prices, as shwon in Table 2. All variables excluding deadweight tonnage, age, and assessed value are categorical data. The
Int J Artif Intell ISSN: 2252 8938
The extracted features such as the embedding vectors can be reused in any subsequent models including a support vector machine, a random forest, and an NN. However, the main focus of this study is to create embedding vectors for categorical variables by using an NN and demonstrate their benefits in the context of asset valuation. Thus, an NN is adopted as the main model in this study.
In Figure 1(b), Monday and Tuesday, Wednesday through Friday, and Saturday and Sunday are similar to each other, respectively, indicating that the entity embedding matrix reasonably captures interrelations between elements within the variable, day of week. Each value in the resultant embedding matrix represents the weights connecting the input layer to the embedding layer in an NN. Therefore, the embedding matrix contains the weights of the embedding layer and can be learned in the same manner as the parameters of other NN layers [17]. Through this representation, rich information among elements in a categorical variable can be captured efficiently, and can subsequently be utilized in an NN training. Lee [18] applied this approach to real estate valuation.
3. METHOD 3.1. Dataset
Province refers to a local government having jurisdiction over the port of ship acquisition; 35.1% of the ships (1,218 of 3,475) were registered in Jeolla Province in 2018. The dominant ship type is a fishing boat (49.6%, 1,725 of 3,475).
The problems discussed next can be alleviated by using the entity embedding technique. First, excessive computational resource consumption caused by the one hot encoding of high cardinality variables can be avoided. Second, different levels of categorical variables can be handled in a meaningful manner instead of these being treated completely independently of each other. Third, domain knowledge is not required once an NN is trained to learn the relationships between values of the same categorical variable efficiently. Finally, learned embeddings can be visualized using a dimensionality reduction technique, which can provide useful insights for stakeholders.
Deep learning based modeling of second hand ship prices in South Korea (Changro Lee) 889
Several studies have estimated ship prices [9], [19] [21], and recently, NNs have begun to be utilized to enhance the predictive accuracy of real estate valuation [22] [24] and ship valuation [10] However, most studies have not employed categorical variables for valuation, and even the few studies that have used these variables have not explicitly exploited the advantages of the aforementioned entity embedding technique. In this study, we attempted to fill this research gap by employing categorical variables actively in deep learning based valuation.
Table 1. Descriptive statistics for 3,475 ships (transactions in 2018) Variable Min Mean Median Max. Acquisition price (million KRW) 10 437 38 67,886 Deadweight tonnage 0.5 984 5 172,146 Age 1 16 15 74 Province (12 levels) Jeolla: 1,218, Gyeongsang: 709, Busan: 488, Jeju: 327, Gyeonggi: 213, Incheon: 155, Chungcheong: 127, Gangwon: 105, Ulsan: 59, Daegu: 47, Gwangju: 18, Daejeon: 9 Ship type (11 levels) Fishing boat: 1,725, Small motor boat: 884, Combination carrier: 249, Barge: 158, General cargo ship: 147, Oil tanker: 104, Tugboat: 102, Passenger ship: 51, Yacht without motor: 43, Yacht with motor: 6, Special cargo ship: 6
Table 2. Input variables Data type Variable Number of levels Remarks
Categorical Province 12 Embedding dimension: 4 Ship type 11 Embedding dimension: 4 Shipbuilding material 5* One hot encoding Engine make 2 (domestic, foreign) One hot encoding Transaction type 4** One hot encoding Buyer type 2 (individual, corp.) One hot encoding Continuous Deadweight tonnage Scaling Age Scaling Assessed value Scaling *: wood, light steel, steel, fiber reinforced plastic, and others, **: sales, auction, import, and others
ISSN: 2252 8938 Int J Artif Intell, Vol. 11, No. 3, September 2022: 886 894 variables were scaled to have a mean of zero and a standard deviation of one. The target variable was the acquisition price, which was log transformed to alleviate a right skewed distribution. The 3,475 ships were split into training data (80%, 2,780 ships) and test data (20%, 695 ships) in a random manner, and the training data were further divided into two sets for ordinary training (2,224 ships) and validation purposes such as hyperparameter tuning (556 ships). The test data were reserved for the final evaluation of NN performance.
In Figure 2, EE stands for entity embedding. The number of levels in input layer 1 (Province) is 12, and its embedding dimension is four. The number of levels in input layer 2 (ship type) is 11, and its embedding dimension is four. The numbers in parentheses in dense layers 1, 2, and 3 and the output layer
890continuous
This study adopted a fully connected layer NN or dense NN. Input layers were created, and embedding layers for the two high cardinality categorical variables (province and ship type) were additionally created and joined to the architecture. A proper number of dimensions had to be defined for each embedding layer, and the prediction performance under various dimension sizes was investigated using the usual validation process. The number of dimensions assigned to each categorical variable based on this validation was four, as shown in the last column of Table 2. Three hidden layers were joined to the architecture to include more parameters to capture minor information contained in data. The final architecture of the NN is schematized in Figure 2. Figure 2. Final NN architecture
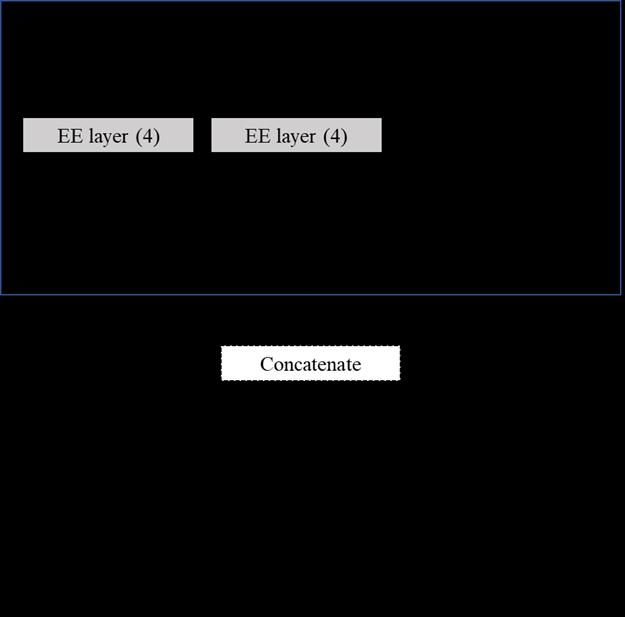
Int J Artif Intell ISSN: 2252 8938
Table 3. Comparisons of model performance Regression model NN without entity embedding NN with entity embedding MAPE 4.6 3.9 3.0
Note: EE stands for entity embedding.
Figure 3 presents the distributions of residuals from the three models in Table 3. There are no specifically notable patterns in any of the three models. One clear result is that the range of residuals was reduced remarkably in the case of the NN with entity embedding layers, indicating its excellent prediction performance.
Deep learning based modeling of second hand ship prices in South Korea (Changro Lee) 891 indicate the numbers of units (neurons) in each layer. That is, the three dense layers following concatenation contain 80, 40, and 10 neurons in order. The output layer is a linear layer with one neuron representing the ship price.The specific implementation details are: a gradient descent optimizer with momentum and Glorot initialization with a uniform distribution were used. A constant learning rate of 0.001 was adopted because the result was only trivially changed by alteration in the learning rate or learning schedule (e.g., exponential scheduling and power scheduling). A rectified linear unit (ReLU) activation function was used for all layers, except that a linear activation function was used for the output layer. The NN was trained for 30 epochs with a batch size of 64, and mean squared error was adopted as a loss function.
4. RESULTS AND DISCUSSION 4.1. Results
The mean absolute percentage error (MAPE) was used to evaluate model performance, as expressed by the following equation: �������� = 100 �� ∑ |�� �� �� |�� ��=1 (1) where �� indicates the observed price, and �� ̂ denotes the estimated price from the NN. MAPE is a measure that is frequently used by property valuation agencies [25]. MAPE indicates the prediction error as a percentage and is convenient for comparisons across different valuation models. According to [26], [27], MAPE values greater than 10.0 are typically regarded as inappropriate for loan collateral programs and a valuation model with MAPE values in excess of 20.0 should not be used in applications involving risk. The dataset of 3,475 records was randomly split into training (80%) and test (20%) datasets. Table 3 shows the MAPE results based on the test dataset. The performances of a few baseline models are presented for comparative purposes. Specifically, we consider a regression model and an NN without entity embedding layers. One hot encoding was accepted for the categorical variables in both the regression model and NN without entity embedding. As listed in the table, MAPE is generally less than 10.0, indicating that the fitted models do not present serious drawbacks. The MAPE of the NN utilizing entity embedding layers is the lowest by a meaningful margin.
Figure 3. Residual distributions for each model on the test dataset
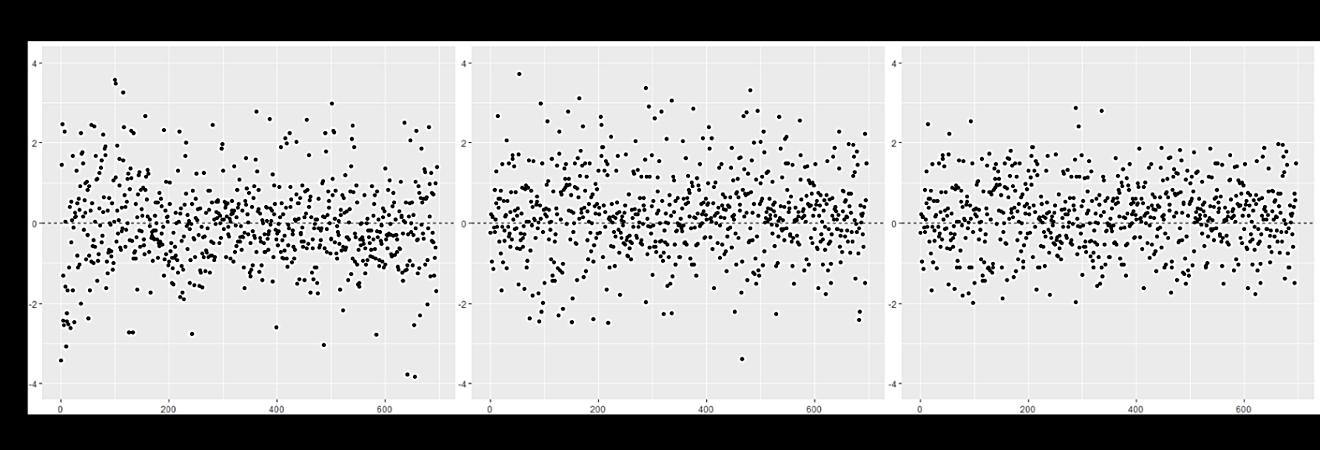
4. Province embedding mapped to a 2D space using t SNE
Although interpreting resultant embedding patterns is difficult and necessarily involves subjective judgment, it is for high cardinality variables such as the province and ship type that the entity embedding technique exhibits noteworthy performance compared to the one hot encoding approach.
The primary aim of entity embedding is to map similar elements in a categorical variable close to each other in an embedding space. Then, a relevant question arises: how does the distribution of elements in a categorical variable appear in the embedding space? To present high dimensional embeddings visually, t SNE was employed to map the embeddings to a 2D space. t SNE is a dimensionality reduction technique that produces outstanding visualizations by reducing the tendency to crowd points together in a map [28]
ISSN: 2252 8938 Int J Artif Intell, Vol. 11, No. 3, September 2022: 886 894 892 4.2. Interpreting learned embedding space
Korea.Figure
Figures 4 and 5 present the results of the province and ship type embeddings represented in a 2D space. The province variable had 12 levels and was transformed to an embedding layer with four dimensions. Figure 4 presents the learned province embedding, which is visualized in a 2D space. Three distinct clusters can be observed. One group consisting of Incheon, Chungcheong, and Gyeonggi shares the same location, that is, all three provinces face the west coast of the Korean Peninsula. Another group consisting of Ulsan, Jeolla, Jeju, and Busan also shares the same location, that is, all four provinces in the group are located in the south of the Korean Peninsula facing the south coast. The third group consisting of the remaining five provinces seems to defy a relevant interpretation. However, it is promising that some of the geographically adjacent provinces are clustered together, despite the algorithm’s ignorance of the geography in South
The ship type variable had 11 levels and was converted into an embedding layer with four dimensions. Figure 5 presents the learned ship type embedding in a 2D space. Two main groups are identifiable in the figure. Passenger ship, general cargo ship, oil tanker, and special cargo ship are close to each other. The ships in this group are relatively expensive vessels with large sizes. The other ships are clustered in an oppositional position in the figure, and the ships in the second group can be interpreted as relatively cheap and small boats. These clustering results are notable because the NN algorithm is unaware of the characteristics of each ship type.
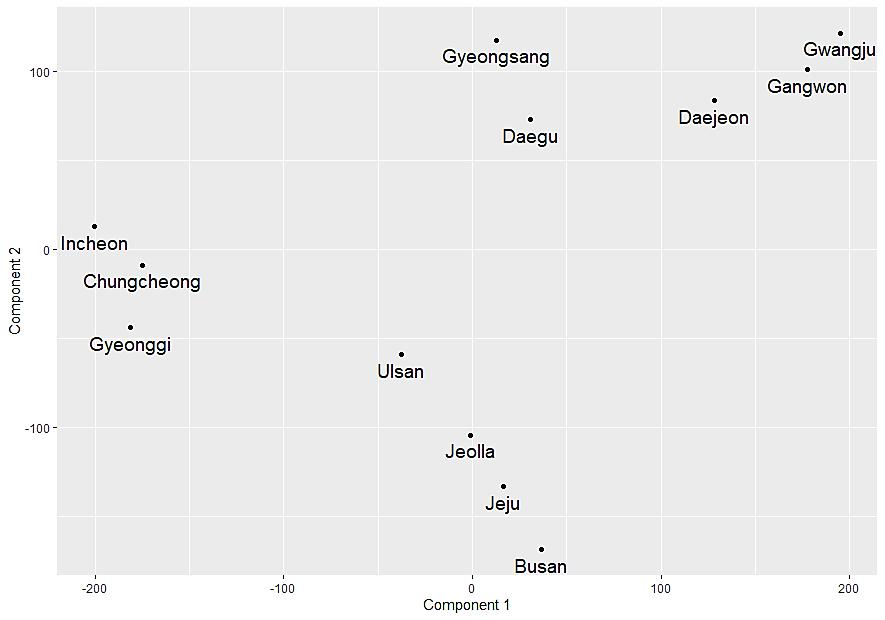
Int J Artif Intell ISSN: 2252 8938 Deep learning based modeling of second hand ship prices in South Korea (Changro Lee) 893
5. CONCLUSION Categorical variables are abundant in ship valuation, and the most common approach to handle these variables is to use one hot encoding; thus, the advantages of entity embedding have not yet been explicitly exploited. We employed the entity embedding technique in this study to improve the performance of an NN by specifying and fitting an NN with entity embedding layers to tax returns for ship acquisitions. The results demonstrated that the NN with entity embedding layers outperformed the baseline models (regression model and NN without entity embedding layers). This improvement can be attributed to the capabilities of the entity embedding layers to capture informative relationships between the elements in each categorical variable. A study limitation is that we only discussed the clustering patterns in a compressed 2D embedding space and did not interpret the values in the embedding matrices. For example, the embedding matrix for the provinces had 12 rows and 4 columns. The element values in this matrix were learned during NN training. Subsequent studies should interpret the values in embedding matrices to provide more explainable deep learning models for stakeholders. Another limitation is the small number of variables used in the valuation model. The variables frequently reported in the literature include the time charter rate, scrap value, oil price, and London Interbank Offered Rate. These variables are primarily responsible for reflecting changes in the shipping market and need to be utilized in future studies, particularly when the dataset consists of time series data.
[4] O. Poursaeed, T. Matera, and S. Belongie, “Vision based real estate price estimation,” Machine Vision and Applications, vol. 29, no. 4, pp. 667 676, May 2018, doi: 10.1007/s00138 018 0922 2.
REFERENCES
[5] S. Law, B. Paige, and C. Russell, “Take a look around,” ACM Transactions on Intelligent Systems and Technology, vol. 10, no. 5, pp. 1 19, Sep. 2019, doi: 10.1145/3342240.
[3] S. McGreal, A. Adair, D. McBurney, and D. Patterson, “Neural networks: the prediction of residential values,” Journal of Property Valuation and Investment, vol. 16, no. 1, pp. 57 70, Mar. 1998, doi: 10.1108/14635789810205128.
Figure 5. Ship type embedding mapped to a 2D space using t SNE
[1] R. Adland and S. Koekebakker, “Ship valuation using cross sectional sales data: a multivariate non parametric approach,” Maritime Economics and Logistics, vol. 9, no. 2, pp. 105 118, Jun. 2007, doi: 10.1057/palgrave.mel.9100174.
[2] M. M. Lenk, E. M. Worzala, and A. Silva, “High‐tech valuation: should artificial neural networks bypass the human valuer?,” Journal of Property Valuation and Investment, vol. 15, no. 1, pp. 8 26, Mar. 1997, doi: 10.1108/14635789710163775.
[6] S. D. Tsolakis, C. Cridland, and H. E. Haralambides, “Econometric modelling of second hand ship prices,” Maritime Economics and Logistics, vol. 5, no. 4, pp. 347 377, Dec. 2003, doi: 10.1057/palgrave.mel.9100086.
[7] R. Adland, H. Jia, and S. Strandenes, “Asset bubbles in shipping? an analysis of recent history in the drybulk market,” Maritime Economics and Logistics, vol. 8, no. 3, pp. 223 233, Sep. 2006, doi: 10.1057/palgrave.mel.9100162.
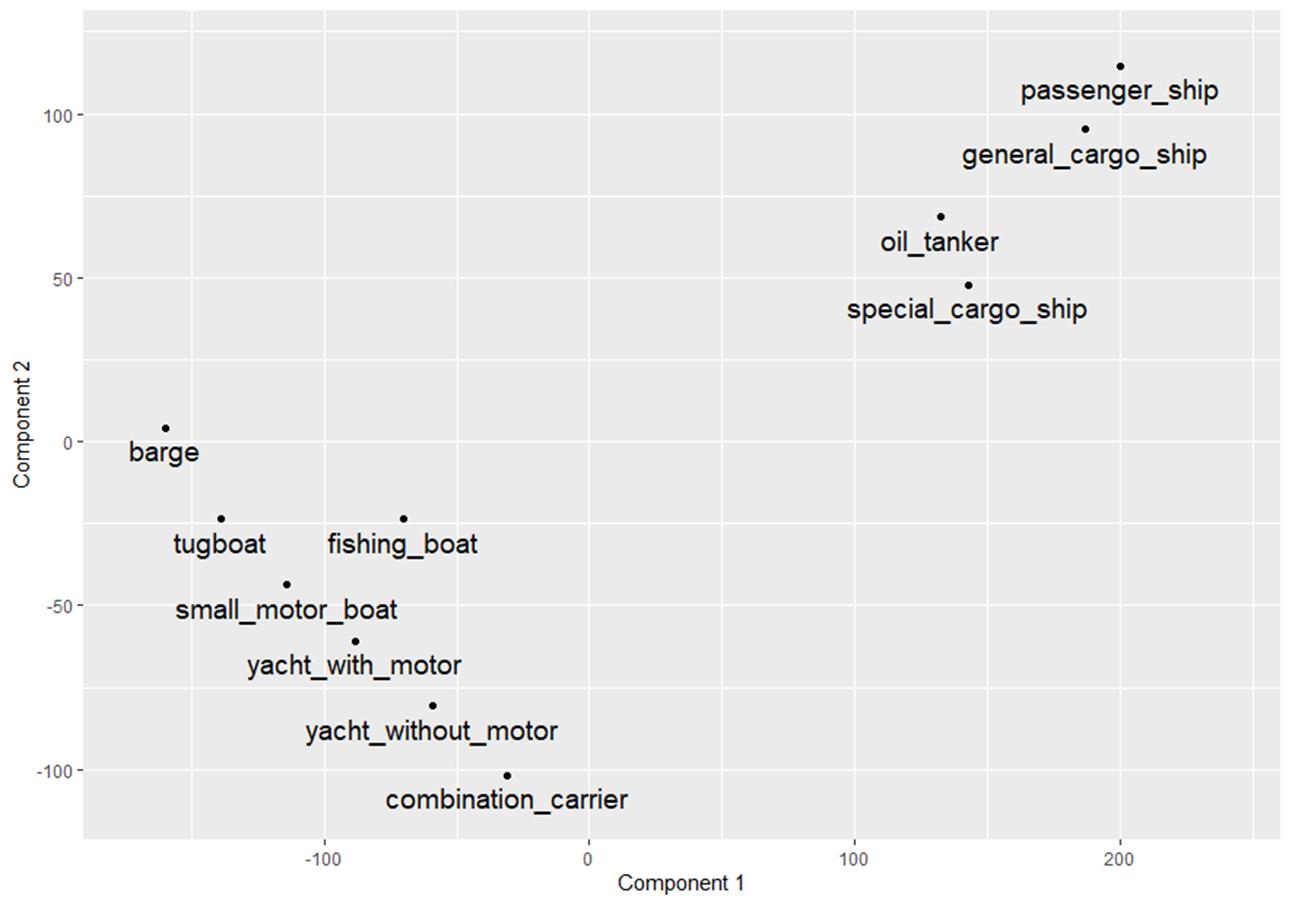
[14] H. Perez, J. H. M. Tah, and A. Mosavi, “Deep learning for detecting building defects using convolutional neural networks,” Sensors, vol. 19, no. 16, Art. no. 3556, Aug. 2019, doi: 10.3390/s19163556.
BIOGRAPHIES OF AUTHORS
[13] M. Alipour, D. K. Harris, and G. R. Miller, “Robust pixel level crack detection using deep fully convolutional neural networks,” Journal of Computing in Civil Engineering, vol. 33, no. 6, Art. no. 04019040, Nov. 2019, doi: 10.1061/(ASCE)CP.1943 5487.0000854.
[22] O. Al Gbury and S. Kurnaz, “Real estate price range prediction using artificial neural network and grey wolf optimizer,” in 2020 4th International Symposium on Multidisciplinary Studies and Innovative Technologies (ISMSIT), Oct. 2020, pp. 1 5, doi: 10.1109/ISMSIT50672.2020.9254972.
[9] J. S. Choi, K. H. Lee, and J. S. Nam, “A ship valuation model based on Monte Carlo simulation,” Journal of Korea Port Economic Association, vol. 31, no. 3, pp. 1 14, 2015.
Keyho Park holds a Bachelor of Engineering (B.Eng.) in Architecture, Master of Urban Planning (MUP), and Ph.D in Computer Science. He has been a professor since 1995 at Department of Geography, Seoul National University (SNU), South Korea. His teaching and research areas of interest include geographic information science, spatio temporal statistics and machine learing. He can be contacted at email: khp@snu.ac.kr.
[18] C. Lee, “Enhancing the performance of a neural network with entity embeddings: an application to real estate valuation,” Journal of Housing and the Built Environment, Aug. 2021, doi: 10.1007/s10901 021 09885 2. [19] A. Miroyannis, “Estimation of ship construction costs,” Massachusetts Institute of Technology, 2006.
[21] L. Dai, H. Hu, F. Chen, and J. Zheng, “The dynamics between newbuilding ship price volatility and freight volatility in dry bulk shipping market,” International Journal of Shipping and Transport Logistics, vol. 7, no. 4, pp. 393 406, 2015, doi: 10.1504/IJSTL.2015.069666.
[25] M. Ecker, H. Isakson, and L. Kennedy, “An exposition of AVM performance metrics,” Journal of Real Estate Practice and Education, vol. 22, no. 1, pp. 22 39, Jan. 2020, doi: 10.1080/15214842.2020.1757352.
ISSN: 2252 8938 Int J Artif Intell, Vol. 11, No. 3, September 2022: 886 894 894 [8] L. Fan and J. Yin, “Analysis of structural changes in container shipping,” Maritime Economics and Logistics, vol. 18, no. 2, pp. 174 191, Jun. 2016, doi: 10.1057/mel.2014.38.
[24] L. N. Yasnitsky, V. L. Yasnitsky, and A. O. Alekseev, “The complex neural network model for mass appraisal and scenario forecasting of the urban real estate market value that adapts itself to space and time,” Complexity, vol. 2021, pp. 1 17, Mar. 2021, doi: 10.1155/2021/5392170.
[27] H. R. Isakson, M. D. Ecker, and L. Kennedy, “Principles for calculating AVM performance metrics,” The Valuation Journal, National Association of Romanian Valuers, vol. 16, no. 2, pp. 38 69, 2020.
Changro Lee is a professor at the Department of Real Estate, Kangwon National University (KNU), South Korea. Before joining KNU, Lee was a researcher at Korea Institute of Local Finance (KILF). He has worked in the fields of real estate management, machine learning, and local financing of real estate. He can be contacted at email: spatialstat@naver.com.
[12] H. Song, “The application of computer vision in responding to the emergencies of autonomous driving,” in 2020 International Conference on Computer Vision, Image and Deep Learning (CVIDL), Jul. 2020, pp. 1 5, doi: 10.1109/CVIDL51233.2020.00008.
[10] D. Kim and J. S. Choi, “Development of ship valuation model by neural network,” Journal of the Korean Society of Marine Environment and Safety, vol. 27, no. 1, pp. 13 21, Feb. 2021, doi: 10.7837/kosomes.2021.27.1.013.
[11] M. T. Duong, T. D. Do, and M. H. Le, “Navigating self driving vehicles using convolutional neural network,” in 2018 4th International Conference on Green Technology and Sustainable Development (GTSD), Nov. 2018, pp. 607 610, doi: 10.1109/GTSD.2018.8595533.
[28] L. van der Maaten and G. Hinton, “Visualizing data using t SNE,” Journal of Machine Learning Research, vol. 9, pp. 2579 2605, 2008.
[26] P. Rossini and P. J. Kershaw, “Automated valuation model accuracy: Some empirical testing.” 2008.
[20] T. Syriopoulos and E. Roumpis, “Price and volume dynamics in second hand dry bulk and tanker shipping markets,” Maritime Policy and Management, vol. 33, no. 5, pp. 497 518, Dec. 2006, doi: 10.1080/03088830601020729.
[17] C. Guo and F. Berkhahn, “Entity embeddings of categorical variables,” Apr. 2016. Available: http://arxiv.org/abs/1604.06737.
[16] A. Agresti, Analysis of ordinal categorical data. Hoboken, NJ, USA: John Wiley and Sons, Inc., 2010.
[23] H. Seya and D. Shiroi, “A comparison of residential apartment rent price predictions using a large data set: kriging versus deep neural network,” Geographical Analysis, Mar. 2021, doi: 10.1111/gean.12283.
[15] R. Gloudemans and R. Almy, Fundamentals of mass appraisal. International Association of Assessing Officers, 2011.
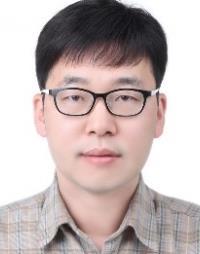



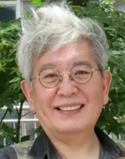



Journal homepage: http://ijai.iaescore.com
1. INTRODUCTION Almost 197 million people in Indonesia have used the internet in their daily life. This figure is directly proportional to the increase in social media users in Indonesia [1]. Indonesian society uses social media to publish their views and opinions under various circumstances [2]. One of them is through the social media used by most users to express their attitudes, thoughts or opinions on various occasions [3], [4]. A social media platform and social networking service that is widely used and generates a large amount of information are Twitter [5].
Article Info ABSTRACT
Article history: Received Oct 2, 2021 Revised Apr 4, 2022 Accepted Apr 28, 2022
Tiara Intana Sari1 , Zalfa Natania Ardilla1, Nur Hayatin1, Ruhaila Maskat2 1Department of Informatics, Faculty of Engineering, University of Muhammadiyah Malang, Malang, Indonesia 2Faculty of Computer and Mathematical Sciences, Universiti Teknologi MARA Shah Alam, Selangor, Malaysia
Half of the entire social media users in Indonesia has experienced cyberbullying. Cyberbullying is one of the treatments received as an attack with abusive words. An abusive word is a word or phrase that contained harassment and is expressed be it spoken or in the form of text. This is a serious problem that must be controlled because the act has an impact on the victim's psychology and causes trauma resulting in depression. This study proposed to identify abusive comments from social media in Indonesian language using a deep learning approach. The architecture used is a hybrid model, a combination between recurrent neural network (RNN) and long short term memory (LSTM). RNN can map the input sequences to fixed size vectors on hidden vector components and LSTM implemented to overcome gradient vector growth components that have the potential to exist in RNN. The steps carried out include preprocessing, modelling, implementation, and evaluation. The dataset used is indonesian abusive and hate speech from Twitter data. The evaluation result showed that the model proposed produced an f measure value of 94% with an increase in accuracy of 23%.
IAES International Journal of Artificial Intelligence (IJ AI) Vol. 11, No. 3, September 2022, pp. 895 904 ISSN: 2252 8938, DOI: 10.11591/ijai.v11.i3 pp895 904 895
Abusive comment identification on Indonesian social media data using hybrid deep learning
This is an open access article under the CC BY SA license. Corresponding Author: Nur DepartmentHayatinof Informatics, Faculty of Engineering, University of Muhammadiyah Malang St. Raya Tlogomas 246 Malang, Indonesia Email: noorhayatin@umm.ac.id
Keywords: Abusive comments Deep learning Long short term memory Recurrent neural network Sentiment analysis
Social media has benefits for communication, marketing, and community education. On the other hand, it can cause offensive outcomes such as hate speech spreading and online harassment, as known by many with the term cyberbullying. The case of cyberbullying has become a point of criticism as well as pressure on popular social media platforms, such as Facebook and Twitter, being a problem that must be solved [6]. There are still many social media users who communicate with abusive and uncontrollable words [7]. Abusive word is one of the types of cyberbullying expressed or spoken using either orally or in text. This treatment can be an act on social media through comments. It can be a word or phrase that are rude or dirty, in the context of jokes, sex harassment, or cursing someone [8]
Komisi Perlindungan Anak Indonesia (The Indonesian Child Protection Commission) stated that there were 37381 complaints regarding bullying cases from 2011 to 2019 where there is a total of 2473 data
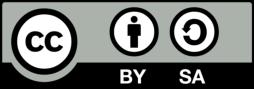
Abusive speech is an expression that is spoken either orally or in text and contains words or phrases that are rude or dirty, either in the context of jokes, a conversation of vulgar sex or of cursing someone [8] Referring to sociolinguistic studies [19], Indonesian abusive word can be affected from daily conversation such as: i) describe an unpleasant situation or condition, e.g.: “gila” (in English: crazy), “bodoh” (in English: stupid), “najis” (in English: excrement), and “celaka” (in English: accurst); ii) compare animals characteristics with individual, e.g.: “anjing” (in English: dog), and “babi” (in English: pig); iii) abusive word that connect about astral being, e.g.: “setan” (in English: satan), and “iblis” (in English: devil); iv) depends on bad reference of that object, e.g.: “tai” (in English: shit), and “gombel” (in English: crap); v) body part that usually related with sex activity or another body part, e.g. “matamu” (in English: your eyes) cause someone make a mistake with their eyes; vi) express of displeasure or annoyed that related with family member usually add with suffix mu, e.g.: “bapakmu” (in English: your father), “kakekmu” (in English: your grandpa), “mbahmu”) (in English: your grandma); and vii) related with profession using phrase about low profession and forbidden by religion, e.g.: “maling” (in English: thief), “babu” (in English: maid), “lonte” (in English: bitch).
Another research implemented a combined architecture of deep learning, i.e.: recurrent neural network (RNN) and LSTM for faults classification. The research succeeds to classify multi label faults quite well even without preprocessing [16] Du et al. also prove that the hybrid architecture of RNN and LSTM is able to classify claritin october twitter dataset with an accuracy that reached approximate 97% [17]. These studies’ results prove that using a hybrid deep learning method to handle classification tasks presents better accuracy than a single deep learning approach. From the problem about the abusive comments issue on social media that was mentioned above, we need to identify abusive comments automatically by adopting the model from previous research to produce a good performance in the classification model. This study aims to identify abusive comments by utilizing the hybrid deep learning approach for Indonesian social media data. The architecture used is a combination between RNN and LSTM. Both are complementary, the RNN algorithm is used to map the input sequence to a fixed size vector to the hidden vector component which is used to summarize all the information in the previous process, then the LSTM algorithm is implemented to help overcome the gradient vector growth components that have the potential to exist in the RNN algorithm [18]. So that it can increase the performance of the abusive comment identification model.
2. STUDY OF LITERATURE 2.1. Sociolinguistic study
Detection of abuse in user generated online content on social media is a difficult but important task [11], [12]. However, identifying abusive comments need a substantial effort if done manually. Machine learning (ML) can be used to classify comments that contained abusive words automatically. Ibrohim and Budi have studied the problem of abusive comments on Indonesian tweets. They compared several methods in machine learning such as random forest decision tree (RFDT), support vector machine (SVM), and naïve Bayes (NB).
ISSN: 2252 8938 Int J Artif Intell, Vol. 11, No. 3, September 2022: 895 904 896related to cyberbullying on social media [9]. Other data, published by the association of Indonesia internet service providers in 2019, shows that cyberbullying cases in Indonesia have reached approximately 49% [10]. These data are proof that cyberbullying is worrying and has to be controlled properly, including utilized abusive words on social media. It impacted a victim’s psychology and caused trauma. Other than that, there is a tendency for the victim to experience anxiety, individualism or even antisocial behaviour, resulting in depression due to prolonged cyberbullying [1] Nowadays, the utilization of abusive words is uncontrollable in social media particularly [8]
The result shows that NB produced higher accuracy than other techniques. However, it does not provide a good enough performance with accuracy is around 70.06% [13]. Deep learning (DL) is a new approach that has a good performance for a classification task which was proven from recent research. The study proposed by Chakraborty and Seddiqui has compared ML and DL approaches. They used machine learning to detect abusive context on social media in the Bengali language. This research implements SVM and multinominal naïve Bayes (MNB) as a method to detection abusive language. They also implement a deep learning method that is using a combined model convolutional neural network (CNN) with long short term memory (LSTM). From all models that were used, the best result was achieved by the SVM method with 78% accuracy [14]. A similar approach was used in [15] to detect an abusive context on Urdu and Roman Urdu social media. Five models of machine learning have been proposed in this research which is NB, SVM, IBK, logistic and JRip. Other than that, four deep learning methods were also proposed for this task which is CNN, LSTM, bidirectional long short term memory (BLSTM), and CLSTM. The result shows that CNN has a better result than other methods that have been used with an accuracy of 96.2% in Urdu and 91.4% in Roman Urdu.
Nevertheless, the result from those studies is not optimal enough to recognize hate speech from Twitter comments in Indonesia language with an average of accuracy under 80%. Table 1 shows existing research on Indonesian abusive comment classification using various machine learning techniques.
Prabowo et.al [20] proposed a classification process to recognize Indonesian abusive comments and hate speech on Twitter by implementing SVM. From the result, it was discovered that SVM with the help of the word unigram feature yields quite good results compared to other methods. However, the accuracy of the resulting system is still low around 68.43%.
Table 1. Existing research on Indonesian abusive comment classification Writers (Year) Contribution Method Result Ibrohim and Budi (2018) [8] Classification of abusive in Indonesia language Naïve Bayes The accuracy of the resulting system is around 86.43%
Int J Artif Intell ISSN: 2252 8938
3. RESEARCH METHOD
The first step conducted is text preprocessing, then proceed with separating the dataset into train and test. Furthermore, the next step is modelling using the proposed hybrid deep learning architecture then validating the model before going to the implementation stage. Finally, the evaluation of the model is conducted to test the model using a confusion matrix table. 3.1. Dataset The dataset that is used in this research is the Indonesian Abusive and Hate Speech from Kaggle with an amount of 13169 tweets. This research utilizes the target data in the Abusive column. The data is divided into two classes: abusive and non abusive. The data distribution is as shown in Figure 2(a). There is an issue with the data related to imbalances. Therefore, the oversampling technique is necessary to overcome that problem. This is needed because the amount of data that is labelled before oversampling differs by almost 50%. This warrants for the oversampling technique to be conducted to increase abusive labelled data. Additionally, it is expected to improve system performance. The distribution of the data after the oversampling technique is carried out as shown in Figure 2(b). 3.2. Preprocessing Preprocessing text involves case folding, filtering, stemming, tokenizing, sequencing and padding. The preprocessing phase started with lower case conversion and punctuation removal, specifically case
Other researches were conducted to detect an abusive comment in Indonesian tweets using various machine learning techniques such as NB [8], binary relevance [13], and logistic regression [21]
Prabowo et al. (2019) [20] Abusive comments and hate speech multi classification on Twitter with Indonesia language SupportMachineVector However, the accuracy of the resulting system is not good enough around 68.43%.
To the best of our knowledge, research on Indonesian abusive comment classification using a deep learning approach is still limited and need to be explored. Neural network (NN) is part of machine learning that adapts hidden layer structures or implicit data patterns and is flexible for use in supervised, semi supervised, or unsupervised learning [22] NN has now been transformed into deep learning with excellent performance and can be implemented in various fields, including RNN and LSTM. Systematically, ANN is in the form of a graph with neurons or nodes (vertex) and synapses (edge) making it easier to explain operations on ANN in the form of linear algebraic notation. [22]. The concept of RNN is to create a network topology that can represent sequential or time series data [22]. The main key of RNN is memorization [23] LSTM is part of the RNN architecture which is specifically designed to model temporal sequences and their remote dependencies more accurately than conventional RNNs [24]. RNN functions to map input sequences to fixed size vectors and LSTM is implemented to overcome gradient vector growth components that have the potential to exist in the RNN algorithm [18].
2.2. Related works
Abusive comment identification on indonesian social media data using hybrid deep learning (Tiara I Sari) 897
Ibrohim and Budi (2019) [13] Multi classification of abusive and hate speech in Indonesia language Binary Relevance The accuracy of the system is around 73.53% Ibrohim et al. (2019) [21] Hate Speech and Abusive Language on Indonesian Twitter RegressionLogistic The result of the system is 79.85%
This research used a classification task to identify abusive comments on Indonesian social media data. The deep learning approach is chosen with RNN and LSTM architectures referred to as Hybrid deep learning. The pipeline of the proposed model for abusive comments identification is shown in Figure 1.
898folding.
[25]Figure
ISSN: 2252 8938 Int J Artif Intell, Vol. 11, No. 3, September 2022: 895 904
1. The pipeline of abusive comments identification using hybrid deep learning
Figure 2. Data balancing visualization (a) before and (b) after oversampling
(b)(a)
The next step is stop word removing which is part of the filtering process. Afterwards, searching of the root word, namely stemming was conducted. In this research, we used Sastrawi stemmer. The last step is Tokenization which is the process of converting sentences into phrases, words or tokens that the system can understand
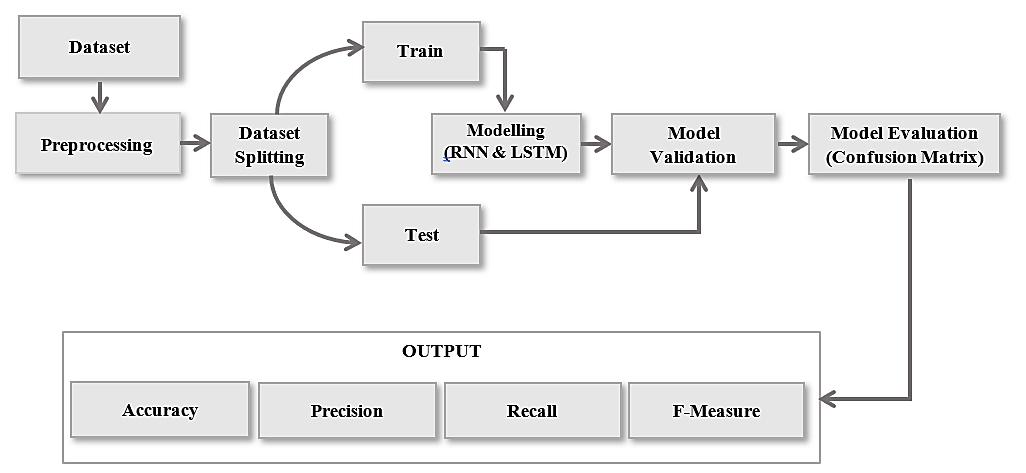
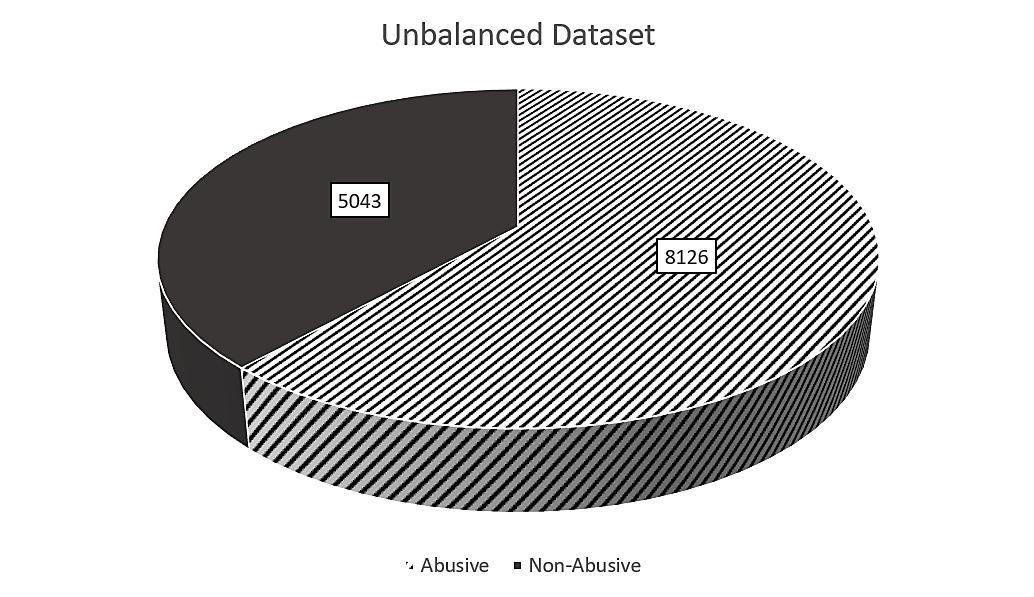
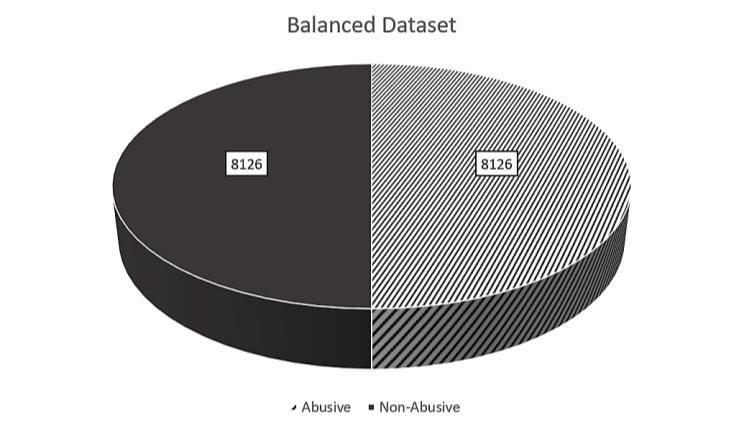
RNN concept can be described as a formula, as in (1). In that equation ���� is hidden state from input of time t and ℎ�� 1 is hidden state in previous time. Whereas �� is the activation function (non linear, can be derived) [22]. The �� function can be replaced with LSTM. ht =��(����,ℎ�� 1,��) (1) it = σ(Wixt +Uiht 1 +bi) (2)
3.4. RNN LSTM
In general, the RNN concept can be visualized in Figure 3 that accordance with the recurrent principle of remembering (memorizing) previous events. Meanwhile, LSTM is an architecture of RNN which was more accurate than the conventional RNN. LSTM is known to improve deficiencies found in conventional RNN. In the LSTM feature, there are several stages [26]
3.3. Data splitting
The detail of LSTM architecture is shown in Figure 4. Figure 4 depicts that LSTM has 3 gates, i.e. forgot gate, input gate, and output gate, which computation process for LSTM [27] is started with stored input value into the cell state when input gate allowed the process. In (1) and (2) show the calculation of the value input gate and possible value from the cell state.
Figure 4. Architecture of LSTM
This process involves dividing the data into two sets for training and testing. The data is divided by the proportion of 80% data for the training set and 20% data for the testing set, with the total number of train data being 6501 for non abusive and 6500 for abusive and the number of test data being 1626 for non abusive and 1625 for abusive.
Figure 3 Concept of RNN
Int J Artif Intell ISSN: 2252 8938 Abusive comment identification on indonesian social media data using hybrid deep learning (Tiara I Sari) 899
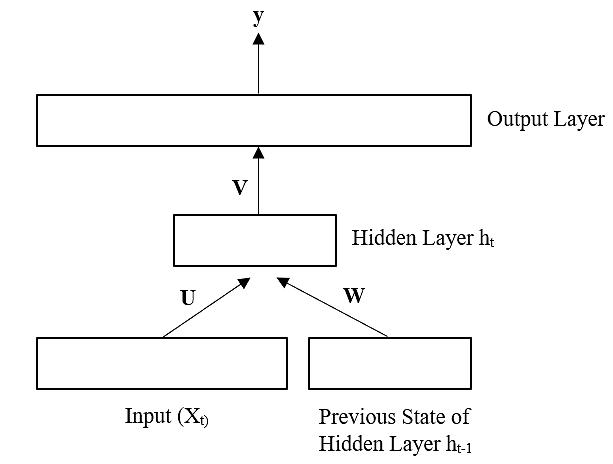
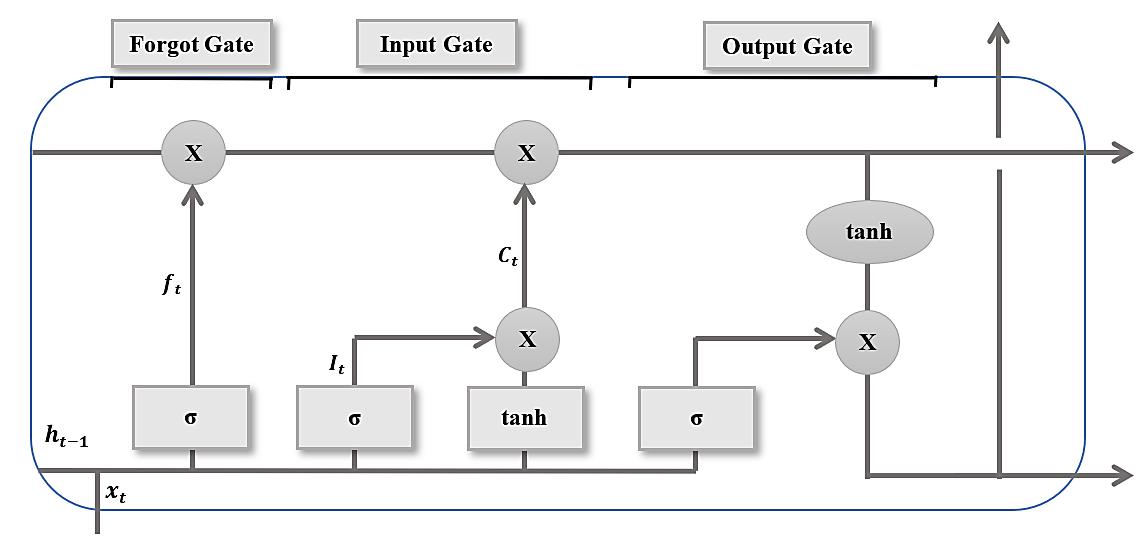
The resulting dimensions are batch size, sequence, and embedding size. Dimension of embedding sets the number of features for the word i.e., the number of hidden units. The embedding result is in the form of a matrix with dictionary length and embedding dimensions. Then, added a RNN Layer with SimpleRNN from tensorflow module. The next step is to enter a LSTM memory block, the LSTM contain a special unit called a memory block in the recurrent hidden layer that detail explained in Figures 3 and 4. Then, added layer dense to create a complexity NN layer and dropout to handle overfitting problems [28]. The last step is data output which is represented with a binary value (0 and 1). These values are the probability, 0 is for non abusive data and 1 for abusive data. This label is used to classify abusive sentences.
3.5. Model validation
The model that has been built should be validated with data validation that is entered in model.fit() function with x variable is an input data which contain train_sequence while y variable is a target data. Both variables can be assigned with Numpy array or Tensorflow tensor while y variable filled with train_labels
The next parameter is batch_size, a number of samples per gradient update and that contain integer on none, we use 32 as a default of batch_size. For Epoch, a number of iterations in train model, we use 10 epochs to train the model. Meanwhile, Callback using [rlrp] is applied during training and last verbose using integer 1 that means progress bar in tensorflow documentation. And after that step, the system will be showing the
ℎ�� = ���� ∗������ℎ(����) (7)
ISSN: 2252 8938 Int J Artif Intell, Vol. 11, No. 3, September 2022: 895 904 900 ���� in (2) represent value input gate, ���� represent of weight value of ��, xt show value of the input in �� condition, Ui represent of weight value of output at ��. Then, ht 1in (1) present value of output in �� 1 condition, ���� the present bias of input gate, and σ call with sigmoid function Ct = tanh(Wcxt +Uihc 1 + bc) (3)
Ct the present possible value of cell state, Wc represent of weight value of the input in �� condition, xt show value of the input in �� condition, Ui represent of weight value of output at ��. Then, hc 1 in (3) present value of output in �� 1 condition, bc the present bias of ��, and tanh can call as tangent function. Therefore, in (4) present process of forgot gate ���� = ��(�������� +����ℎ�� 1 +����) (4)
Value of cell state present with Ct, the value of input gate value shown at it, Ct present possible of cell state, the value of forget gate present in ft and value of previous cell state show with Ct 1. The next process after generating new memory of cell state has been done, the process of output gate started in (6).
���� = ��(�������� +����ℎ�� 1 +����) (6)
The value that represents of output gate is ot, Wo represent of weight value of the input in �� condition, xt show value of the input in �� condition, Uo show value of the input in �� condition, ht 1 in (6) present value of output in �� 1 condition, output gate bias present with bo, and sigmoid function represents at σ symbol. The final output process shows in (7).
The final output represented with ht and output gate represent in ot value, Ct present of new memory cell state value and tanh called as tangent function.
Value of forget gate present with ft, Wf represent of weight value of the input in �� condition, xt show value of the input in �� condition, Uf represent of weight value of output at ��, ℎ�� 1 in (4) present value of output in �� 1 condition, the bias of forget gate represent in ����, and σ called as sigmoid function. Therefore, in (5) present process of cell state ���� = ���� × ���� + ���� × ���� 1 (5)
3.4. RNN-LSTM implementation In the modelling process, the combination of RNN and LSTM is used to develop the optimal model.
The train data is entered into the RNN and LSTM classification models as a data input that has been created in Figure 5. The input layer is defined as an embedding layer, where in this layer there is a vocabulary retrieval process that is coded with an array of integers and embedding vectors for each word index.
This section shows the results obtained during the training, validation, testing process and presents the important findings obtained from these results. One of which is the effect of using the RNN LSTM hybrid deep learning model and the impact of balanced data. The distribution of the previous data shows that the data used is unbalanced by using the random oversampling technique. This technique takes some random data from the abuse category with an adjusted amount based on the largest number of classes that will be used as data into balanced proportion as in Table 2. The data after oversampling and the source code about the research that distributed on github [30] The use of oversampling can provide a fairly good increase in system performance. The use of balanced data greatly affects the system in classifying a text, the results of the comparison between the use of oversampling and data that did not go through the oversampling process. The results obtained provide an
Int J Artif Intell ISSN: 2252 8938 Abusive comment identification on indonesian social media data using hybrid deep learning (Tiara I Sari) 901 accuracy of the training model. Finally, the modelling is done and produced the values of the training process, e.i.: loss and accuracy of each epoch.
Figure 5. The architecture of RNN and LSTM 3.6. Model evaluation
The evaluation measurement is used to test the reliability of the model to get accuracy, precision, recall and f measure values based on the confusion matrix table. The confusion matrix summarizes the classification performance of the system in actual and predicted form through the entered test data [29]. From the confusion matrix table, the accuracy, precision, recall and f measure score can be calculated using (8) (11). It means when the system predicts a word as an abusive class and actually declared as abusive then the statement is represented as true positive (TP). If the system predicts a word as a non abusive class but actually in the dataset the sentence is stated rudely then the statement is represented with false negative (FN).
Accuracy = ����+���� (����+����+����+����) (8) ������������������ = ���� ����+���� (9) ������������ = ���� ����+���� (10) ���������������� = 2×(������������×������������������) ������������+������������������ (11)
Meanwhile, the system predicts a word as an abusive class but actually declared as a non abusive then the statement is represented as false positive (FP). However, when the system predicts a word as a non abusive class and actually declared as a non abusive then the statement is represented as true negative (TN).
4. RESULTS AND DISCUSSION
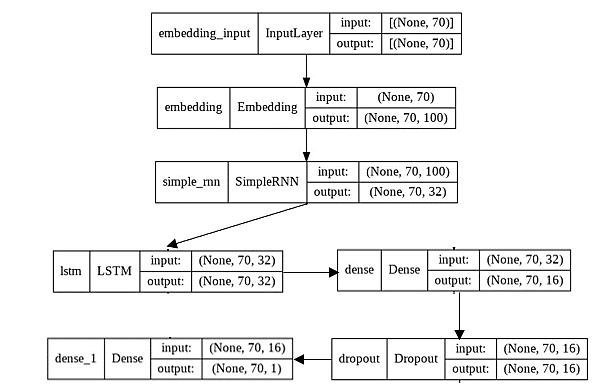
Table 5. Test Model with Other Data Test Sentences Probability najis ih (dirty) 93.38% wkwkwk jijik euh (mucky) 04.92% dia memang kurang berpendidikan (less educated) 86.71% otaknya ngga dipakai (the brain is not used) 13.62%
ISSN: 2252 8938 Int J Artif Intell, Vol. 11, No. 3, September 2022: 895 904 902increase of 6% to 8% for precision, recall, and f1 score as shown in Table 3. Meanwhile, The use of RNN LSTM also provides an increase in the use of several machine learning methods in previous studies [8].
Table 3. Comparation of oversampling and no oversampling System Performance No Oversampling Oversampling Precision 88% 94% Recall 86% 95% F1 Score 87% 94% Table 4. Comparison of proposed method with previous research Method F1 Score NB with word unigram [8] 86.43% NB with unigram+bigram [8] 86.12% NB with trigram+quadgram [8] 86.17% RNN LSTM with oversampling (proposed) 94.00%
As in Table 4 which shows the system performance using f1 score, the previous method using NB shows the highest average results of 86% and the use of RNN LSTM gives an increase of 8% for the f1 score value.
The use of RNN LSTM with parameter settings in Figure 5 which has a fairly complex layer arrangement was able to give good results in classifying coarse text. However, the layer is too complex causes overfitting in the model [28] as shown in the graph in Figure 6. Overfitting is a condition when a model learns the training dataset too well but does not perform well on a data test [31].
Furthermore, through the results of the confusion matrix, the ability of the model to predict the test data given can be known. The proposed model can provide correct predictions of 1521 of 1626 test data given in the non abusive category. As for the rough category, the system can provide correct predictions as many as 1537 of the 1625 test data provided. There are quite a lot of correct data predicted by the system, this is reinforced by classifying it with test data that comes from outside the dataset used, as in Table 4. Then, Table 5 shows a row of sentences along with the probability values issued by the system based on the model that has been built and trained previously. The higher the probability value, it indicates that the sentence contains abusive words quite high. Meanwhile, the smaller the probability, the lower the abusive word in a sentence.
Figure 6. Graph accuracy and loss for RNN LSTM model
Table 2. Proportion of Data Before Oversampling After Oversampling Abusive Non Abusive Abusive Non Abusive 5043 8126 8126 8126
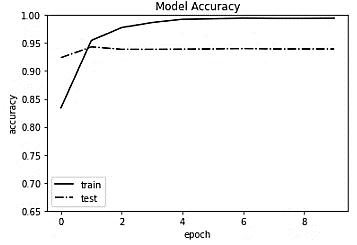
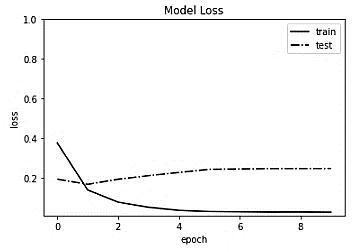
[5] S. E. Saad and J. Yang, “Twitter sentiment analysis based on ordinal regression,” IEEE Access, vol. 7, pp. 163677 163685, 2019, doi: 10.1109/ACCESS.2019.2952127.
[4] H. Gong, A. Valido, K. M. Ingram, G. Fanti, S. Bhat, and D. L. Espelage, “Abusive language detection in heterogeneous contexts: dataset collection and the role of supervised attention,” 2021, [Online]. Available: http://arxiv.org/abs/2105.11119.
[6] S. D. Swamy, A. Jamatia, and B. Gambäck, “Studying generalisability across abusive language detection datasets,” in Proceedings of the 23rd Conference on Computational Natural Language Learning (CoNLL), 2019, pp. 940 950, doi: 10.18653/v1/K19 1088.
[18] L. Kurniasari and A. Setyanto, “Sentiment analysis using recurrent neural network lstm in bahasa Indonesia,” J. Eng. Sci. Technol., vol. 15, no. 5, pp. 3242 3256, 2020.
[19] F. Friyanto and A. Ashadi, “The acquisition of swear words by students in Central Kalimantan,” RETORIKA J. Bahasa, Sastra, dan Pengajarannya, vol. 13, no. 2, pp. 1 26, Aug. 2020, doi: 10.26858/retorika.v13i2.13803.
The results of the study are used to classify rude comments on social media, especially twitter using a hybrid RNN and LSTM architecture. From the test results, the hybrid architecture RNN and LSTM can form a system that can identify abusive comments in comments uploaded on Twitter with optimal performance as evidenced by the results of precision, recall and f measure with each number is 94%, 95% and 94% respectively. The confusion matrix also displays the system’s performance in predicting the test data provided is quite good, 1537 abusive data can be predicted correctly by the system from a total of 1652 test data provided. Moreover, when the system test on other datasets, the system can predict better by showing a probability of abusive words. In addition, the use of oversampling techniques to handle imbalanced data can also contribute to improving system performance by 4% and a significant increase in precision, recall and f measure by 6%, 9%, and 7% respectively for abusive data. The proposed model can carry out initial identification of cyberbullying through the classification of abusive comments on social media. For future work, it can be further developed to build an automatic blocking system in support of government programs regarding cyberbullying prevention. In future work, our research will focus on trying other combinations of deep learning methods, such as LSTM CNN, BiLSTM, and several methods that are considered more sophisticated to improve system performance, especially in reducing overfit states in the model. In addition, we will try to implement this method on a larger amount of data to see if the method is able to produce good performance on data that has much more capacity.
[20] F. A. Prabowo, M. O. Ibrohim, and I. Budi, “Hierarchical multi label classification to identify hate speech and abusive language on Indonesian Twitter,” in 2019 6th International Conference on Information Technology, Computer and Electrical Engineering (ICITACEE), Sep. 2019, pp. 1 5, doi: 10.1109/ICITACEE.2019.8904425.
[7] S. Tuarob and J. L. Mitrpanont, “Automatic discovery of abusive thai language usages in social networks,” in Lecture Notes in Computer Science (including subseries Lecture Notes in Artificial Intelligence and Lecture Notes in Bioinformatics), 2017, pp. 267 278. [8] M. O. Ibrohim and I. Budi, “A dataset and preliminaries study for abusive language detection in Indonesian social media,” Procedia Comput. Sci., vol. 135, pp. 222 229, 2018, doi: 10.1016/j.procs.2018.08.169. [9] I. Krisnana et al., “Adolescent characteristics and parenting style as the determinant factors of bullying in Indonesia: a cross sectional study,” Int. J. Adolesc. Med. Health, vol. 33, no. 5, p. 1, Oct. 2021, doi: 10.1515/ijamh 2019 0019.
[15] M. P. Akhter, Z. Jiangbin, I. R. Naqvi, M. AbdelMajeed, and T. Zia, “Abusive language detection from social media comments using conventional machine learning and deep learning approaches,” Multimed. Syst., no. 0123456789, Apr. 2021, doi: 10.1007/s00530 021 00784 8. [16] G. S. Chadha, A. Panambilly, A. Schwung, and S. X. Ding, “Bidirectional deep recurrent neural networks for process fault classification,” ISA Trans., vol. 106, pp. 330 342, Nov. 2020, doi: 10.1016/j.isatra.2020.07.011.
[17] J. Du, C. M. Vong, and C. L. P. Chen, “Novel efficient RNN and LSTM like architectures: recurrent and gated broad learning systems and their applications for text classification,” IEEE Trans. Cybern., vol. 51, no. 3, pp. 1586 1597, Mar. 2021, doi: 10.1109/TCYB.2020.2969705.
[3] N. Cécillon, V. Labatut, R. Dufour, and G. Linarès, “Graph embeddings for abusive language detection,” SN Comput. Sci., vol. 2, no. 1, p. 37, Feb. 2021, doi: 10.1007/s42979 020 00413 7.
[11] J. H. Park and P. Fung, “One step and two step classification for abusive language detection on Twitter,” in Proceedings of the First Workshop on Abusive Language Online, 2017, pp. 41 45, doi: 10.18653/v1/W17 3006.
[21] M. O. Ibrohim, M. A. Setiadi, and I. Budi, “Identification of hate speech and abusive language on indonesian Twitter using the Word2vec, part of speech and emoji features,” in Proceedings of the International Conference on Advanced Information Science and System, Nov. 2019, pp. 1 5, doi: 10.1145/3373477.3373495.
Int J Artif Intell ISSN: 2252 8938 Abusive comment identification on indonesian social media data using hybrid deep learning (Tiara I Sari) 903
REFERENCES
[1] M. Amin et al., “Security and privacy awareness of smartphone users in Indonesia,” J. Phys. Conf. Ser., vol. 1882, no. 1, p. 12134, May 2021, doi: 10.1088/1742 6596/1882/1/012134.
[2] S. D. A. Putri, M. O. Ibrohim, and I. Budi, “Abusive language and hate speech detection for indonesian local language in social media text,” in Recent Advances in Information and Communication Technology 2021, 2021, pp. 88 98.
[12] C. Nobata, J. Tetreault, A. Thomas, Y. Mehdad, and Y. Chang, “Abusive language detection in online user content,” in Proceedings of the 25th International Conference on World Wide Web, Apr. 2016, pp. 145 153, doi: 10.1145/2872427.2883062.
[13] M. O. Ibrohim and I. Budi, “Multi label hate speech and abusive language detection in Indonesian twitter,” in Proceedings of the Third Workshop on Abusive Language Online, 2019, pp. 46 57, doi: 10.18653/v1/W19 3506.
5. CONCLUSION
[10] R. Wahanisa, R. Prihastuty, and M. Dzikirullah H. Noho, “Preventive measures of cyberbullying on adolescents in indonesia: a legal analysis,” Lentera Huk., vol. 8, no. 2, p. 267, Jul. 2021, doi: 10.19184/ejlh.v8i2.23503.
[14] P. Chakraborty and M. H. Seddiqui, “Threat and abusive language detection on social media in Bengali language,” in 1st International Conference on Advances in Science, Engineering and Robotics Technology 2019, ICASERT 2019, 2019, pp. 1 6, doi: 10.1109/ICASERT.2019.8934609.
BIOGRAPHIES OF AUTHORS Tiara Intana Sari, student who are pursuing undergraduate studies in the Department of Informatics, Faculty of Engineering at the University of Muhammadiyah Malang. Her area of interest is Data Science. She can be contacted at email: tiaraintana@webmail.umm.ac.id
Nur Hayatin is a lecturer at the Informatics Department of Engineering Faculty, University of Muhammadiyah Malang, Indonesia. She received her Master in Informatics Engineering from the Institute of Technology Sepuluh Nopember Surabaya Indonesia. Currently, she is undertaking a Graduate Research Assistant program at Universiti Malaysia Sabah. Her area of interest is data science specific in Natural Language Processing, social media analytics, Data Mining, and Information Retrieval. She can be contacted at email: noorhayatin@umm.ac.id Ruhaila Maskat is a senior lecturer at the Faculty of Computer and Mathematical Sciences, Universiti Teknologi MARA Shah Alam, Malaysia. In 2016, she was awarded a Ph.D. in Computer Science from the University of Manchester, United Kingdom. Her research interest then was in Pay As You Go dataspaces which later evolved to Data Science where she is now an EMC Dell Data Associate as well as holding four other professional certifications from RapidMiner in the areas of machine learning and data engineering. Recently, she was awarded the Kaggle BIPOC grant. Her current research grant with the Malaysian government involves conducting analytics on social media text to detect mental illness. She can be contacted at email: ruhaila@fskm.uitm.edu.my
ISSN: 2252 8938 Int J Artif Intell, Vol. 11, No. 3, September 2022: 895 904 904 [22] Z. Ahmad, A. S. Khan, C. W. Shiang, J. Abdullah, and F. Ahmad, “Network intrusion detection system: A systematic study of machine learning and deep learning approaches,” Trans. Emerg. Telecommun. Technol., vol. 32, no. 1, pp. 1 235, Jan. 2021, doi: 10.1002/ett.4150. [23] S. Marsella and J. Gratch, “Computationally modeling human emotion,” Commun. ACM, vol. 57, no. 12, pp. 56 67, Nov. 2014, doi: 10.1145/2631912.
[26] S. Hochreiter and J. Schmidhuber, “Long short term memory,” Neural Comput., vol. 9, no. 8, pp. 1735 1780, Nov. 1997, doi: 10.1162/neco.1997.9.8.1735.
[27] H. Chung and K. Shin, “Genetic algorithm optimized long short term memory network for stock market prediction,” Sustainability, vol. 10, no. 10, p. 3765, Oct. 2018, doi: 10.3390/su10103765.
[28] H. il Lim, “A study on dropout techniques to reduce overfitting in deep neural networks,” in Lecture Notes in Electrical Engineering, 2021, vol. 716, pp. 133 139, doi: 10.1007/978 981 15 9309 3_20.
[29] D. Chicco, V. Starovoitov, and G. Jurman, “The benefits of the matthews correlation coefficient (MCC) over the diagnostic odds ratio (DOR) in binary classification assessment,” IEEE Access, vol. 9, no. Mcc, pp. 47112 47124, 2021, doi: 10.1109/ACCESS.2021.3068614.
[30] T. I. Sari, “Abusive Twitter identification fithub.” [Online]. Available: https://github.com/tiaraintana/Abusive Twitter Identification.
[25] A. K. Uysal and S. Gunal, “The impact of preprocessing on text classification,” Inf. Process. Manag., vol. 50, no. 1, pp. 104 112, Jan. 2014, doi: 10.1016/j.ipm.2013.08.006.
[31] A. Power, Y. Burda, H. Edwards, I. Babuschkin, and V. Misra, “Grokking: generalization beyond overfitting on small algorithmic datasets,” pp. 1 10, Jan. 2022, [Online]. Available: http://arxiv.org/abs/2201.02177.
Zalfa Natania Ardilla is a student of Bachelor degree at Informatics Department of Engineering Faculty, University of Muhammadiyah Malang. Her area of interest in data science. She can be contacted at email: zalfaardilla@webmail.umm.ac.id
[24] D. Lee et al., “Long short term memory recurrent neural network based acoustic model using connectionist temporal classification on a large scale training corpus,” China Commun., vol. 14, no. 9, pp. 23 31, Sep. 2017, doi: 10.1109/CC.2017.8068761.
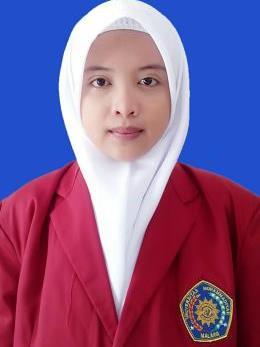



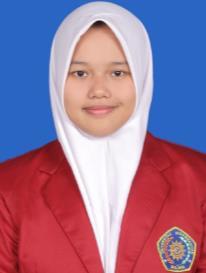



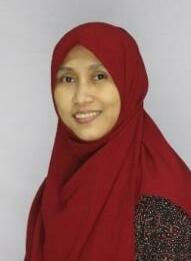



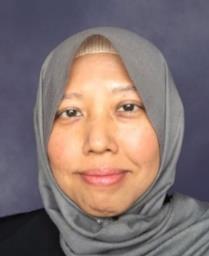



This is an open access article under the CC BY SA license.
Journal homepage: http://ijai.iaescore.com
1. INTRODUCTION
Corresponding Author: Oumaima LaboratoryHourraneInformation Technology and Modeling, Faculty of Sciences Ben Msik, Hassan II University of Email:MoroccoCasablancaoumaima.hourrane@gmail.com
Plagiarism is the use of original text data without providing adequate references. This phenomenon is accentuated when the root of plagiarism is in a different language, which is known as cross lingual plagiarism. Although some research works have been carried out on monolingual plagiarism analysis, to our awareness, cross lingual plagiarism analysis is still an emerging natural language processing task that has been studied in the literature. The task can be described as follows: Given a suspect document in a certain language, we are interested in checking if it is plagiarized from one or a set of original documents written in other language.Current cross lingual plagiarism detection approaches usually employ syntactic and lexical properties, external machine translation (MT) systems, or computing similarities between multilingual documents. Yet, these methods are conceived for literal plagiarism such as copy and paste, and their performance is diminished when handling complex cases of plagiarism including paraphrasing. The literal plagiarism form “copy and paste” in theory, the most easily detected and identifiable textual similarity. Certainly, the detection of this form is similar to checking the identity between two texts. To ingenuously
Graph transformer for cross-lingual plagiarism detection
Oumaima Hourrane, El Habib Benlahmar Laboratory Information Technology and Modeling, Faculty of Sciences Ben Msik, Hassan II University of Casablanca, Casablanca, Morocco
Article Info ABSTRACT
Article history: Received Sep 8, 2021 Revised Apr 21, 2022 Accepted May 20, 2022
The existence of vast amounts of multilingual textual data on the internet leads to cross lingual plagiarism which becomes a serious issue in different fields such as education, science, and literature. Current cross lingual plagiarism detection approaches usually employ syntactic and lexical properties, external machine translation systems, or finding similarities within a multilingual set of text documents. However, most of these methods are conceived for literal plagiarism such as copy and paste, and their performance is diminished when handling complex cases of plagiarism including paraphrasing. In this paper, we propose a new graph based approach that represents text passages in different languages using knowledge graphs. We put forward a new graph structure modeling method based on the Transformer architecture that employs precise relation encoding and delivers a more efficient way for global graph representation. The mappings between the graphs are learned both in semi supervised and unsupervised training mechanisms. The results of our experiments in Arabic English, French English, and Spanish English plagiarism detection show that our graph transformer method surpasses the state of the art cross lingual plagiarism detection approaches with and without paraphrasing cases, and provides further insights on the use of knowledge graphs on a language independent model.
IAES International Journal of Artificial Intelligence (IJ AI) Vol. 11, No. 3, September 2022, pp. 905 915 ISSN: 2252 8938, DOI: 10.11591/ijai.v11.i3.pp905 915 905
Keywords: Cross lingual plagiarism Graph neural network Graph Knowledgetransformergraphs
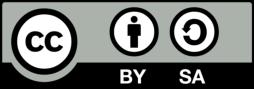
ISSN: 2252 8938 Int J Artif Intell, Vol. 11, No. 3, September 2022: 905 915 906carry out this analysis automatically, it is required to carry out a word for word comparison of texts. Since this process is far too time consuming to be integrated into commercially oriented or online solutions, as is the case with most of the anti plagiarism tools, alternative methods had to be produced, which is one of the goals of this present Furthermore,work.itis an accepted fact that automatically detecting textual semantic similarities such as paraphrase does not amount to detecting a possibility of plagiarism. Plagiarism is copying or paraphrasing text without citing the original reference, but in the case of textual similarity, we cannot know if the texts are similar literally or semantically, and consequently to correlate this similarity with plagiarism. It will then be up to a human to identify whether or not any similarities detected count as plagiarism. Certainly, they can be resulting from coincidences or from properly cited references. In this work, we do not pass judgment or make any decisions; we only focus on finding similar passages between two texts. We can describe plagiarism detection process as a system composed of two consecutive tasks [1] The first task is the candidate source retrieval of suspicious documents to compare later, and the second task is the detailed comparison, which is finding alignments of similar passages of pairs of documents, between the suspect document being processed and each of the sources returned by the first task. This paper focuses only on the second task: the cross lingual comparison between suspect texts and a fixed number of candidate source textsInthis paper, we proposed cross lingual graph transformer based analysis (CL GTA), an approach for cross lingual plagiarism detection that aim to represent the whole context by using knowledge graphs simultaneously to broaden and connect the concepts in a textual document. For graph representation, we propose a new model called Graph Transformer that depends completely on the multi head attention mechanism [2]. The graph transformer enables direct representation of relations between any two nodes without considering their remoteness in the graph. At last, we evaluate our method and compare it against the state of the art using a dataset composed of manually and automatically created paraphrases, we also evaluate the performance of the analysis using paraphrases only. The rest of the paper is structured as follows. In Section 2 we cite the state of the art methods in cross lingual plagiarism detection. In Section 3 we describe the background on transformers and graph neural networks. Then we describe the knowledge graph creation and the graph transformer model for graph representation, and then we conclude the section with the general framework for cross lingual plagiarism detection. We evaluate in section 4 our approach for Spanish English, French English, and Arabic English corpora, and comparing our results with various state of the art approaches. We also show the results of detecting only paraphrases.
2. RELATED WORK
This section reviews the methods of cross language similarity computing that have been employed for cross Lingual plagiarism detection. An effectual algorithm for cross languages with lexical and syntactic similarities is the cross language character n gram (CL CnG) [3]. It is basically similar to some other monolingual plagiarism detection models [4], [5]. This model is syntax based that employs character n grams to model texts, namely, after text segmentation into 3 grams, the authors transformed it into tf idf matrices of character 3 grams, after that, they used a weighting mechanism and cosine similarity as a metric for similarityVariouscomputing.methods exist that use parallel corpora, which is called cross language alignment based similarity analysis (CL ASA) [6], [7]. This type of analysis is usually based on a statistical Machine Translation system. It determines how a text passage is probably the translation of other text using a statistical bilingual dictionary generated with parallel a corpus which contains translation pairs. To make text alignment, this method takes into account the translation probability distributions and the variances in size of parallel texts in distinct languages. There are two other approaches employing concepts from knowledge graphs like in this paper, they are referred to as cross language thesaurus based similarity analysis (CL TSA) models. The first approach is called MLPlag [8], where the authors used EuroWordNet ontology [9] that changes words into language independent forms. They also presented two measures of similarity: Symmetric Similarity Measures (MLPlag SYM) which is derived in part from the traditional vector space model (VSM), the second measure is Asymmetric Similarity Measure (MLPlag ASYM) which is the opposite of the previous measure. other similar method employs a multilingual semantic graph to construct knowledge graphs that represent the context of documents [10] Other cross language similarity analysis is the cross language explicit semantic analysis (CL ESA) [11]. It is built on the classic explicit semantic analysis (ESA) model. This approach models the semantic
Int J Artif Intell ISSN: 2252 8938 Graph transformer for cross lingual plagiarism detection (Oumaima Hourrane) 907
meaning of a text by an embedding based on the vocabulary retrieved from Wikipedia, to find a document within a multilingual corpus.
In recent years, more approaches based on word embedding have been proposed for the cross lingual semantic similarity. Lo and Simard [16] uses BERT with a similarity metric for cross lingual semantic textual similarity. The metric is based on a unified adequacy oriented Machine Translation quality evaluation and estimation metric for multi languages. Another approach [17] uses word embeddings for cross lingual textual similarity detection instead of lexical dictionaries. They present syntactic weighting in the sentence embedding. By using the Multivac toolkit that includes word2vec, paragraph vector and bilingual distributed representation features, and then assigning weights to the Part Of Speech tag of each word in the sentence. Asghari et al. [18] used Continuous Bag of Words Model (CBOW) and skip gram models, and employed an averaging approach to combine word embedding to create of sentence embeddings, which are then compared using Cosine Similarity metric between source and suspect documents. Finally, an approach called Language Agnostic Sentence Representation (LASER) [13] provides a Bidirectional Long short term memory (BiLSTM) encoder which was trained on 93 languages, so they obtain sentence embeddings from the encoder via max pooling of the last layer outputs and applying cosine similarity on corresponding sentence embeddings of each sentence pair.
Where d is the dimension of k and q. By arranging k attention layers into the multi head attention, the outputs of all attention heads are combined and projected to the original dimension of x, followed by feed forward layers, residual connection, and layer normalization, The output matrix can be written as: ������������������(��,��,��) = ������������(ℎ������1,...,ℎ��������)���� , ℎ������ = ������������������(������ ��,������ �� , ������ ��) Where ���� ��,���� ��,���� �� , are the projection matrices of head i To clarify the whole procedure, we denote the mechanism presented previously as a single function denoted as ��������(x,y1:m) . Given an input sentence ��1:��, the self attention encoder iteratively calculates the sentence representation by: ���� �� = ��������(���� ��,x1:�� �� 1) Where L is the number of layers and ��1:�� 0 is word embeddings. Thus, this representation can build a direct relation with other long distance representations. In order to preserve the sequential order of words, the position encoding technique is proposed [2] to show the
One of the obvious ways to analyze the cross language plagiarism is the Translation + Monolingual Analysis (T+MA). For example, in [12], the system is simply divided into two components. The MT system that translates suspicious documents into English, they employ the transformer framework for the MT. The second component is the source retrieval it receives as inputs to the translated suspicious document’s n grams and returns documents ids from the reference English collection. Finally, the system performs the comparison between translated suspicious documents and the sources. In other approach [13], OpenNMT Library [14] is used to train an MT model as an additional requirement to estimate the pairwise similarity between sentences. For the last model, they fine tuned the Bidirectional Encoder Representations from Transformers (BERT) Multilingual model [15] for the sentence pair classification and put the linear layer for on top of the pooled output of BERT.
3.1.1. Transformer Transformer [2] is a neural network model primarily employed for neural Machine Translation systems. It uses a self attention mechanism for creating both the encoder and the decoder [19] [21], that directly represents relationships between words in a sentence, regardless of their particular position. The encoder consists of multiple identical layers and sub layers. The first layer is a multi head self attention mechanism, and the second layer is a position wise fully connected feed forward network. The multi head attention puts together multiple dot product attention layers that supports parallel running. Each dot product attention layer takes a set of queries, keys, values (q, k, v) as inputs. After that, it calculates the dot products of the query with all keys and applies a Softmax function to get the weights on the values. By stacking the set of (q, k, v)s into matrices (Q, K, V), it accepts highly optimized matrix multiplications. More precisely, the outputs can be structured as a matrix: ������������������(��,��,��) = ��������������(������ /√��)��
3. METHODOLOGY 3.1. Preliminaries
3.1.2. Graph neural networks (GNNs) Graph neural networks have gained attention in different domains, such as knowledge graphs, social networks, citation networks, and drug discovery. Graph neural networks build representations of entities and edges in graph data. Their key process lies in message passing process between the entities, where each node gathers features from its neighbors to update its representation of the local graph structure around it. The message passing operation iteratively updates the hidden features Hv of a node v, by concatenating the hidden states of v’s neighboring entities and edges. In each layer, the following equation is applied: hv k = σ(Wk∑ hu k 1 |N(v)| +Bkhv k 1) Where:k = 1,…,k 1
Nouns, verbs, adjectives, and adverbs are clustered into sets of synonyms denoted as synsets, each representing a discrete concept. Synsets are interrelated using conceptual lexical and semantic relations. Secondly, WordNet labels the semantic relations among words, whereas the groupings of words in a dictionary do not follow any specific pattern other than meaning similarity. As mentioned before, we use Extended Multilingual Wordnet with large wordnets over 26 languages and smaller ones for 57 languages. It is made by combining wordnets with open data from Wiktionary, and the Unicode Common Locale Data Repository. 3.2. Model architecture 3.2.1. Creating knowledge graphs In this section, we present the steps to create the Knowledge Graphs. We build the knowledge graph by searching WordNet for paths connecting pairs of synsets in V. At first, we preprocess the text segment using tokenization, multi word extraction, lemmatization, part of speech tagging (POS), and to obtain the list of tuples (lemma, tag). Next, we create an initially empty knowledge graph G = (V,E), such that V = E = ∅. We populate the vertex set V with the set of all the synsets in WordNet which contain any <lemma, tag> tuple T in the text segment language L Finally, for each pair {v,v’}∈ V such that v and v’ do not share any lexicalization in T, foreach path in WordNet �� →��1 → → ���� → ��′, we set: �� ∶=��⋃ {��1, ,����} and �� ∶= �� ⋃{(��,��1), ,(����,��’)} Consequently, we put all the path nodes and edges to graph G. The length of each path is limited to a maximum of three [25]. Finally, we obtain a knowledge graph that represents the semantic context of the text by populating the graph with intermediate relations and nodes.
3.2.2. Knowledge graph notation We denote E and R as the set of entities and relations respectively. A triple is defined as (h, r, t), where ℎ ∈�� is the head, �� ∈�� is the relation, and �� ∈�� is the tail of the triple. Let ���� represent the set of all triples that are true in a world, and ����’ represent the false ones. A knowledge graph is a subset of ����
ISSN: 2252 8938 Int J Artif Intell, Vol. 11, No. 3, September 2022: 905 915 908position order to the model. Therefore, the input representation will be the concatenation of word embedding and position encoding.
The first part bellow of the equation is averaging all the neighbors of node v: Wk∑ hu k 1 |N(v)| , while the second part is the embedding layer of node v multiplied by a bias Bk that is a trainable weight matrix generally, this part is called a self loop activation for node v and can be described as follow: Bkhv k 1. Then the non linearity activation such as sigmoid function is performed on the two parts. After L operations of message passing, the hidden states of the last layer K are used as the embeddings of the entities, and can be described as zv = hv K 3.1.3. Knowledge graph A knowledge graph is a graph relating entities and concepts and can assist a machine to learn human common sense. The core of our approach is to use a graph representation that allows an alignment across languages. To build knowledge graphs for this purpose we employ Extended Open Multilingual Wordnet [22] which offers a wider set of concepts in several languages to date We will present this semantic network in the next paragraph, then in Section 3.2.2, we introduce the steps needed to obtain our multilingual knowledge graphs of documents. WordNet. Wordnet is a wide electronic lexical database for English [23], [24], with a hierarchical formation of concepts, where more specific concepts derive information from their neighbors, more general concepts.
Figure 1. Graph transformer architecture Node representation. The most essential characteristic of this model is that it has a fully connected interface of random input graphs. |Each node can directly send and get information to another node no matter if they are directly connected or not. This is achieved by the relation enhanced global attention setting. In short, the relation between any node pair is presented as the shortest relation path between them. These paths between the two entities are used then as an input to relation encoding process, we denote the resulting learned vector as rij: the relation between node i and j. As the vanilla multi head attention, we compute our attention as follow:[����→�� ; ����→��]= ����������, where we divide the relation encoding into forward encoding ri>�� and backward encoding encoding rj>��. The node vectors are initiated by the sum of the node embedding and position encoding. Multiple layers of the global attention are then combined to calculate the final node representation. For each layer, a node vector is updated based on all other node vectors and the corresponding relation encodings. Relation encoding. In this work, to represent the relationship between two nodes we used the shortest path approach, because it usually offers the nearest and most crucial relationship between them. Based on the sequential characteristic of this relationship, we used a bi directional Gated Recurrent Unit (GRU) [26] to get a probabilistic representation of it. We denote the shortest path between node i and node j as pij: ����>�� =��������(����>�� 1,������) ����>�� =��������(����>�� 1,������)
3.2.3. Graph transformer After creating graph knowledge, the next step consists of representing the graphs by weighting all concepts (entities) and semantic relations. Current graph neural networks calculate the node representation using a function of the input node and all its the receptive field of adjacent neighborhoods, which leads to inefficient long distance information exchange. Therefore, we propose a new mechanism as shown in Figure 1, and known as Graph Transformer which enables relation aware global communication.
The final relation encoding is expressed as [����>�� .����>��]which is the addition of the last hidden states of forward and backward GRUs.
Int J Artif Intell ISSN: 2252 8938
Graph transformer for cross lingual plagiarism detection (Oumaima Hourrane) 909
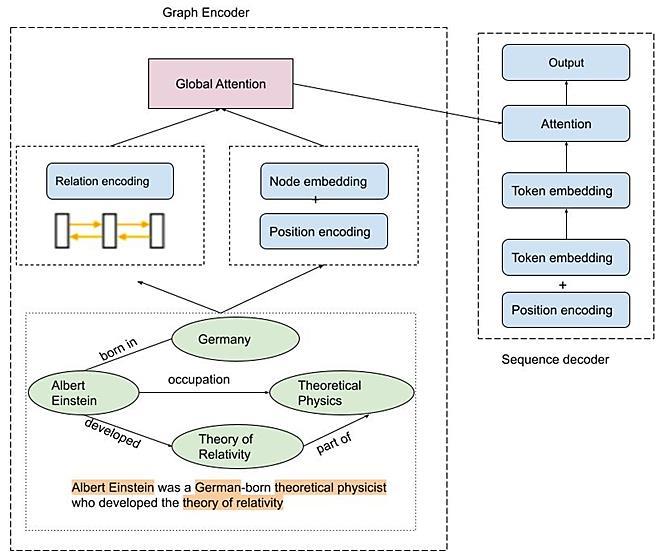
ISSN: 2252 8938 Int J Artif Intell, Vol. 11, No. 3, September 2022: 905 915 910
Sequence decoder. After the graph encoding, we learn a mapping between two graphs: G →G′ , whereG = (node1, ,noden). This mapping is learned both in semi supervised and unsupervised training mechanisms. We use the encoder decoder mechanism to map the node vectors into low dimensional space. The encoder learns the node representation of the input sentence and the decoder employs this representation to rebuild in reverse order the sentence. The sequence decoder reflects the same process as the transformer decoder. We update the hidden state at each time step by computing a multi head attention mechanism over the output of the encoder and the previously generated words. Finally, we minimize the error between input sentences and reconstructed output sentence during the training as follow: Erec = ‖s ˆs‖2 3.2.4. Cross-language plagiarism detection framework We explain in this section in detail the framework for cross lingual plagiarism detection. It is originally proposed by [10] as well as the post processing analysis of similarities between text segments. As shown in Figure 2. Given a source document ���� in a language L and a suspicious document ����0 in a languageL0, we process documents in a four main step: (i) Text segmentation. We first segment the documents to be compared, to obtain the sets of segments ���� and ����0 by using a sliding window of five sentences with a two sentences step to produce the segments. (ii) Creating knowledge graphs. Next, we implement the procedure presented in Section 3.1 to create the graph sets G and ��0of the text segments ����and ����0 (iii) Graph representation. It is the global graph representation as presented in Section 3.3. (iv) Knowledge graphs similarity. Find K nearest vectors by cosine similarity from source documents. (v) Post processing analysis of similarities. After obtaining the set of the similarities between the text chunks of the source document ���� and suspected documents����0, we use the method proposed by [27] to analyze the similarity scores and identify which segments of the suspected document are cases of plagiarism. Briefly, for each text chunk of ����, we get the top five most similar chunks of document ����. Then, iteratively we run the algorithm until convergence that aggregates the segments of ���� with a distance δ lower than a threshold ��ℎ������1. At last, we select as plagiarism the cases which combine more than ��ℎ������2 text segments. A function offsets offers the start and end offsets of the plagiarism case. We use this algorithm to evaluate all the models compared in the evaluation section.
Figure 2. Cross language plagiarism detection framework
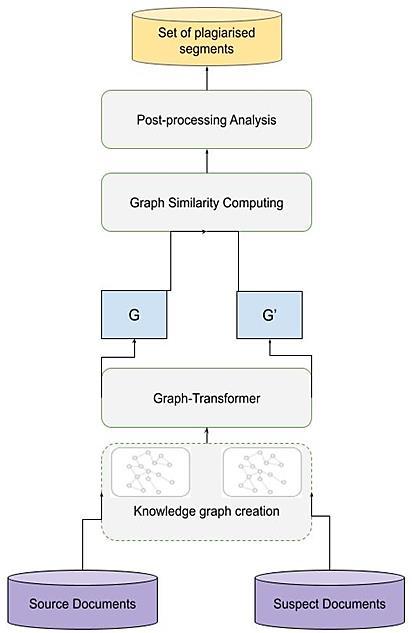
- English Arabic parallel corpora. The corpus of the Arabic English case was taken from different parallel corpora. It consists of 547 aligned passages from 58,911 pairs from the United Nations Parallel Corpora [32], the OPUS collection of translated texts from the web [33] and King Saud University corpus [34]. We used another corpus prepared by [35] which has roughly 2085 of paraphrased translated pairs which will be used when evaluating only paraphrasing cases.
The ������������ is an F measure macro which takes into account the size of the plagiarized passages instead of only considering the absolute number of plagiarized passages. This is the harmonic mean between precision and recall. It can be expressed as follow:������������ = 2������������������(��,��)������������(��,��) ������������������(��,��)+������������(��,��)
Int J Artif Intell ISSN: 2252 8938 Graph transformer for cross lingual plagiarism detection (Oumaima Hourrane) 911 4. EXPERIMENTS
The recall represents the fraction of plagiarized text that has been found. It measures the number of characters correctly returned as plagiarized over the number total number of characters to be returned as plagiarized. The recall can be expressed as follow:������������(��,��)= 1 |����|∑ |⋃ ��∩����∈�� | ��|��| ∈��
PAN 2011 corpus has been used for the cross lingual plagiarism detection competition of PAN at CLEF [27]. This corpus contains portions of writings of similar books in multiple languages. These texts are from books freely accessible on the Gutenberg Project website, available as Spanish English (ES EN) pairs. - Conference papers. We used the processed conference papers corpus [31]. These are English French conference papers that were first published in one language and then translated by their authors to be published in other language. A sum of 35 pairs of English French conference papers was retrieved.
There is an issue that plagiarism detectors sometimes report overlapping or multiple detections for a single plagiarism case, and precision and recall do not perform well for that. We approach this problem by measuring the detector’s granularity. The granularity is a measure first introduced in the work of Potthast et al. [28]. It determines whether a fragment is detected in whole or in pieces. This measure penalizes cases where passages, which are found, plagiarized, overlap. The granularity can be expressed as follow: ����������������������(��,��) = 1 |����| , where ���� ∈�� are cases identified by detectors in R, and ���� ∈ �� are detections of S. Plagdet (plagiarism detection) is a measure combining precision and recall oriented for plagiarism detection and granularity. Plagdet is expressed as follow: ��������������(��,��)= ������������ log2(1+����������������������(��,��)) .
We evaluate and compare our CL GTA for plagiarism detection model with several state of the art approaches in the task of cross lingual plagiarism analysis. Given a collection of source documents DL0 in a language L0 and a suspect document dL in a language L, we would like to identify all the plagiarized segments of dL from the source documents DL0. We used as an evaluation metric the scores: precision, recall, granularity, and plagdet [28].
4.1. Evaluation metrics
We used as an evaluation metric the scores: precision, recall, granularity, and plagdet [28]. We denote S as the set of plagiarism cases in the suspect documents and R as the set of plagiarized sequences. The characters for a plagiarized case are denoted as s ∈ S. Likewise, the characters for a plagiarized text are represented as r ∈ R. Following these notations, and we measure the precision and the recall at the character level of R under S as follow: The precision represents the fraction of fragments found which cases of plagiarism are really. It measures the number of characters correctly returned as plagiarized on the total number of characters returned. Theprecision can be expressed as follow: ������������������(��,��)= 1 |��|∑ |⋃ ��∩����∈�� | ��|��| ∈��
4.2. Construction and properties of the corpus In this work, our dataset consists of English, French, and Spanish documents. We decide to reuse already existing collections of parallel and comparable corpora in order to constitute a base for our corpus. These datasets are presented as follow: Europarl [29] is a corpus for cross language and MT research. This corpus embodies about 10,000 parallel documents of the European Parliament exchanging transcriptions, in French, English and Spanish languages.
JRC Acquis [30] is usually used in cross language and MT tasks. This corpus represents extracts of Acquis Communautaire. It consists of 10,000 parallel documents, available in French, English, and Spanish languages. Wikipedia is usually used as a parallel corpus of multiple languages. We chose to use 10,000 of French, English, and Spanish aligned documents. In total, this corpus contains 30,000 documents.
The results of our experiments were broken down in two folds: (i) we compared our model with the state of the art approaches, assessing the performance when detecting the cross lingual plagiarism cases of our corpora ES EN, FR EN, AR EN; (ii) we examined the performance on solely the cross lingual paraphrasing cases of plagiarism for the Spanish English and Arabic English partitions.
ISSN: 2252 8938 Int J Artif Intell, Vol. 11, No. 3, September 2022: 905 915 912 4.3. Evaluation protocol
To precisely evaluate the methods of detecting cross lingual plagiarism on our corpus, we present as follow, a dedicated evaluation protocol. We denote a parallel or comparable corpus as C, which is made up of N pairs of documents, such that for each document ���� ∈ �� a corresponding document ��0�� ∈ ��0 exists, where i is an integer between 1 and N, we decide to compare all the N documents available in D to M documents of ��0. This has the advantage of avoiding making ��2comparisons and thus having much too long computation times in the case of too large corpora. Each document ���� is compared to its corresponding document ���� and to M 1 other documents randomly selected with replacement in ��0. The ��0 document can thus be set more than once. M is set at 1,000 documents agreeing with the state of the art [36] The graph encoder and sequence decoder use randomly initialized node and word embeddings respectively. To prevent overfitting, we apply dropout with the drop rate of 0.2 [34]. We apply a special UNK token to replace with a rate of 0.33 the input nodes. We used Adam optimizer for parameter optimization with ��������1 =0.9 and ��������1 =0.999 [37]. We adopt the same learning rate of a standard transformer [2], and then we encode all shortest paths into vector representation by the relation encoding. For a fair evaluation, we compared our CL GTA model with the state of the art Cross Language Character N Gram CL C3G [3], Cross Language Alignment based SimilarityAnalysis CL ASA [38], Cross Language Explicit Semantic Analysis CL ESA [11], and Cross Language Knowledge Graph Analysis (CL KGA) in [39] models. We also used the length model of [40] as a baseline.
5.1. Comparison with the state of the art 5.1.1. Results
Table 1 shows the results obtained for Spanish English (ES EN), French English (FR EN), and Arabic English (AR EN) partition, For the Spanish English (ES EN). Partition, the CL GTA approach has the best Plagdet score of 0.62, followed by the length Model with a score of 0.604, then the Cross Language Conceptual Thesaurus base Similarity CL CTS model with a score of 0.584. The difference between the scores of all the other approaches is not huge, except for the CL C3G with a score of 0.169. This is far lower than the State of the art CL GTA. For or the French English (FR EN), same as the Spanish English cases, the CL GTA reaches the best Plagdet score of 0.584, followed by the Length Model with a score of 0.553, then the Cl CTS with a score of 0.584. For the Arabic English partition, the results prove a different outcome, with the CL CTS in the first place with a Plagdet score of 0.534 followed by our model CL GTA with a score of 0.522.
5. RESULTS AND DISCUSSION
5.1.2. Discussion The results for French English compared to Spanish English were similar but with reduced performance. Spanish, French, and English do not share many grammatical characteristics. For all partitions, the CL C3G got the lowest result, since syntactic and lexical features are important for high character n gram overlap. After that comes CL ESA, since it is based on computing similarities with document collections, the
Table 1. Results of comparison with the state of the art Method Length Model CL C3G CL ASA CL ESA CL CTS CL GTA (1). Spanish English Plagdet 0.075 0.018 0.056 0.070 0.092 0.112 Precision 0.149 0.048 0.153 0.160 0.186 0.203 Recall 0.058 0.020 0.041 0.019 0.063 0.085 Granularity 1.000 1.000 1.001 1.061 1.000 1.000 (2). French English Plagdet 0.553 0.065 0.405 0.395 0.504 0.584 Precision 0.469 0.067 0.343 0.300 0.452 0.506 Recall 0.683 0.306 0.029 0.356 0.633 0.690 Granularity 1.007 1.099 1.103 1.112 1.017 1.000 (3). Arabic English Plagdet 0.520 0.192 0.690 0.303 0.534 0.522 Precision 0.401 0.203 0.465 0.278 0.452 0.409 Recall 0.598 0.025 0.758 0.423 0.633 0.576 Granularity 1.010 1.082 1.203 1.105 1.007 1.101
5.2. Cross language plagiarism detection with paraphrasing 5.2.1. Results Table 2 shows the results of the paraphrasing cases evaluation on the PAN competition (PAN PC 11) corpus. Our model CL GTA reaches the state of the at on this task by a Plagdet score of 0.112, followed by the CL CTS model with a score of 0.092; then the Length Model in the third place with a score of 0.075. We report as well the results of the paraphrasing cases of the Arabic English paraphrased translated pairs. Same as the previous results, our method CL GTA proved superior with a Plagdet score of 0.108, followed with the Length Model with a score of 0.105, then the CL CTS model with a score of 0.099. The difference between the scores of all the other approaches is not big for both datasets, except for the CL C3G with a score of 0.021. This is far again lower than the State of the art CL GTA.
6. CONCLUSION
To conclude, in this paper, we have introduced a new approach for detecting cross lingual semantic textual similarities based on knowledge graph representations and we have also augmented a state of the art method by introducing these representations. We referred to our method as CL GTA. We then introduced the notion of graph transformer, which is a new graph representation method based on the transformer architecture that employed explicit relation encoding and offers a more efficient way to represent global
5.2.2. Discussion
Int J Artif Intell ISSN: 2252 8938 Graph transformer for cross lingual plagiarism detection (Oumaima Hourrane) 913 model obtained a higher number of false positives. The CL ASA is comparable with CL ESA but with higher precision. The third best in ranking is the CL CTS, which is also based on knowledge graphs, but with classical weighting for nodes. The length model offered higher performance compared to the state of the art. However, our CL GTA model obtained the best results, suggesting that the proposed model benefits from the explicit relation encoding which provides a more efficient way for global graph representation, leading to better results to measure cross lingual similarity. Regarding the Arabic English case, we got a slightly different outcome with the CL CTS better than our model CL GTA, since the two approaches are based on graphs, the main difference is that the CL CTS used different Knowledge graph sources and different techniques of graph representation. In this case, the classical weighting proves Superior for the Arabic language. However, by changing the weights and using a far richer Knowledge graphs construction, the CL GTA can prove to be effective prospectively.
Table 2. Results of the cross language plagiarism detection with paraphrasing Method Length Model CL C3G CL ASA CL ESA CL CTS CL GTA (1). Spanish English Plagdet 0.075 0.018 0.056 0.070 0.092 0.112 Precision 0.149 0.048 0.153 0.160 0.186 0.203 Recall 0.058 0.020 0.041 0.019 0.063 0.085 Granularity 1.000 1.000 1.001 1.061 1.000 1.000 (2). French English Plagdet 0.553 0.065 0.405 0.395 0.504 0.584 Precision 0.469 0.067 0.343 0.300 0.452 0.506 Recall 0.683 0.306 0.029 0.356 0.633 0.690 Granularity 1.007 1.099 1.103 1.112 1.017 1.000 (3). Arabic English Plagdet 0.520 0.192 0.690 0.303 0.534 0.522 Precision 0.401 0.203 0.465 0.278 0.452 0.409 Recall 0.598 0.025 0.758 0.423 0.633 0.576 Granularity 1.010 1.082 1.203 1.105 1.007 1.101
As mentioned before, the PAN PC 11 dataset contains cross lingual paraphrasing cases of plagiarism, which is a more complex form of plagiarism to detect since it restates the text using other terms in order to conceal plagiarism. We conducted hereby a further experiment to examine only paraphrasing cases of plagiarism extracted from the corpus. We observe that the differences between the results of the models were identical to the previous results using the entire dataset at a smaller scale. CL GTA obtained higher performance compared to the other baselines. We conducted another experiment on the Arabic English paraphrased translated partition. In contrary to the previous results on literal plagiarism case, our model overcame the CL CTS model with a score of 0.108, which proves that in terms of semantic similarity, is better represented with our graph transformer architecture. This result is true even when representing the linear text sequence and this is due to the attention mechanism of the transformer, which allows a model to focus on the most relevant parts of the graph, thus representing the global graph dependencies in an efficient way. This is the main goal of this paper.
[9] C. Jacquin, E. Desmontils, and L. Monceaux, “French EuroWordNet Lexical Database Improvements,” in Computational Linguistics and Intelligent Text Processing, Springer Berlin Heidelberg, 2007, pp. 12 22.
[19] J. Cheng, L. Dong, and M. Lapata, “Long Short Term Memory Networks for Machine Reading,” 2016, doi: 10.18653/v1/d16 1053. [20] W. Kryściński, R. Paulus, C. Xiong, and R. Socher, “Improving Abstraction in Text Summarization,” 2018, doi: 10.18653/v1/d18 1207. [21] H. Chen et al., “Low Dose CT With a Residual Encoder Decoder Convolutional Neural Network,” IEEE Transactions on Medical Imaging, vol. 36, no. 12, pp. 2524 2535, Dec. 2017, doi: 10.1109/tmi.2017.2715284.
[7] D. Pinto, J. Civera, A. Barrón Cedeño, A. Juan, and P. Rosso, “A statistical approach to crosslingual natural language tasks,” Journal of Algorithms, vol. 64, no. 1, pp. 51 60, Jan. 2009, doi: 10.1016/j.jalgor.2009.02.005.
[23] G. A. Miller, “Wordnet: a lexical database for english,” Communications of the ACM, vol. 38, no. 11, pp. 39 41, 1995.
[4] P. Clough, “Old and new challenges in automatic plagiarism detection,” National Plagiarism Advisory Service, 2003.
[5] H. A. Maurer, F. Kappe, and B. Zaka, “Plagiarism a survey,” Journal of Universal Computer Science, vol. 12, no. 8, pp. 1050 1084, 2006.
[8] Z. Ceska, M. Toman, and K. Jezek, “Multilingual Plagiarism Detection,” in Artificial Intelligence: Methodology, Systems, and Applications, Springer Berlin Heidelberg, pp. 83 92.
[11] E. Gabrilovich and S. Markovitch, “Computing semantic relatedness using wikipedia based explicit semantic analysis,” IJCAI, vol. 7, pp. 1606 1611, 2007. [12] O. Bakhteev, A. Ogaltsov, A. Khazov, K. Safin, and R. Kuznetsova, “Crosslang: the system of cross lingual plagiarism detection,” 33rd Conference on Neural Information Processing Systems (NeurIPS 2019), pp. 1 9, 2019.
[17] J. Ferrero, L. Besacier, D. Schwab, and F. Agnès, “Using Word Embedding for Cross Language Plagiarism Detection,” 2017, doi: 10.18653/v1/e17 2066.
[24] C. Fellbaum, “A Semantic Network of English: The Mother of All WordNets,” in EuroWordNet: A multilingual database with lexical semantic networks, Springer Netherlands, 1998, pp. 137 148. [25] R. Navigli and S. P. Ponzetto, “Multilingual wsd with just a few lines of code: the babelnet api,” in Proceedings of the 50th Annual Meeting of the Association for Computational Linguistics, 2012, pp. 67 72.
REFERENCES
[3] P. McNamee and J. Mayfield, “Character N Gram Tokenization for European Language Text Retrieval,” Information Retrieval, vol. 7, no. 1/2, pp. 73 97, Jan. 2004, doi: 10.1023/b:inrt.0000009441.78971.be.
[1] K. Leilei, Q. Haoliang, W. Shuai, D. Cuixia, W. Suhong, and H. Yong, “Approaches for candidate document retrieval and detailed comparison of plagiarism detection,” 2012.
[13] D. Zubarev and I. Sochenkov, “Cross language text alignment for plagiarism detection based on contextual and context free models,” in Papers from the Annual International Conference “Dialogue,” 2019, pp. 809 820. [14] G. Klein, Y. Kim, Y. Deng, J. Senellart, and A. Rush, “OpenNMT: Open Source Toolkit for Neural Machine Translation,” 2017, doi: 10.18653/v1/p17 4012. [15] J. Devlin, C. Ming Wei, K. Lee, and K. Toutanova, “(BERT): Pre training of deep bidirectional transformers for language understanding,” in Proceedings of the 2019 Conference of the North American Chapter of the Association for Computational Linguistics: Human Language Technologies, 2018, pp. 4171 4186.
[10] M. Franco Salvador, P. Gupta, and P. Rosso, “Cross Language Plagiarism Detection Using a Multilingual Semantic Network,” in Lecture Notes in Computer Science, Springer Berlin Heidelberg, 2013, pp. 710 713.
[2] A. Vaswani et al., “Attention is all you need,” 2017.
[18] H. Asghari, O. Fatemi, S. Mohtaj, H. Faili, and P. Rosso, “On the use of word embedding for cross language plagiarism detection,” Intelligent Data Analysis, vol. 23, no. 3, pp. 661 680, Apr. 2019, doi: 10.3233/ida 183985.
[22] F. Bond and R. Foster, “Linking and extending an open multilingual wordnet,” in Proceedings of the 51st Annual Meeting of the Association for Computational Linguistics, 2013, pp. 1352 1362.
[6] A. Barron Cedeno, P. Rosso, D. Pinto, and A. Juan, “On cross lingual plagiarism analysis using a statistical model,” 2008.
[16] C. Lo and M. Simard, “Fully Unsupervised Crosslingual Semantic Textual Similarity Metric Based on BERT for Identifying Parallel Data,” in Proceedings of the 23rd Conference on Computational Natural Language Learning (CoNLL), 2019, pp. 206 215, doi: 10.18653/v1/k19 1020.
ISSN: 2252 8938 Int J Artif Intell, Vol. 11, No. 3, September 2022: 905 915 914graph dependencies. To build knowledge graphs, we used extended open multilingual wordnet since it provides a wide set of concepts and languages to date. We then constructed a knowledge graph that represents the semantic context of the text segment, by creating the graph with intermediate edges and vertices. The next step was to represent the graphs by weighting all concepts (entities) and semantic relations, by using our graph transformer based on the attention mechanism. The mappings between the graphs are learned both in semi supervised and unsupervised training mechanisms. After the graph representation step, we explain more in detail the framework for cross lingual plagiarism detection as well as the post processing analysis of similarities between text segments. To measure the efficiency of our methods, we compare our CL GTA for plagiarism detection model with multiple states of the art approaches in the task of cross lingual plagiarism detection. We used as evaluation metrics the scores: precision, recall, granularity, and plagdet. The experimental results show that the use of the graph transformer mechanism provided our model with state of the art performance on the Spanish English, French English, and Arabic English pairs. The experiments also demonstrated its advantage with cross language paraphrasing cases for the Spanish English and Arabic English pairs. For future work, we will further improve the model to reaches the state of the art on the Arabic English literal translated cases, we will as expand the experiment to cover more languages and continue exploring the use of our proposed graph transformer and multilingual Knowledge graphs for other cross lingual similarity tasks such as multilingual text classification and cross lingual information retrieval.
El Habib Benlahmar is a Full Professor of Higher Education in the Department of Mathematics and Computer Science at Faculty of Science Ben M'Sik, Hassan II University, Casablanca, Morocco since 2008. He received his PhD in Computer Science from the National school For Computer Science (ENSIAS), Rabat, Morocco, in 2007. His research interests span Web semantic, Natural Language Processing, Information Retrieval, Mobile platforms, and Data Science. He is the author of over 70 research studies published in national and international journals, as well as conference proceedings and book chapters. He can be contacted by email: h.benlahmer@gmail.com.
[33] J. Tiedemann, “Parallel data, tools and interfaces in opus,” Lrec, pp. 2214 2218, 2012.
[35] S. Alzahrani and H. Aljuaid, “Identifying cross lingual plagiarism using rich semantic features and deep neural networks: A study on Arabic English plagiarism cases,” Journal of King Saud University Computer and Information Sciences, vol. 34, no. 4, pp. 1110 1123, Apr. 2022, doi: 10.1016/j.jksuci.2020.04.009.
[34] K. Alotaibi, “The Relationship Between Self Regulated Learning and Academic Achievement for a Sample of Community College Students at King Saud University,” Education Journal, vol. 6, no. 1, p. 28, 2017, doi: 10.11648/j.edu.20170601.14.
Int J Artif Intell ISSN: 2252 8938 Graph transformer for cross lingual plagiarism detection (Oumaima Hourrane) 915
BIOGRAPHIES OF AUTHORS Oumaima Hourrane is a PhD candidate in the Department of Mathematics and Computer Science at Faculty of Science Ben M'Sik, Hassan II University, Casablanca, Morocco. She majored in Computer Engineering in her master study at the National School of Applied Science, Safi, Morocco in 2016. Her research interests include Machine Learning, Artificial Intelligence, Natural Language Processing, and Information Retrieval. She has published multiple research papers in national and international journals as well as conference proceedings. She can be contacted by email: oumaima.hourrane@gmail.com.
[36] A. Barrón Cedeño, M. Potthast, P. Rosso, B. Stein, and A. Eiselt, “Corpus and evaluation measures for automatic plagiarism detection,” 2010. [37] D. P. Kingma and J. L. Ba, “Adam: A method for stochastic optimization,” 2015. [38] A. Barr´on Cede˜no, P. Rosso, E. Agirre, and G. Labaka, “Plagiarism detection across distant language pairs,” in Proceedings of the 23rd International Conference on Computational Linguistics (Coling 2010), 2010, pp. 37 45. [39] M. Franco Salvador, P. Rosso, and M. Montes y Gómez, “A systematic study of knowledge graph analysis for cross language plagiarism detection,” Information Processing & Management, vol. 52, no. 4, pp. 550 570, Jul. 2016, doi: 10.1016/j.ipm.2015.12.004. [40] B. Pouliquen, R. Steinberger, and C. Ignat, “Automatic identification of document translations in large multilingual document collections,” Proceedings of the International Conference Recent Advances in Natural Language Processing (RANLP’03), pp. 401 408, 2006.
[26] K. Cho et al., “Learning Phrase Representations using RNN Encoder Decoder for Statistical Machine Translation,” 2014, doi: 10.3115/v1/d14 1179. [27] P. Gupta, A. Barrón Cedeño, and P. Rosso, “Cross Language High Similarity Search Using a Conceptual Thesaurus,” in Information Access Evaluation. Multilinguality, Multimodality, and Visual Analytics, Springer Berlin Heidelberg, 2012, pp. 67 75. [28] M. Potthast, B. Stein, A. B. on C. no, and P. Rosso, “An evaluation framework for plagiarism detection,” in COLING 2010, 23rd International Conference on Computational Linguistics, 2010, pp. 997 1005. [29] P. Koehn, “Europarl: A parallel corpus for statistical machine translation,” in Proceedings of Machine Translation Summit X: Papers, 2005, pp. 79 86. [30] R. Steinberger et al., “The JRC Acquis: A multilingual aligned parallel corpus with 20+ languages,” 2006. [31] J. Ferrero, L. Besacier, D. Schwab, and F. Agnès, “Deep Investigation of Cross Language Plagiarism Detection Methods,” 2017, doi: 10.18653/v1/w17 2502. [32] M. Ziemski, M. Junczys Dowmunt, and B. Pouliquen, “The united nations parallel corpus v1. 0,” Proceedings of the Tenth International Conference on Language Resources and Evaluation (LREC’16), pp. 3530 3534, 2016.
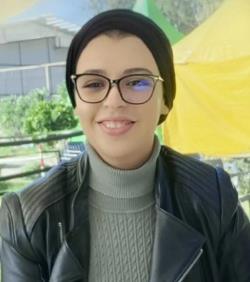



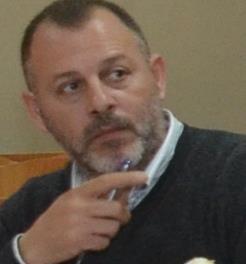



A new approach to solve the of maximum constraint satisfaction problem
Article history: Received Oct 28. 2021 Revised Apr 1, 2022 Accepted Apr 25, 2022 The premature convergence of the simulated annealing algorithm, to solve many complex problems of artificial intelligence, refers to a failure mode where the process stops at a stable point that does not represent to an overall solution. Accelerating the speed of convergence and avoiding local solutions is the concern of this work. To overcome this weakness in order to improve the performance of the solution, a new hybrid approach is proposed. The new approach is able to take into consideration the state of the system during convergence via the use of Hopfield neural networks. To implement the proposed approach, the problem of maximum constraint satisfaction is modeled as a quadratic programming. This problem is solved via the use of the new approach. The approach is compared with other methods to show the effectiveness of the proposed approach.
Mohammed El Alaoui, Mohamed Ettaouil Laboratory for Modelling and Scientific Computing, Sidi Mohamed ben Abdellah University, Fez, Morocco Article Info ABSTRACT
Journal homepage: http://ijai.iaescore.com
Constraint programming is closely related to constraint satisfaction theory, which offers a simple formal scheme for representing and solving combinatorial problems of artificial intelligence [1]. Among the tasks solved by constraint programming: checking electronic circuits, calendar planning, schedule planning, as well as many combinatorial tasks [2] [4]. Constraint programming is a programming paradigm in which relationships between variables are specified in the form of constraints. Formally, the maximum constraint satisfaction problem (Max CSP) is defined by a set of variables which are linked by a set of constraints following a domain of definition for each variable. Max CSP solution is an instantiation to satisfy the maximum of constraints [5]. The concept introduction of Max CSP leads to extensive research for choosing an appropriate resolution method. In addition, exact methods require very high computational time due to the size and complexity of the problem. Whereas approximate methods are necessary for the mission to find an instantiation for the maximum constraint satisfaction problem. The simulated annealing algorithm, in recent years, has been used to solve real problems especially optimization problems [6]. The peculiarity of the simulated annealing algorithm lies in its flexibility to adapt with any optimization problem [7]. This makes the simulated annealing algorithm more efficient, faster and easier to program to solve many optimization problems [8] In view of the attention given to the simulated annealing approach to solve many optimization problems, this work adopted this approach as a method of solving Max CSP. The simulated annealing method is able to avoid local minima to find the optimal solution. The optimal choice of a set of parameters like the cooling model, the initial temperature and the final temperature is essential to ensure good
1. INTRODUCTION
Keywords: Constraint satisfaction problem Hopfield neural networks Simulated annealing algorithm
This is an open access article under the CC BY SA license. Corresponding Author: Mohammed El Alaoui Laboratory for Modelling and Scientific Computing, Sidi Mohamed ben Abdellah University Fez, Email:Moroccomd.elalaoui@gmail.com
IAES International Journal of Artificial Intelligence (IJ AI) Vol. 11, No. 3, September 2022, pp. 916 922 ISSN: 2252 8938, DOI: 10.11591/ijai.v11.i3 pp916 922 916
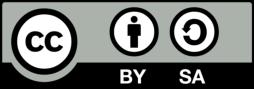
The maximum constraint satisfaction problem is defined by a tuple �� =<��,��,��,�� > such that: �� ={��1,��2, ,����}: Set of �� variables �� ={��(��1),��(��2), ,��(����)}: Set of domains �� ={��1,��2, ,����}: Set of �� constraints ��: objectiveThefunctionbasicidea for solving the maximum constraint satisfaction problem is based on assigning a value to a variable with minimization of number of violated constraints. In this context, a quadratic model under linear constraints is proposed. The modeling phase requires the declaration of the following mathematical notations: ���� is a decision variable, ���� is the size of decision variable ����, ���� is the value assigned to the decision variable ����, and �� is the sum of the size of all variables. The decision variable is defined by (1): ������ = {1 ���� ���� =���� 0 ����ℎ������������ (1) A unique value is selected for each decision variable. This expression is defined by (2): ∑ ������ =1���� ��=1 ∀�� ∈{1,2,…,��} (2) A relation ������ between the variable ���� and the variable ���� makes it possible to define a binary constraint ������. In this modelization, a matrix �� of dimension �� is built starting from the checking of each constraint between the two variables ���� and ����. An element of matrix �� of row �� (i.e. variable ����) and column �� (i.e. variable ����) is defined: ���������� ={1 ����(����,����) ∉������ 0 ����(����,����) ∈������ \ �� ∈{1, ,��},�� ∈{1, ,��} (3) The constraint ������ is expressed: ������ =∑ ∑ ���������������������� ���� ��=1 ���� ��=1 (4) The objective function is defined: ��(��)=∑ ∑ ∑ ∑ ���������������������� ���� ��=1 ���� ��=1 �� ��=1 �� ��=1 (5)
Int J Artif Intell ISSN: 2252 8938 A new approach to solve the of maximum constraint satisfaction problem (Mohammed El Alaoui) 917 convergence. The limitation of the simulated annealing method lies in the inability of the method to take into account the behavior of the problem during convergence. In contrast, Hopfields neural network has proven its ability in the field of machine learning. In this article, we propose a hybrid approach for solving maximum constraint satisfaction problems. The idea is to improve the simulated annealing algorithm in order to build a powerful system that can be adapted with any type of quadratic problem. To achieve this goal, we adopt the Hopfield network which is capable of taking the system state upon convergence to the simulated annealing algorithm to avoid the local solution. In order to perfect the proposed approach, three cooling models are used for the simulated annealing algorithm.This work is structured in five sections. The section 2 presents a quadratic model for the maximum constraint satisfaction problem. The section 3 describes the hybrid approach which combines the neural network and simulated annealing. The section 4 allows to implement the proposed approach to solve the Max CSP. The section 5 gives a conclusion and proposes an alternative avenue of research on another field of application.
2. MODELLING OF MAX CSP
The constraint satisfaction problem can be defined as a network of variables that are related to each other. In this network, the assignment of a value to a variable with the satisfaction of all the constraints between each pair of variables is necessary to find a solution for the constraint satisfaction problem [9]. In some cases, satisfaction of all constraints is impossible given the complexity and size of the problem [10]. To deal with this problem, reducing the number of violated constraints is necessary as a partial solution [11]. This paradigm is known in the literature by the maximum constraint satisfaction problem. The Max CSP consists in assigning a value to a variable for the entire network with the maximum constraint satisfaction [12]. More formally, the maximum constraint satisfaction problem is a form of model that is represented by a set of variables and a set of constraints. The aim of this work is to study the binary constraints of Max CSP.
3. THE PROPOSED MODEL SOLVED BY NEW APPROACH
The matrix �� is a symmetric matrix of dimension ���� that represents the relationship between the decision variables. The matrix �� is of dimension ����, which represents the linear constraint. The vector �� is of dimension ��. To solve this proposed model of Max CSP, a new approach is proposed to solve it.
ISSN: 2252 8938 Int J Artif Intell, Vol. 11, No. 3, September 2022: 916 922 918The matrix form of the objective function ��(��) is expressed ��(��)=�������� (6)
3.1. Simulated annealing
This section gives a representation of a hybrid approach that combines the simulated annealing method and the Hopfield neural network to solve the maximum constraint satisfaction problems. In the subsection of the simulated annealing approach, different cooling models are represented. Then Hopfield's neural network is represented as an adaptive approach to solving any quadratic problem. The last subsection presents a new hybrid approach that combines simulated annealing and the Hopfield neural network. First, a detailed description of the simulated annealing approach is shown in the next subsection.
The simulated annealing method mimics the physical phenomenon of crystallization [13] [16]
��
The application of the simulated annealing method requires the use of a good cooling model to reduce the temperature of the energy function [17] [19]. Controlled cooling makes it possible to switch from a high energy level to a low energy level. In the simulated annealing algorithm, choosing a good cooling model is important for better convergence. The following subsection describes the cooling models used in this Simulatedwork.
annealing algorithm pseudocode Simulated annealing(��������,��������, ����) ��≔�� ���� ≔�������� ���������� ≔���� While ���� >�������� do ����������:=������������(���� ��) ∆��:=��(����������) ��(���� ��) If ��(∆��,����)≥������������(��,��) then ����:=���������� Else ���� ≔���� �� End if If ��(����)<��(����������) then ���������� ≔���� End if ��≔��+�� ����+�� ≔����������(����) Return ����������
Crystallization is an operation that allows a substance to transition from a liquid phase to a solid phase. This process was used in the simulated annealing method to solve an optimization problem. The operation of the simulated annealing method is related to a set comprises the initial temperature, final temperature and cooling model. Controlled cooling participates to ensure good convergence. The following notations are used for the simulated annealing method: �� is a possible solution, ��(��) is an energy function, �������� ihe maximum temperature, �������� ihe minimum temperature, ������������ is a random transformation function, ������������ function generating a new state, and �� is the transition probability defined by the following expression: ��(∆E,T)={1 ∆E≤0 ∆E ���� ∆E>0
The Max CSP problem is modeled as a new quadratic programming, which constitutes an objective function subjected to a linear constraint. (����)= { ��������(��)= 1 2 �������� ������������������������������ ���� =�� �� ∈{0,1}�� (7)
The Lundy Mees model is temperature cooling technique described by the following formula ����+1 =��×(1+������) 1 [22]. The parameter �� is defined by the following relation: �� =(��0 ����)× (�� ��0 ����) 1 . The parameter ��0 represents the initial temperature, the parameter ���� represents the final temperature, and the parameter �� is the number of iterations.
The logarithmic model was first proposed by Geman and Geman by the following formula : ����+1 = ��×(������(��+1)) 1 [21]. The logarithmic model proposes a relationship between the initial temperature and the final temperature. The temperature decreases in two phases: the first phase marks a rapid change in temperature in only a few first iterations. The second phase is characterized by a very slow change in temperature. Therefore, the convergence of this model is very slow and this requires considerable computation time. 3.1.3. Logarithmic model
3.2. Continuous hopfield network Physicist John Hopfield proposed the Hopfield model in 1982, it was a major breakthrough in the field of neural networks [23]. The Hopfield model not only allows to function as associative memory to help object recognition in image processing domain but also it is able to solve a lot of optimization problem such as the problem of installing a surveillance camera, the traveling salesman and the problems of maximum satisfaction of constraints [24], [25]. Due to the great use of this model, it has become the center of attraction for many researchers. Hopfields neural network is a fully connected network [26]. More formally, it is represented by a symmetrical matrix to guarantee the stability of this network. The Hopfield neural network is composed of n interconnected neurons [27]. The dynamics of the Hopfield neural network is described by the following differential (8): ���� ���� = �� �� +����+���� (8) The vector �� =(����) is the input vector of neurons and �� =(����) is the output vector of neurons with 1≤�� ≤�� and ���� ∈{0,1}. The weight matrix is given by �� =(����,��) and ���� is the neuron bias. The hyperbolic function is used to calculate the output of each neuron. Neuron output is expressed: ���� = 1 2(1+tanh(���� ��0)), ��0 >0 (9) where ��0 is a parameter used to control the gain of the activation function. Hopfield proved that the symmetry of the zero diagonal matrix �� is a sufficient condition for the existence of the Lyapunov function [28]. Therefore, the existence of the equilibrium point is guaranteed [29]. Continuous Hopfield networks are capable of solving combinatorial problems that have an energy function taking the following form: { ��������(��)= 1 2 �������� ������������������������������ ���� =�� �� ∈{0,1}�� (10) 3.3. Proposed hybrid approach A hybrid algorithm consists of combining two or more different algorithms in order to arrive at an optimal solution. One of the objectives achieved in this work is to propose a quadratic model for the problem of maximum constraint satisfaction and to solve this model via a robust hybrid algorithm. This hybrid algorithm is a combination of two different approaches: the Hopfield neural network and the simulated annealing algorithm. Hopfield's neural network methodology has been widely used in optimization problems since their arrival. In this work, the Hopfield network was adopted to improve the convergence of the simulated annealing algorithm. This section presents a hybrid algorithm that can solve different problems of maximum constraint satisfaction.
3.1.1. Geometric model
Int J Artif Intell ISSN: 2252 8938
The geometric cooling model is inspired by an arithmetic geometric sequence in which each term makes it possible to deduce the next by multiplication by a constant factor [20]. This model is defined by the relation ���� =������ 1 +��. The factor �� is selected from the interval [0.1]. The relation is an arithmetic sequence when �� =1 and is a geometric sequence when �� =0. Therefore, the parameter �� must be different from 0 and 1. 3.1.2. Logarithmic model
A new approach to solve the of maximum constraint satisfaction problem (Mohammed El Alaoui) 919
50403020100
4.1. Experiments with scens instance
ISSN: 2252 8938 Int J Artif Intell, Vol. 11, No. 3, September 2022: 916 922 920 Hybrid algorithm ��≔�� ��≔�� �� ���� ≔�������� ���������� ≔���� While ���� >�������� do ����������:=������������(���� ��) ∆��:=��(����������) ��(���� ��) If ��(����)<��(���� ��) then ���� ≔���� �� +����(���� ��) End if If ��(∆��,����)≥������������(��,��) then ����:=���������� Else ���� ≔���� �� End if ��≔��+�� ����+�� ≔����������(����) Return ���������� 4. RESULTS AND DISCUSSION
The scens instance represents that are used to compare the proposed approach with other methods. Figure 1(a) shows the average execution time for instance scenes with a fixed number of variables that is equal to 100 and a number of constraints varies between 1,178 and 1,222. Figure 1(b) shows the same instance scens but relatively large with a number of variables ranging from 82 to 458 and with a number of constraints varying from 382 to 5,286. The hybrid approach that combines the Hopfield neural network and simulated annealing with the Lundy cooling model (HA+ Lundy) has proven to be the most robust solver in terms of quality and runtime.(a) (b)
The proposed approach that combines simulated annealing and the Hopfield neural network is used as a solver for the maximum constraint satisfaction problem. To assess the effectiveness of the proposed approach, a series of instances that represent real problems is used in this work. In this section, the basic simulated annealing algorithm is used to solve the maximum constraint satisfaction problem. In addition, the proposed approach is also used to carry out the research process to ensure good convergence. This section presents the different instances (scens, CNF) used to evaluate the performance of the proposed approach. Software and hardware prerequisites are required to implement the proposed approach. Instances are run on a 3.0 GHs processor desktop and 4 GB RAM. The proposed algorithm is programmed through the use of Java object oriented programming language. Given the stochastic nature of the proposed approach (the complexity of the algorithm and the structure of the test instance), the experiment was carried out 30 times. When implementing the proposed approach, a number of parameters can help with good convergence. These parameters are determined through preliminary experiment. The preliminary experiment made it possible to set the value of �� and �� at 0.99 and 3.5 respectively.
Figure 1. Instance scens (a) number of variable is fixed at 100 and (b) number of variables beetwen 82 and 458 151050 20 25 1178 1203 1210 1215 1221 1222 Time(s) number of constraints HA+Lundy HA+geometric HA+logarithmic Simulated annealing 82 197 200 201 458 Time(s) number of variables HA+Lundy HA+geometric HA+logarithmic Simulated annealing
In this experiment, the conjunctive normal form (CNF) instance is used to evaluate the performance of the proposed approach. The first step in this experiment is to extract the data from extensible markup language (XML) file. The second step is to represent the relationships that lie between the variables in a decision function that evaluate the maximum constraint satisfaction problem. Figure 2(a) shows the average execution time of the CNF instance with a number of variables fixed at 40. Figure 2(b) shows the average execution time of the CNF instance with a number of variables fixed at 80. (a) (b) Figure 2. CNF instance (a) number of variable is fixed at 40 and (b) number of variable is fixed at 80
4.2. Experiments with CNF instance
[4] D. Steurer, “Fast SDP algorithms for constraint satisfaction problems,” in Proceedings of the Twenty First Annual ACM SIAM Symposium on Discrete Algorithms, Jan. 2010, pp. 684 697, doi: 10.1137/1.9781611973075.56.
Int J Artif Intell ISSN: 2252 8938 A new approach to solve the of maximum constraint satisfaction problem (Mohammed El Alaoui) 921
[7] I. Boussaïd, J. Lepagnot, and P. Siarry, “A survey on optimization metaheuristics,” Information Sciences, vol. 237, pp. 82 117, Jul. 2013, doi: 10.1016/j.ins.2013.02.041.
[9] M. Charikar, K. Makarychev, and Y. Makarychev, “Near optimal algorithms for maximum constraint satisfaction problems,” in Proceedings of the Annual ACM SIAM Symposium on Discrete Algorithms, 2007, pp. 62 68. 10 2 76543 660 668 673 674 676 677 678 680 683 685 686 687 688 693 694 700 Time(s) number of constraints HA+Lundy HA+geometric HA+logarithmic Simulated annealing 210 3 7654 926 927 933 934 937 946 947 989 1001 1012 1066 1068 1088 1102 1132 1139 1175 1210 1260 1428 1485 1496 Time(s) number of constraints HA+Lundy HA+geometric HA+logarithmic Simulated annealing
REFERENCES
[3] G. Dozier, J. Bowen, and A. A. Homaifar, “Solving constraint satisfaction problems using hybrid evolutionary search,” IEEE Transactions on Evolutionary Computation, vol. 2, no. 1, pp. 23 33, Apr. 1998, doi: 10.1109/4235.728211.
[2] V. Kumar, “Algorithms for constraint satisfaction problems: a survey,” AI Magazine, vol. 13, no. 1, pp. 32 44, 1992
[5] M. C. Cooper, “High order consistency in valued constraint satisfaction,” Constraints, vol. 10, no. 3, pp. 283 305, Jul. 2005, doi: 10.1007/s10601 005 2240 3. [6] T. Dokeroglu, E. Sevinc, T. Kucukyilmaz, and A. Cosar, “A survey on new generation metaheuristic algorithms,” Computers and Industrial Engineering, vol. 137, Nov. 2019, doi: 10.1016/j.cie.2019.106040.
[8] K. Hussain, M. N. Mohd Salleh, S. Cheng, and Y. Shi, “Metaheuristic research: a comprehensive survey,” Artificial Intelligence Review, vol. 52, no. 4, pp. 2191 2233, Dec. 2019, doi: 10.1007/s10462 017 9605 z.
5. CONCLUSION Hopfield's neural network was used in this work to improve the simulated annealing algorithm. This makes it possible to build a new hybrid approach. Hopfield's neural network is a robust algorithm that takes into account the previous information to improve the direction of the algorithm towards a better solution. The simulated annealing is fed by Hopfield's neural network during the research process. The proposed approach gives better results comparing with other conventional methods. The proposed approach has made it possible to solve the scens instance of variable number between 82 and 458 in a better execution time. And also allows to solve the CNF instance of variable number is set to 40 and to 80 in a better execution time better compared to other approaches. Future research should attempt to model and solve the quadratic model associated with the query optimization problem in databases.
[1] K. Hirayama and M. Yokoo, “Distributed partial constraint satisfaction problem,” in Lecture Notes in Computer Science (including subseries Lecture Notes in Artificial Intelligence and Lecture Notes in Bioinformatics), vol. 1330, 1997, pp. 222 236, doi: 10.1007/BFb0017442.
[29] P. M. Talaván and J. Yáñez, “A continuous Hopfield network equilibrium points algorithm,” Computers and Operations Research, vol. 32, no. 8, pp. 2179 2196, Aug. 2005, doi: 10.1016/j.cor.2004.02.008.
[25] K. Cheng, J. Lin, and C. Mao, “The application of competitive Hopfield neural network to medical image segmentation,” IEEE Transactions on Medical Imaging, vol. 15, no. 4, pp. 560 567, Aug. 1996, doi: 10.1109/42.511759.
BIOGRAPHIES OF AUTHORS Mohammed El Alaoui is a Doctorate Status in applied computer sciences and mathematics from the Laboratory of Modeling and Scientific computing at the Faculty of Sciences and Technology of Fez, Morocco. He is a member of Artificial intelligence for engineering sciences group in the Laboratory of mathematical modeling, Operational Research and computer sciences. He works on Neural Network, Artificial Intelligence, classification problems, Database, constraint satisfaction problem and machine learning and Data warehouse. He can be contacted at email: md.elalaoui@gmail.com Mohamed Ettaouil is a Doctorate Status in Operational Research and Optimization, FST University Sidi Mohamed Ben Abdellah USMBA, Fez. Ph.D. in Computer Science, University of Paris 13, Galilee Institute, Paris France. He is a professor at the Faculty of Science and technology of Fez FST, and he was responsible for research team in modelization and pattern recognition, operational research and global optimization methods. He was the Director of Unit Formation and Research UFR: Scientific computing and computer science, Engineering Sciences. He is also a responsible for research team in Artificial Neural Networks and Learning, modelization and engineering sciences, FST Fez. He is an expert in the fields of the modelization and optimization, engineering sciences. He can be contacted at email: mohamedettaouil@yahoo.fr.
ISSN: 2252 8938 Int J Artif Intell, Vol. 11, No. 3, September 2022: 916 922 922 [10] M. Ettaouil and C. Loqman, “Constraint satisfaction problems solved by semidefinite relaxations,” WSEAS Transactions on Computers, vol. 7, no. 7, pp. 951 961, 2008 [11] M. Ettaouil, C. Loqman, K. Haddouch, and Y. Hami, “Maximal constraint satisfaction problems solved by continuous hopfield networks,” WSEAS Transactions on Computers, vol. 12, no. 2, pp. 29 40, 2013 [12] J. Larrosa and P. Meseguer, “Optimization based heuristics for maximal constraint satisfaction,” in Lecture Notes in Computer Science (including subseries Lecture Notes in Artificial Intelligence and Lecture Notes in Bioinformatics), vol. 976, 1995, pp. 103 120, doi: 10.1007/3 540 60299 2_7. [13] A. Franzin and T. Stützle, “Revisiting simulated annealing: a component based analysis,” Computers and Operations Research, vol. 104, pp. 191 206, Apr. 2019, doi: 10.1016/j.cor.2018.12.015.
[17] A. K. Peprah, S. K. Appiah, and S. K. Amponsah, “An optimal cooling schedule using a simulated annealing based approach,” Applied Mathematics, vol. 8, no. 8, pp. 1195 1210, 2017, doi: 10.4236/am.2017.88090. [18] S. Parthasarathy and C. Rajendran, “A simulated annealing heuristic for scheduling to minimize mean weighted tardiness in a flowshop with sequence dependent setup times of jobs a case study,” Production Planning and Control, vol. 8, no. 5, pp. 475 483, Jan. 1997, doi: 10.1080/095372897235055. [19] D. Bertsimas and J. Tsitsiklis, “Simulated annealing,” Statistical Science, vol. 8, no. 1, pp. 10 15, Feb. 1993, doi: 10.1214/ss/1177011077. [20] D. Henderson, S. H. Jacobson, and A. W. Johnson, “The theory and practice of simulated annealing,” in Handbook of Metaheuristics, Boston: Kluwer Academic Publishers, 2006, pp. 287 319, doi: 10.1007/0 306 48056 5_10.
[24] A. Maurer, M. Hersch, and A. G. Billard, “Extended hopfield network for sequence learning: application to gesture recognition,” in Lecture Notes in Computer Science (including subseries Lecture Notes in Artificial Intelligence and Lecture Notes in Bioinformatics), vol. 3696, 2005, pp. 493 498, doi: 10.1007/11550822_77.
[26] M. El Alaoui, K. El Moutaouakil, and M. Ettaouil, “A multi step method to calculate the equilibrium point of the continuous hopfield networks: application to the max stable problem,” WSEAS Transactions on Systems and Control, vol. 12, pp. 418 425, 2017 [27] J. J. Hopfield, “Neural networks and physical systems with emergent collective computational abilities,” in Proceedings of the National Academy of Sciences, Apr. 1982, vol. 79, no. 8, pp. 2554 2558, doi: 10.1073/pnas.79.8.2554.
[28] I. Goldhirsch, P. L. Sulem, and S. A. Orszag, “Stability and Lyapunov stability of dynamical systems: a differential approach and a numerical method,” Physica D: Nonlinear Phenomena, vol. 27, no. 3, pp. 311 337, Aug. 1987, doi: 10.1016/0167 2789(87)90034 0.
[21] D. Long, J. Viovy, and A. Ajdari, “My IOPscience,” Journal of Physics. Condensed Matter, vol. 8, 1996 [22] M. Lundy and A. Mees, “Convergence of an annealing algorithm,” Mathematical Programming, vol. 34, no. 1, pp. 111 124, Jan. 1986, doi: 10.1007/BF01582166. [23] J. J. Hopfield and D. W. Tank, “‘Neural’ computation of decisions in optimization problems,” Biological Cybernetics, vol. 52, no. 3, pp. 141 152, Jul. 1985, doi: 10.1007/BF00339943.
[14] D. Abramson, M. Krishnamoorthy, and H. Dang, “Simulated annealing cooling schedules for the school timetabling problem,” Asia Pacific Journal of Operational Research, vol. 16, no. 1, pp. 1 22, 1999 [15] B. Ceranic, C. Fryer, and R. W. Baines, “An application of simulated annealing to the optimum design of reinforced concrete retaining structures,” Computers and Structures, vol. 79, no. 17, pp. 1569 1581, Jul. 2001, doi: 10.1016/S0045 7949(01)00037 2. [16] M. F. Cardoso, R. L. Salcedo, and S. F. de Azevedo, “Nonequilibrium simulated annealing: a faster approach to combinatorial minimization,” Industrial and Engineering Chemistry Research, vol. 33, no. 8, pp. 1908 1918, Aug. 1994, doi: 10.1021/ie00032a005.
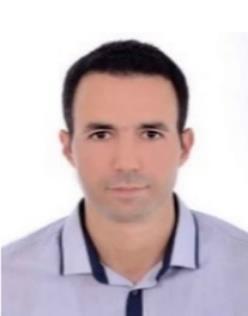



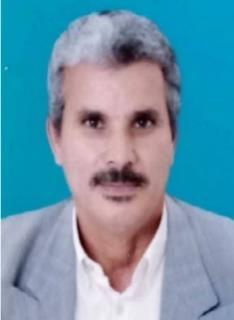



Article Info ABSTRACT
Corresponding Author: Nazrul IntelligentEffendyandEmbedded
Journal homepage: http://ijai.iaescore.com
System Research Group, Department of Nuclear Engineering and Engineering Physics, Faculty of Engineering, Universitas Gadjah Mada Jl. Grafika 2, Yogyakarta, Indonesia Email: nazrul@ugm.ac.id 1. INTRODUCTION
Article history: Received Sep 25, 2021 Revised Apr 7, 2022 Accepted Apr 21, 2022 The oxygen content of the gas fired boiler flue gas is used to monitor boiler combustion efficiency. Conventionally, this oxygen content is measured using an oxygen content sensor. However, because it operates in extreme conditions, this oxygen sensor tends to have the disadvantage of high maintenance costs. In addition, the absence of other sensors as an element of redundancy and when there is damage to the sensor causes manual handling by workers. It is dangerous for these workers, considering environmental conditions with high risk hazards. We propose an artificial neural network (ANN) and random forest based soft sensor to predict the oxygen content to overcome the problems. The prediction is made by utilizing measured data on the power plant’s boiler, consisting of 19 process variables from a distributed control system. The research has proved that the proposed soft sensor successfully predicts the oxygen content. Research using random forest shows better performance results than ANN. The random forest prediction errors are mean absolute error (MAE) of 0.0486, mean squared error (MSE) of 0.0052, root mean square error (RMSE) of 0.0718, and Std Error of 0.0719. While the errors using ANN are MAE of 0.0715, MSE of 0.0087, RMSE of 0.0935, and Std Error of 0.0935.
Nazrul Effendy1, Eko David Kurniawan1, Kenny Dwiantoro1, Agus Arif1, Nidlom Muddin2 1Intelligent and Embedded System Research Group, Department of Nuclear Engineering and Engineering Physics, Faculty of Engineering, Universitas Gadjah Mada, Yogyakarta, Indonesia 2Project Development Division, PT. Pertamina (Persero), Jakarta, Indonesia
The prediction of the oxygen content of the flue gas in a gasfired boiler system using neural networks and random forest
A steam boiler system is a closed vessel that uses fuel or electricity to generate steam to supply the power plant system [1] [3]. The energy produced by the boiler in the form of heat comes from the combustion process in the combustion chamber or furnace. In general, the combustion reaction in the boiler furnace can be made using three main components, namely fuel, air, and fire from the lighter. The combustion efficiency in the boiler furnace describes the ability of a burner to burn the entire fuel entering the furnace. The ideal furnace combustion reaction occurs when the oxygen in the air is sufficient to burn the whole fuel [4] [6]. So, there is no remaining oxygen or energy in the flue gas. However, the oxygen volume will be insufficient to burn all the fuel when there is an imperfect mixture of fuel and oxygen. Therefore, it is necessary to have an appropriate combination of the required amount of energy and oxygen. In addition, more fresh air is needed to burn the entire fuel. This air is known as excess air. There is still oxygen content in the flue gas in combustion with excess air. Therefore, the amount of unburned fuel and the remaining oxygen excess air on the flue gas can be utilized to estimate the combustion
IAES International Journal of Artificial Intelligence (IJ AI) Vol. 11, No. 3, September 2022, pp. 923 929 ISSN: 2252 8938, DOI: 10.11591/ijai.v11.i3.pp923 929 923
This is an open access article under the CC BY SA license.
Keywords: Flue SoftRandomPredictionOxygenNeuralgasnetworkscontentforestsensor
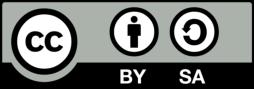
2. RESEARCH METHOD
Figure 1. Diagram of the proposed soft sensor system to predict the oxygen content of the steam boiler flue gas using neural networks and random forest
To overcome the problems, we propose to use a soft sensor using two types of machine learning as the soft sensor: an artificial neural network (ANN) [10] [12] and random forest [13], [14]. The soft sensor is a software based method that utilizes an intelligent system to solve a problem based on the input output relationship [15] [19]. Several researchers have applied machine learning for prediction and pattern recognition as the primary key of the soft sensor [20] [25]. Other researchers proposed the soft sensor to indicate the oxygen content of flue gas using the support vector model and mixed model [26] The rest of this paper is described as follows. First, the research method is described in detail in section 2. Then, section 3 describes the experimental results, including the training and testing of the soft sensor using ANN and random forest. Finally, in section 4, the conclusion of this study is offered.
The optimal oxygen content depends on the type of furnace used. If the oxygen content is too low, unburned fuel will decrease air quality. On the other hand, if the oxygen content is too high, the furnace will be inefficient due to a large amount of energy lost through the flue gas.
The oxygen content of the flue gas of a steam boiler system can be conventionally measured by oxygen sensors, such as using zirconium oxide. Zirconium oxide is a material capable of measuring oxygen levels in flue gas. However, oxygen sensors with zirconium oxide tend to have the disadvantage of high maintenance costs. In addition, the absence of other sensors as an element of redundancy and when there is damage to the sensor causes manual handling by workers using portable measuring devices. It is dangerous for these workers, considering the environmental conditions with high risk hazards.
2.1. Data collection Data from the steam boiler system of a 32 MW power plant in an oil refinery unit, Indonesia, are collected from 1 January until 28 August. The boiler used in this study is a type of water tube boiler used to heat water to become superheated steam. The steam is fed to the steam turbine generator. The generator supplies all power requirements for processing operations at the oil refinery unit. The collected data consisted of 19 parameters, including oxygen content, and was acquired from a distributed control system historical data system. Table 1 lists the process variables of the steam boiler used in the research.
After data collection, the data preprocessing is carried out. The preprocessing consists of several steps: handling missing values, separating training data and test data, and data normalization. The results of the data preprocessing are then used to feed ANN and the random forest system
In this paper, the proposed soft sensor to predict the oxygen content in the steam boiler flue gas is shown in Figure 1. This research was conducted in several stages: data collection, data preprocessing, soft sensor design using ANN and random forest, training, and performance evaluation of the soft sensor. The schematic diagram of a power plant’s boiler is shown in Figure 2 [27]
2.2. Data preprocessing
ISSN: 2252 8938 Int J Artif Intell, Vol. 11, No. 3, September 2022: 923 929 924efficiency [7]. In addition to saving fuel use, high combustion efficiency will also reduce air pollution generated from this combustion process [8], [9].
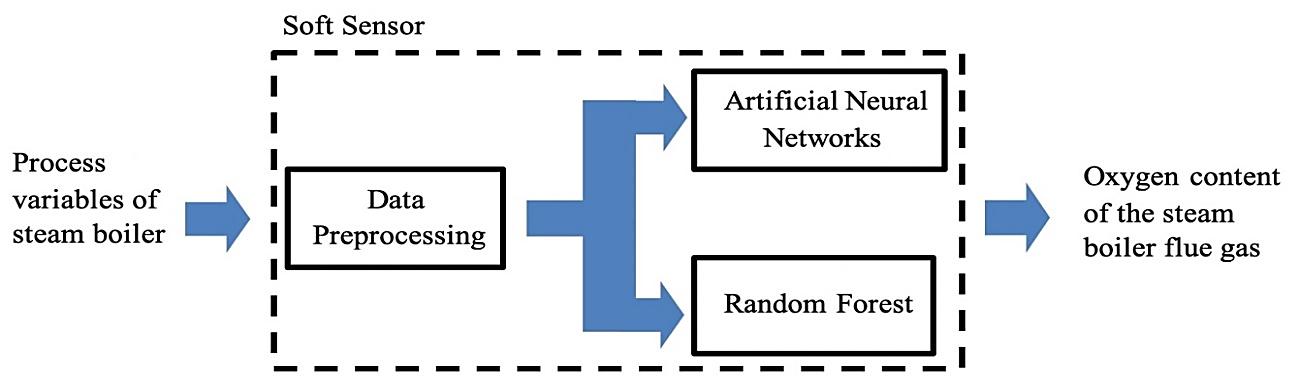
3
16
8
13
Table 1. Process variables of the steam boiler system Process variables Unit 1 Deaerator level mm 2 The feed flow rate of boiler water to the superheater ton/hour The temperature of advanced steam in the superheater C Feedwater flow rate Kg/hour Main gas inlet flow rate to the furnace N.m3/hour Fuel gas pressure behind the control valve Kg/cm2 Combustion air flow rate Kg/ hour Air pressure of burner box mmH2O 9 Main steam temperature C Furnace exhaust gas pressure mmH2O The temperature of boiler Flue gas C 12 Boiler steam pressure kg/cm2 Wind box pressure mmH2O 14 Combustion air temperature C Steam drum boiler levels % Primary steam header flow rate kg/hour The water inlet temperature of the economizer C The water outlet temperature of the economizer C 19 Oxygen content % 2.3. ANN soft sensor
11
15
17
After preprocessing the data, we design an ANN soft sensor. The ANN soft sensor is created using Python3 with libraries of NumPy, pandas, Matplotlib, Keras, and TensorFlow framework [28] [30]. The hyperparameters used in the research are the number of neurons, the number of epochs, feature selection, and the early stopping strategy [31], [32]. The hidden layer consists of various neurons from 4 to 64. We used ReLU as the activation function in the hidden layer and mean squared error (MSE) as the loss function. The optimizer used as the determinant of the learning process is SGD [33] [36]. After ANN soft sensor was designed, it was trained with a certain number of epochs. After the ANN training, the next step is to test the ANN. The test was conducted by comparing the prediction results of ANN with the target.
10
Int J Artif Intell ISSN: 2252 8938 The prediction of the oxygen content of the flue gas in a gas fired boiler system using … (Nazrul Effendy) 925 Figure 2. A schematic diagram of a steam boiler system [26]
5
6
4
No
7
18
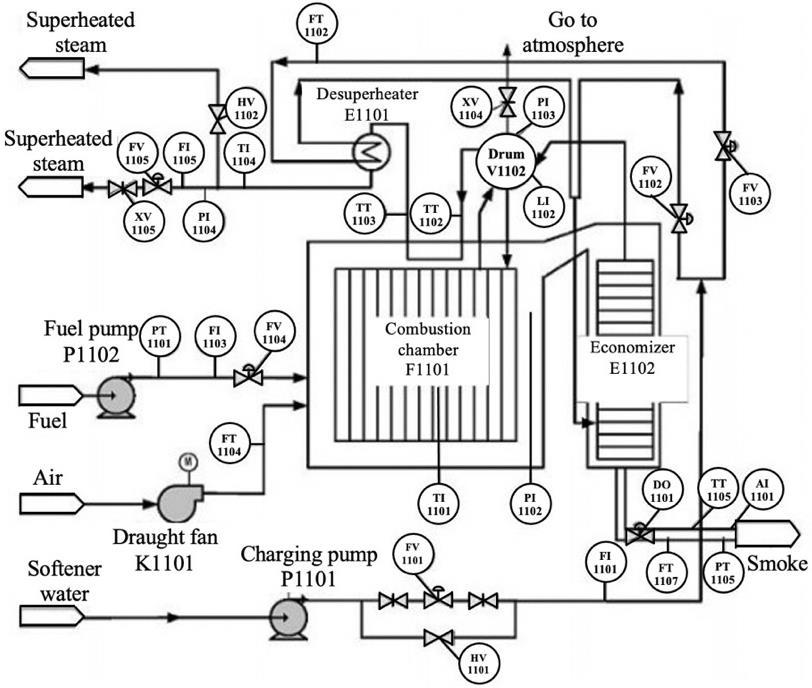
In this study, experiments were also conducted on the design of the oxygen content prediction system using a random forest. Figure 5 shows the system prediction error results compared with the oxygen content prediction system using ANN. The results of this study show that the random forest outperforms the ANN. The random forest prediction errors are MAE of 0.0486, MSE of 0.0052, RMSE of 0.0718, and Std Error of 0.0719. While the errors using ANN are MAE of 0.0715, MSE of 0.0087, RMSE of 0.0935, and Std Error of 0.0935. The model performance can also be investigated through the relationship between the flue gas's predicted and measured oxygen content. The relation of the random forest soft sensor is shown in Figure 6. The predicted and the measured values are almost close to the linear line.
ISSN: 2252 8938 Int J Artif Intell, Vol. 11, No. 3, September 2022: 923 929 926 2.4. Random forest soft sensor Besides using ANN, we also design a soft sensor using a random forest for the oxygen content prediction of the flue gas. The performance of both models is then compared We compare the mean absolute error (MAE), MSE, root mean square error (RMSE), and Std Error of both models.
Figure 3 shows the MAE of the ANN soft sensor with 60 neurons in the hidden layer. MAE in this experiment tends to decrease with increasing epoch. There is a slight increase in validation errors in certain epochs, even though this validation error generally tends to decrease with increasing epochs. The experimental results indicate that this ANN successfully predicts the oxygen content of the steam boiler flue gas. Figure 4 shows the histogram of the prediction error of ANN. The distribution of error is typically distributed at the slightest error. It means that most of the prediction results have a relatively small error.
Figure 4 Histogram of the prediction error of the soft sensor with 60 neurons in the hidden layer
After training with 1,000 epochs, ANN soft sensor performance was evaluated using test data. The experiments show that the best ANN architecture in this research is with 60 neurons in the hidden layer.
Figure 3. MAE of the ANN soft sensor with 60 neurons in the hidden layer
3. RESULTS AND DISCUSSION
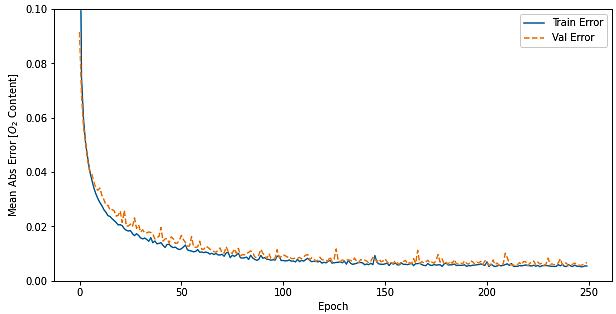
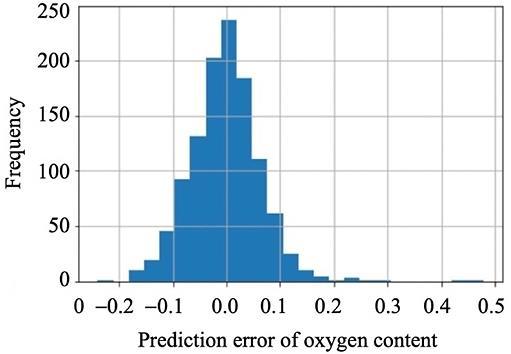
Int J Artif Intell ISSN: 2252 8938 The prediction of the oxygen content of the flue gas in a gas fired boiler system using … (Nazrul Effendy) 927
Figure 5. Comparison of the prediction errors of the soft sensors using artificial neural networks and random forest
Figure 6. The predicted and measured oxygen content of flue gas using a random forest soft sensor system
[4] Y. F. Wang et al., “Fuzzy modeling of boiler efficiency in power plants,” Inf. Sci. (Ny)., vol. 542, pp. 391 405, Jan. 2020, doi: 10.1016/j.ins.2020.06.064.
[3] M. Trojan, “Modeling of a steam boiler operation using the boiler nonlinear mathematical model,” Energy, vol. 175, pp. 1194 1208, May 2019, doi: 10.1016/j.energy.2019.03.160.
ACKNOWLEDGEMENTS
4. CONCLUSIONS In this paper, we propose a soft sensor system to predict the oxygen content of the steam boiler flue gas using ANN and random forest. From the experimental results, this soft sensor system has been proven to be successful in predicting the oxygen content in the flue gas of the steam boiler. The random forest oxygen content prediction system showed better performance than the ANN system. The random forest prediction errors are MAE of 0.0486, MSE of 0.0052, RMSE of 0.0718, and Std Error of 0.0719. While the errors using ANN are MAE of 0.0715, MSE of 0.0087, RMSE of 0.0935, and Std Error of 0.0935.
[1] Y. Camaraza Medina, Y. Retirado Mediaceja, A. Hernandez Guerrero, and J. Luis Luviano Ortiz, “Energy efficiency indicators of the steam boiler in a power plant of Cuba,” Therm. Sci. Eng. Prog., vol. 23, p. 100880, Jun. 2021, doi: 10.1016/j.tsep.2021.100880. [2] P. Madejski and P. Żymełka, “Calculation methods of steam boiler operation factors under varying operating conditions with the use of computational thermodynamic modeling,” Energy, vol. 197, p. 117221, Apr. 2020, doi: 10.1016/j.energy.2020.117221.
[5] J. Luo, L. Wu, and W. Wan, “Optimization of the exhaust gas oxygen content for coal fired power plant boiler,” Energy Procedia, vol. 105, pp. 3262 3268, May 2017, doi: 10.1016/j.egypro.2017.03.730.
[6] Y. Cheng, Y. Huang, B. Pang, and W. Zhang, “ThermalNet: a deep reinforcement learning based combustion optimization system
The authors would like to acknowledge Universitas Gadjah Mada and PT Pertamina for the facilities while conducting this research. Part of this paper was developed using data from a thesis by the third author.
REFERENCES
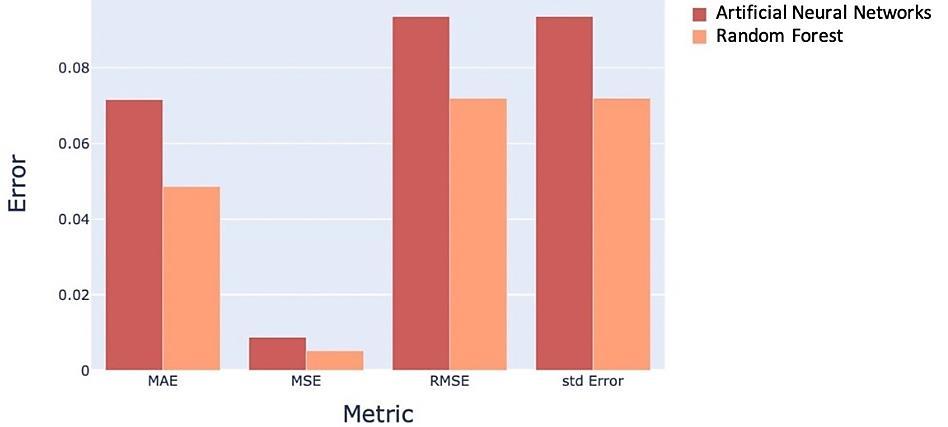
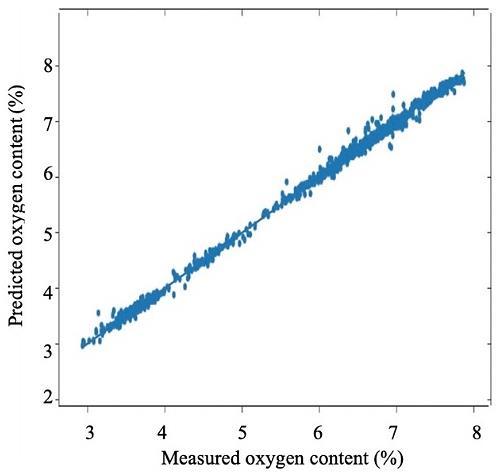
ISSN: 2252 8938 Int J Artif Intell, Vol. 11, No. 3, September 2022: 923 929 928 for coal fired boiler,” Eng. Appl. Artif. Intell., vol. 74, pp. 303 311, Sep. 2018, doi: 10.1016/j.engappai.2018.07.003.
[16] Y. Ye, H. Wang, and X. An, “An indirect online method for measuring the boiling rate of BFG boiler economizer,” Energy Reports, vol. 6, pp. 703 709, Feb. 2020, doi: 10.1016/j.egyr.2019.11.141.
[20] S. Nafisah and N. Effendy, “Voice biometric system: The identification of the severity of cerebral palsy using mel frequencies stochastics approach,” Int. J. Integr. Eng., vol. 11, no. 3, pp. 194 206, Sep. 2019, doi: 10.30880/ijie.2019.11.03.020.
[22] N. Effendy, N. C. Wachidah, B. Achmad, P. Jiwandono, and M. Subekti, “Power estimation of G.A. siwabessy multi purpose reactor at start up condition using artificial neural network with input variation,” in Proceedings 2016 2nd International Conference on Science and Technology Computer, ICST 2016, Oct. 2017, pp. 133 138., doi: 10.1109/ICSTC.2016.7877362.
[33] G. Habib and S. Qureshi, “Optimization and acceleration of convolutional neural networks: A survey,” J. King Saud Univ. Comput. Inf. Sci., Oct. 2020, doi: 10.1016/j.jksuci.2020.10.004. [34] X. Feng, Q. M. J. Wu, Y. Yang, and L. Cao, “A compensation based optimization strategy for top dense layer training,” Neurocomputing, vol. 453, pp. 563 578, Sep. 2021, doi: 10.1016/j.neucom.2020.07.127.
[32] Y. J. Yoo, “Hyperparameter optimization of deep neural network using univariate dynamic encoding algorithm for searches,” Knowledge Based Syst., vol. 178, pp. 74 83, Aug. 2019, doi: 10.1016/j.knosys.2019.04.019.
[35] V. H. Nhu et al., “Effectiveness assessment of Keras based deep learning with different robust optimization algorithms for shallow landslide susceptibility mapping at tropical area,” Catena, vol. 188, p. 104458, May 2020, doi: 10.1016/j.catena.2020.104458. [36] Z. Chang, Y. Zhang, and W. Chen, “Electricity price prediction based on hybrid model of adam optimized LSTM neural network and wavelet transform,” Energy, vol. 187, p. 115804, Nov. 2019, doi: 10.1016/j.energy.2019.07.134.
[15] A. Ramakalyan, A. Sivakumar, C. Aravindan, K. Kannan, V. Swaminathan, and D. Sarala, “Development of KSVGRNN: a hybrid soft computing technique for estimation of boiler flue gas components,” J. Ind. Inf. Integr., vol. 4, pp. 42 51, Dec. 2016, doi: 10.1016/j.jii.2016.09.001.
[17] R. S. Lakshmi, A. Sivakumar, G. Rajaram, V. Swaminathan, and K. Kannan, “A novel hypergraph based feature extraction technique for boiler flue gas components classification using PNN A computational model for boiler flue gas analysis,” J. Ind. Inf. Integr., vol. 9, pp. 35 44, Mar. 2018, doi: 10.1016/j.jii.2017.11.002.
[13] M. B. Nafouanti, J. Li, N. A. Mustapha, P. Uwamungu, and D. AL Alimi, “Prediction on the fluoride contamination in groundwater at the Datong Basin, Northern China: comparison of random forest, logistic regression and artificial neural network,” Appl. Geochemistry, vol. 132, p. 105054, Sep. 2021, doi: 10.1016/j.apgeochem.2021.105054.
[10] P. Tóth, A. Garami, and B. Csordás, “Image based deep neural network prediction of the heat output of a step grate biomass boiler,” Appl. Energy, vol. 200, pp. 155 169, Aug. 2017, doi: 10.1016/j.apenergy.2017.05.080.
[23] N. Effendy, D. Ruhyadi, R. Pratama, D. F. Rabba, A. F. Aulia, and A. Y. Atmadja, “Forest quality assessment based on bird sound recognition using convolutional neural networks,” Int. J. Electr. Comput. Eng., vol. 12, no. 4, pp. 4235 4242, doi: 10.11591/ijece.v12i4.pp4235 4242. [24] R. Y. Galvani, N. Effendy, and A. Kusumawanto, “Evaluating weight priority on green building using fuzzy AHP,” in Proceedings 12th SEATUC Symposium, SEATUC 2018, Mar. 2018, pp. 1 6., doi: 10.1109/SEATUC.2018.8788887.
[25] N. Effendy, E. Maneenoi, P. Charnvivit, and S. Jitapunkul, “Intonation recognition for Indonesian speech based on fujisaki model,” in 8th International Conference on Spoken Language Processing, ICSLP 2004, Oct. 2004, pp. 2973 2976., doi: 10.21437/interspeech.2004 746. [26] S. Lingfang and W. Yechi, “Soft sensing of oxygen content of flue gas based on mixed model,” Energy Procedia, vol. 17, pp. 221 226, 2012, doi: 10.1016/j.egypro.2012.02.087. [27] Z. Dong, L. Xie, and Q. Zhang, “Design of boiler control system based on PCS7 and SMPT 1000,” in Proceedings 2015 7th International Conference on Intelligent Human Machine Systems and Cybernetics, IHMSC 2015, Aug. 2015, vol. 2, pp. 546 550., doi: 10.1109/IHMSC.2015.212. [28] A. Gulli and S. Pal, Deep learning with Keras : implementing deep learning models and neural networks with the power of Python. Packt Publishing, 2017. [29] S. Osah, A. A. Acheampong, C. Fosu, and I. Dadzie, “Deep learning model for predicting daily IGS zenith tropospheric delays in West Africa using TensorFlow and Keras,” Adv. Sp. Res., vol. 68, no. 3, pp. 1243 1262, May 2021, doi: 10.1016/j.asr.2021.04.039. [30] F. Chollet, Deep Learning with Python, 2nd ed. USA: Manning Publications Co., 2021. [31] W. Li, W. W. Y. Ng, T. Wang, M. Pelillo, and S. Kwong, “HELP: an LSTM based approach to hyperparameter exploration in neural network learning,” Neurocomputing, vol. 442, pp. 161 172, Jun. 2021, doi: 10.1016/j.neucom.2020.12.133.
[11] C. C. Aggarwal, “Training deep neural networks,” in Neural Networks and Deep Learning, Cham: Springer International Publishing, 2018, pp. 105 167., doi: 10.1007/978 3 319 94463 0_3.
[19] S. N. Sembodo, N. Effendy, K. Dwiantoro, and N. Muddin, “Radial basis network estimator of oxygen content in the flue gas of debutanizer reboiler,” Int. J. Electr. Comput. Eng., vol. 12, no. 3, pp. 3044 3050, doi: 10.11591/ijece.v12i3.pp3044 3050.
[8] J. Li and W. Li, “Combustion analysis and operation adjustment of thermal power unit,” in Proceedings of the 2015 Asia Pacific Energy Equipment Engineering Research Conference, 2015, vol. 9., doi: 10.2991/ap3er 15.2015.1.
[18] W. Yan, D. Tang, and Y. Lin, “A data driven soft sensor modeling method based on deep learning and its application,” IEEE Trans. Ind. Electron., vol. 64, no. 5, pp. 4237 4245, May 2017, doi: 10.1109/TIE.2016.2622668.
[7] Z. Tang, Y. Li, and A. Kusiak, “A deep learning model for measuring oxygen content of boiler flue gas,” IEEE Access, vol. 8, pp. 12268 12278, 2020, doi: 10.1109/ACCESS.2020.2965199.
[21] N. Effendy, K. Shinoda, S. Furui, and S. Jitapunkul, “Automatic recognition of Indonesian declarative questions and statements using polynomial coefficients of the pitch contours,” Acoust. Sci. Technol., vol. 30, no. 4, pp. 249 256, 2009, doi: 10.1250/ast.30.249.
[12] M. S. Khrisat and Z. A. Alqadi, “Solving multiple linear regression problem using artificial neural network,” Int. J. Electr. Comput. Eng., vol. 12, no. 1, pp. 770 775, Feb. 2022, doi: 10.11591/ijece.v12i1.pp770 775.
[14] M. M. Islam, M. A. Kashem, and J. Uddin, “Fish survival prediction in an aquatic environment using random forest model,” IAES Int. J. Artif. Intell., vol. 10, no. 3, pp. 614 622, Sep. 2021, doi: 10.11591/ijai.v10.i3.pp614 622.
[9] Y. Ding, J. Liu, J. Xiong, M. Jiang, and Y. Shi, “Optimizing boiler control in real time with machine learning for sustainability,” in Proceedings of the 27th ACM International Conference on Information and Knowledge Management, Oct. 2018, pp. 2147 2154., doi: 10.1145/3269206.3272024.
Nidlom Muddin received the B.Sc. Degree in Organic Chemistry from Universitas Sebelas Maret in 2008. Currently, he is a Sr. Analyst of the New Refinery Project for PT. Kilang Pertamina International. He is interested in conducting research in an area related to energy management systems, especially boiler and furnace operation. He proposed a standard boiler and furnace assessment method and periodically conducted boiler and furnace optimization training. He can be contacted at email: nidlom.muddin@pertamina.com.
Agus Arif received the B S degree in nuclear engineering from Universitas Gadjah Mada, Indonesia, in 1991 and the M.Eng. degree in Engineering Physics from Institut Teknologi Bandung, Indonesia, in 2000. He was a research assistant in the Department of Electrical and Electronic Engineering, Universiti Teknologi PETRONAS, Malaysia, from 2009 to 2011. He is currently an assistant professor and a member of the Intelligent and Embedded System Research Group, the Department of Nuclear Engineering and Engineering Physics, Faculty of Engineering, Universitas Gadjah Mada, Yogyakarta, Indonesia. His research interests are instrumentation, control, machine learning, and applications. He can be contacted at email: agusarif@ugm.ac.id.
Nazrul Effendy received the B.Eng. Degree in Instrumentation Technology of Nuclear Engineering and the M.Eng. degree in Electrical Engineering from Universitas Gadjah Mada in 1998 and 2001. He received a Ph.D. degree in Electrical Engineering from Chulalongkorn University, Thailand, in 2009. He was a research fellow at the Department of Control and Computer Engineering, Polytechnic University of Turin, Italy, in 2010 and 2011 and a visiting researcher in Shinoda Lab (Pattern Recognition & Its Applications to Real World), Tokyo Institute of Technology, Japan, in 2009. Currently, he is an Associate Professor and the coordinator of the Intelligent and Embedded System Research Group, the Department of Nuclear Engineering and Engineering Physics, Faculty of Engineering, Universitas Gadjah Mada. He is a member of the Indonesian Association of Pattern recognition, the Indonesian Society for Soft Computing, and the Indonesian Artificial Intelligence Society. He can be contacted at email: nazrul@ugm.ac.id.
BIOGRAPHIES OF AUTHORS
Int J Artif Intell ISSN: 2252 8938 The prediction of the oxygen content of the flue gas in a gas fired boiler system using … (Nazrul Effendy) 929
Eko David Kurniawan is a final year bachelor student in Engineering Physics, Department of Nuclear Engineering and Engineering Physics, Faculty of Engineering Universitas Gadjah Mada, Yogyakarta, Indonesia. He is a research assistant at the Intelligent and Embedded System Research Group, Department of Nuclear Engineering and Engineering Physics, Faculty of Engineering, Universitas Gadjah Mada. His research interests are machine learning and its application in engineering. He can be contacted at email: ekodavid98@mail.ugm.ac.id. Kenny Dwiantoro received the B.Eng. Degree in Engineering Physics from Universitas Gadjah Mada in 2020. He is a research assistant at the Intelligent and Embedded System Research Group, Department of Nuclear Engineering and Engineering Physics, Faculty of Engineering, Universitas Gadjah Mada. He is interested in conducting research in machine learning and its application in engineering. He can be contacted at email: kennydwiantoro14@gmail.com.
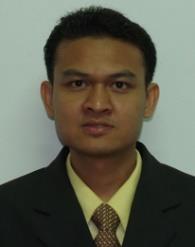



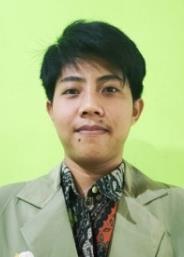



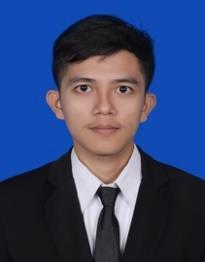



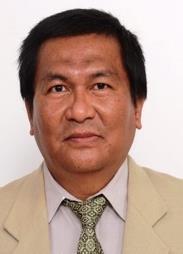



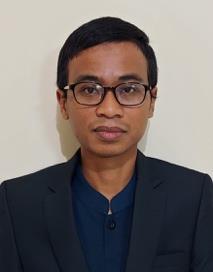



Monitoring forest cover changes is an important task for forest resource management and planning. In this context, remote sensing images have shown a high potential in forest cover changes detection. In Vietnam, although the existence of a large number of such images and ground truth labels, current researches still relied on classical methods employed manual indices, such as multi variant change vector analysis (MVCA) and normalized difference vegetation index. These methods highly require domain knowledge to determine threshold values for forest change that are applicable only for studied areas. Therefore, in this paper, we propose a method to detect coastal forest cover changes, which can exploit available dataset and ground truth labels. Moreover, the proposed method does not require much domain knowledge. We used multi temporal Sentinel 2 imagery to train a segmentation model, that is based on the U Net network. It was used then to detect forest areas at the same location taken at different times. Lastly, we compared obtained results to identify forest disturbances. Experimental results demonstrated that our method provided a high accuracy of 95.4% on the testing set. Furthermore, we compared our model with the MVCA method and found that our model outperforms this popular method by 3.8%.
Coastal forest cover change detection using satellite images and convolutional neural networks in Vietnam Khanh Nguyen Trong1, Hoa Tran Xuan2
Article Info ABSTRACT
Journal homepage: http://ijai.iaescore.com
1Faculty of Information Technology, Posts and Telecommunications Institute of Technology, Ha Noi, Vietnam 2Department of Informatics, Vietnam National University of Forestry, Ha Noi, Vietnam
Article history: Received Oct 16, 2021 Revised Apr 5, 2022 Accepted Apr 25, 2022
IAES International Journal of Artificial Intelligence (IJ AI) Vol. 11, No. 3, September 2022, pp. 930 938 ISSN: 2252 8938, DOI: 10.11591/ijai.v11.i3 pp930 938 930
Keywords: Deep Forestlearningcoverchange detection Forest monitoring system Image USensingsegmentationimagesNet
This is an open access article under the CC BY SA license. Corresponding Author: Khanh Nguyen Trong Faculty of Information Technology, Posts and Telecommunications Institute of Technology Km10 Nguyen Trai, Hanoi, Vietnam Email: khanhnt@ptit.edu.vn
1. INTRODUCTION Coastal forests are an important part of tropical biodiversity. They provide a lot of important services for our ecosystem, such as extreme weather protection, erosion prevention, environments for different species, and storage of blue carbon, which allow to mitigate climate change [1]. However, these forests are increasingly vulnerable to degradation as a result of climate change, sea level rise or anthropogenic processes such as deforestation [2]. To address these issues, accurate and automated forest cover monitoring is crucial [3]. In this context, high resolution remote sensing images collected from satellites, such as the European Sentinel 2A, 2B, or LandSat 8, offer potential and cost efficient sources for an automatic solution [4] Most previous studies focused on traditional methods using hand crafted features, such as multi variant change vector analysis (MVCA), normalized difference vegetation index (NVDI), and so on [1], [2], [5] [9]. They have drawbacks that prevent their wide application, especially for non domain experts in forestry and remote sensing technology. On the one hand, they require more effort and time due to the
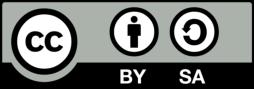
Cover change analysis: lastly, based on forest covers at the two different times, we detected and calculated changes. The model training consists of data preparation, model training, and testing. While the last two steps are composed of model using and GIS analysis. At each of these steps, we applied related techniques in deep learning, satellite image processing, GIS, and so on. The following sections will detail these steps.
The paper is organized as: section 2 introduces our research method. Section 3 presents the experimental results and discussion. Lastly, section 4 concludes the work conducted and proposes some future works.
2. RESEARCH METHOD 2.1. Method overview
Forest cover detection: after training, we applied the model to classify forest and non forest areas of images of the same location taken in different times.
Int J Artif Intell ISSN: 2252 8938 Coastal forest cover change detection using satellite images and … (Khanh Nguyen Trong) 931 excessive dependence on handcrafted features. On the other hand, they are ad hoc solutions that are suitable only for specific regions. Therefore, these methods are time consuming and inefficient.
Recently, with the development of deep learning technology, the field of object detection in remote sensing images has made significant progress. Deep neural networks allow an automatic feature extraction, avoid feature selection and reduce manual steps in monitoring forest cover change [10] [12]. Convolutional neural networks (CNNs) are one of the well known deep learning algorithms that have been widely used in remote sensing image classification. They allow us to extract more meaningful features, the classification of these images usually results in higher performance [13] For example, de Bem et al. [10] presented a method that used CNN and Landsat data for deforestation detection in the Brazilian Amazon. The authors applied three CNN architectures including U Net, ResUnet, and SharpMask to classify the change between the years of 2017 2018 and 2018 2019. The experiment results show that the network achieved a high accuracy, without any post processing for noise cancelling. Stoian et al. [11] also proposed an application of CNN to build land cover maps using high resolution satellite image time series. Based on data from Sentinel 2 L2A, the U Net network was applied in this study to deal with sparse annotation data while maintaining high resolution output. Such networks are even applicable with incomplete satellite imagery in similar problems. For instance, Khan et al. [14] detected forest cover changes over 29 years (1987 2015), in which the authors faced issues of incomplete and noisy data. By using a deep CNN network, they mapped the raw data to more separable features. These features were employed to detect the changes. Many similar applications can be found in the literature, such as the works of [12], [15] [20] and so on. We are interested in monitoring forest cover change using deep learning. Numerous works, which applied deep neural networks, such as CNN, U Net, and satellite images to detect forest loss areas, have been proposed worldwide [10], [11], [14], [21], [22]. However, in Vietnam, traditional machine learning is still widely used. In this paper, we proposed a method for coastal forest cover change detection in Vietnam. Based on sensing images from the European Sentinel 2A and B, we trained a U Net model to detect forest and non forest areas. We then combined geographic information systems (GIS) information to compute the forest cover changes and evaluated results with available information from the national forest monitoring system. The proposed method is capable of applying to different areas, with less effort from domain experts.
The main objective of this study is to automatically detect and calculate coastal forest cover changes of Hai Phong city, Northern Vietnam. We performed pixel level semantic segmentation on Sentinel 2A and 2B images, to classify forest and non forest areas. These images were chosen from the same areas between two periods times. Therefore, combining with GIS information, we can detect and calculate forest cover changes. To do so, we realized three big steps, as presented in Figure 1, including: Figure 1. The proposed method Model training: in this first step, we trained a semantic segmentation model, which was based on U Net neural network. The training dataset came from the Sentinel 2. We also evaluated the trained model using the forest cover layers extracted from the national forest monitoring system (FRMS) of Vietnam.
The model was based on U Net network architecture [24]. We used satellite images for model training. While, the information extracted from FMRS (forest cover layers), combined with GIS, was employed for model evaluation. The following section will detail the data preparation step.
We collected satellite images of Hai Phong city from the Sentinel 2 MSI: Multispectral Instrument, Level 2A [25] dataset available from March 28, 2017. Hai Phong is a port city, which locates in northern Vietnam, between 20030’N ÷ 21001’N and 106023’E ÷ 107008’E. The North borders with Quang Ninh province; Hai Duong province in the West; Thai Binh province in the South; and the East Sea in the east. The city possesses 3 a long mangrove coastal forest, with a total area of 26.127,58 hectare. Since techniques to capture remote sensing and natural optical images are different, there are several challenges while working with satellite images. Therefore, several pre processing steps should be performed before model training, as illustrated in Figure 3. First, we selected suitable scene images from Sentinel 2. For this purpose, remote sensing image processing was performed (the upper process in Figure 3):
ISSN: 2252 8938 Int J Artif Intell, Vol. 11, No. 3, September 2022: 930 938 932
2.3. Data preparation
We adapted a traditional deep learning procedure, as shown in Figure 2 to train our model. Since remote sensing images are more complex and blurrier than others, we should perform several data preparation steps to clean and normalize the input data. Furthermore, to have an objective result, we based on real data, extracted from FRMS, to evaluate the trained model. This system supports state management in monitoring forest cover changes. The data is manually and regularly updated by Vietnamese local forest rangers, through a quantum geographic information system (QGIS) plug in, developed by the development of a management information system for the forestry sector in Viet Nam (FORMIS) phase II project [23]. In short, after the data preparation step, we obtained two types of data: i) forest satellite images that were obtained after a series of data collection and pre processing steps (more detail in the next section) and ii) forest cover layers that were extracted from the FRMS system and manually verified.
Figure 2. Model training process
2.2. Model training process
Figure 3. Data preparation and pre processing
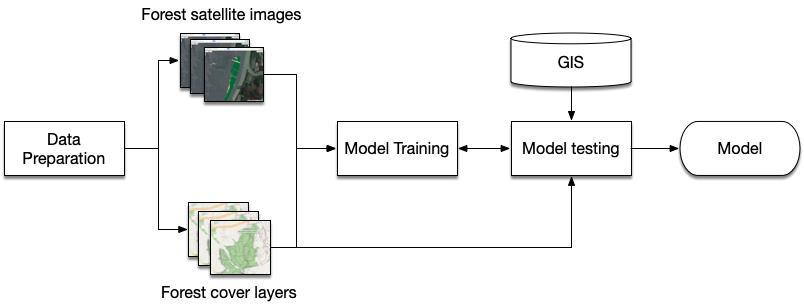
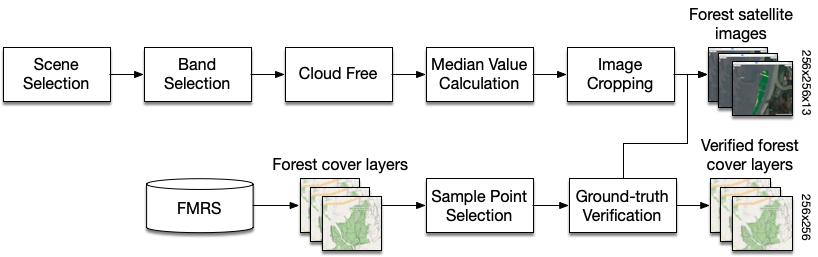
Cloud free: we removed the cloud using the QA60 band, which is a bitmask band with cloud mask information [27]. Since bits 10 and 11 specify clouds and cirrus, we could filter all cloudy pixels.
Int J Artif Intell ISSN: 2252 8938
Figure 4(a) and Figure 4(b) show an example of selected images before and after cloud free.
Figure 4. Satellite images before (a) and after (b) cloud free
Coastal forest cover change detection using satellite images and … (Khanh Nguyen Trong) 933 Scene detection: we selected only image scenes at the coastal and mangrove forest of Hai Phong city. Then, we filtered and kept only images captured in 2018 and 2019. Lastly, the images with cloud rate greater than 30% were eliminated. After this step, we obtained 26 and 32 images captured in 2018 and 2019 Bandrespectively.selection:sentinel 2 have 13 spectral bands, with different bandwidth and spatial resolution. In this study, we directly used ten bands for input features, including the bands from 2 to 8, 8A, 11 and 12 with wavelength of 0.490 µm, 0.560 µm, 0.665 µm, 0.705 µm, 0.740 µm, 0.783 µm, 0.842 µm, 0.865 µm, 1.610 µm, and 2.190 µm. The bands 1, 9, and 10 were ignored because they are not relevant to vegetation [26]. Moreover, we also computed three indices: normalized difference vegetation index (NDVI), normalized difference snow index (NDSI), normalized difference water index (NDWI), which are widely applied in similar problems. They are computed as in (1).
These scene images were then combined with forest cover layers extracted from FRMS to build a labeled pixel level dataset for model training, as shown in the bottom process in Figure 3. We extracted four important pieces of information from FRMS, including administrative information, coordinates, forest observations (0 for non forest, 1 for forest), detailed plot information. Since the forest cover layers were manually entered to FRMS by local rangers, therefore we conducted several field trips to verify the ground truth labels. Based on the available resources, we selected a number of sample points to manually check if the information is correct (forest or non forest). After verification, we got 1,500 sample points with correct labels. Centering on these points, two corresponding neighborhood patches were created, including i) image patches of size 256×256×13 from cropped satellite images and ii) forest cover layer of size 256×256, as presented in Figure 3. Finally, we obtained a dataset of size 256×256×14 for model training.
2.4. U-net neural networks In this study, we applied U Net which is a convolutional network for multi class image segmentation [24]. It supports the per pixel classification that allows us to predict the class of each pixel. We adapted the architecture proposed in [28] with fewer filters since our training set is limited, which also prevents over fitting, as shown in Figure 5. Since the input size is 256×256×14, thus we have adapted the network architecture accordingly. Sigmoid activation functions were used to ensure that output pixel values range between 0 and 1.
�������� = ������ ������ ������+������;�������� = ���������� �������� ����������+�������� ;�������� = ������ �������� ������+�������� (1) where near infrared reflectance (NIR) is band 8, Red is band 4, Green is band 3, and short wave infrared (SWIR) is band 11.
Median value calculation: to improve the quality of images, we applied a median filter that moves through the image pixel by pixel, and replaced each value with the median value of neighboring ones. Image cropping: at this step, we cropped images to focus only on studied areas. (a) (b)
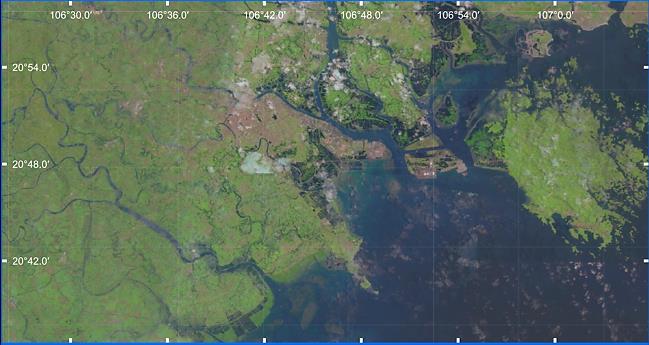
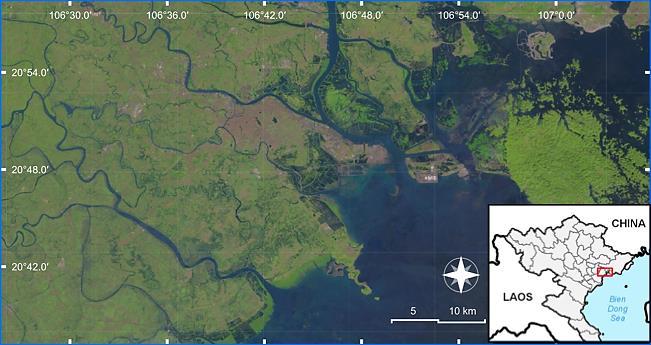
ISSN: 2252 8938 Int J Artif Intell, Vol. 11, No. 3, September 2022: 930 938 934
2.6.
Forest cover change analysis
We split the collected data into three datasets: the training set containing 1,000 image patches, the validation set containing 300 patches, and the testing set containing 200 patches. The model was trained using binary cross entropy as loss function, Adam optimizer (e=10−7, β1=0.9, and β2=0.999), a mini batch with a size of 100, and early stopping criteria on the validation set. Before creating the batches, we also shuffled data, which helps our model to lean it better with more objective results. To evaluate the experiments, the F1 score, precision, recall, and accuracy were applied. The TensorFlow framework 2.2.0, Keras 2.3.1, Python 3.6, Tesla K80 GPU, and Intel Xeon (R) were used to implement our model. Figure 5. U Net architecture [28]
2.5. Training and validation setup
After training and validating, we determined forest cover changes, as illustrated in Figure 6. The trained model detected forest and non forest areas of images captured at different times on the same location. Obtained results were then compared to identify the cover changes. Combining with GIS information extracted from FRMS, we can calculate area changes. Figure 6. Forest cover change analysis
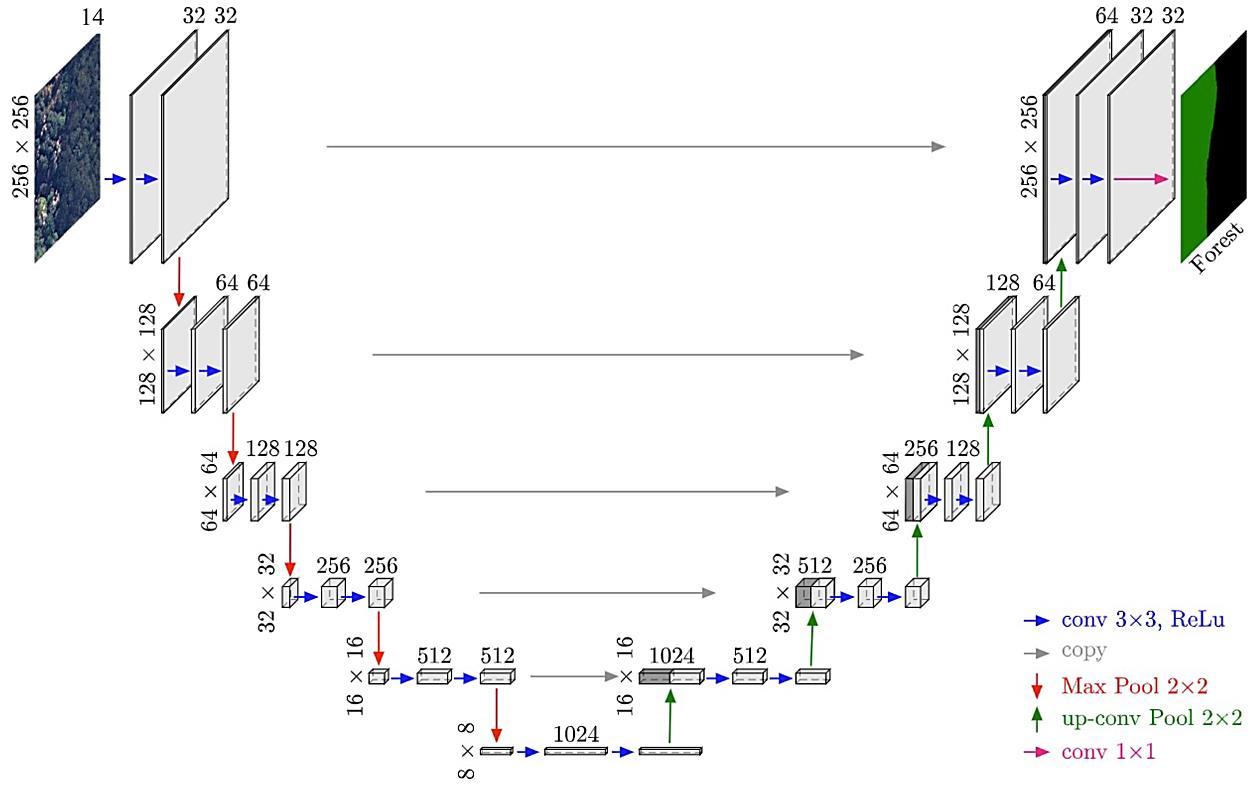
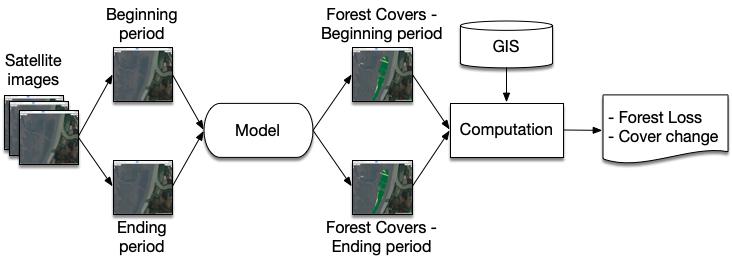
��ℎ������������������1
��ℎ������������������2
With early stopping, the training stopped at the 14th epoch. Figure 7(a) and Figure 7(b) show the model training progress over time in terms of accuracy and loss. The training and validation accuracy increase while training and valuation loss decrease as the number of training iterations increases. The gap between the curves is also small which indicates that no overfitting occurs. The model achieved a high accuracy of 97.7% on the validation set and 96.4% on the testing set. This high performance can be explained by the fact that the spectral and textural features of forest cover on RGB images are differentiable by the human eye, as presented in Figure 8. Due to the imbalance of labeled pixels, the precision, recall, and F1 score are 87.5%, 89.3%, and 87.2%, which are lower than the accuracy.
(a) (b) Figure 7. Progress of accuracy (a) and loss (b) on the training and validation set
��ℎ������������������1>48 and
To detect forest cover changes, we applied the trained model to images of the same location taken in 2018 and 2019. The obtained results were then used to detect and calculate forest cover changes, as shown in Figure 8. The model accurately detected forest areas at the beginning and ending period (2018 Figure 8(a), and 2019 Figure 8(b)). Then, we mapped the two results and performed several GIS operations to get the forest cover changes, as detailed in Figure 8(c). According to the policy of the Vietnam government, an increase or decrease of forest covers, which is greater than 0.3 ha, will be considered to be a change. Therefore, we calculated and detected five forest loss areas, as presented in Figure 8(d) (red parts). The results were similar to those reported by local rangers in 2019. Therefore, our model is capable of accurately detecting forest cover changes. Compared with existing methods that are widely applied in Vietnam, our proposed method is more robust and more accurate in forest cover detection. Experimental results show that our method outperforms MVCA by 3.8% (91.6% on the testing set). It allows detecting a higher level of forest disturbances, as shown in Figure 9. Figure 9(a) and Figure 9(b) shows forest cover changes (the white part and yellow part), predicted by MVCA and our proposed method, respectively. Our method produced results that are closer to the real data reported by local rangers.
Int J Artif Intell ISSN: 2252 8938 Coastal forest cover change detection using satellite images and … (Khanh Nguyen Trong) 935 2.7. MVCA method
3. RESULTS AND DISCUSSION
For performance evaluation, we compared the proposed method with MVCA that is widely used in Vietnam [7], [29]. This method is based on NDVI and NDSI of the beginning (����������, ����������) and ending (����������, ����������) period to calculate two change vectors, as shown in (2) and (3). Then, with the help of expert knowledge, the method uses two thresholds to determine forest loss. In this study, there is forest loss if ��ℎ������������������2>168 = √(���������� ����������)2 +(���������� ����������)2 (2) = (���������� ����������)+(���������� ����������) (3)
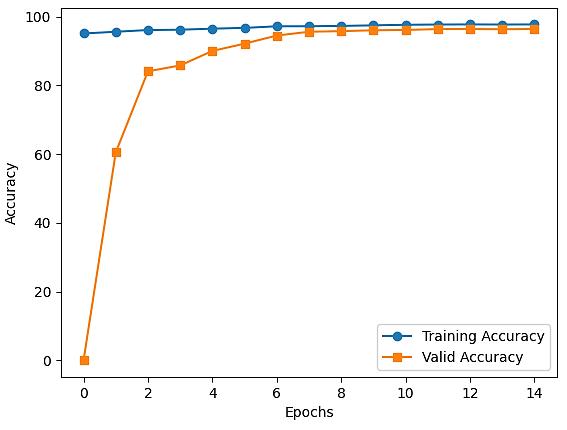
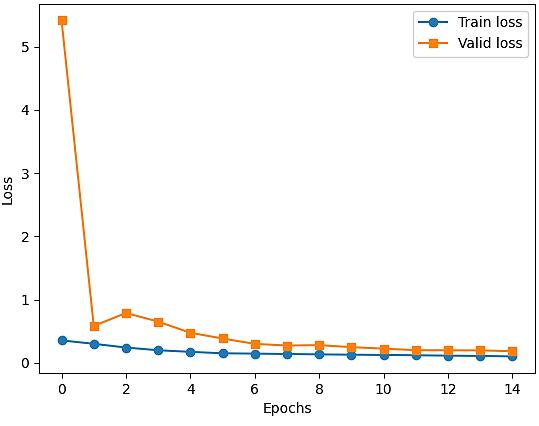
ISSN: 2252 8938 Int J Artif Intell, Vol. 11, No. 3, September 2022: 930 938 936
Moreover, the proposed method requires less expert knowledge than methods based on NVDI, NVSI as in [1], [2], [7], [9], [29]. For these methods, domain experts are highly required to determine threshold values that are applicable only for a specific area. In contrast, our method does not require these thresholds. The model automatically learns useful features from input data to detect forest cover. Furthermore, as a deep learning model, our model can be incrementally trained with the new target areas. It means that the model can be gradually provided with new samples to update its weights and thus improve its classifications with time.
Figure 9. Forest and forest plot cover change prediction by (a) MVCA (green: forest covers; white: forest cover change) and (b) U Net (green: forest covers; yellow: forest cover change)
Figure 8. Comparing forest cover (dark green part) in (a) 2018 and (b) 2019 to compute (c) all forest changes (yellow part) and (d) the ones greater than 0.3 ha (red part)
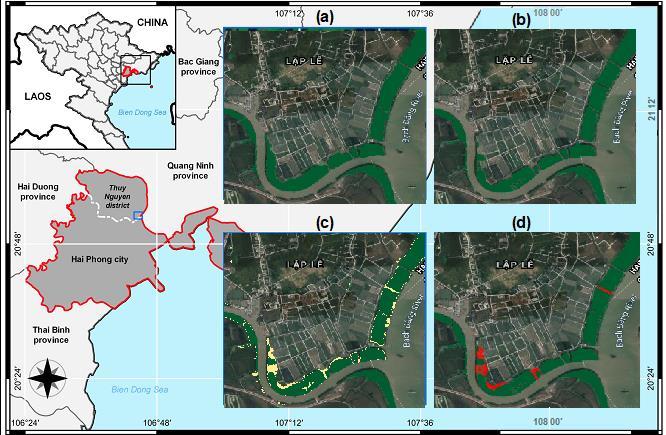
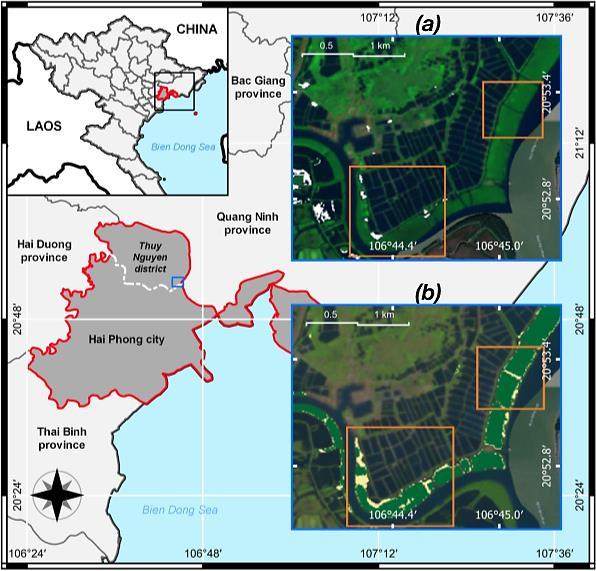
[19] Z. Mohammed, C. Hanae, and S. Larbi, “Comparative study on machine learning algorithms for early fire forest detection system using geodata,” International Journal of Electrical and Computer Engineering (IJECE), vol. 10, no. 5, pp. 5507 5513, Oct. 2020, doi: 10.11591/ijece.v10i5.pp5507 5513.
[3] B. K. Kogo, L. Kumar, and R. Koech, “Forest cover dynamics and underlying driving forces affecting ecosystem services in western Kenya,” Remote Sensing Applications: Society and Environment, vol. 14, pp. 75 83, Apr. 2019, doi: 10.1016/j.rsase.2019.02.007.
[20] A. AlDabbas and Z. Gal, “Cassini Huygens mission images classification framework by deep learning advanced approach,” International Journal of Electrical and Computer Engineering (IJECE), vol. 11, no. 3, pp. 2457 2466, Jun. 2021, doi: 10.11591/ijece.v11i3.pp2457 2466.
[8] D. Tien Bui, K. T. T. Le, V. C. Nguyen, H. D. Le, and I. Revhaug, “Tropical forest fire susceptibility mapping at the cat ba national park area, hai phong city, vietnam, using gis based kernel logistic regression,” Remote Sensing, vol. 8, no. 4, 2016. doi: 10.3390/rs8040347. [9] N. Van Thi, T. Q. Bao, L. S. Doanh, P. Van Duan, N. N. Hai, and T. X. Hoa, “Study on deforestation detection in Gia Lai province using sentinel 2 optical satellite image and sentinel 1 radar data,” Journal of Vietnam Agricultural Science and Technology, vol. 5, pp. 105 112, 2020 [10] P. P. de Bem, O. A. de Carvalho, R. F. Guimarães, and R. A. T. Gomes, “Change detection of deforestation in the brazilian amazon using landsat data and convolutional neural networks,” Remote Sensing, vol. 12, no. 6, Mar. 2020, doi: 10.3390/rs12060901.
[5] E. Gyamfi Ampadu, M. Gebreslasie, and A. Mendoza Ponce, “Multi decadal spatial and temporal forest cover change analysis of Nkandla Natural Reserve, South Africa,” Journal of Sustainable Forestry, pp. 1 24, Feb. 2021, doi: 10.1080/10549811.2021.1891441.
4. CONCLUSION
[13] C. Shi, T. Wang, and L. Wang, “Branch feature fusion convolution network for remote sensing scene classification,” IEEE Journal of Selected Topics in Applied Earth Observations and Remote Sensing, vol. 13, pp. 5194 5210, 2020, doi: 10.1109/JSTARS.2020.3018307.
[18] R. Senthilkumar, V. Srinidhi, S. Neelavathi, and S. Renuga Devi, “Forest change detection using an optimized convolution neural network,” IETE Technical Review, pp. 1 8, Oct. 2020, doi: 10.1080/02564602.2020.1827987.
[17] L. Bragagnolo, R. V. da Silva, and J. M. V. Grzybowski, “Amazon forest cover change mapping based on semantic segmentation by u nets,” Ecological Informatics, vol. 62, May 2021, doi: 10.1016/j.ecoinf.2021.101279.
Int J Artif Intell ISSN: 2252 8938 Coastal forest cover change detection using satellite images and … (Khanh Nguyen Trong) 937
[11] A. Stoian, V. Poulain, J. Inglada, V. Poughon, and D. Derksen, “Land cover maps production with high resolution satellite image time series and convolutional neural networks: adaptations and limits for operational systems,” Remote Sensing, vol. 11, no. 17, Aug. 2019, doi: 10.3390/rs11171986.
In this study, a deep learning based method for coastal forest cover change detection has been pro posed. We used multi temporal Sentinel 2 imagery to train a segmentation model based on U Net neural net work. Furthermore, we evaluated the model with forest cover information extracted from the national forest resource monitoring system of Vietnam. The results shown that our method achieved a good performance on remote sensing images. The trained model achieved a high accuracy of 95.4% on the testing set and outperformed the popular methods based on thresholds in Vietnam. Future works will focus on tree species classification by improving the network architecture, increasing our dataset and proposing augmentation methods for forest cover images.
REFERENCES
[12] T. Chang, B. Rasmussen, B. Dickson, and L. Zachmann, “Chimera: a multi task recurrent convolutional neural network for forest classification and structural estimation,” Remote Sensing, vol. 11, no. 7, Mar. 2019, doi: 10.3390/rs11070768.
[7] T. H. Nguyen, V. D. Pham, S. D. Le, and V. D. Nguyen, “Determining the locations of deforestation using multi variant change vector analysis (MCVA) on Landsat 8 satellite data,” Journal of Forestry Science and Technology, vol. 4, no. 96 105, 2017
[6] T. V. Ramachandra, B. Setturu, and S. Vinay, “Assessment of forest transitions and regions of conservation importance in Udupi district, Karnataka,” Indian Forester, vol. 147, no. 9, Oct. 2021, doi: 10.36808/if/2021/v147i9/164166.
Despite their advantages, the proposed method is not as easy to implement as MVCA and similar methods based on thresholds. It requires, on the one hand, a relatively large quantity of samples, and on the other hand, ground truth masks that can be challenging and time consuming. Whereas the MCVA and similar methods work with simpler sampling schemes and can produce reasonably acceptable results. However, in Vietnam thanks to FRMS, we already have ground truth labels that are regularly entered by local rangers. Therefore, the proposed method is able to be widely applied for automatically monitoring forest covers.
[4] M. Jaskulak and A. Grobelak, “Forest degradation prevention,” in Handbook of Ecological and Ecosystem Engineering, Wiley, 2021, pp. 377 390., doi: 10.1002/9781119678595.ch20.
[16] K. Hufkens et al., “Historical aerial surveys map long term changes of forest cover and structure in the Central Congo Basin,” Remote Sensing, vol. 12, no. 4, Feb. 2020, doi: 10.3390/rs12040638.
[2] H. H. Nguyen et al., “Monitoring changes in coastal mangrove extents using multi temporal satellite data in selected communes, Hai Phong City, Vietnam,” Forest and Society, vol. 4, no. 1, Apr. 2020, doi: 10.24259/fs.v4i1.8486.
[15] M. Pritt and G. Chern, “Satellite image classification with deep learning,” in 2017 IEEE Applied Imagery Pattern Recognition Workshop (AIPR), Oct. 2017, pp. 1 7., doi: 10.1109/AIPR.2017.8457969.
[14] S. H. Khan, X. He, F. Porikli, and M. Bennamoun, “Forest change detection in incomplete satellite images with deep neural networks,” IEEE Transactions on Geoscience and Remote Sensing, vol. 55, no. 9, pp. 5407 5423, Sep. 2017, doi: 10.1109/TGRS.2017.2707528.
[1] H. T. T. Nguyen et al., “Mangrove forest landcover changes in coastal vietnam: a case study from 1973 to 2020 in Thanh Hoa and Nghe An provinces,” Forests, vol. 12, no. 5, May 2021, doi: 10.3390/f12050637.
BIOGRAPHIES OF AUTHORS Dr. Khanh Nguyen Trong holds a Doctor of Computer Science from University of Paris VI, France, in 2013. He received his M.Sc. (System and Networking) from University of Lyon I in 2008, his B.S (Information Technology) at Hanoi University of Science and Technology in 2005. He is now a lecturer at Faculty of Information Technology, Posts and Telecommunications Institute of Technology, Hanoi, Vietnam. He is a member of Naver AI lab (PTIT and Korean Naver cooperation) since 2020. His research includes Machine Learning, Deep Learning, Distributed Systems, Agent based Modelling and Simulation, Collaborative and Participatory Simulation and Modelling, and Computer Support Collaborative Work. He can be contacted at email: khanhnt@ptit.edu.vn Hoa Tran Xuan received his B.S in Information System at Vietnam Forestry University in 2012, and MSc in Information System at Posts and Telecommunications Institute of Technology, Hanoi, Vietnam in 2020. His main research interests are the application of information technology in forestry, Geographic Information Systems, Forest Cover Change Detection, and Forest Species Classification. Currently, Hoa Tran Xuan is a lecturer of Computer Science at Vietnam National University of Forestry. He can be contacted at email: hoatx@vnuf.edu.vn.
ISSN: 2252 8938 Int J Artif Intell, Vol. 11, No. 3, September 2022: 930 938 938 [21] D. Peng, Y. Zhang, and H. Guan, “End to end change detection for high resolution satellite images using improved UNet++,” Remote Sensing, vol. 11, no. 11, Jun. 2019, doi: 10.3390/rs11111382.
[29] N. T. Hoan, H. T. Quynh, L. M. Hang, N. M. Ha, H. T. H. Ngoc, and D. X. Phong, “Estimation of of land use changes in tan rai bauxite mine by multi variants change vector analysis (MCVA) on multi temporal remote sensing data,” Journal of Geoscience and Environment Protection, vol. 08, no. 03, pp. 70 84, 2020, doi: 10.4236/gep.2020.83006.
https://www.niras.com/projects/formis ii/ (accessed Mar. 14, 2022) [24] O. Ronneberger, P. Fischer, and T. Brox, “U Net: vonvolutional networks for biomedical image segmentation,” in Lecture Notes in Computer Science (including subseries Lecture Notes in Artificial Intelligence and Lecture Notes in Bioinformatics), vol. 9351, Springer International Publishing, 2015, pp. 234 241., doi: 10.1007/978 3 319 24574 4_28. [25] “Sentinel 2 msi: multispectral instrument, level 2a,” Google https://developers.google.com/earth engine/ datasets/catalog/COPERNICUS_S2_SR (accessed Mar. 14, 2022) [26] X. Zhou et al., “Discriminating urban forest types from sentinel 2A image data through linear spectral mixture analysis: a case study of Xuzhou, East China,” Forests, vol. 10, no. 6, May 2019, doi: 10.3390/f10060478. [27] K. Soudani et al., “Potential of C band synthetic aperture radar sentinel 1 time series for the monitoring of phenological cycles in a deciduous forest,” International Journal of Applied Earth Observation and Geoinformation, vol. 104, Dec. 2021, doi: 10.1016/j.jag.2021.102505. [28] F. H. Wagner et al., “Using the U‐net convolutional network to map forest types and disturbance in the Atlantic rainforest with very high resolution images,” Remote Sensing in Ecology and Conservation, vol. 5, no. 4, pp. 360 375, Dec. 2019, doi: 10.1002/rse2.111.
[23] “Forest management information system in Viet Nam ensures sustainability by providing up to date information,” Niras, 2018.
[22] J. V. Solórzano, J. F. Mas, Y. Gao, and J. A. Gallardo Cruz, “Land use land cover classification with u net: advantages of combining sentinel 1 and sentinel 2 imagery,” Remote Sensing, vol. 13, no. 18, Sep. 2021, doi: 10.3390/rs13183600.
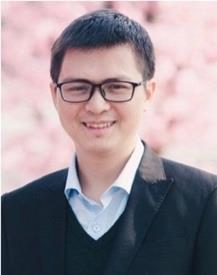



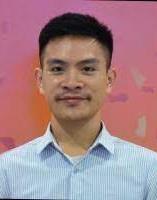



1. INTRODUCTION
Corresponding Author: Refed Adnan Jaleel
Improving prediction of plant disease using k-efficient clustering and classification algorithms
Journal homepage: http://ijai.iaescore.com
Article history: Received Sep 22, 2021 Revised Apr 7, 2022 Accepted May 6, 2022 Because plant disease is main cause of most plants’ damage, improving prediction plans for early detection of plant where it has disease or not is an essential interest of decision makers in the agricultural sector for providing proper plant care at appropriate time. Clustering and classification algorithms have proven effective in early detection of plant disease. Making clusters of plants with similar features is an excellent strategy for analyzing features and providing an overview of care quality provided to similar plants. Thus, in this article, we present an artificial intelligence (AI) model based on k nearest neighbors (k NN) classifier and k efficient clustering that integrates k means with k medoids to take advantage of both k means and k medoids to improve plant disease prediction strategies. Objectives of this article are to determine performance of k mean, k medoids and k efficient also we compare k NN before clustering and with clustering in prediction of soybean disease for selecting best one for plant disease forecasting. These objectives enable us to analysis data of plant that help to understand nature of plant. Results indicate that k NN with k efficient is more efficient than other in terms of inter class, intra class, normal mutual information (NMI), accuracy, precision, recall, F measure, and running time.
Asraa Safaa Ahmed1, Zainab Kadhm Obeas2, Batool Abd Alhade2, Refed Adnan Jaleel3 1Department of Computer Sciences, College of Science, Diyala University, Diyala, Iraq 2College of Science, Al Qasim Green University, Babylon, Iraq 3
IAES International Journal of Artificial Intelligence (IJ AI) Vol. 11, No. 3, September 2022, pp. 939 948 ISSN: 2252 8938, DOI: 10.11591/ijai.v11.i3 pp939 948 939
Department of Information and Communication Engineering, Information Engineering College Al Nahrain University, Baghdad, Iraq Email: Iraq_it_2010@yahoo.com
Article Info ABSTRACT
Keywords: K means K medoids K nearest neighbors Plant SoybeanPredictiondisease
Detection of Plant disease is an important aspect of precision agriculture since it focuses on detecting diseases in their early stages [1]. Agriculture is the sort of productivity that the world needs to develop. Despite the fact that there are several projects to expand each sector, there has been little progress in the agriculture farming sector. The application for the detection of plants is therefore to enhance the agricultural sector and to increase safety criteria so that the development of the plant growth can be developedPlant[2] monitoring is one of the greatest frameworks that agriculture sector institutions should build because of the spread of plant disease [3]. Also, early detection of plant disease is the greatest approach to enhance prediction, and this is where machine learning (ML) algorithms may help in the current work. The integration of clustering with classification facilitates the use of very smart frameworks which take account of the operational performance and efficiency of all ML affiliated institutions [4], [5] and detect knowledge patterns that correlated to the nature of plant diseases. ML can address critical concerns connected to plant
This is an open access article under the CC BY SA license.
Department of Information and Communications Engineering, Information Engineering College, Al Nahrain University, Baghdad, Iraq
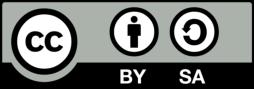
In fact, thanks to clustering and classification algorithms, creating clusters of plants with similar characteristics allows for a better understanding of the quality of care given to various plants and by using classifiers it is possible to build a forecasting model of a plant disease by examining the historical data. This article was conducted to understand better the most novel and practical applications of integrating clustering and classification algorithms of ML in the soybean plant disease prediction. Soybeans are an useful crop since it’s processed for their oil and meal [7]. People eat meat and oil from the meal, which is then given to animals that are commonly eaten by humans. Different diseases damage soybean crops [8]
ISSN: 2252 8938 Int J Artif Intell, Vol. 11, No. 3, September 2022: 939 948 940 disease if information about the plants is obtained. It’s utilized to derive diagnostic rules and give decision makers a precise prediction method [6].
Previous articles have found that k means, SVM and k NN are widely applied because of their easy to train, ease of interpretation, and thus have been applied in several articles of classification plant disease. Also have found that k NN are typically the most accurate than SVM. Depend on the literature; it has been discovered that to date, most articles have applied data of images for plants. But although the literature presents several classification algorithms with k means clustering for controlling plant disease, none of these articles adopt a complete, integrated, and customized framework that uses an effective clustering algorithm or k medoids algorithm in plant disease to analyze and forecast plant disease, despite that, clustering algorithms are essential to analyze plant disease data.
The rest of this articles is organized as: section 2 discusses the research methodology. Section 3 presents results and analysis. Section 4 discusses the results of this article. Section 5 presents a conclusion as well as recommendations for future research.
Recently, several researchers have studied the application of the integration of clustering and classification algorithms into plant disease prediction as a response to the expansion of the plant disease pandemic. The research question is being followed. Research question (RQ): How does combining clustering and classification help predict and control a pandemic of plant diseases? Based on this research question, we collect articles from different good scientific databases. The key search terms were ML, clustering, classification, data mining, k medoids, k means, plant disease, and soybean. Boolean operations such as OR and AND used literary search in the many databases to provide important results for the research articles concerned.Kaushal and Bala [9] proposed using k mean for the segmentation of input images and support vector machine (SVM) classifier was applied for classifying the input image into two classes and they enhanced performance of SVM classifier by replacing it with k nearest neighbors (k NN) classification. Prakash et al [10] used k means for segmenting a leaf image to find infected areas and SVM was used for classification purpose. Bhuvana et al. [11] used k means and multi class SVM for the detection of leaf diseases depend on images dataset and the accuracy obtained equal to 98%. Adit et al. [12] applied convolutional nural network (CNN) to analyze palnt disese and produced reliable platform. Khan et al. [13] examined how ML approaches have been evolved to deep learning for detection of plant disease.
This article aims to improve prediction of a plant disease with a k efficient clustering and classification model based on data set of soybean that used in [18] to achieve accurate and meaningful results to support agriculture decision makers. This model enables a better understanding of the nature of the soybean disease. Figure 1 offers block diagram for our proposed model. After we load the data the preprocess
This article observes that able to use clustering algorithm in predicting plant disease depend on raw dataset. For observing that we take the same data in the article, which presents by Morgan et al. [18] applied classification algorithms on soybean data and observe that k NN performed best from other classification algorithm in predicting soybean disease where indicated accuracy of k NN equal to 91.83%. While by implementing our proposed model k NN achieved 100% accuracy. Therefore, through an in depth research on classification algorithms and available comparison methods, we have shown that the best way to select an algorithm is to try them all on the same data set.
Geetha et al. [14] used four stages for detect the type of plant disease contained pre processing, k means (segmentation of leaf), extraction of feature and classification using k NN. Sankaran et al. [15] used k means, principal component analysis (PCA) and SVM proposed for detect leaf disease. They describe that accuracy (ACC) of k means in predicting best from SVM and SVM best from PCA. Mariyappan [16] used k means and SVM for predicting of disease based on data that including healthy and diseased leaf image.
2. RESERCH METHOD
Yousuf and Khan [17] suggested technique workd in segmentation by k means, feature selection by random forest (RF), and classification by k NN for plant disease. The proposed method outperformed SVM, according to the results of the experiments.
Soybean dataset include duplicates, outliers, errors (noisy) and some values are missed (incomplete). Data preprocessing is responsible for dealing with these problems. Data preprocessing is a technique of ML and a data mining that converts raw data into a format that ML algorithms can understand [21]. Because of bad data, no meaningful findings are obtained by the ML models or even incorrect replies that can lead to incorrect conclusions.
Int J Artif Intell ISSN: 2252 8938
Before beginning data preprocessing, it’s a good idea to figure out what data the ML algorithm need in order to achieve good results. In this article we use the clustering algorithms. Thus, we remove irrelevant observations, duplicates, unnecessary columns, and errors. Also we handle outliers, inconsistent data, and noise [22]. The soybean dataset’s attribute values were also numerically coded. A decision was made to run k NN classification and clustering algorithms on cleaning and balanced soybean dataset.
step done, after that the clustering algorithms implemented in the cleaning and balance data, then the k NN implemented on each disease cluster of soybean data, and finally we evaluating the proposed framework. We use clustering algorithms before classification algorithms of ML. Since we know, the problem in particular of soybean dataset that containing 35 attributes and 307 instances, the data are multi type and large. These characteristics present enormous challenges for ML in predicting plant disease. So, we found that integrating of clustering and classification it very suitable in raw data of plant disease. Thus, in this article, we are improving the prediction of plant disease by using an efficient clustering (integrating between k means and k medoids) that addresses the key requirements of interoperability, scalability, context discovery, and reliability.
Figure 1. Block diagram of proposed AI model 2.1. Description and preprocessing of soybean data
The soybean dataset, which was downloaded from the UCI machine learning repository, has 307 observations from soybean plants affected with 18 disease and one class for healthy and 35 categorical attributes [19], [20]. These data serve the ML community to investigate their algorithms on a case by case basis. According to Google Scholar, the archive has been cited at least 52 times [20]. Soybean data are selected on the basic of their respective large size and its raw data in order to test the clustering algorithms impact on effectiveness, efficiency and scalability of classification algorithms for predicting soybean disease and to show that it is enable for using raw data instead of images for predicting disease of plants.
Improving prediction of plant disease using k efficient clustering and … (Asraa Safaa Ahmed) 941
2.2. K Means and k medoids clustering algorithms
The procedure of k medoids is same to that of k means. But it different from k means in the step of updatd and replacd medoid by comparing the sum of distances between a point and other points and the sum
The procedure of k means (unsupervised ML) starts by randomly selecting k (cluster’s number) and data points (cluster’s center). Then each point is placed in the cluster with the closest center to it. The closest between points and centers calculated based on distance of Euclidean. The centers of the clusters are updated and replaced by the means of the points of the cluster. It is iterated until the clusters have reached stability [23]. It is easy to use, however the selection of the integer k can vary the results [24]. The approach is prone to outliers and noise because the average of the points within the cluster induces early convergence. It also restricts non numerical data types such as ordinal data and categorical [25]
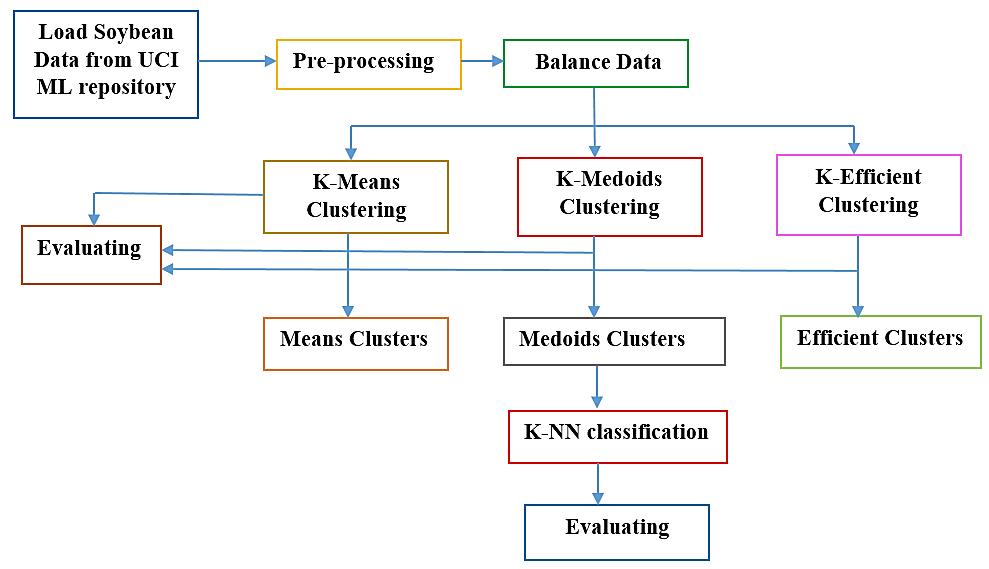
ISSN: 2252 8938 Int J Artif Intell, Vol. 11, No. 3, September 2022: 939 948 942ofdistances between points and medoid. As a result, k medoids outperform k means in terms of noise and outliers. Because the use of medoids to represent the centers inhibits early convergence, the findings are relatively successful [26], [27] We offer k efficient clustering technique for soybean disease prediction that depends on the merging of k means and k medoids. The flowchart in Figure 2 summarizes the algorithm of k efficient clustering. In theory, the complication is the same as k medoids. It serves as a bridge between k means and k medoids.
2.2.1. K nearest neighbours classification algorithm k NN (supervised ML) algorithm is a recognition of pattern that is used in various areas. It is known for its good interpretation, simple procedure, and its low calculation time [28]. The procedure of k NN depend on the hypothesis that points with same inputs have same outputs. In many data grouping or classification jobs, the distance or similarity between two items plays an important role. For numerical variables in datasets, traditional distances such as Euclidean can be used. The Figure 3 displays a k NN algorithm flowchart [29]. ACC is more important than any other factor in detecting soybean disease. For the following reasons, the k NN algorithm may compete with the most accurate models in very accurate predictions [30]: When making real time predictions, k NN saves the training dataset and solely learns from it. As a result, the k NN training method is significantly more rapid than the alternatives, such as linear regression, SVM, and so on. As no prediction training is necessary for the k NN, new data may be smoothly inserted that will not influence the algorithm ACC. k NN is a simple algorithm to implement. Only the value of K and the distance function are necessary to implement k NN [25]
Figure 2. Flowchart of integrating k means and k medoids
Since the third instruction executes less iterations, intuitively it is close to the k means. k means produce good ones compared to random start centers. As a result, k efficient clustering can handle large data sets. It is good at least as k medoids because k medoids are called at the end of k efficient clustering. Thus, we can also conclude that it is better than K means. Because of the simultaneous call of the k medoids and k means, there is a drawback of random number k initialization, which is less biased.
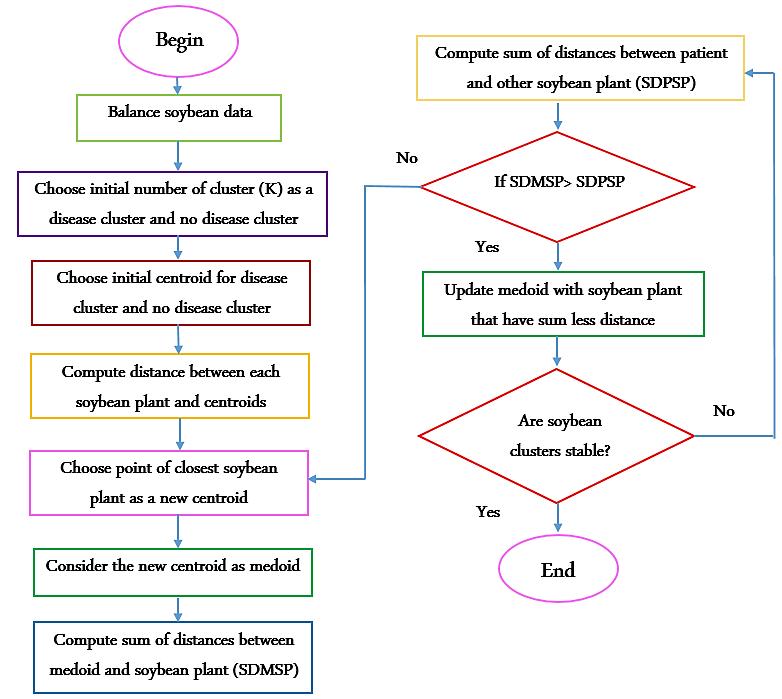
The number of correctly classified instances (i.e. TP and FP) splitting by the total number of examples yields ACC. The fraction of relevant instances to the retrieved instances is known as precision. Divide the number of TP classes by the sum of the number of TP classifications and the number of FN classifications to get the recall. Finally, the F measure is computed by multiplying the recall and precision, dividing this value by the sum of the recall and precision, and finally multiplying this number by two. To demonstrate the effectiveness of clustering algorithms, we used the normal mutual information (NMI) for measuring the degree of closeness of the one that should be in reality to the result of the classification. When the NMI is near to 1, the results are significant. In addition, we employed the inertia to test the algorithm’s effectiveness. Two types of inertia were examined [33], [34]: The interia of intra class, it is a value to
2.2.2. Evaluating of clustering and classification algorithms k NN is a supervised learning algorithm that is used to solve regression and classification problems while clustering algorithms are unsupervised learning algorithms that are used to solve clustering problems. This is the basic difference between clustering and classification. Thus the evaluating of k NN is different from evaluating of k means, k medoids, and k efficent clustering [31]. The metrics of evaluation assess the model of classification depend on the ability to accurately forecast the target classes of the unlabeled instances. Forecasting the target classes of the unlabeled instances in a two class issue can be categorized into 4 kinds namely false negative (FN), true positive (TP), false positive (FP), and true negative (TN) as described in Table 1 [32]. The ACC, F measure, recall, and precision are used for evaluating classification model.
Int J Artif Intell ISSN: 2252 8938 Improving prediction of plant disease using k efficient clustering and … (Asraa Safaa Ahmed) 943 Figure 3. Flowchart of k NN
Table 1. Soybean confusion matrix No Soybean Disease Soybean Disease No Soybean Disease TN FP Specificity=TN/(TN+FP) Soybean Disease FN TP Recall=TP/(TP+FN) Negative Value=TN/(TN+FN)Predictive Precision=TP/(TP+FP) Accuracy=(TP+TN)/(TP+TN+FP+FN)
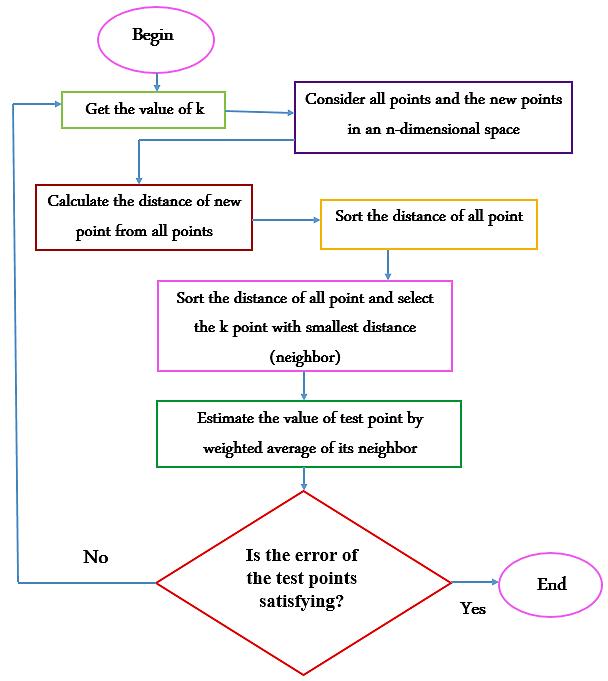
ISSN: 2252 8938 Int J Artif Intell, Vol. 11, No. 3, September 2022: 939 948 944minimize because it represents the distance between a cluster elements, centroid, and the inertia of interclass, which calculates the distance between the clusters and centers. A high value expresses an interesting result, hence it is a value to maximize. The equations of calculated these metrics have been shown in [34] 3.
RESULTS AND ANALYSIS
The classification and clustering algorithms were tested on cleaning soybean dataset in seven experiment, first experiment implementing k NN without any clustering algorithm, second experiment implement k means clustering algorithm, third experiment implement k medoids clustering algorithm, fourth experiment implement k efficient clustering, fifth experiment k NN with K means, sixth experiment k NN with k medoids, and finally k NN with k efficient clustering. The measures previously determined were reported for algorithm of classification and for clustering algorithms have been used for evaluating the proposed model. Before implementing classification methods to a dataset, a classifier’s ACC can be increased by using clustering techniques. All the experiments were running in Java and on a machine with 2.60 GHz CPU, Core (TM) i5 3230M of 4.00 GB and a RAM processor Intel (R) under Windows 10 64 bit. We put the algorithms to the test with soybean data from the UCI machine learning repository. 3.1.
Clustering algorithms
The results of inertia and NMI experiments for the three clustering algorithms shown in Figure 4 and Table 2. K medoid intra classes are lower than k means intra class, and because the distance between positive (each time, one disease type is assigned to the positive class, while the others are assigned to the negative class) and negative clusters in k means clustering is considerable while the distance between plants and centroid is minimal, the inter class is larger than k means. The k efficient clustering has the shortest distance between positive and negative clusters (intra class inertia) and the biggest inter class inertia. In other words, k efficient clustering is the best strategy for distributing soybean data across disease clusters when compared to other methods. Then we conclude that the inertia of other techniques is exceeded by k efficient clustering. We refer that k efficient clustering performs better than k medoids and k means in NMI metric. Also, we conclude that k efficient clustering is practically as well as k medoids even if k efficient clustering results in better inertia. Finally, we note that k efficient clustering takes longer to execute than k means but less time than k medoids for forecasting soybean disease. Because it is faster than k medoids, it fits our scenario. K efficient clustering is effective in obtaining the best of both techniques for the benchmark: k medoids precision and k means speed.Figure 4. Results of clustering evaluating
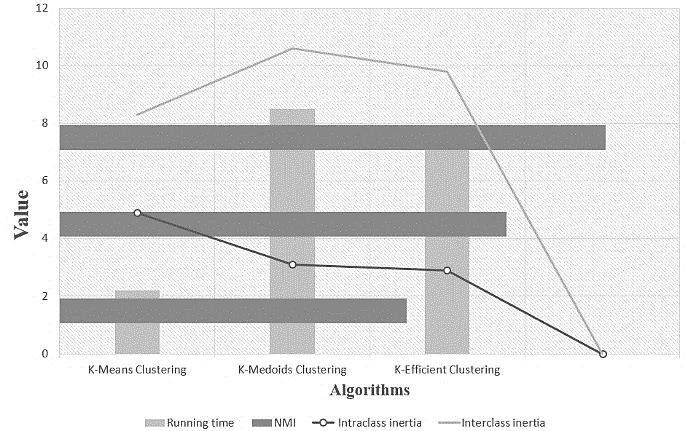
3.2. Integrating clustering and classification
In comparing the results that shown in Figure 5 and Table 3 of four experiments, k NN performed best on the soybean dataset with k means from without using clustering, k NN performed best on the data of soybean with k medoids from k NN with k means while k NN performed at 100% accuracy with k efficient clustering. It is to be expected that most of the classification algorithms would perform best with k efficient clustering because it is integrating the benefits from k means and k medoids, despite the data of soybean that was tested has 19 disease classes. When using a k NN classifier with clustering, the ACC and true positive rate are greater than when using a k NN classifier alone to predict soybean disease. We would like the precision number to be 100 and the false positive rate to be zero in an ideal scenario. Integration of k NN with clustering techniques has a greater precision value than simple classification with a k NN classifier. Considering results presented in Figure 5, F measure of k efficient clustering is best from other algorithms.
Figure 5. Results of integrating evaluating Table 3. Results of integrating evaluating Algorithm ACC Precision Recall F Measure k NN 81.2 84 88.7 86.2 k NN with K Means 91.5 94.7 93.3 93.8 k NN with K Medoids 94.7 88.4 97 92.5 k NN with K Efficient Clustering 100 100 100 100
Table 2. Results of clustering evaluating Algorithm Intra class inertia Inter class inertia NMI Running time K Means Clustering 4.9 3.4 1.4 2.2 K Medoids Clustering 3.11 7.5 1.8 8.5 K Efficient Clustering 2.9 6.9 2.2 7.2
4. DISSCUSSION Integration of k NN with clustering techniques has a greater precision value than simple classification with a k NN classifier. To aid in the early diagnosis of plant diseases, rapid and accurate models are required. For this article, we applied a data that included 18 different types of soybean diseases as well as a healthy class. The data was then split into train and test set for evaluating k NN classification algorithm without clustering algorithms and with algorithms of clustering were all trained applying 10 fold
Int J Artif Intell ISSN: 2252 8938 Improving prediction of plant disease using k efficient clustering and … (Asraa Safaa Ahmed) 945
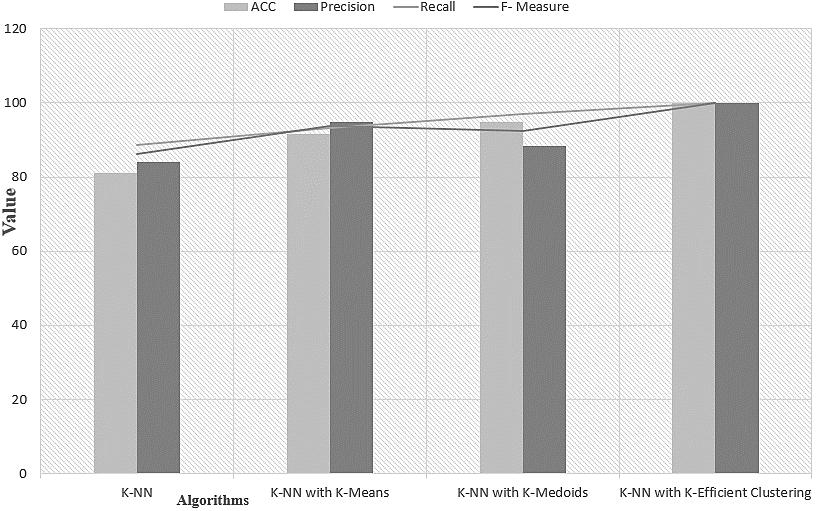
As shown in the results for the k NN classification algorithm with k efficient clustering tested on the data of soybean in Figure 5, the values of recall and precision are equal to 100, this refers that the number of TP classifications are much larger than the number of FN and FP classifications. If the k NN algorithm were being implemented without clustering, our values of recall and precision values would be much lower. The results of clustering obtained in this article are similar to those resulted in another article done by Drias et al. [34] on breast cancer data set and COIL 100 images dataset. These are used to identify the performance of integrating k means and k medoids clustering. The trained k NN classifier achieved an accuracy of 91.83% in the study implemented by Morgan et al., which compared to our 100% ACC achieved by our proposed model. We compared the result of k NN our proposed model by Maria study because they used the same dataset. However, the article makes an important contribution by building the possibility of using raw data depend on clustering and classification to predict plant disease. As refers in the section of introduction, which discusses the literature review, k means with k NN are almost applied articles of agricultural. We should think about this as k NN was a high performing algorithm in the soybean dataset [18]. One of the most significant challenges encountered during the preparation of this study was the lack of data included that the clustering algorithm not implemented truly on data that has been not preprocessed, so the preprocess step was very important and must be implemented for unbalance data. In clustering algorithms, data that are unstable require additional techniques of data preparation as to not skew the findings of the algorithms of classification. It would be interesting to test the proposed model with a different set of raw data, or to see if the models could categorize images of plant diseases as soon as they appeared. Farmers could benefit from using the suggested model, which combines k NN with k efficient clustering, to diagnose soybean and other plant diseases.
ISSN: 2252 8938 Int J Artif Intell, Vol. 11, No. 3, September 2022: 939 948 946(cross validation). The rows within the data are randomly restructured and divide into 10 folds with equal size. With each iteration of the training k NN, one fold is applied as the test data and 9 folds are applied as the training data. This technique is repeated ten times more until each fold is applied as the test data. The resultant classification model is an average of the training process’s 10 iterations. The study worked with seven experiments including k means, k medoids, k efficient clustering, k NN, k means with k NN, k NN with k medoids, and k NN with k efficient clustering. The evaluating of classification algorithms was different from evaluating the clustering algorithms, whereas k NN algorithm was subjected to an evaluation depend on the measures: F measure, precision, recall, and ACC. While evaluating clustering algorithms was based on NMI, intra class, and inter class. The results show that k NN with k efficient clustering had the best performance while k NN without clustering, posted the least performance. We expect this new technology will lead to huge new discoveries and be a highly valuable addition to the sector of agriculture.
REFERENCES
[1] A. M. Abdu, M. M. M. Mokji, and U. U. U. Sheikh, “Machine learning for plant disease detection: an investigative comparison between support vector machine and deep learning,” IAES Int. J. Artif. Intell., vol. 9, no. 4, pp. 670 683, Dec. 2020, doi: 10.11591/ijai.v9.i4.pp670 683. [2] S. B. Jadhav, “Convolutional neural networks for leaf image based plant disease classification,” IAES Int. J. Artif. Intell., vol. 8, no. 4, pp. 328 341, Dec. 2019, doi: 10.11591/ijai.v8.i4.pp328 341.
5. CONCLUSIONS In this article, we implement seven experiments for testing k means, k medoids, k efficient clustering, k NN, and k NN classifier with the three clustering algorithms to forecast presence of disease in classify and predict disease in a data of soybean. In the soybean dataset, we have shown that k NN with k efficient clustering are the best classifiers in terms of ACC, but k NN without clustering have less performance from other algorithms. The goal of these tests was to develop clustering and classification methods that could be applied to plant datasets that contained real measurements rather than images. The results of this study can be replicated using similar plant datasets, and they can also be used to train other classification algorithms with clustering for forecasting classification of disease in animal or human data with raw metrics. This article’s work decreases the monitoring effort for large scale agriculture, and the agricultural scale can profit greatly. This work produces a high quality product while minimizing the impact on plant productivity and economic profit in the resource progress. Finally, the proposed AI model for improving allowing agriculture to progress.
[3] M. A. I. Aquil and W. H. W. Ishak, “Evaluation of scratch and pre trained convolutional neural networks for the classification of Tomato plant diseases,” IAES Int. J. Artif. Intell., vol. 10, no. 2, pp. 467 475, Jun. 2021, doi: 10.11591/ijai.v10.i2.pp467 475. [4] T. U. Rehman, M. S. Mahmud, Y. K. Chang, J. Jin, and J. Shin, “Current and future applications of statistical machine learning algorithms for agricultural machine vision systems,” Comput. Electron. Agric., vol. 156, pp. 585 605, Jan. 2019, doi: 10.1016/j.compag.2018.12.006.
[10] R. M. Prakash, G. P. Saraswathy, G. Ramalakshmi, K. H. Mangaleswari, and T. Kaviya, “Detection of leaf diseases and classification using digital image processing,” in 2017 International Conference on Innovations in Information, Embedded and Communication Systems (ICIIECS), Mar. 2017, pp. 1 4., doi: 10.1109/ICIIECS.2017.8275915.
[16] B. Mariyappan, “Crop leaves disease identification using k means clustering algorithm and support vector machine,” 2020 [17] A. Yousuf and U. Khan, “Ensemble classifier for plant disease detection,” Int. J. Comput. Sci. Mob. Comput., vol. 10, no. 1, pp. 14 22, Jan. 2021, doi: 10.47760/ijcsmc.2021.v10i01.003.
Int J Artif Intell ISSN: 2252 8938 Improving prediction of plant disease using k efficient clustering and … (Asraa Safaa Ahmed) 947
[24] P. Govender and V. Sivakumar, “Application of k means and hierarchical clustering techniques for analysis of air pollution: A review (1980 2019),” Atmos. Pollut. Res., vol. 11, no. 1, pp. 40 56, Jan. 2020, doi: 10.1016/j.apr.2019.09.009. [25] A. Poompaavai and G. Manimannan, “Clustering study of indian states and union territories affected by coronavirus (COVID 19) using k means algorithm,” Int. J. Data Min. Emerg. Technol., vol. 9, no. 2, p. 43, 2019, doi: 10.5958/2249 3220.2019.00006.5.
[33] H. Drias, N. F. Cherif, and A. Kechid, “K MM: a hybrid clustering algorithm based on k means and k medoids,” in Advances in Intelligent Systems and Computing, Springer International Publishing, 2016, pp. 37 48., doi: 10.1007/978 3 319 27400 3_4. [34] H. Drias, A. Kechid, and N. Fodil Cherif, “A hybrid clustering algorithm and web information foraging,” Int. J. Hybrid Intell. Syst., vol. 13, no. 3 4, pp. 137 149, Feb. 2017, doi: 10.3233/HIS 160231.
[15] K. S. Sankaran, N. Vasudevan, and V. Nagarajan, “Plant disease detection and recognition using k means clustering,” in 2020 International Conference on Communication and Signal Processing (ICCSP), Jul. 2020, pp. 1406 1409., doi: 10.1109/ICCSP48568.2020.9182095.
[22] J. G. A. Barbedo, “Impact of dataset size and variety on the effectiveness of deep learning and transfer learning for plant disease classification,” Comput. Electron. Agric., vol. 153, pp. 46 53, Oct. 2018, doi: 10.1016/j.compag.2018.08.013.
[21] M. A. Khan et al., “An optimized method for segmentation and classification of apple diseases based on strong correlation and genetic algorithm based feature selection,” IEEE Access, vol. 7, pp. 46261 46277, 2019, doi: 10.1109/ACCESS.2019.2908040.
[14] G. Geetha, S. Samundeswari, G. Saranya, K. Meenakshi, and M. Nithya, “Plant leaf disease classification and detection system using machine learning,” J. Phys. Conf. Ser., vol. 1712, no. 1, p. 12012, Dec. 2020, doi: 10.1088/1742 6596/1712/1/012012.
[5] G. Prem, M. Hema, L. Basava, and A. Mathur, “Plant disease prediction using machine learning algorithms,” Int. J. Comput. Appl., vol. 182, no. 25, pp. 1 7, Nov. 2018, doi: 10.5120/ijca2018918049.
[27] F. Rahman, I. I. Ridho, M. Muflih, S. Pratama, M. R. Raharjo, and A. P. Windarto, “Application of data mining technique using k medoids in the case of export of crude petroleum materials to the destination country,” IOP Conf. Ser. Mater. Sci. Eng., vol. 835, no. 1, p. 12058, Apr. 2020, doi: 10.1088/1757 899X/835/1/012058.
[30] R. A. Jaleel, I. M. Burhan, and A. M. Jalookh, “A proposed model for prediction of COVID 19 depend on k nearest neighbors classifier:iraq case study,” in 2021 International Conference on Electrical, Communication, and Computer Engineering (ICECCE), Jun. 2021, pp. 1 6., doi: 10.1109/ICECCE52056.2021.9514171.
[8] M. G. Roth et al., “Integrated management of important soybean pathogens of the United States in changing climate,” J. Integr. Pest Manag., vol. 11, no. 1, Jan. 2020, doi: 10.1093/jipm/pmaa013.
[23] S. H. Toman, M. H. Abed, and Z. H. Toman, “Cluster based information retrieval by using (K means) hierarchical parallel genetic algorithms approach,” TELKOMNIKA (Telecommunication Comput. Electron. Control., vol. 19, no. 1, pp. 349 356, Feb. 2021, doi: 10.12928/telkomnika.v19i1.16734.
[32] R. Ramya, P. Kumar, D. Mugilan, and M. Babykala, “A review of different classification techniques in machine learning using weka for plant disease detection,” Int. Res. J. Eng. Technol., vol. 5, no. 5, pp. 3818 3823, 2018
[28] D. C. Corrales, “Toward detecting crop diseases and pest by supervised learning,” Ing. y Univ., vol. 19, no. 1, p. 207, Jul. 2015, doi: 10.11144/Javeriana.iyu19 1.tdcd. [29] J. Han, M. Kamber, and J. Pei, Data mining: concepts and techniques, 3rd ed. 2012.
[11] S. Bhuvana, B. K. Bharati, P. Kousiga, and S. R. Selvi, “Leaf disease detection using clustering optimization and multi class classifier,” WSEAS Trans. Comput. Arch., vol. 17, 2018 [12] V. V. Adit, C. V. Rubesh, S. S. Bharathi, G. Santhiya, and R. Anuradha, “A Comparison of Deep Learning Algorithms for Plant Disease Classification,” Adv. Cybern. Cogn. Mach. Learn. Commun. Technol. Lect. Notes Electr. Eng., vol. 643, pp. 153 161, 2020 [13] R. U. Khan, K. Khan, W. Albattah, and A. M. Qamar, “Image based detection of plant diseases: from classical machine learning to deep learning journey,” Wirel. Commun. Mob. Comput., vol. 2021, pp. 1 13, Jun. 2021, doi: 10.1155/2021/5541859.
[31] A. A. Amer and H. I. Abdalla, “A set theory based similarity measure for text clustering and classification,” J. Big Data, vol. 7, no. 1, p. 74, Dec. 2020, doi: 10.1186/s40537 020 00344 3.
[6] E. Fujita, Y. Kawasaki, H. Uga, S. Kagiwada, and H. Iyatomi, “Basic investigation on a robust and practical plant diagnostic system,” in 2016 15th IEEE International Conference on Machine Learning and Applications (ICMLA), Dec. 2016, pp. 989 992., doi: 10.1109/ICMLA.2016.0178.
[7] E. Khalili, S. Kouchaki, S. Ramazi, and F. Ghanati, “Machine learning techniques for soybean charcoal rot disease prediction,” Front. Plant Sci., vol. 11, Dec. 2020, doi: 10.3389/fpls.2020.590529.
[26] S. A. Abbas, A. Aslam, A. U. Rehman, W. A. Abbasi, S. Arif, and S. Z. H. Kazmi, “K means and k medoids: cluster analysis on birth data collected in city muzaffarabad, kashmir,” IEEE Access, vol. 8, pp. 151847 151855, 2020, doi: 10.1109/ACCESS.2020.3014021.
[18] M. Morgan, C. Blank, and R. Seetan, “Plant disease prediction using classification algorithms,” IAES Int. J. Artif. Intell., vol. 10, no. 1, pp. 257 264, Mar. 2021, doi: 10.11591/ijai.v10.i1.pp257 264. [19] D. Aha, “UCI machine learning repository.” 1987. [20] R. S. Michalski and R. L. Chilausky, “Learning by being told and learning from examples: an experimental comparison of the two methods of knowledge acquisition in the context of developing an expert system for soybean disease diagnosis,” Int. J. Policy Anal. Inf. Syst., vol. 4, no. 2, 1980
[9] G. Kaushal and R. Bala, “GLCM and KNN based algorithm for plant disease detection,” Int. J. Adv. Res. Electr. Electron. Instrum. Eng., vol. 6, no. 7, pp. 5845 5852, 2017, doi: 10.15662/IJAREEIE.2017.0607036.
ISSN: 2252 8938 Int J Artif Intell, Vol. 11, No. 3, September 2022: 939 948 948 BIOGRAPHIES OF AUTHORS
Asraa safaa ahmed holds a master’s degree Computer Science from the University of Diyala in 2019. She also received his B.Sc (Computer Science) from University of Diyala 2009 2010, My research includes machine learning, data mining, artificial intelligence, brain signal classification, and electromagnetic signal classification. She holds the scientific title of Assistant Lecturer at Diyala University in 2019. Published 6 research papers in international fields and scientific conferences from 2019 to 2021. She can be contacted at email: asraasafaa@uodiyala.edu.iq Zainab Kadhm Obeas she holds a master’s degree Computer Science from the University of Diyala in 2019. She also received his B.Sc. (Computer Science) from University of Babylonian 2007, My research includes machine learning, data mining, artificial intelligence, brain signal classification, and electromagnetic signal classification. She holds the scientific title of Assistant Lecturer at Al Qasim Green University in 2019. Published 4 research papers in international fields and scientific conferences from 2019 to 2021. She can be contacted at email: zainabkadhm1@gmail.com. Batool Abd Alhade she holds a master’s degree Computer Science from the University of Diyala in 2019. She also received his B.Sc. (Computer Science) from University of Babylon in 2008, My research includes machine learning, data mining, artificial intelligence, brain signal classification, and electromagnetic signal classification. She holds the scientific title of Assistant Lecturer at Al Qasim Green University in 2019. Published 4 research papers in international fields and scientific conferences from 2019 to 2021. She can be contacted at email: batool@uoqasim.edu.iq Refed Adnan Jaleel received the B.Sc. & M.Sc. degree in Information and Communications Engineering in 2014 and 2020, respectively, from Baghdad University Al Khawarizmi Engineering College and Al Nahrain University Information Engineering College, Baghdad, Iraq. Her research interests are in Internet of Things, Software Defined Networks, Security, Wireless Sensor Networks, Information Systems, Meta heuristic Algorithms, Artificial Intelligence, Machine Learning, Data Mining, Data Warehouse, Recommender Systems, Image Processing, Cloud Computing, Fuzzy Logic Techniques, and Database Management Systems. She worked as an Editor & Reviewer and she has published many articles in international journals and conference proceedings. She also has many certificates of participation in scientific symposia and electronic workshops. She can be contacted at email: iraq_it_2010@yahoo.com
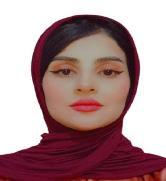



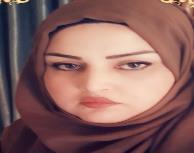



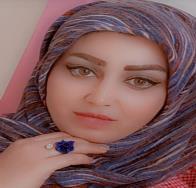



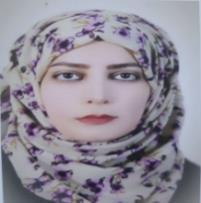



Journal homepage: http://ijai.iaescore.com
Sethu Selvi Selvan1, Sharath Delanthabettu2,3, Menaka Murugesan4, Venkatraman Balasubramaniam4 , Sathvik Udupa1, Tanvi Khandelwal1, Touqeer Mulla1, Varun Ittigi1 1Department of Electronics and Communication, M S Ramaiah Institute of Technology, Bengaluru, India 2Centre for Imaging Technology, M S Ramaiah Institute of Technology, Bengaluru, India 3Department of Chemistry, M S Ramaiah Institute of Technology, Bengaluru, India 4Safety, Quality and Resource Management Group, Indira Gandhi Center for Atomic Research, Kalpakkam, India
Keywords: Auto encoder Deep PulsedHigherDefectlearningdetectabilityorderstatisticsthermography
Article Info ABSTRACT
This is an open access article under the CC BY SA license. Corresponding Author: Sethu Selvi DepartmentSelvanofElectronics and Communication, M.S. Ramaiah Institute of Technology Bengaluru, Karnataka 560,054, India Email: selvi@msrit.edu 1. INTRODUCTION In nuclear, process and petrochemical industries regular inspection of in service components is important to detect and characterize service induced defects. This helps in enhancing the life cycle of the component and ensures the safety of the components and the workers. Non destructive evaluation (NDE) methods evaluate the inherent properties of materials and identify any defect or irregularity without damaging it. This not only helps in detecting defects but also predicts whether a defect is probable to occur in future or not which is a crucial factor in preventing major crisis in an industry. Deep learning approaches are widely used in the field of non destructive testing (NDT) to enhance the signal to noise ratio (SNR) and thus improving the defect visibility and to measure the size and depth of defects automatically. Pulsed thermography (PT) is one of the advanced NDE methods in which the front side of the object under inspection is exposed to a short and high energy optical pulse [1]. The front surface of the object absorbs the optical energy and converts it to thermal energy resulting in increase of surface temperature. As the rear end of the object is at ambient temperature, thermal waves diffuse from front surface to rear end causing decrease in front surface temperature. Any defect in the material alters this diffusion rate and the surface temperature above it, which is easily detected by an infrared camera [2]. It allows materials to be inspected very quickly for near surface defects and bonding weakness. Compared to conventional techniques
Article history: Received Oct 7, 2021 Revised May 26, 2022 Accepted Jun 3, 2022 Non destructive evaluation (NDE) is very essential in measuring the properties of materials and in turn detect flaws and irregularities. Pulsed thermography (PT) is one of the advanced NDE technique which is used for detecting and characterizing subsurface defects. Recently many methods have been reported to enhance the signal and defect visibility in PT In this paper, a novel unsupervised deep learning based auto encoder (AE) approach is proposed for enhancing the signal to noise ratio (SNR) and visualize the defects clearly. A detailed theoretical background of AE and its application to PT is discussed. The SNR and defect detectability results are compared with the existing approaches namely, higher order statistics (HOS), principal component thermography (PCT) and partial least square regression (PLSR) thermography. Experimental results show that AE approach provides better SNR at the cost of defect detectability.
IAES International Journal of Artificial Intelligence (IJ AI) Vol. 11, No. 3, September 2022, pp. 949 960 ISSN: 2252 8938, DOI: 10.11591/ijai.v11.i3.pp949 960 949
A deep learning approach based defect visualization in pulsed thermography
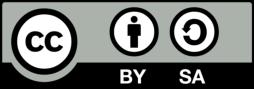
ISSN: 2252 8938 Int J Artif Intell, Vol. 11, No. 3, September 2022: 949 960 950like radiography and ultrasound testing, PT has advantages like non contactless measurement and fast inspection rate, whereas the limitation is that it is confined to surface and subsurface defect detection.
Noise is an unwanted but integral part of a signal. In PT, noise is associated with the temperature response recorded in infrared (IR) camera. This noise can affect the detection of deeper and smaller defects. Reduction of such noise is essential, which can be considered as a post processing step. In PT numerous methods have been reported for reduction of noise and thus improve SNR, an important parameter which indicates the effectiveness of noise reduction. Some of the important methods based on statistical and regression based algorithms are higher order statistics (HOS) [3], thermal signal reconstruction (TSR) [4], principal component thermography (PCT) [5], and partial least square thermography (PLST) [6]. In recent years the trend has been shifted towards neural network (NN) based algorithms for signal enhancement.
Extensive work has been reported in the field of automated detection and characterization of defects in PT [7] [12], [13] [18]. Various neural networks like multilayer back propagation NN [7] [10], [12], [13], Kohonen and perceptron based NN [11], convolutional neural network (CNN) [14] and deep feed forward NN [15] [17] have been used for defect detection and depth quantification in materials like plastics, composites and aluminium. These algorithms provide reasonable accuracy in defect detection and depth estimation. Apart from defect depth and size estimation, improving the defect visibility by enhancing the SNR is also important. SNR enhancement using neural networks has not been explored in PT. In [18] stacked auto encoder (AE) method was reported for enhancing the delamination visibility in composites using PT. In this approach, pre processed temporal pixel information is used to train the neural network. The study showed that this method significantly improved the delamination contrast. In this paper, an unsupervised AE based neural network approach is explored to enhance SNR in PT experiments for AISI 316 L Stainless Steel material and the performance is compared with other approaches, which gives one or few images as output, namely HOS, PCT, and PLST in terms of SNR and defect visibility. Similar to HOS, the output is a single image obtained from 3D raw PT data. This saves on the time for inspection, as one need not go through the complete image sequence to locate the defect. The paper is organized as follows: section 2 provides a detailed theoretical background on PT and the existing algorithms for signal enhancement. Section 3 elaborates the proposed algorithm for enhancing PT signal and section 4 provides the comparison of the performance of the proposed algorithm with the existing algorithms. Finally, the paper is concluded and future directions for the proposed work is provided in section 5. 2. THEORETICAL BACKGROUND AND EXISTING ALGORITHMS
2.1. Pulsed thermography (PT) In PT, a short and high power pulse is impinged on the surface of the object under inspection. The absorption of the optical pulse by the surface of the object results in instantaneous increase in its surface temperature. The diffusion of heat then results in decrease in the surface temperature which is monitored using an IR camera. Any interference, like a defect, alters the diffusion rate, which causes a change in the surface temperature picked up by the IR camera. The signal acquired by PT is of three dimensions with spatial information (camera pixels) recorded as a function of time. If ���� ���� is the resolution or the number of pixels captured by the IR camera and ���� =���� �� is the total number of thermal images, where ���� is the frame rate and �� is the duration of the PT experiment, then the raw PT data set is of size ���� ���� ����. The schematic diagram of the PT set up is shown in Figure 1. Figure 1. Schematic diagram of PT experimental set up
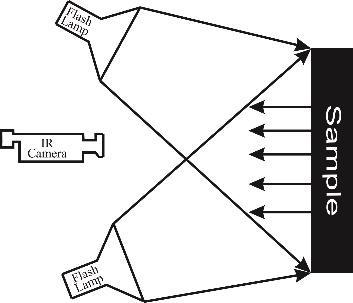
2.2. Higher order statistics (HOS)
The temperature response signals of PT tend to be monotonic and, so oscillatory basis functions are inappropriate. This method [5] constructs a set of empirical orthogonal functions (EOF) which are statistical modes that provide the strongest projection for the data and offers a very compact representation. The thermal behavior associated with underlying defects of a material is compactly described through a singular value decomposition (SVD) of the data matrix. In general, any matrix �� of size ���� ���� can be decomposed as in (2): �� =�������� (2) where the three matrices are: �� whose columns comprise the set of EOF that models spatial variations, �� is a diagonal matrix with singular values on its diagonal and rows of ���� are the principal component vectors which describe the characteristic time behaviour. The first few columns of matrix �� are used to reconstruct the data to reduce the redundancy in the original data set.
In contrast to PCA where the matrix �� describes the variance, partial least squares regression (PLSR) [6] computes loadings (��) and scores (��) vectors that are correlated with the predicted matrix ��, which describes the variation in matrix �� similar to principal component regression. The matrix �� is the surface temperature matrix, while �� is the observation time of the thermal images. The result of the bilinear decomposition is a new set of thermal images and observation time vector composed of latent variables in a new subspace which considers only the most important variations.
2.4. Partial least square thermography (PLST)
PLSR is based on the basic latent component decomposition of �� and �� matrices into a combination of loadings, scores and residuals [6]. Mathematically, the PLS model is expressed as in (3) and (4). The scores are orthogonal and are expressed as linear combinations of the original variables �� with the coefficients �� as expressed in (5): �� =������+�� (3) �� =������+�� (4)
Int J Artif Intell ISSN: 2252 8938 A deep learning approach based defect visualization in pulsed thermography (Sethu Selvi Selvan) 951
HOS analysis [3] is employed to process IR images and to compress the most useful information into a unique image for inspection. PT response with respect to time is described through its statistical behaviour. This statistical behaviour is used to analyze the different characteristics of thermal images as higher order statistical parameters have a relation to thermal conductivity in the longitudinal direction of a material. Various statistical moments such as skewness, kurtosis, hyper skewness and hyper flatness are considered, and these moments are then combined to form one image for each of the statistical moment. The ����ℎ standardized central moment is calculated using (1) as skewness is 3rd order central moment, kurtosis is 4th order central moment, hyper skewness is 5th order and hyper flatness is 6th order central moment: ���� = ��[(�� ��[��])��] ���� (1) where �� is the data distribution, �� is the standard deviation and ��[��] is the mean of ��. Skewness represents a measure of symmetry, or the lack of symmetry of a distribution. Kurtosis characterizes the heaviness of the tail of the distribution, compared to the normal distribution. Hyper skewness measures the symmetry of the tails, while hyper flatness measures similarly but with heavier focus on outliers than the fourth moment. Odd order moments quantify relative tailedness and even order moments quantify total tailedness. Standardized central moments of higher order provide larger values due to the higher power terms and cannot be defined physically as they are associated with the presence of outliers. Kurtosis and skewness are used for enhancing the defect visibility and SNR in PT. 2.3. Principal component thermography (PCT)
Principal component analysis (PCA) applied to PT data in the form of thermogram sequence is called PCT [5] and cannot be applied directly to a 3 D thermographic data matrix. A pre processing step of data unfolding is necessary which converts the 3D matrix of size ���� ���� ���� to a 2D expanded matrix ((���� ���� )����). The unfolded data matrix is normalized by subtracting the mean from each column divided by standard deviation of that column. The normalisation ensures that pixel to pixel variations does not influence the decomposition. PCT yields high levels of thermal contrast for underlying defects in composite materials which results in defect detection compared to conventional thermographic algorithms.
In this study [20], artificial intelligence was applied in combination with infrared thermography to detect and segment defect on laminates. Segmentation was performed on both mid wave and long wave infrared sequences obtained during PT experiments through a deep neural network for each wavelength. The F1 score for mid wave images based model is 92.74%, while for long wave images is 87.39%.
ISSN: 2252 8938 Int J Artif Intell, Vol. 11, No. 3, September 2022: 949 960 952 �� =���� (5) where �� is the score matrix, �� and �� are loading or coefficient matrices and describe how the variables in �� relate to the original matrices �� and ��. �� and �� are residual matrices and represent noise or irrelevant variability in �� and �� respectively.
In this paper [21], synthetic data from the standard finite element models (FEM) are combined with experimental data to build large datasets with mask region based convolutional neural networks (Mask RCNN), learn essential features of objects of interest and achieve defect segmentation automatically. The results prove the efficiency of adapting inexpensive synthetic data together with the experimental dataset for training the neural networks to obtain an achievable performance from a limited collection of the annotated experimental data of a PT experiment. In this paper [22], an artificial neural network (ANN) is employed to detect depth of the defects in composite samples, coupled with PT. The study presents a proof of concept using a Multiphysics FEM simulation model of the inspection process, to generate a training dataset and the proposed NN was further tested experimentally to validate its accuracy and performance. The accuracy of the developed NN for the synthetic data was more than 97% and for the experimental data was around 90%.
3. AUTOENCODER BASED DEFECT VISUALIZATION
Once the scores matrix �� is obtained, the loading matrices �� and �� are estimated by regression of �� and �� onto ��. The residual matrices �� and �� are found by subtracting the estimated versions of ������ and ������ from �� and ��, respectively. The regression coefficients are obtained using (6) and the regression model is given as in (7). �� =������ (6) �� =����+�� =��������+�� (7) 2.5. Deep learning based algorithms Infrared imaging based PT is used to automatically inspect, detect, and analyse infrared images. Passive thermography is based on visible light and is a imaging tool for self heating objects such as the human body and electrical power devices. Active thermography is a NDT method for quality and safety evaluation of non self heating objects. The rapid development of deep learning makes PT more intelligent and highly automated, thus considerably increasing its range of applications. This paper [19] reviews the principle, cameras, and PT data to discuss the applications of deep learning.
Kovács et al. [23] investigate two deep learning approaches to recover temperature profiles from PT images in NDT. In the first method, a deep neural network (DNN) was trained in an end to end fashion with surface temperature measurements as input. In the second method, the surface temperature measurements were converted to virtual waves, which was then fed to the DNN. For a dataset of 1,00,000 simulated temperature measurement images both the end to end and hybrid approach outperformed the baseline algorithms in terms of reconstruction accuracy. The end to end approach requires less domain knowledge and is computationally efficient. The hybrid approach requires extensive domain knowledge and is computationally expensive than the end to end approach. The virtual waves are useful features, that yields better reconstructions with the same number of training samples compared to the end to end approach. It also provided more compact network architectures and is suitable for NDT in two dimensions. In [24] Fang et al. propose a specific depth quantifying technique by using gated recurrent units (GRUs) in composite material samples via PT. The proposed GRU model automatically quantified the depth of defects and was evaluated for accuracy and performance of synthetic carbon fiber reinforced polymer (CFRP) data from FEM for defect depth predictions. In [25], Lou et al. propose a spatial and temporal hybrid deep learning architecture, which has the capability to significantly minimize the uneven illumination and enhance the detection rate. The results show that Visual Geometry Group Unet (VGG Unet) significantly improves the contrast between the defective and non defective regions.
Neural network architectures have seen tremendous success in the past few years. CNN is one of the key architectures which performs well on a lot of tasks such as image classification and object detection. It does so by capturing the inherent features of the images in the convolution layers. The convolution layers
output(����)=bias(����)+∑ weight(����)input(��)������ 1 ��=0 (8) ��������(��)=max(0,��) (9)
�� =∑ ∑ ∑ [��(��,��) �� ��(��,��) �� ] ��2 ��=1 �� ��=1 �� ��=1 (11)
In the encoder section, multiple convolution operations are applied to learn a large number of feature maps. These feature maps contain predominant information from the input images. These feature maps are reconverted back into the shape of input images in the decoder section. Training this network over the input images optimizes a loss function to output a new image with considerable SNR improvement.
Figure 2. Autoencoder structure
In the decoder, different transpose convolution operations are stacked which upsamples the image back to its original size. A sigmoid activation function as shown in (10) is included at the end of last layer, which squashes the values between 0 and 1 providing the probability values.
��(��)= 1 1+�� �� (10)
To train this network, mean squared error as in (11) is used as a pixel level loss function between original and reconstructed images which employs Adam optimizer based gradient descent algorithm. Hyperparameters are chosen for loss convergence and which provides a performance exceeding a pre defined SNR threshold.
A deep learning approach based defect visualization in pulsed thermography (Sethu Selvi Selvan) 953 perform convolution operations over the image with a set of kernels, and in this case, convolution refers to Hadamard product. These layers are stacked together many times and additional methods are incorporated in between such as pooling and activation functions. These set of layers are then optimised towards a target and the loss encured is backpropagated. This process modifies the kernel weights until the loss converges. Once the architecture is optimised, the kernels capture the subtle features in the dataset and represents them in a very high dimension. The integration property of CNNs aids in feature representation.
The proposed algorithm consists of 3 layers of convolution followed by 3 layers of transpose convolution. The number of input channels vary as 1 8 16 8 1 during this process. Convolution filters of size 11, 22 and 33 is considered. A batch size of 4 was considered as there were less number of images for training and learning rate was set to 1×10 4 .
Int J Artif Intell ISSN: 2252 8938
The architecture consists of convolution layers denoted by ����������,�� =1,2,3 and transpose convolution layers ������������,�� =1,2,3. After each convolution layer an activation function ��(��)=��������(��) is used. In the last layer of transpose convolutions, another function ��(��) =��������������(��) is used. The convolution operation in each layer produces a feature map after it is multiplied with predefined number of kernels as shown in (8), where ���� is the feature map of layer ��, ������ is the dimension of the input to ����ℎ layer and ‘weight’ and ‘bias’ are the parameters learnt by the network. During convolution, rectified linear units (ReLu) defined in (9) are inserted as activation functions to include non linearity and to help in loss convergence.
Autoencoder is an unsupervised learning algorithm which tries to learn the function ℎ(��)≈��. A well trained function ℎ(��) generates a new thermal image with better representation of features compared to any single input image obtained from input images used for training. The structure of the autoencoder is as shown Figure 2
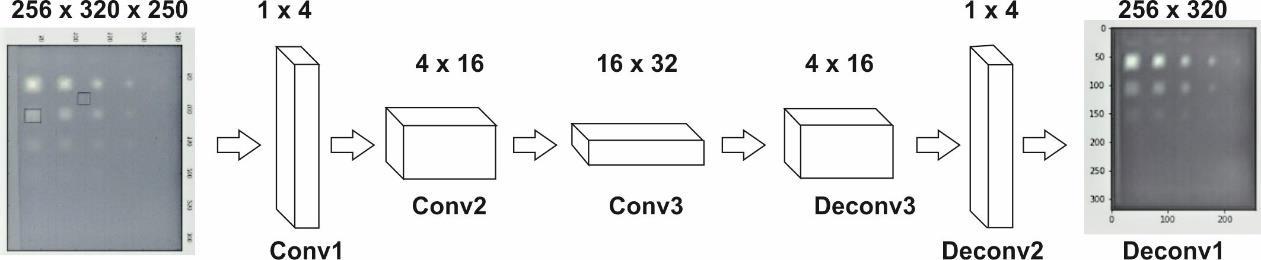
The thermal images acquired are stored as a 3D matrix. The spatial x and y coordinates correspond to the pixel locations, respectively, and the z coordinate represents time. The acquisition frequency (fs) is 125 Hz (Sampling time t = 1/fs = 0.008s), which is the maximum full frame rate achieved for a 256×320 pixel array. Furthermore, a total of N = 250 frames were collected during the cooling regime, where the acquisition window is t = 2s. For analysing the proposed algorithm, different defects of varying sizes and depths are considered as depicted in Table 1 and in the defect name R indicates the defect row number and C indicates the defect column number in the thermal images.
Figure 3. Photograph of a sample
PERFORMANCE COMPARISON
4.3. Data acquisition
4.1. Material
ISSN: 2252 8938 Int J Artif Intell, Vol. 11, No. 3, September 2022: 949 960 954 4.
Table 1. Defects considered for analysis Sl. No. Defect Name Dimensions Depth 1. R1C2 10×10 mm 0.4 mm 2. R2C2 8×8 mm 0.4 mm 3. R1C3 10×10 mm 1.13 mm 4. R2C3 8×8 mm 1.13 mm 5. R1C4 10×10 mm 1.78 mm 6. R2C4 8×8 mm 1.78 mm 4.4. Higher order statistics (HOS)
The PT image is reconstructed using four different statistical moments. The SNR for different defects have been calculated and tabulated in Table 2. From the results, it is observed that maximum SNR is obtained with skewness based reconstruction in most of the defects. Kurtosis based reconstructed image also provides good SNR improvement but lower than skewness. It is also observed that hyper skewness and hyper flatness provide smaller SNR values than that of the unprocessed raw image.
For the study, AISI 316L grade stainless steel plate of dimension 150×100×3.54 mm with artificially induced defects of size 10 mm, 8 mm, 6 mm, 4 mm and 2 mm at depths 0.4 mm, 1.13 mm, 1.78 mm, 2.48 mm, 3.17 mm, and 3.36 mm was used. The front surface of the sample was coated with black paint to improve the emissivity and light absorption. The photograph of the sample is given in Figure 3
4.2. Experimental set up CEDIP Silver 420 infrared camera was used for the experiment. The camera has a focal plane array of 320×256 pixels which is made up of Indium Antimonide (InSb) detector with Stirling cooling system. It detects the infrared radiations in 3 5μm region. The maximum achievable temperature resolution is 25 mK with a frame rate of 176 Hz. For PT experiment, two Xenon flash lamps of power 1600 W each were used. The flash duration was less than 2 ms Experiment was carried out in reflection mode. The non defective area temperature decreases until stabilization is reached.
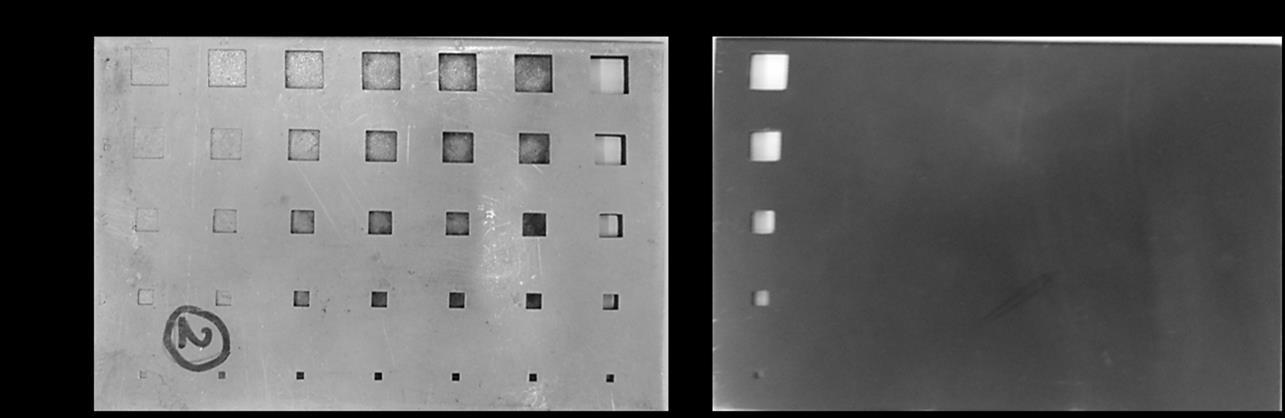
The first five components of the EOF matrix are reconstructed into 5 images along with the raw image for analysis as shown in Figure 4. These five components were extracted from the PCA of the reshaped thermal data. By extracting the most dominant features, the defects are predominantly seen in the feature space.Theprincipal components provide a very high contrast image with respect to the defects. This helps in clear visibility of the defects and also increases the SNR. The SNR values of six defects are tabulated for the first five principal components and then compared with the SNR values of the defects in the raw image as tabulated in Table 3. From the illustrations, it is observed that the first principal component corresponding to EOF 1 preserves more information about the defects and also visibility and contrast reduces in further components, when compared with the raw image.
Table 2. SNR in dB for HOS based algorithm Sl. No. Defect SNR (dB) Raw Image Skewness Kurtosis Hyper skewness Hyper flatness 1. R1C2 32.090 37.346 32.264 24.354 22.651 2. R1C3 20.968 27.611 27.480 17.897 16.877 3. R2C2 33.091 36.877 32.017 22.400 22.190 4. R2C3 18.639 11.198 6.689 18.464 17.290 5. R3C2 31.478 37.116 32.129 23.967 22.332
4.5. Principal component thermography (PCT)
Int J Artif Intell ISSN: 2252 8938
A deep learning approach based defect visualization in pulsed thermography (Sethu Selvi Selvan) 955
Figure 4. Different components obtained through PCT Table 3. Different principal components obtained after applying PCT Sl. No. Defect SNR (dB) Raw Image EOF 1 EOF 2 EOF 3 EOF 4 EOF 5 1. R1C2 32.09 56.382 39.378 39.368 43.669 35.288 2. R2C2 33.09 61.486 45.451 40.381 45.032 35.314 3. R1C3 31.48 58.098 44.312 41.922 42.895 33.700 4. R2C3 18.63 59.561 46.183 44.597 43.606 34.660 5. R1C4 14.75 55.890 44.763 44.403 41.817 32.928 6. R2C4 7.92 63.898 47.653 47.882 44.292 35.268 4.6. Partial least square regression (PLSR) Figure 5 provides the PLS components of the defects considered for the experiment. The SNR values of six defects were tabulated for the first six PLS components in Table 4 and then compared with the SNR values of the defects in the raw image. It is observed that various defect depths achieve maximum SNR in different PLS components.
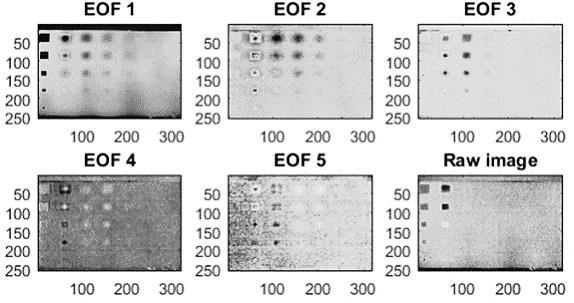
4.8. Comparison of SNR for different methods
The performance of defect detection is compared in terms of SNR obtained by different methods and tabulated in Table 6. From the table it is observed that PCT performs better than other signal processing algorithms in terms of SNR increase. The proposed deep learning architecture based autoencoder exhibits the best SNR for all the defects. As shown in the table, reconstructed images from randomly sampled input thermal images exhibit a higher SNR for the proposed autoencoder architecture compared to other signal processing approaches. This observation shows promise on how further research in this area on customised algorithms and objective functions can result in optimal performance without any fine tuning.
Figure 5. Different PLS components obtained after applying PLSR
Table 4. SNR values of different defects for varying PLS components Sl. No. Defect SNR (dB) Raw Image PLS 1 PLS 2 PLS 3 PLS 4 PLS 5 PLS 6 1. R1C2 32.09 53.77 40.09 25.26 5.89 23.55 13.47 2. R2C2 33.09 54.27 38.94 10.18 2.86 23.92 13.66 3. R1C3 31.48 44.94 16.85 31.35 2.32 19.84 2.20 4. R2C3 18.63 33.41 26.57 29.62 8.63 11.44 7.69 5. R1C4 14.75 46.25 19.86 23.38 15.81 20.61 3.60 6. R2C4 7.92 54.84 6.14 23.22 14.43 18.06 4.72
ISSN: 2252 8938 Int J Artif Intell, Vol. 11, No. 3, September 2022: 949 960 956
4.7. Autoencoder based approach
The tradeoff considered in this work is SNR improvement vs defect visibility. The autoencoder architecture is capable of providing output images with higher SNR, though the defects may not be visible always. Depending on the requirements or specifications, the parameters defined for the neural network is varied to maximize either SNR or defect visibility. The reconstructed images for R1C2 defect for varying SNR are shown in Figure 6. The images in Figure 6(a) to Figure 6(i) are the reconstructed outputs from randomly sampled input thermal images. The changes in SNR obtained during different epochs of training the autoencoder is presented in Figure 7 and maximum SNR obtained is tabulated in Table 5. Neural networks perform very well in optimizing an objective function. In this case, the network is trained to reconstruct the images. The non linearity of the model and differing statistics of the training data ensure that the reconstructed image is not a copy of the input. This is utilised to select the parameters for which the reconstructed images provide a higher SNR and have good amount of defect visibility. This has a drawback of not providing the best or optimum performance unless fine tuned.
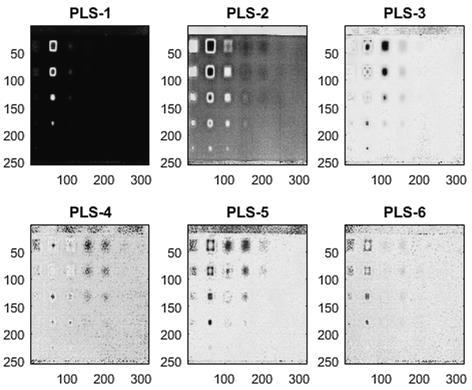
Int J Artif Intell ISSN: 2252 8938 A deep learning approach based defect visualization in pulsed thermography (Sethu Selvi Selvan) 957 (a) (b) (c) (d) (e) (f) (g) (h) (i) Figure 6 Reconstructed images for R1C2 defect with varying SNR (a) SNR=32.04 dB, (b) SNR=40.17 dB, (c) SNR=120.82 dB, (d) SNR=42.73 dB, (e) SNR=124.08 dB, (f) SNR=37.38 dB, (g) SNR=117.84 dB, (h) SNR=39.08 dB, and (i) SNR=38.06 dB Figure 7. SNR (dB) variation for various defects
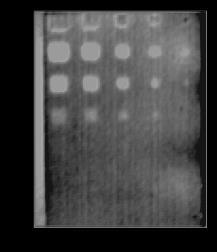
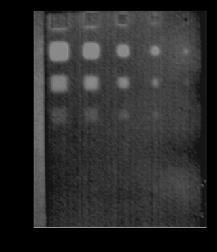
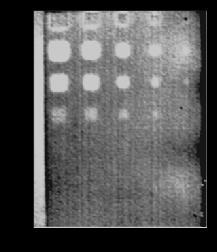
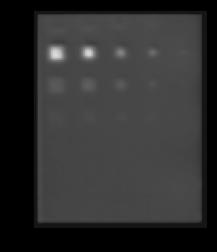
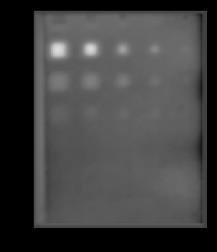
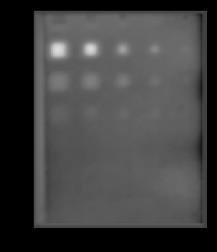
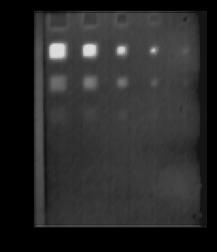
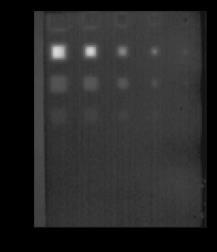
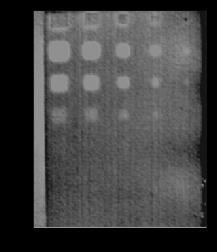
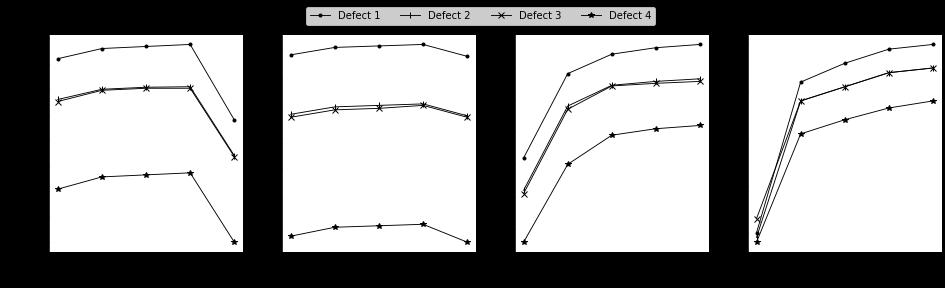
[8] H. Trétout, D. David, J. Y. Marin, M. Dessendre, M. Couet, and I. Avenas Payan, “An evaluation of artificial neural networks applied to infrared thermography inspection of composite aerospace structures,” in Review of Progress in Quantitative Nondestructive Evaluation, Boston, MA: Springer US, 1995, pp. 827 834., doi: 10.1007/978 1 4615 1987 4_103.
[12] A. Darabi and X. Maldague, “Neural network based defect detection and depth estimation in TNDE,” NDT E Int., vol. 35, no. 3, pp. 165 175, Apr. 2002, doi: 10.1016/S0963 8695(01)00041 X. [13] N. Saeed, M. A. Omar, and Y. Abdulrahman, “A neural network approach for quantifying defects depth, for non destructive testing thermograms,” Infrared Phys. Technol., vol. 94, pp. 55 64, Nov. 2018, doi: 10.1016/j.infrared.2018.08.022.
The evaluation and extensive analysis of standard signal processing approaches provided a good baseline for SNR improvement and defect visibility. HOS not being used for defect visualization improved the SNR of the defects. PCT displayed considerable contrast enhancement between defect and non defect regions which led to better defect visualization and higher SNR values as compared to other algorithms. PLSR provided the best performance among all the algorithms in terms of SNR improvement, defect visibility and contrast enhancement. The proposed autoencoder architecture based algorithm performs better than signal processing approaches with noticeable increase in SNR. It improves defect visibility in some cases and this can be attributed to embedding the input data into higher dimensions using convolution kernels and using non linear activation functions in this space. During reconstruction, activation function is used only at the last layer to normalise the output. The advantage is that large convolution filters are not necessary for this task. This is due to the fact that representation in a higher dimensional space is more important than learning large dimensions spatial information for this task. This network when optimised can represent and reconstruct any given image effectively. It also helps in data compression with the architecture able to learn the important features. Some of the limitations of this algorithm is that neural network architectures do not perform well with distribution shift, i.e., when the test set is statistically very different from the training set. Normalization or adaptative algorithms may ensure that test data is similar to the data the model is trained on.
[7] P. Bison, C. Bressan, R. Di Sarno, E. Grinzato, S. Marinetti, and G. Manduchi, “Thermal NDE of delaminations in plastic materials by neural network processing,” 1994., doi: 10.21611/qirt.1994.032.
[4] S. M. Shepard, “Reconstruction and enhancement of active thermographic image sequences,” Opt. Eng., vol. 42, no. 5, May 2003, doi: 10.1117/1.1566969.
[6] F. Lopez, C. Ibarra Castanedo, V. de Paulo Nicolau, and X. Maldague, “Optimization of pulsed thermography inspection by partial least squares regression,” NDT E Int., vol. 66, pp. 128 138, Sep. 2014, doi: 10.1016/j.ndteint.2014.06.003.
[9] G. Manduchi, S. Marinetti, P. Bison, and E. Grinzato, “Application of neural network computing to thermal non destructive evaluation,” Neural Comput. Appl., vol. 6, no. 3, pp. 148 157, Sep. 1997, doi: 10.1007/BF01413826.
These models are still in its nascent stage compared to signal processing approaches and neural networks are indeed a high dimensional learnable black box function, and so the generated models are not explainable. To conclude, signal processing and neural network based algorithms provide a set of tools to increase the SNR, enhance defect visibility and provide data compression in many applications for thermal imaging of stainless steel material with PT approach. Neural network architectures show a lot of promise for this application. Emerging neural network based approaches such as generative adverserial networks (GANs), variational autoencoders (VAE) and attention based networks can be considered to represent thermal images for better SNR and defect visibility as compared to autoencoders.
ISSN: 2252 8938 Int J Artif Intell, Vol. 11, No. 3, September 2022: 949 960 958Table 5. Maximum SNR values of different defects Sl. No. Defect SNR (dB) Raw Image AE 1. R1C2 32.09 65.180 2. R2C2 33.09 73.430 3. R1C3 31.48 73.120 4. R2C3 18.63 77.670 Table 6. SNR in dB of different algorithms No.Sl. Defect SNR (dB) ImageRaw HOS PCT PLSR AE 1. R1C2 32.09 37.346 56.382 53.770 65.180 2. R2C2 33.09 27.611 58.098 44.940 73.430 3. R1C3 31.48 36.877 61.486 54.270 73.120 4. R2C3 18.63 11.198 59.561 33.410 77.670
[2] X. Maldague, Theory and practice of infrared technology for non destructive testing. Wiley Interscience, 2001.
[10] M. B. Saintey and D. P. Almond, “An artificial neural network interpreter for transient thermography image data,” NDT E Int., vol. 30, no. 5, pp. 291 295, Oct. 1997, doi: 10.1016/S0963 8695(96)00071 0. [11] S. Vallerand and X. Maldague, “Defect characterization in pulsed thermography: a statistical method compared with Kohonen and perceptron neural networks,” NDT E Int., vol. 33, no. 5, pp. 307 315, Jul. 2000, doi: 10.1016/S0963 8695(99)00056 0.
[14] N. Saeed, N. King, Z. Said, and M. A. Omar, “Automatic defects detection in CFRP thermograms, using convolutional neural
[5] N. Rajic, “Principal component thermography for flaw contrast enhancement and flaw depth characterisation in composite structures,” Compos. Struct., vol. 58, no. 4, pp. 521 528, Dec. 2002, doi: 10.1016/S0263 8223(02)00161 7.
5. CONCLUSION
REFERENCES
[1] S. K. Lau, D. P. Almond, and J. M. Milne, “A quantitative analysis of pulsed video thermography,” NDT E Int., vol. 24, no. 4, pp. 195 202, Aug. 1991, doi: 10.1016/0963 8695(91)90267 7.
[3] F. J. Madruga, C. Ibarra Castanedo, O. M. Conde, J. M. López Higuera, and X. Maldague, “Infrared thermography processing based on higher order statistics,” NDT E Int., vol. 43, no. 8, pp. 661 666, Nov. 2010, doi: 10.1016/j.ndteint.2010.07.002.
[21] Q. Fang, C. Ibarra Castanedo, and X. Maldague, “Automatic defects segmentation and identification by deep learning algorithm with pulsed thermography: synthetic and experimental data,” Big Data Cogn. Comput., vol. 5, no. 1, Feb. 2021, doi: 10.3390/bdcc5010009.
[16] N. Saeed, H. Al Zarkani, and M. A. Omar, “Sensitivity and robustness of neural networks for defect depth estimation in CFRP composites,” J. Non destructive Eval., vol. 38, no. 3, Sep. 2019, doi: 10.1007/s10921 019 0607 4.
[17] Y. Duan et al., “Automated defect classification in infrared thermography based on a neural network,” NDT E Int., vol. 107, Oct. 2019, doi: 10.1016/j.ndteint.2019.102147.
Int J Artif Intell ISSN: 2252 8938 A deep learning approach based defect visualization in pulsed thermography (Sethu Selvi Selvan) 959 networks and transfer learning,” Infrared Phys. Technol., vol. 102, Nov. 2019, doi: 10.1016/j.infrared.2019.103048.
[20] Z. Wei, H. Fernandes, H. G. Herrmann, J. R. Tarpani, and A. Osman, “A deep learning method for the impact damage segmentation of curve shaped CFRP specimens inspected by infrared thermography,” Sensors, vol. 21, no. 2, Jan. 2021, doi: 10.3390/s21020395.
[25] Q. Luo, B. Gao, W. L. Woo, and Y. Yang, “Temporal and spatial deep learning network for infrared thermal defect detection,” NDT E Int., vol. 108, p. 102164, Dec. 2019, doi: 10.1016/j.ndteint.2019.102164.
[15] D. Müller, U. Netzelmann, and B. Valeske, “Defect shape detection and defect reconstruction in active thermography by means of two dimensional convolutional neural network as well as spatio temporal convolutional LSTM network,” Quant. Infrared Thermogr. J., vol. 19, no. 2, pp. 126 144, 2022, doi: 10.1080/17686733.2020.1810883.
BIOGRAPHIES OF AUTHORS
[18] C. Xu, J. Xie, C. Wu, L. Gao, G. Chen, and G. Song, “Enhancing the visibility of delamination during pulsed thermography of carbon fiber reinforced plates using a stacked autoencoder,” Sensors, vol. 18, no. 9, Aug. 2018, doi: 10.3390/s18092809.
Sethu Selvi Selvan Professor, Department of ECE, Ramaiah Institute of Technology obtained her Ph.D from Indian Institute of Science in 2001 under Prof. Anamitra Makur in the area of Image Compression. She completed her B.E from Thiagarajar College of Engineering, Madurai in 1992 and M.E from Anna University in 1994. She joined the Faculty of Department of Electronics and Communication at M.S. Ramaiah Institute of Technology, Bangalore, in 2002 as Assistant Professor. She has numerous publications to her name in the field of Machine Learning, Pattern Recognition and Signal and Image Processing. Her fields of interests are Digital Image Processing, Machine/Deep Learning, Video Processing, Character Recognition and Biometrics. She has authored a chapter titled “Image Algebra and Image Fusion” in the book “Data Fusion Mathematics: Theory and Practice”, CRC Press, 2017 and has been listed as a noteworthy technical contributor by Marquis Who's Who (World), 2009. She can be contacted at email: selvi@msrit.edu
Sharath Delanthabettu is working as Research Scientist in Center for Imaging Technologies at M S Ramaiah Institute of Technology, Bengaluru. He has obtained his Ph.D. from Homi Bhabha National Institute, IGCAR Kalpakkam Campus in 2015. He is working in the areas of Infrared Imaging and its applications in Non Destructive Evaluation and health care, and image processing. He has published 18 articles in international and national journals. He can be contacted at email: sharathd@msrit.edu
[19] Y. He et al., “Infrared machine vision and infrared thermography with deep learning: a review,” Infrared Phys. Technol., vol. 116, Aug. 2021, doi: 10.1016/j.infrared.2021.103754.
[22] N. Saeed, Y. Abdulrahman, S. Amer, and M. A. Omar, “Experimentally validated defect depth estimation using artificial neural network in pulsed thermographyy,” Infrared Phys. Technol., vol. 98, pp. 192 200, May 2019, doi: 10.1016/j.infrared.2019.03.014. [23] P. Kovács, B. Lehner, G. Thummerer, G. Mayr, P. Burgholzer, and M. Huemer, “Deep learning approaches for thermographic imaging,” J. Appl. Phys., vol. 128, no. 15, Oct. 2020, doi: 10.1063/5.0020404. [24] Q. Fang and X. Maldague, “Defect depth estimation in infrared thermography with deep learning,” in 3rd International Symposium on Structural Health Monitoring and Non destructive Testing, 2020, pp. 1 12.
Menaka Murugesan is a postgraduate in Physics and has over 19 years of experience in the field of NDE for materials characterization. She has specialized in the areas of thermal imaging, image processing and digital radiography. She is presently heading Radiation Application and Metrology section at Radiological Safety Division of IGCAR, Kalpakkam. Her field of interests are material characterization using thermal NDE and thermal imaging as diagnostic tool in healthcare. She is an American Society for NDT certified Level III in Infrared Thermal Testing. She can be contacted at email: menaka@igcar.gov.in
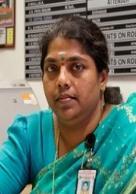




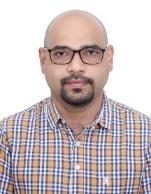




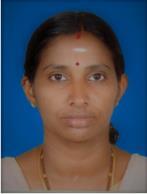




Tanvi Khandelwal graduated in Electronics and Communication from Ramaiah Institute of Technology, Bangalore in 2019. She is working as ATC Application Design Engineer in Alstom, Bangalore. Her interests are in image processing and AI. She can be contacted at email: tanvi.khandelwal717@gmail.com
Varun Ittigi graduated in Electronics and Communication from Ramaiah Institute of Technology, Bangalore in 2019. He has worked in Innocirc Ventures as an Artificial Intelligence Research Engineer. His interests are in AI and Robotics. He can be contacted at email: varunittigi10@gmail.com
Sathvik Udupa is working as a Research Associate in Indian Institute of Science (IISc), Bangalore. He obtained his B.E from Ramaiah Institute of Technology, 2019. He is working in the areas of speech recognition, speech synthesis and multimodal machine learning. He can be contacted at email: sathvikudupa66@gmail.com
ISSN: 2252 8938 Int J Artif Intell, Vol. 11, No. 3, September 2022: 949 960 960 Venkatraman Balasubramaniam is Distinguished Scientist and Director of IGCAR, Kalpakkam and CMD of BHAVINI, Kalpakkam. He post graduated in Physics from St. Joseph College (Autonomous), Tiruchirappalli and obtained his Ph. D from Madras University. With a research career spanning 37 years, he has combined the physics of Non Destructive Evaluation (NDE) with engineering and technology and consistently provided excellent R & D support and robust NDE based solutions to technologically challenging problems in nuclear and other strategic and core industries. His significant milestone activities for the nuclear industry include Procedures for X ray and neutron radiography of highly irradiated fuel pins, comprehensive NDE for evaluation of tube to tube sheet welds of PFBR steam generator and radiometric testing of shielding structures. He has been primarily responsible for establishing the conventional and digital X ray, neutron radiography and thermal imaging facilities at IGCAR. He is recipient of various prestigious awards and fellowships. He can be contacted at email: bvenkat@igcar.gov.in
Touqueer Mulla graduated in Electronics and Communication from Ramaiah Institute of Technology, Bangalore in 2019. He is working as Program Analyst for Cognizant, India. His interests are in AI and embedded systems. He can be contacted at email: touqeer.004@gmail.com
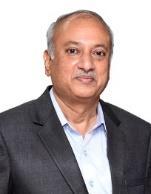




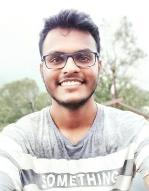




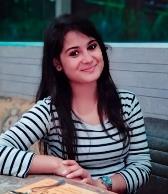




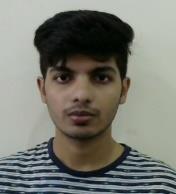




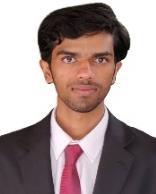




Adversarial machine learning Convolutional neural networks Deep Denoisinglearning
Sandhya Aneja1 , Nagender Aneja2 , Pg Emeroylariffion Abas1 , Abdul Ghani Naim2 1Faculty of Integrated Technologies, Universiti Brunei Darussalam, Bandar Seri Begawan, Brunei Darussalam 2School of Digital Science, Universiti Brunei Darussalam, Bandar Seri Begawan, Brunei Darussalam
This is an open access article under the CC BY SA license.
Journal homepage: http://ijai.iaescore.com
Article history: Received Aug 22, 2021 Revised May 20, 2022
Article Info ABSTRACT
Keywords: Adversarial attacks
Corresponding Author: Nagender Aneja School of Digital Science, Universiti Brunei Darussalam Bandar Seri Begawan, Brunei Darussalam
Email: nagender.aneja@ubd.edu.bn
IAES International Journal of Artificial Intelligence (IJ AI) Vol. 11, No. 3, September 2022, pp. 961 968 ISSN: 2252 8938, DOI: 10.11591/ijai.v11.i3.pp961 968 961
Accepted Jun 6, 2022 Despite substantial advances in network architecture performance, the susceptibility of adversarial attacks makes deep learning challenging to implement in safety critical applications. This paper proposes a data centric approach to addressing this problem. A nonlocal denoising method with different luminance values has been used to generate adversarial examples from the Modified National Institute of Standards and Technology database (MNIST) and Canadian Institute for Advanced Research (CIFAR 10) data sets. Under perturbation, the method provided absolute accuracy improvements of up to 9.3% in the MNIST data set and 13% in the CIFAR 10 data set. Training using transformed images with higher luminance values increases the robustness of the classifier. We have shown that transfer learning is disadvantageous for adversarial machine learning. The results indicate that simple adversarial examples can improve resilience and make deep learning easier to apply in various applications.
1. INTRODUCTION Machine learning helps address the challenges of different industries, including transportation [1], [2], cybersecurity [3] [7], retail [8], smart home [9], social networks [10], health sciences [11], [12], fake news detection [13], and financial services sectors [14]. In particular, deep learning is advantageous in classification problems involving image recognition [15], object recognition, speech recognition, and language translation [16] to give better classification performance. However, recent research has indicated that deep learning algorithms are prone to attacks and can be manipulated to influence algorithmic output [17] [20]. Evtimov et al. [21] demonstrated an adversarial attack to manipulate an autonomous vehicle or a self driving car to misclassify stop signs such as the speed limit, a significant concern in the rapidly developing autonomous transportation domain. In cybersecurity, Kuchipudi et al. [22] and Yang et al [23] have shown that deep learning based spam filters and artificial intelligence based malware detection tools, respectively, can be bypassed by deploying adversarial instruction learning approaches.
Similarly, despite advanced progress in face recognition and speech recognition that led to their deployment in real world applications, such as retail, social networks, and intelligent homes, Vakhshiteh et al. [24] and Schonherr et al [25] have demonstrated their vulnerabilities against numerous attacks to illustrate potential research directions in different areas. Even in the area of finance and health, which traditionally requires a high level of robustness in the system, adversarial attacks are capable of manipulating the system, for
Defense against adversarial attacks on deep convolutional neural networks through nonlocal denoising
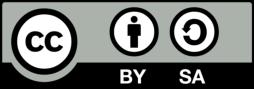
Algorithmically crafted perturbations, no matter how small, can be used as adversarial instructions to manipulate the classification results of deep neural network (DNN) based image classifiers, with some of these adversaries capable of finding the relevant perturbations without access to the network architecture. Goodfellow et al [18] demonstrate a picture of a panda classified as a gibbon, with the addition of perturbations in the picture, despite human eyes still perceiving the picture as that of a panda. Similarly, Evtimov et al [21] have demonstrated that subtle perturbations, unnoticed by the naked eye, can alter the result of the DNN based image classifier from that of a stop sign to a speed limit. It can be argued that these attacks might be challenging to execute on a real time system, such as an autonomous vehicle since they require the real time images obtained from the vehicle sensors to be intercepted and then fed to the perturbations before being passed to the classifier. However, the presence of large types of attacks proposed by several researchers requires the development of robust attack agnostic defense mechanisms against these attacks [32] [34]
Figure 1. Fast Gradient Sign Attack on MNIST
Similarly, Amini et al [38] presented evidential models that capture the increased uncertainty in samples that have been adversarially perturbed. Subsequently, the evidential deep learning method learned a grounded representation of the uncertainty of the data without the need for sampling. Specifically addressing gradient based adversarial attacks, Carbone et al [39] proved that Bayesian neural networks (BNNs) trained with Hamiltonian Monte Carlo (HMC) and variational inference could provide a robust and accurate solution to such attacks in a suitably defined large data limit.
Figure 1 illustrates the fast gradient sign attack [18] in the Modified National Institute of Standards and Technology database (MNIST) data set, with the eps value reflecting the proportion of perturbation of the fast gradient sign method added to the image. Without perturbation, that is, eps: 0, all numbers are correctly classified, whereby the notation 3→3 indicates that the number 3 has been correctly classified as the number 3. However, as the perturbation is added, some numbers are incorrectly classified. In Figure 1, the numbers 2, 6, 2, 9, and 2 have been incorrectly classified as 8, 8, 8, 4, and 7. Goodfellow et al [18] have shown that the accuracy of classification models decreased as the perturbation value increased. Hendrycks et al. [35] curated two data sets, ImageNet A and ImageNet O, to function as natural adversarial example test sets. It has been demonstrated that the performances of different machine learning models deteriorate significantly from adversarial attacks, with the DenseNet 121 model showing 2% and near random chance level accuracies on the adversarial data sets ImageNet A and ImageNet O, respectively. Thus, a robust mechanism is required to protect against these attacks.
Generally, two approaches can be adopted to improve the robustness of a classification model against adversarial attacks: the data centric approach and the model centric approach [36]. In a data centric approach, data are the primary asset to increase the robustness of a classification model while keeping the algorithm, architecture, and hyperparameters constant. The data centric approach requires the creation of an additional data set to improve the performance of a given fixed model. On the other hand, the model and its parameters vary in a model centric approach, with the training data constant. Yan et al. [37] illustrated a model centric approach by integrating an adversarial perturbation based regularizer into the classification objective. The authors have demonstrated that the addition of the regularizer can significantly outperform other methods in terms of robustness and accuracy. Using multi layer perceptron (MLP) on the MNIST data set, the resultant model provides more than two times better robustness to DeepFool adversarial attack, and an improvement in accuracy of 46.69% during fast gradient signed (FGS) attack, over traditional MLP; illustrating the capability of DNN model with the regularizer in learning to resist potential attacks, directly and precisely.
ISSN: 2252 8938 Int J Artif Intell, Vol. 11, No. 3, September 2022: 961 968 962example, by deceiving fraud detection engines to register fraudulent transactions [26], manipulating the health status of individuals [27], and fooling text classifiers [28] [31].
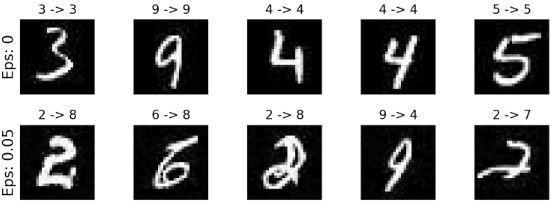
Xie et al [40] presented the network architecture, which uses denoise image features using non local means to improve robustness. The authors have demonstrated a 55.7% accuracy on the ImageNet data set under white box attacks, a substantial improvement in accuracy over the 27.9% accuracy using the traditional method. The authors proposed a network architecture with blocks to denoise the features using non local means. The denoise operation of the authors is processed by 1×1 convolutional layer and then added to the input of the block by a residual connection. Xie et al. [41] proposed using adversarial examples in the training data set with different auxiliary batch norms to improve ImageNet trained models. The auxiliary batch norm has marked differences compared to a normal example. The EfficientNet B7 architecture has been used in ImageNet, with an improvement of 0.7% accuracy shown to increase robustness under different attacks. Moosavi Dezfooli et al [42] proposed dividing the input image into multiple overlapping patches, further denoising each patch independently and reconstructing the denoised image by averaging the pixels in overlapping patches. The authors set the overlap to 75% of the patch size. Liao et al. [43] proposed a high level representation guided denoiser (HGD) defense for image classification. The authors used the loss function as the difference between the top level outputs of the target model induced by the original and adversarial examples instead of denoising pixels. The authors used a UNet like autoencoder for denoising. Chow et al. [44] proposed to combine the unsupervised model denoising ensemble with the supervised model verification ensemble. The authors used denoising ensembles to remove different types of noise and create the number of denoised images. Noise is removed with the help of an auto encoder. The verification ensemble then votes on all denoised images. Thang and Matsui [45] used image transformation and filter techniques to identify adversarial examples sensitive to geometry and frequency and to remove adversarial noise. Most of the prior publications have considered denoising a promising approach for adversarial robustness. However, previous published studies have considered a model centric approach in which the emphasis is on developing different architectures or tuning various hyperparameters. However, this paper studies the performance of a classification model against attack by adding adversarial examples in the training data set and keeping the architecture and hyperparameters constant, hence falling under the data centric approach.
Defense against adversarial attacks on deep convolutional neural networks … (Sandhya Aneja) 963
Int J Artif Intell ISSN: 2252 8938
2. METHOD This paper considers a data centric solution to the MNIST [46] and Canadian Institute for Advanced Research (CIFAR 10) [47] data sets. The MNIST data set consists of 60,000 training images and 10,000 test images, with images comprised of handwritten grayscale digits between 0 and 9 and of size 28×28 pixels. The images in the data set are centered and normalized so that the pixel value is in the range of [0, 1]. On the other hand, the CIFAR 10 data set consists of 60,000 colored images of 10 classes of items: airplane, automobile, bird, cat, deer, dog, frog, horse, ship, and truck. There are 6,000 images per class, and of the 60,000 colored images, 50,000 are training images and 10,000 are test images. Each image has a size of 32×32 pixels.Inthis study, three additional data sets using the nonlocal denoising method were created from the MNIST and CIFAR 10 data sets. The algorithm selects a pixel, takes a small window around the selected pixel, and then performs a scan for similar windows around the original image to average all windows. The average value, which represents estimates of the original image after noise suppression, is then used to replace the pixel value. Given that a grayscale image from the MNIST data set is represented by Ig and a colored image from the CIFAR 10 data set is represented by Ic, where c ∈{R,G,B} represents the red, green, and blue channels, respectively. The gray value of image Ig and the color value of image Ic in pixel p may be represented by ����(��),�� ∈���� and ����(��),�� ∈���� respectively. For simplicity, c can be used to describe either the three color channels of a colored image, that is, c ∈{R,G,B}, or the grayscale image, i.e. c = g, so that Ic can be interchangeably used to represent both grayscale and colored images. The respective estimates �� ̂��(��) of the original image Ic at pixel p using the nonlocal denoising method may be represented by ����(��) = 1 ��(��)∑ ����(��)��∈Ω(��) ��(��,��), where Ω(p) is a square patch of size 21×21 centered at p, representing the search zone for the nonlocal denoising filter around the vicinity of the pixel p. C(p) represents the normalizing factor of pixel p and W (p, q) denotes the weighting factor of pixel q on pixel p. Both parameters are related by ��(��) = ∑ ��(��,��)��∈Ω(��) The weighting factor W(p, q) of the pixel q on p is calculated as ��(��,��) = �� ������(��2(��(��)��(��)) 2��2 0) ℎ2 , where h represents the luminance or color component, and σ denotes the standard deviation of the noise. The large value of h removes more noise from the image; however, it may also decrease its quality. ��2(��(��),��(��)) is the squared Euclidean distance between the two square patches of size 7×7 centered at p and q, i.e. ω(p) and ω(p) which can be calculated from ��2(��(��),��(��)) =
ISSN: 2252 8938 Int J Artif Intell, Vol. 11, No. 3, September 2022: 961 968 964 1 ��(��)×72 ∑ ∑ [����(��+��) ����(��+��)]2 ����∈Ω(0) , where n(c) represents the number of elements in c, i.e. n(c) = 1 for the grayscale image, and n(c)=3 for the colored image. In this paper, three values of h were considered, h∈{3,5,15}; representing the values of small, medium, and large luminance/color components, respectively, to produce three additional data sets from the MNIST and CIFAR 10 data sets. These additional data sets formed adversarial examples and were used to train the classifiers, in addition to the original training data set. ResNet18 and ResNet50 have been used for the MNIST and CIFAR 10 data sets, respectively. Models pretrained and non pretrained on ImageNet have been utilized for comparative purposes.ResNet18 is a residual network with 18 layers, consisting of 17 convolutional layers and one fully connected layer. The residual network is comparatively different from the traditional neural network in which each layer feeds to the next layer. In a residual network, skip connections with double or triple layer skips are used, containing nonlinear (ReLU) activations and batch normalization in between. Thus, the residual network feeds to the next layer and layers 2 3 hops away. The concept of a skip connection is based on brain structure; for example, neurons in cortical layer VI receive input from layer I and thus skip intermediary layers [48]. Skipping connections solve the problem of vanishing gradients and accuracy saturation. ResNet50, also a residual network, but with 50 layers deep, has been used for the CIFAR 10 data set. The more complex and deeper network used for the classification task of the CIFAR 10 data set reflects the more complex data present in the CIFAR 10 data set. The stochastic gradient descent function has been used as an optimizer with a learning rate of 0.01, a momentum of 0.9, and a weight decay of 5e−4 CosineAnnealingLR scheduler is also added to update the learning rate based on the difference between the target and actual examples. The training was carried out for 30 epochs with a batch size of 256. The perturbation values varied from [0, 0.05, 0.1, 0.15, 0.2, 0.25, 0.3].
This section presents experimental results and analysis on the effect of using additional data sets generated using the nonlocal denoising method from the MNIST and CIFAR 10 data sets on the robustness of the classifiers. Different luminance values, i e , h={3, 5, 15}, have been used to generate the data sets to form adversarial examples for additional training data sets. In particular, the performances of the classifiers trained using the data sets generated with the nonlocal denoising method are compared with the classifier trained with the original training data set. Experimental results of all approaches have been shown for the model pretrained using transfer learning and the non pretrained model. Figures 2 and 3 present the performance of the ResNet18 classifier in the MNIST data set for different perturbation values. The ResNet18 classifier in Figure 2 has used ImageNet transfer learning for its initial weights. In contrast, the ResNet18 classifier in Figure 3 has been trained without transfer learning, but using only the architecture with initial weights from a gaussian distribution. In Figure 2, it can be seen that the accuracy decreases with an increase in the perturbation. A drop in accuracy of about 60.6% with a perturbation value of 0.3 is shown for the classifier trained on the original training data set, while the classifier trained with images transformed using the nonlocal denoising method with luminance component 15 shows a 51.3% drop in accuracy; a gain of around 9.3% despite keeping the algorithm, and hyperparameters unchanged. A similar reduction in classification accuracy with increased perturbation can be seen in Figure 3 with the non pretrained ResNet18 classifier. A drop in accuracy of about 48.9% with a perturbation value of 0.3 is shown for the classifier trained on the original training data set. This contrasts with a drop of 44.7% with similar perturbation values for the classifier trained with adversarial examples with luminance component 3, which represents an improvement of 4.2% over the classifier trained with the original training data Comparisonset.between the pretrained and non pretrained ResNet18 classifiers using transfer learning indicates that using transfer learning puts the classifier into a worse position for adversarial attacks with a more significant drop in accuracy in the advent of attacks. It is also noted that the images transformed using the nonlocal method with the luminance component 3 perform marginally better (3.2% difference) than with luminance component 15 for the non pretrained ResNet18 classifier in Figure 3. In contrast, for the pretrained ResNet18 classifier in Figure 3, the higher luminance component performs better (7.6% difference) than the lower luminance component. Figures 4 and 5 present the performance of the ResNet50 classifier in the CIFAR 10 data set for different perturbation values. The ResNet50 classifier has used transfer learning with initial weights from ImageNet in Figure 4, while the ResNet50 classifier in Figure 5 has used initial weights from a Gaussian distribution only. A general drop in accuracy can be observed with increasing perturbation value in both Figures 4 and 5. For the ResNet50 classifier using transfer learning in Figure 4, it can be seen that the
3. RESULTS AND DISCUSSION
Int J Artif Intell ISSN: 2252 8938
Comparing Figures 4 and 5 shows that using transfer learning in the ResNet50 classifier provides higher accuracy in the absence of perturbation. However, the perturbation affects the pretrained model more than the non pretrained model, resulting in a more significant drop of accuracy as more perturbation is introduced. This indicates that the pretrained model which utilizes transfer learning, worsens the robustness of the classifier. Overall, these results indicate that introducing adversarial examples in the training data set, by utilising transformed images using the nonlocal denoising method with high luminance value, without pretraining the network via transfer learning, is advantageous for adversarial machine learning.
Convolutional networks are prone to adversarial attacks, which present a challenge to safety critical domains where calibrated, robust, and efficient measures of data uncertainty are crucial. Several different deep learning techniques are summarized in this paper to improve the robustness of a classification model even in the presence of adverse perturbations. Two general approaches may be adopted for this purpose: data centric and model centric approaches. In this paper, a data centric approach has been demonstrated, in which the MNIST and CIFAR 10 data sets have been craftily perturbed using the nonlocal denoising method
Figure 4. CIFAR 10 (pretrained) with ResNet50 Figure 5. CIFAR 10 (not pretrained) with ResNet50
Again, this illustrates the advantage of using transformed images using the nonlocal denoising method as adversarial examples in the training data set, albeit with a lower improvement of only 4%. Thus, the classifier trained with transformed images using the nonlocal denoising method is generally better than the classifier trained only with the original training data set. This is true for both ResNet50 classifiers utilizing transfer learning, i.e., pretrained and non pretrained models, in Figures 4 and 5, respectively.
4. CONCLUSION
Figure 2. MNIST (pretrained) with ResNet18 Figure 3. MNIST (not pretrained) with ResNet18
Defense against adversarial attacks on deep convolutional neural networks … (Sandhya Aneja) 965 accuracy drops by approximately 73% and 60% with a perturbation value of 0.3 for the classifier trained with the original training data set only and with images transformed using the nonlocal denoising method with luminance component 15, respectively. This illustrates that training using the transformed images improves accuracy performance by 13% while keeping the algorithm and hyperparameters unchanged. Figure 5 shows accuracy drops of around 66% and 56% with a perturbation value of 0.3 for classifiers trained with the original training data set only and with transformed images with luminance component 15, respectively.
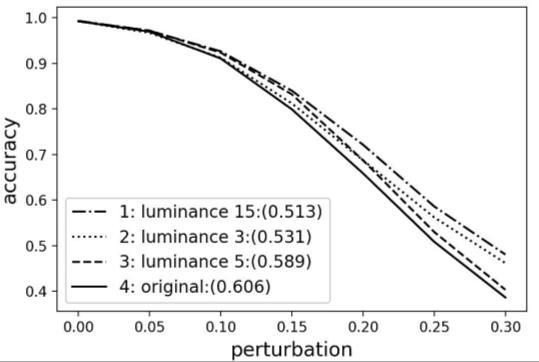
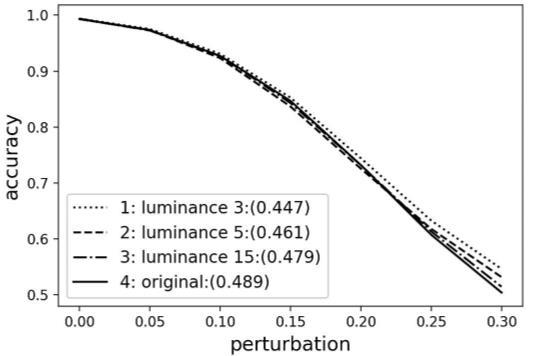
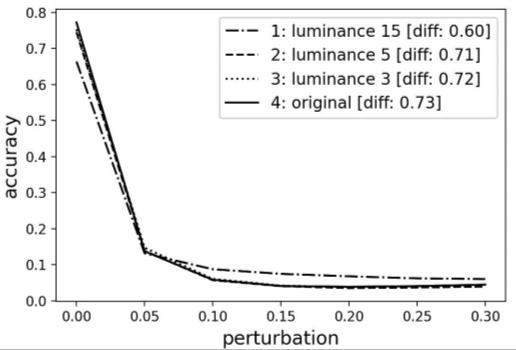
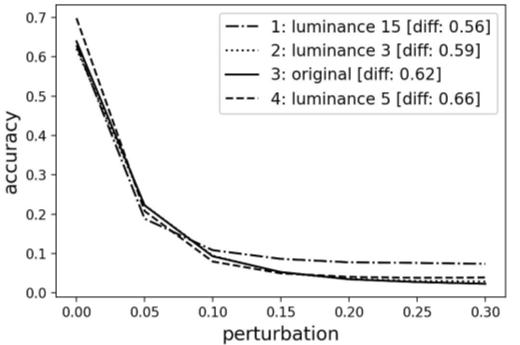
[1] P. N. Huu and C. V. Quoc, “Proposing WPOD NET combining SVM system for detecting car number plate,” IAES International Journal of Artificial Intelligence (IJ AI), vol. 10, no. 3, p. 657, Sep. 2021, doi: 10.11591/ijai.v10.i3.pp657 665. [2] F. M.S., M. E. D. M.M., and E. S. H.A., “Deep learning versus traditional methods for parking lots occupancy classification,” Indonesian Journal of Electrical Engineering and Computer Science, vol. 19, no. 2, pp. 964 973, 2020, doi: 10.11591/ijeecs.v19i2.pp964 973. [3] I. Idrissi, M. Boukabous, M. Azizi, O. Moussaoui, and H. El Fadili, “Toward a deep learning based intrusion detection system for IoT against botnet attacks,” IAES International Journal of Artificial Intelligence (IJ AI), vol. 10, no. 1, p. 110, Mar. 2021, doi: 10.11591/ijai.v10.i1.pp110 120. [4] S. Aneja, M. A. X. En, and N. Aneja, “Collaborative adversary nodes learning on the logs of IoT devices in an IoT network,” in 2022 14th International Conference on COMmunication Systems & NETworkS (COMSNETS), Jan. 2022, pp. 231 235, doi: 10.1109/COMSNETS53615.2022.9668602.
[18] I. J. Goodfellow, J. Shlens, and C. Szegedy, “Explaining and harnessing adversarial examples,” Dec. 2015, [Online]. Available: http://arxiv.org/abs/1412.6572.
[15] N. Aneja and S. Aneja, “Transfer learning using CNN for handwritten devanagari character recognition,” in 2019 1st International Conference on Advances in Information Technology (ICAIT), Jul. 2019, pp. 293 296, doi: 10.1109/ICAIT47043.2019.8987286.
[9] S. Pandey, S. H. Saeed, and N. R. Kidwai, “Simulation and optimization of genetic algorithm artificial neural network based air quality estimator,” Indonesian Journal of Electrical Engineering and Computer Science, vol. 19, no. 2, pp. 775 783, Aug. 2020, doi: 10.11591/ijeecs.v19.i2.pp775 783.
[13] N. Aneja and S. Aneja, “Detecting fake news with machine learning,” in International Conference on Deep Learning, Artificial Intelligence and Robotics, 2019, pp. 53 64. [14] M. Reza Pahlawan, E. Riksakomara, R. Tyasnurita, A. Muklason, F. Mahananto, and R. A. Vinarti, “Stock price forecast of macro economic factor using recurrent neural network,” IAES International Journal of Artificial Intelligence (IJ AI), vol. 10, no. 1, p. 74, Mar. 2021, doi: 10.11591/ijai.v10.i1.pp74 83.
ACKNOWLEDGEMENT
[6] S. Aneja, N. Aneja, B. Bhargava, and R. R. Chowdhury, “Device fingerprinting using deep convolutional neural networks,” International Journal of Communication Networks and Distributed Systems, vol. 28, no. 2, pp. 171 198, 2022, doi: 10.1504/IJCNDS.2022.121197.
[16] S. Aneja, S. Nur Afikah Bte Abdul Mazid, and N. Aneja, “Neural Machine Translation model for University Email Application,” in 2020 2nd Symposium on Signal Processing Systems, Jul. 2020, pp. 74 79, doi: 10.1145/3421515.3421522. [17] C. Szegedy et al., “Intriguing properties of neural networks,” arXiv preprint arXiv:1312.6199, Dec. 2013, [Online]. Available: http://arxiv.org/abs/1312.6199.
ISSN: 2252 8938 Int J Artif Intell, Vol. 11, No. 3, September 2022: 961 968 966with different luminance values. Experimental results have indicated that the introduction of transformed images as adversarial examples in the training data set is capable of increasing the robustness of the classification model. The method has been shown to provide absolute accuracy improvements of up to 9.3% and 13% on the MNIST and CIFAR 10 data sets, respectively, over classifier trained on the original data sets only, under perturbations. Introducing transformed images with high luminance values gives a more robust classifier. Furthermore, it has been demonstrated that the use of transfer learning is disadvantageous for adversarial machine learning. Future work may include generative adversarial examples for ImageNet and generating denoised adversarial examples using generative adversarial networks. Future work may also involve exploring the effectiveness of Bayesian inference for adversarial defense.
[7] R. R. Chowdhury, S. Aneja, N. Aneja, and P. E. Abas, “Packet level and IEEE 802.11 MAC frame level network traffic traces data of the D Link IoT devicesNo Title,” Data in Brief, vol. 37, p. 107208, 2021, doi: 10.1016/j.dib.2021.107208.
[5] S. Aneja, N. Aneja, and M. S. Islam, “IoT device fingerprint using deep learning,” in 2018 IEEE International Conference on Internet of Things and Intelligence System (IOTAIS), Nov. 2018, pp. 174 179, doi: 10.1109/IOTAIS.2018.8600824.
[19] A. Demontis et al., “Why do adversarial attacks transfer? explaining transferability of evasion and poisoning attacks,” in 28th USENIX Security Symposium (USENIX Security 19), 2019, pp. 321 338. [20] S. Mei and X. Zhu, “Using machine teaching to identify optimal training set attacks on machine learners,” in Twenty Ninth AAAI Conference on Artificial Intelligence, 2015, pp. 2871 2877. [21] I. Evtimov et al., “Robust physical world attacks on machine learning models,” CoRR, vol. abs/1707.0, 2017, [Online]. Available: http://arxiv.org/abs/1707.08945.
[11] S. Aneja, N. Aneja, P. E. Abas, and A. G. Naim, “Transfer learning for cancer diagnosis in histopathological images,” IAES International Journal of Artificial Intelligence (IJ AI), vol. 11, no. 1, pp. 129 136, Mar. 2022, doi: 10.11591/ijai.v11.i1.pp129 136. [12] A. K. Jaiswal, I. Panshin, D. Shulkin, N. Aneja, and S. Abramov, “Semi supervised learning for cancer detection of lymph node metastases,” 2019, [Online]. Available: http://arxiv.org/abs/1906.09587.
[8] N. Sakinah Shaeeali, A. Mohamed, and S. Mutalib, “Customer reviews analytics on food delivery services in social media: a review,” IAES International Journal of Artificial Intelligence (IJ AI), vol. 9, no. 4, p. 691, Dec. 2020, doi: 10.11591/ijai.v9.i4.pp691 699.
REFERENCES
The authors acknowledge the support given by the SDS Research Grant of Universiti Brunei Darussalam awarded to Dr. Nagender Aneja and Dr. Sandhya Aneja, grant number UBD/RSCH/1.18/FICBF(b)/2021/001
[10] R. A. Bagate and R. Suguna, “Sarcasm detection of tweets without #sarcasm: data science approach,” Indonesian Journal of Electrical Engineering and Computer Science, vol. 23, no. 2, p. 993, Aug. 2021, doi: 10.11591/ijeecs.v23.i2.pp993 1001.
[33] H. Yakura, Y. Akimoto, and J. Sakuma, “Generate (non software) bugs to fool classifiers,” in Proceedings of the AAAI Conference on Artificial Intelligence, Apr. 2020, vol. 34, no. 01, pp. 1070 1078, doi: 10.1609/aaai.v34i01.5457.
[37] Z. Yan, Y. Guo, and C. Zhang, “Deep defense: training DNNs with improved adversarial robustness,” in Advances in Neural Information Processing Systems, 2018, pp. 419 428. [38] A. Amini, W. Schwarting, A. Soleimany, and D. Rus, “Deep evidential regression,” in Advances in Neural Information Processing Systems, 2020, pp. 1 20. [39] G. Carbone, M. Wicker, L. Laurenti, A. Patane, L. Bortolussi, and G. Sanguinetti, “Robustness of bayesian neural networks to gradient based attacks,” in Advances in Neural Information Processing Systems, 2020, pp. 15602 15613.
[46] Y. LeCun, C. Cortes, and C. J. C. Burges, “The MNIST database of handwritten digits,” yann.lecun.com, 1998.
[48] A. M. Thomson, “Neocortical layer 6, a review,” Frontiers in Neuroanatomy, vol. 4, p. 13, 2010, doi: 10.3389/fnana.2010.00013.
[27] S. G. Finlayson, J. D. Bowers, J. Ito, J. L. Zittrain, A. L. Beam, and I. S. Kohane, “Adversarial attacks on medical machine learning,” Science, vol. 363, no. 6433, pp. 1287 1289, Mar. 2019, doi: 10.1126/science.aaw4399.
[28] B. Liang, H. Li, M. Su, P. Bian, X. Li, and W. Shi, “Deep text classification can be fooled,” in Proceedings of the Twenty Seventh International Joint Conference on Artificial Intelligence, Jul. 2018, pp. 4208 4215, doi: 10.24963/ijcai.2018/585.
[22] B. Kuchipudi, R. T. Nannapaneni, and Q. Liao, “Adversarial machine learning for spam filters,” in Proceedings of the 15th International Conference on Availability, Reliability and Security, Aug. 2020, pp. 1 6, doi: 10.1145/3407023.3407079.
Defense against adversarial attacks on deep convolutional neural networks … (Sandhya Aneja) 967
Int J Artif Intell ISSN: 2252 8938
[31] J. Ebrahimi, A. Rao, D. Lowd, and D. Dou, “HotFlip: white box adversarial examples for text classification,” in Proceedings of the 56th Annual Meeting of the Association for Computational Linguistics (Volume 2: Short Papers), 2018, pp. 31 36, doi: 10.18653/v1/P18 2006.
[23] C. Yang et al., “DeepMal: maliciousness Preserving adversarial instruction learning against static malware detection,” Cybersecurity, vol. 4, no. 1, p. 16, Dec. 2021, doi: 10.1186/s42400 021 00079 5.
[36] J. D. Bosser, E. Sorstadius, and M. H. Chehreghani, “Model centric and data centric aspects of active learning for deep neural networks,” in 2021 IEEE International Conference on Big Data (Big Data), Dec. 2021, pp. 5053 5062, doi: 10.1109/BigData52589.2021.9671795.
[41] C. Xie, M. Tan, B. Gong, J. Wang, A. L. Yuille, and Q. V Le, “Adversarial examples improve image recognition,” in 2020 IEEE/CVF Conference on Computer Vision and Pattern Recognition (CVPR), Jun. 2020, pp. 816 825, doi: 10.1109/CVPR42600.2020.00090.
[44] K. H. Chow, W. Wei, Y. Wu, and L. Liu, “Denoising and verification cross layer ensemble against black box adversarial attacks,” in 2019 IEEE International Conference on Big Data (Big Data), Dec. 2019, pp. 1282 1291, doi: 10.1109/BigData47090.2019.9006090.
[26] I. Fursov et al., “Adversarial attacks on deep models for financial transaction records,” in Proceedings of the 27th ACM SIGKDD Conference on Knowledge Discovery & Data Mining, Aug. 2021, pp. 2868 2878, doi: 10.1145/3447548.3467145.
[25] L. Schonherr, K. Kohls, S. Zeiler, T. Holz, and D. Kolossa, “Adversarial attacks against automatic speech recognition systems via psychoacoustic hiding,” 2019, doi: 10.14722/ndss.2019.23288.
[43] F. Liao, M. Liang, Y. Dong, T. Pang, X. Hu, and J. Zhu, “Defense against adversarial attacks using high level representation guided denoiser,” in 2018 IEEE/CVF Conference on Computer Vision and Pattern Recognition, Jun. 2018, pp. 1778 1787, doi: 10.1109/CVPR.2018.00191.
[45] D. D. Thang and T. Matsui, “Adversarial examples identification in an end to end system with image transformation and filters,” IEEE Access, vol. 8, pp. 44426 44442, 2020, doi: 10.1109/ACCESS.2020.2978056.
[47] A. Krizhevsky, G. Hinton, and others, “Learning multiple layers of features from tiny images,” Citeseer, 2009.
BIOGRAPHIES OF AUTHORS Dr Sandhya Aneja is working as Assistant Professor of Information and Communication System Engineering at the Faculty of Integrated Technologies, Universiti Brunei Darussalam. Her primary areas of research interest include wireless networks, high performance computing, internet of things, artificial intelligence technologies machine learning, machine translation, deep learning, data science, and data analytics. Further info on her website: https://sandhyaaneja.github.io She can be contacted at email: sandhya.aneja@gmail.com.
[29] V. Kuleshov, S. Thakoor, T. Lau, and S. Ermon, “Adversarial examples for natural language classification problems,” 2018. [30] S. Garg and G. Ramakrishnan, “Bae: Bert based adversarial examples for text classification,” arXiv preprint arXiv:2004.01970, 2020.
[42] S. M. Moosavi Dezfooli, A. Shrivastava, and O. Tuzel, “Divide, denoise, and defend against adversarial attacks,” arXiv preprint arXiv:1802.06806, Feb. 2018, [Online]. Available: http://arxiv.org/abs/1802.06806.
[35] D. Hendrycks, K. Zhao, S. Basart, J. Steinhardt, and D. Song, “Natural adversarial examples,” in 2021 IEEE/CVF Conference on Computer Vision and Pattern Recognition (CVPR), Jun. 2021, pp. 15257 15266, doi: 10.1109/CVPR46437.2021.01501.
[40] C. Xie, Y. Wu, L. van der Maaten, A. L. Yuille, and K. He, “Feature denoising for improving adversarial robustness,” in 2019 IEEE/CVF Conference on Computer Vision and Pattern Recognition (CVPR), Jun. 2019, pp. 501 509, doi: 10.1109/CVPR.2019.00059.
[34] P. Sanjeewani, B. Verma, and J. Affum, “A novel evolving classifier with a false alarm class for speed limit sign recognition,” in 2021 IEEE Congress on Evolutionary Computation (CEC), Jun. 2021, pp. 2211 2217, doi: 10.1109/CEC45853.2021.9504710.
[32] B. Ye, H. Yin, J. Yan, and W. Ge, “Patch Based attack on traffic sign recognition,” in 2021 IEEE International Intelligent Transportation Systems Conference (ITSC), 2021, pp. 164 171.
[24] F. Vakhshiteh, A. Nickabadi, and R. Ramachandra, “Adversarial attacks against face recognition: comprehensive study,” CoRR, vol. abs/2007.1, Jul. 2020, [Online]. Available: https://arxiv.org/abs/2007.11709.
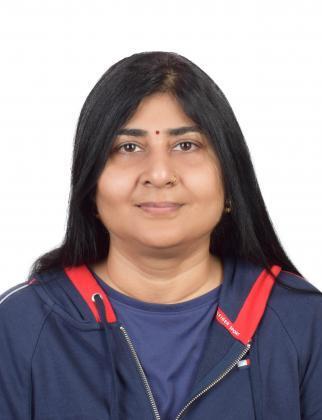



Dr Nagender Aneja is working as Assistant Professor at School of Digital Science, Universiti Brunei Darussalam. He did his Ph.D. in Computer Engineering from J.C. Bose University of Science and Technology YMCA, and M.E. Computer Technology and Applications from Delhi College of Engineering. He is currently working in deep learning, computer vision, and natural language processing. He is also the founder of ResearchID.co Further info on his website: http://naneja.github.io. He can be contact at email: naneja@gmail.com.
Dr Abdul Ghani Naim is a Senior Assistant Professor at School of Digital Science, Universiti Brunei Darussalam. He did his Ph.D. in Information Security from the Royal Holloway College, London. His present research interests include computer security, cryptography, high performance computing, and machine learning. Further info on his homepage: https://expert.ubd.edu.bn/ghani.naim He can be contacted at email: ghani.naim@ubd.edu.bn
ISSN: 2252 8938 Int J Artif Intell, Vol. 11, No. 3, September 2022: 961 968 968
Pg Dr Emeroylariffion Abas received his B.Eng. Information Systems Engineering from Imperial College, London in 2001, before obtaining his Ph.D. Communication Systems in 2005 from the same institution. He is now working as an Assistant Professor in General Engineering, Faculty of Integrated Technologies, Universiti Brunei Darussalam. His present research interest are data analytic, energy systems and photonics. Further info on his homepage: https://expert.ubd.edu.bn/emeroylariffion.abas. He can be contacted at email: emeroylariffion.abas@ubd.edu.bn
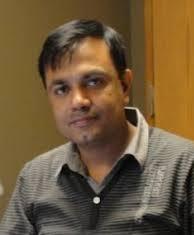



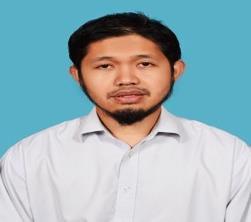



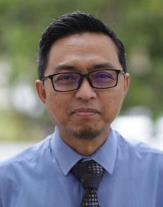



Journal homepage: http://ijai.iaescore.com
Return on investment framework for profitable crop recommendation system by using optimized multilayer perceptron regressor
Surekha Janrao1, Deven Shah2 1Department of Computer Engineering, Terna Engineering College, Navi Mumbai, India 2Department of Computer Engineering, Thakur College of Engineering and Technology, Mumbai, India
ReturnRegressionRecommendationMultilayerlearningperceptronsystemoninvestment
Article history: Received Jun 9, 2021 Revised May 24, 2022 Accepted Jun 3, 2022 Return on investment (ROI) plays very important role as a financial dimension in the agriculture sector. Many government agencies like Indian space research organization (ISRO), Indian council of agricultural research (ICAR), and Nitiayog are working on different agriculture projects to improve profitability and sustainability. This paper presents ROI framework to recommend more profitable crop to the farmers as per the current market price and demand which is missing in the existing crop recommendation system. Crop price prediction (CPP) and crop yield prediction (CYP) system are integrated in the ROI framework to predict more demandable crop to yield. This framework is designed by applying data analysis to provide regression statistics which further helps for model selection and improve the performance also. Optimized multilayer perceptron regressor algorithm has been evaluated through experimental results and it has been observed that it gives better performance as compared to other existing regression techniques.
This is an open access article under the CC BY SA license. Corresponding Author: Surekha AssistantJanraoProfessor in Department of Computer Engineering, Terna Engineering College Nerul, Navi Mumbai, India Email: surekhajanrao@ternaengg.ac.in
Keywords: Machine
IAES International Journal of Artificial Intelligence (IJ AI) Vol. 11, No. 3, September 2022, pp. 969 976 ISSN: 2252 8938, DOI: 10.11591/ijai.v11.i3.pp969 976 969
1. INTRODUCTION Agriculture is very bright sector in Indian economic growth. So, there is a need to do the research work in agriculture domain. As Indian population is increasing day by day so there is more requirement of crop yield. To increase the crop yield and profit for the farmers more accurate and profitable crop should be cultivated. This is achieved by considering financial dimension as a return on investment (ROI) which helps for the farmers to take more accurate and an intelligent decision for the crop selection based on the profit and loss as per the market price and demand [1]. Transition is very much required from traditional thinking to more advanced thinking. This can be achieved by providing accurate information on the tip of the finger to the farmer as a knowledge data discovery using modern technology like machine learning (ML), deep learning (DL), and internet of things (IoT) [2]. In this paper we are presenting the work on development of ROI framework by using more efficient machine leaning techniques which can improve the performance of the crop recommendation system. In this paper an emphasis given on the agricultural problems and prospectus of yeola taluka which is located in Nashik district of Maharashtra state. There is uneven distribution of rainfall in this study area. The socio economic status of this area is primly bound to agriculture. In our research study we find the low productivity of land, scarcity of water, traditional methods of farming, uneven climatic changes, economically backwardness of farmers, fragmentation of farm and enormous low market prices for agricultural
Article Info ABSTRACT
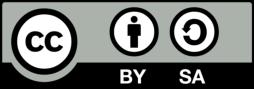
In the reference paper [13] research work has been done for crop recommendation system by using convolutional neural networks (CNN) which is the most widely used deep learning algorithm. But results show that no specific conclusion can be drawn as to what the best model is, but they clearly show that some machine learning models are used more than the others .We are integrating two different systems as CPP and CYP to get ROI framework and output is continuous real value. In the reference [14] researchers done an extensive experimental survey of regression methods by using all the regression datasets of the union cycliste internationale (UCI) machine learning repository. In this survey they have evaluated more than 77 regression models belonging to 19 different families like nearest neighbors, regression trees and rules, RF, bagging and boosting, neural networks, DL, and support vector regression.
ISSN: 2252 8938 Int J Artif Intell, Vol. 11, No. 3, September 2022: 969 976 970products. These are the basic problems of this region which motivates us to do the research work in such type of area which can increase economic growth of this farmers by providing more efficient crop recommendation system [3]. ROI framework is designed by integrating crop yield prediction (CYP) system and crop price prediction (CPP) system. This framework is developed by using data set collected from yeola region with the help of different government agencies. In this research work we have applied data analysis on collected data set to get regression statistics. We have implemented multiple regression model considering soil fertility index (SFI) as a most important feature and climatic factors as other attributes for CYP. And different levels of market price as min, max, avg for the CPP [4]. In this paper we have evaluated performance of different regression algorithms and results shown that improved sequential minimal optimization (ISMO) and multilayer perceptron (MLP) regression model gives better performance as compared to other machine learning algorithms for regression. Later MLP model will get optimized to get better results by applying hypertunning process on the existing one [5]. Much research worked on traditional machine learning algorithms as support vector machine (SVM), naïve Bayes (NB), random forest (RF), and decision tree (DT) for analyzing and predicting the crop based on soil and whether parameters, detailed description of this work is given in the reference [6], [7]. But the main disadvantage is that due to lack of optimization technique these algorithms are not giving better performance, and which is overcome in our current research work by applying hyper parameter tuning process for accurate model selection. Some authors worked on different neural network algorithms, hybrid approach of different machine learning algorithm, boosting, and bagging techniques, adaptive clustering methods, association mining techniques for crop recommendation system. But in this research work financial dimension is missing i.e., ROI which is very important component which helps to improve economic growth of the farmers if accurate information is provided to them as well as other agriculture experts by using intelligent approach of modelling in the crop recommendation framework [8] [10] Some authors worked on ontology based farming and analysis of agriculture data using data mining techniques [11], [12] In our research work we have developed ROI framework by integrating crop yield and CPP system to recommend more suitable crop to the farmers.
In our experimental research work we observed that sequential minimal optimization (SMO) regressor (SMO algorithm for SVM regression) and MLP regression working more efficiently as compared to other regression techniques like bagging regressor, Gaussian regressor, RF regressor, AdaBoost regressor [15]. In this paper, researchers address the SVM regression problem and proposed an iterative algorithm, called SMO, for solving the regression problem using SVM. This algorithm is an extension of the SMO algorithm proposed by platt for SVM classifier design. They have suggested two modifications of the SMO algorithm that overcome the problem by efficiently maintaining and updating two threshold parameters. Their computational experiments show that these modifications speed up the SMO algorithm significantly in most situations.
In the reference [16] researchers worked on MLP regressor for multiple linear regression analysis and artificial neural network (ANN) as tools for performance measurement has been employed in this work. In the reference [17] researchers concludes that with respect to the parametric model, the ANN has shown better results from the statistical analysis that it is a better modelling technique to support decision making for various type of recommendations. Nashik district is a major agriculturally dominant district in the Maharashtra. Therefore, it is important to highlight the less developed agricultural region and try to promote agricultural development. So, our present work is an attempt in the same direction but at taluka level we have selected as yeola regions. In topographical research study of Nashik district at tehsil level titled as “Spatial analysis of agricultural development in Nashik district: A Tahsil level study” [18] This research study helps us to identify research challenges and understands topographical condition of yeola region so that we can move forward in proper direction.
2. RESEARCH METHOD FOR RETURN ON INVESTMENT (ROI) DIMENSION ROI framework has been designed by using CYP system and CPP system. Performance analysis of various machine learning algorithms are evaluated to identify more efficient ML algorithm. This framework will predict accurate and profitable crop based on the profit and loss calculated by considering all type of
3. RESULTS AND DISCUSSION
Proposed framework describes that crop recommendation system has been developed for yeola region in which total 121 villages are there which are merged into total 6 circles. This recommendation incudes ROI dimension for crops cotton and corn. ROI value will be calculated by using CYP system and CPP system. Each of this system undergoes data collection from different government agencies and market committee, data analysis for regression statistics, and model deployment for multiple regression algorithms, performance evaluation analysis and final recommendation based on the provided input. Then crop price and crop yield value is used for ROI system. In this system balance sheet has been generated by considering all type of expense cost from initial cropping to final harvesting. Then profit or loss will be calculated to recommend more profitable crop as price [19]
Figure 1. Proposed framework for ROI system
Return on investment framework for profitable crop recommendation system by …(Surekha Janrao) 971
expense cost from initial cropping to final harvesting. This framework recommends more profitable crop as final output by integrating CYP and CPP model as shown in the following Figure 1.
3.1. Performance evaluation for identifying optimized machine learning algorithm for crop yield prediction (CYP) system To design CYP system last three years (2018, 2019, 2020) circle wise data has been collected for yeola region of Nashik district. In this CYP system data has been collected from various digital sources and government agencies for 121 villages from yeola region. All parameters required in data analysis for
Int J Artif Intell ISSN: 2252 8938
In this section, the validation of the proposed ROI framework against existing regression techniques are illustrated with several parameter metrics in our experimental work. In the first subsection evaluation of multiple regression statistics has been done. Further data analysis for significance testing of predictors are evaluated.
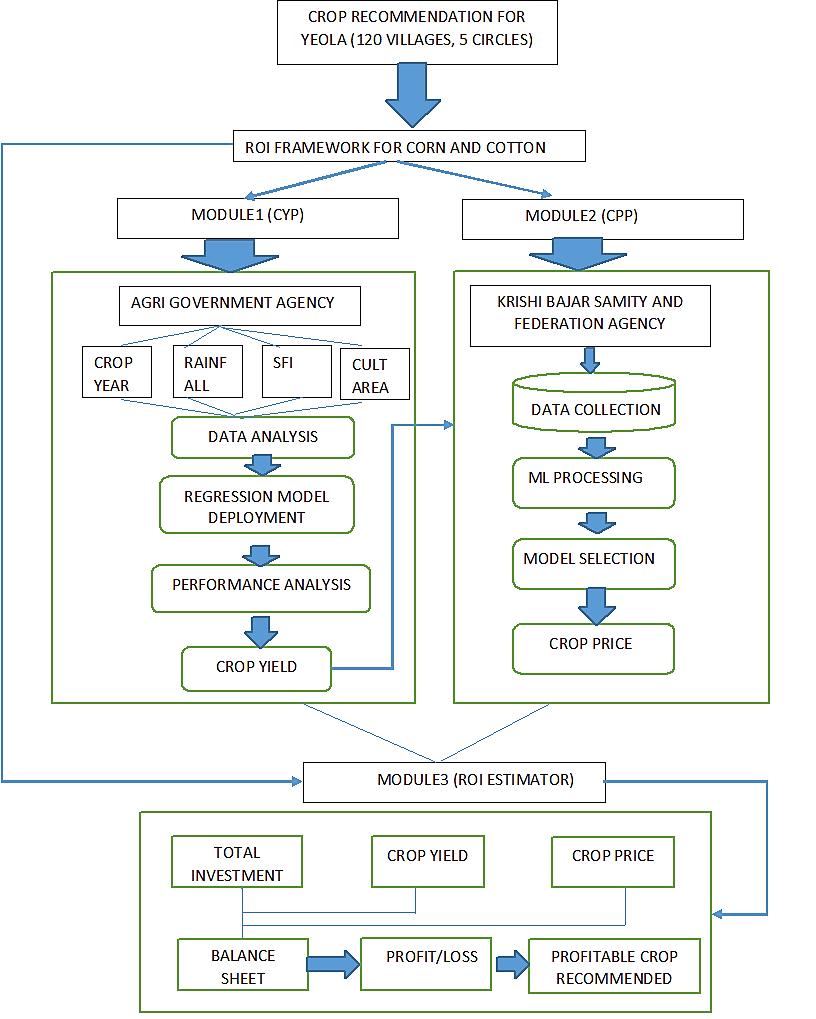
ISSN: 2252 8938 Int J Artif Intell, Vol. 11, No. 3, September 2022: 969 976
Regression parameters Regression statistics Multiple R 0.99991337 R Square 0.999826747 Adjusted R Square 0.9998253 Standard Error 12.64813767 Observations 484
3.2. Model deployment and performance evaluation of CYP system
Table 3. Data analysis for significance testing of predictors Parameters Coefficients Standard error t Stat P value Intercept 2901.332 6.5836 0.4406 0.0065963 Crop _Year 1.438531 3.2633 0.4408 0.65955 Rainfall 0.000696 0.0078 0.0888 0.0429219 SFI 0.768672 1.4166 0.5425 0.0015876 Cultivation _Area 3.924554 0.0023 1662.2 0.0362905
Results for significance testing has been observed that overall multiple regression model was significant for (4,479)=F (1.16603E 19), P<0.05, ��2=0.999826747, where α=0.05 then REJECT Null hypothesis H0 and ACCEPT alternative hypothesis H1 for multiple regression equation; (2018, 301, 2.19, 567)=2225.072 Y (Crop Yield)=2901.33+( 1.438531382*Crop Year)+( 0.000696782*Rainfall)+(0.000696782*SFI)+ (3.924554098*Cultivation Area)
Table 1. Evaluation of regression statistics
Total six machine learning algorithm as sequential minimal optimization regressor (SMO REG), improved SMO regressor (ISMO REG), multilayer perceptron neural network regressor (MLP REG), bagging regressor (BAGG REG), Gaussian regressor (G REG), random forest regressor (RF REG), and AdaBoost regressor (AB REG) has been used for multiple regression to predict accurate yield of the crops [22]. These models are evaluated by using various performance metrics as shown in following Table 4. And from the results it has been concluded that SMO REG, ISMO REG, MLP REG are giving better performance as compared to other algorithms .But to optimize the results and minimize the error hyper tuning process has been applied on MLP regressor by using stochastic gradient method, learning rate and momentum parameters [23]. Hyper tuning process achieved global minimum error as shown in the following Table 5. From optimized results it has been observed that at the value of learning rate Ƞ=0.5 and momentum M=0.2 root mean squared error (RMSE) error has been minimized to 12.32 from 26.79 and which is the great achievement for us as we have reached to global minima. Graphical representation of hyper parameter tuning results has been presented in the following Figures 2 to 4 for data analysis of regression statistics of crop data set [24]
Table 2. ANOVA test for input features Parameters df SS MS F Significance F Regression 4 4.42E+08 110553285.4 691064.3 1.16603E 19 Residual 479 76628.21 159.9753866 Total 483 4.42E+08
972regression statistics to check significance level testing explained in detail in the reference [20]. Data analysis has been done by using multiple regression statistics in which standard error are calculated as shown in the following Table 1. Then analysis of variance (ANOVA) test has been applied for checking significance level [21] of input parameters such as crop year, rainfall, cultivation area, SFI as shown in Table 2.
Results of ANOVA test following null hypothesis (��0) and alternative hypothesis (��1) has been defined in the following testing. Hypothesis Testing: α=0.05 and P value should be less than the threshold then only predictors use to predict output are significant as shown in Table 3 otherwise it rejects the null hypothesis. H0: Crop_Year (β1) =Rainfall (β2) =SFI (β3) =Cultivation_Area (β4) ==0 H1: At least, β1 OR β2 OR β4 OR β3≠0 then ACCEPT H1 and REJECT H0
Figure 4. RMSE curve for momentum=1.3 and 1.4
Table
TUNNING OF HYPERPARAMETERS(Ƞ,M)→ RMSE (0.1,0.1)=26.79 (0.1,0.2)=29.21 (0.1,0.3)=28.32 (0.1,0.1)=27.49 (0.2,0.1)=25.57 (0.2,0.2)=25.10 (0.2,0.3)=24.73 (0.2,0.4)=24.45 (0.3,0.1)=26.66 (0.3,0.2)=26.79 (0.3,0.3)=26.01 (0.3,0.4)=28.52 (0.4,0.1)=29.98 (0.4,0.2)=23.47 (0.4,0.3)=12.52 (0.4,0.4)=12.90 (0.5,0.1)=12.52 (0.5,0.2)=12.32 (0.5,0.3)=12.83 (0.5,0.4)=13.44
Figure 2. Data analysis for LR (Learning Rate) vs. RMSE Figure 3. RMSE curve for momentum=1.1 and 1.2
Table 4. Performance analysis of ML algorithm for CYP
Table 5. Optimized results after hyper parameter tuning
3.3. Results and discussion on performance evaluation for crop price prediction system (CPP)
ALGO COREL COEF MAE RMSE RAE RRSE MLP REG 0.9996 18.76 26.79 2.93% 2.80% ISMO REG 0.9999 5.63 14.025 0.0812% 1.46% SMO REG 0.9999 16.85 24.262 2.6386% 2.538% BAGG REG 0.9956 19.85 94.699 3.107% 9.9% G REG 0.9803 178.9 237.65 28.009% 2.42% RF REG 0.9976 17.99 74.045 2.8158% 7.74% AB REG 0.7386 435.3 644.41 68.135% 67.41%
Regression parameters Regression statistics Multiple R 0.999757 R Square 0.999513 Adjusted R Square 0.999511 Standard Error 32.95615 Observations 730 50010 1 2 3 4 5 6 7 RMSELR Iterations LR vs RMSE LR RMSE 500 0 0.2 0.4 0.6 0.8 RMSE Learning Rate(ƞ) RMSE CURVE RMSE CURVE FOR M=1.1 500 0 0.1 0.2 0.3 0.4 0.5 0.6 0.7 0.8 RMSE Learning Rate(ƞ) RMSE CURVE RMSE CURVE FOR M=1.3 RMSE CURVE FOR M=1.4
Process defined for CPP is same as we have seen in previous section. Here only results have been presented in the following tables. Regression statistic analysis demonstrated in the Table 6. Analysis of variance (ANOVA) test has been applied and results has been displayed in the Table 7. Significance and hypothesis testing has been done and results are evaluated in the Table 8. Model evaluation and selection has been done in Table 9. 6. Evaluation of regression statistics for CPP
Int J Artif Intell ISSN: 2252 8938
Return on investment framework for profitable crop recommendation system by …(Surekha Janrao) 973
1
0.9999 0.996 0.9965 0.997 0.9975 0.998 0.9985 0.999 0.9995 1 1.0005 COREL
Graphical representation of Table 9 results has been presented in the following Figures 5 to 9 i.e., performance evaluation analysis for multiple regression model for CPP [25] evaluated by using performance parametersFigure5.
ISSN: 2252 8938 Int J Artif Intell, Vol. 11, No. 3, September 2022: 969 976 974 Table 7. ANOVA test for input features for CPP Parameters df SS MS F Significance F Regression 4 1.62E+09 4.04E+08 372145.29 1.93E 26 Residual 725 787428.4 1086.108 Total 729 1.62E+09
MLP REG 1 1.7433 2.0 0.1 % 0.1 % 256.32 ISMO REG 1 4.2731 7.6 0.2 % 0.51% 21.53 SMO REG 1 6.5401 9.7 0.4 % 0.65 % 72.67 BAGG REG 0.9999 13.112 21.63 0.8 % 1.45 % 0.08 G REG 0.9975 339.59 350.58 22.8% 23.7 % 5.04 ADDITIVE REG 0.9999 14.103 16.97 0.9 % 1.14% 0.07
Table 8. Data analysis for significance testing of predictors Parameters Coefficients Standard error t Stat P value Intercept 194.003 27.76939 6.9862 6.40344E 12 Min_Price 0.188153 0.045594 4.126717 4.10703E 05 Max_price 0.04276 0.038175 1.12017 0.263013278 Commodity_Traded_Min 0.48156 0.051445 9.360633 9.73816E 20 Commodity_Traded_Max 0.344199 0.038713 8.890943 4.76037E 18 α=0.05 AND P value should be less than the threshold then only predictors use to predict output are significant otherwise it rejects the null hypothesis. Min_Price(β1)=Max_price(β2)=Commodity_Traded_Min(β3)=Commodity_Traded_Max H1:Atleast, β1 OR β2 OR β3≠0 then ACCEPT H1 and REJECT H0. Table 9. Performance analysis of ML Algorithm for CPP ALGO COREL COEF MAE RMSE RAE RRSE Time (sec)
Performance evaluation for COREL COEF Figure 6. Performance evaluation for mean absolute error (MAE) 3.4. ROI value estimator based on crop yield prediction (CYP) and crop price prediction (CPP) analysis ROI Estimator module is used to calculate profit and loss by using various expenses cost used for the cultivation of crop by farmers. In this module crop yield and crop price has been taken from CPP and CYP module explained in previous section. For the reference we have considered two crops as corn and cotton for yeola region for our experimental work. 1 1 0.9999 0.9975 COEF REGRESSIOR COREL COEF 1.7433 4.2731 6.540113.1128339.591714.103400350300250200150100500MAE REGRESSOR MAE
I would like to express my special thanks to Agro Officer of yeola Region, Nashik district (Mr. Arvind Adhav), Talathi of Nashik district (Mr. Vasant Dhumse), and Gramsevak of yeola region (Mr. Machindra Deore and his team) who helped me a lot for collecting the various type of data.
[1] C. N. Vanitha, N. Archana, and R. Sowmiya, “Agriculture analysis using data mining and machine learning techniques,” in 2019 5th International Conference on Advanced Computing and Communication Systems (ICACCS), Mar. 2019, pp. 984 990, doi: 10.1109/ICACCS.2019.8728382.
[7] R. Kumar, M. P. Singh, P. Kumar, and J. P. Singh, “Crop selection method to maximize crop yield rate using machine learning technique,” in 2015 International Conference on Smart Technologies and Management for Computing, Communication, Controls, Energy and Materials (ICSTM), May 2015, pp. 138 145, doi: 10.1109/ICSTM.2015.7225403.
REFERENCES
ACKNOWLEDGEMENTS
[4] J. Kogan, C. Nicholas, and M. Teboulle, Eds., Grouping multidimensional data. Berlin/Heidelberg: Springer Verlag, 2006.
Int J Artif Intell ISSN: 2252 8938 Return on investment framework for profitable crop recommendation system by …(Surekha Janrao) 975 Figure 7. Performance evaluation for root absolute error (RAE) Figure 8. Performance evaluation for root relative squared error (RRSE) Figure 9. Performance evaluation for time
[6] A. Kumar, S. Sarkar, and C. Pradhan, “Recommendation system for crop identification and pest control technique in agriculture,” in 2019 International Conference on Communication and Signal Processing (ICCSP), Apr. 2019, pp. 185 189, doi: 10.1109/ICCSP.2019.8698099.
[8] E. Manjula and S. Djodiltachoumy, “A model for prediction of crop yield,” Int. J. Comput. Intell. Informatics, vol. 6, no. 4, pp. 298 305, 2017. 0.12%0.29%0.44%0.88%22.87%0.95%10.00%0.00% 20.00% 30.00% RAE REGRESSOR RAE 0.14% 0.52% 0.65% 1.45%23.55%1.14%10.00%0.00% 20.00% 30.00% RRSE REGRESSOR RRSE 256.32 21.53 72.67 0.08 5.04 0.07TIME Regressor TIME
3002001000
[2] R. Medar, V. S. Rajpurohit, and S. Shweta, “Crop yield prediction using machine learning techniques,” Mar. 2019, doi: 10.1109/i2ct45611.2019.9033611.
4. CONCLUSION ROI framework for profitable crop recommendation system has been developed by using optimized MLP regressor algorithm. By applying stochastic gradient decent (SGD) method and hyper tuning parameters i.e., learning rate (Ƞ) and momentum (M) process, RMSE is minimized to 12.32 from 26.79. And data analysis has been applied to get accurate regression statistics which helps us to select appropriate model for crop recommendation system. Knowledge based agriculture system is continuously benefiting our earth and helping people in various aspects of life in terms of crop management and yield improvement.
[5] R. Johnson and T. Zhang, “Learning nonlinear functions using regularized greedy forest,” IEEE Trans. Pattern Anal. Mach. Intell., vol. 36, no. 5, pp. 942 954, May 2014, doi: 10.1109/TPAMI.2013.159.
[3] S. T. Arote and S. M. Lawande, “Agricultural problems and prospects of yeola taluka,” Indian Streams Res. J., vol. 1, no. 5, 2011.
[22] G. B. Huang, H. Zhou, X. Ding, and R. Zhang, “Extreme learning machine for regression and multiclass classification,” IEEE Trans. Syst. Man, Cybern. Part B, vol. 42, no. 2, pp. 513 529, Apr. 2012, doi: 10.1109/TSMCB.2011.2168604.
[21] D. F. Specht, “A general regression neural network,” IEEE Trans. Neural Networks, vol. 2, no. 6, pp. 568 576, 1991, doi: 10.1109/72.97934.
[13] T. van Klompenburg, A. Kassahun, and C. Catal, “Crop yield prediction using machine learning: A systematic literature review,” Comput. Electron. Agric., vol. 177, p. 105709, Oct. 2020, doi: 10.1016/j.compag.2020.105709.
Prof. Surekha Janrao is working as an assistant professor in Terna Engineering College, Navi Mumbai from 2010. She has done her bachelors and master’s in computer engineering from Mumbai University. She has published more than 8 papers in international journal. Her area of interest is Machine Learning, Data Mining, IoT. She is Research scholar and doing research in Machine learning and IoT under the Guidance of Dr. Deven shah. She can be contacted at email: surekhajanrao@ternaengg.ac.in
[11] Aqeel ur Rehman and Z. A. Shaikh, “ONTAgri: scalable service oriented agriculture ontology for precision farming,” 2011.
[12] J. Majumdar, S. Naraseeyappa, and S. Ankalaki, “Analysis of agriculture data using data mining techniques: application of big data,” J. Big Data, vol. 4, no. 1, p. 20, Dec. 2017, doi: 10.1186/s40537 017 0077 4.
[10] D. Sindhura, B. N. Krishna, K. S. P. Lakshmi, B. M. Rao, and J. R. Prasad, “Effects of climate changes on agriculture,” Int. J. Adv. Res. Comput. Sci. Softw. Eng., vol. 6, no. 3, pp. 56 60, 2016.
Dr. Deven Shah is a professor working as the Vice Principal of Thakur College of Engineering and Technology, Mumbai. He is a techie having 25 years of experience in corporate as well as academia. Dr. Deven Shah has served as Principal during previous tenure and has the honorary position of Chairman, Board of Studies (Information Technology) in University of Mumbai. His research work is commendable, and he has filed five patents. During his tenure in industry, he has served as All India Technical Head of the MNC, handling various clients in networking domain. His work in industry was accorded with Best Employment Award. He can be contacted at email: sir.deven@gmail.com.
BIOGRAPHIES OF AUTHORS
[15] S. K. Shevade, S. S. Keerthi, C. Bhattacharyya, and K. R. K. Murthy, “Improvements to the SMO algorithm for SVM regression,” IEEE Trans. Neural Networks, vol. 11, no. 5, pp. 1188 1193, 2000, doi: 10.1109/72.870050.
[24] M. M. Rahman, N. Haq, and R. M. Rahman, “Application of data mining tools for rice yield prediction on clustered regions of Bangladesh,” in 2014 17th International Conference on Computer and Information Technology (ICCIT), Dec. 2014, pp. 8 13, doi: 10.1109/ICCITechn.2014.7073081.
[18] S. D. Pagar, “Spatial analysis of agricultural development in Nashik district: a Tahsil level study,” Peer Rev. Int. Res. J. Geogr. Maharashtra Bhugolshastra Sanshodhan Patrika, vol. 32, no. 1, pp. 37 44, 2015.
[17] P. K. Patra, M. Nayak, S. K. Nayak, and N. K. Gobbak, “Probabilistic neural network for pattern classification,” in Proceedings of the 2002 International Joint Conference on Neural Networks. IJCNN’02 (Cat. No.02CH37290), 2002, pp. 1200 1205, doi: 10.1109/IJCNN.2002.1007665.
[16] O. A. Olanrewaju, A. A. Jimoh, and P. A. Kholopane, “Comparison between regression analysis and artificial neural network in project selection,” in 2011 IEEE International Conference on Industrial Engineering and Engineering Management, Dec. 2011, pp. 738 741, doi: 10.1109/IEEM.2011.6118014.
[23] L. Breiman and P. Spector, “Submodel selection and evaluation in regression. The X random case,” Int. Stat. Rev. Int. Stat., vol. 60, no. 3, p. 291, Dec. 1992, doi: 10.2307/1403680.
[25] L. Breiman and D. Freedman, “How many variables should be entered in a regression equation?,” J. Am. Stat. Assoc., vol. 78, no. 381, pp. 131 136, Mar. 1983, doi: 10.1080/01621459.1983.10477941
ISSN: 2252 8938 Int J Artif Intell, Vol. 11, No. 3, September 2022: 969 976 976 [9] N. Jain, A. Kumar, S. Garud, V. Pradhan, and P. Kulkarni, “Crop selection method based on various environmental factors using machine learning,” Int. Res. J. Eng. Technol., vol. 4, no. 2, pp. 1530 1533, 2017.
[19] J. Lacasta, F. J. Lopez Pellicer, B. Espejo García, J. Nogueras Iso, and F. J. Zarazaga Soria, “Agricultural recommendation system for crop protection,” Comput. Electron. Agric., vol. 152, pp. 82 89, Sep. 2018, doi: 10.1016/j.compag.2018.06.049.
[20] A. Sharma, A. Jain, P. Gupta, and V. Chowdary, “Machine learning applications for precision agriculture: a comprehensive review,” IEEE Access, vol. 9, pp. 4843 4873, 2021, doi: 10.1109/ACCESS.2020.3048415.
[14] M. Fernández Delgado, M. S. Sirsat, E. Cernadas, S. Alawadi, S. Barro, and M. Febrero Bande, “An extensive experimental survey of regression methods,” Neural Networks, vol. 111, pp. 11 34, Mar. 2019, doi: 10.1016/j.neunet.2018.12.010.
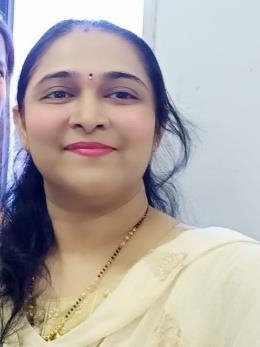



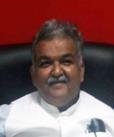



Journal homepage: http://ijai.iaescore.com
1. INTRODUCTION
Article Info ABSTRACT
IAES International Journal of Artificial Intelligence (IJ AI) Vol. 11, No. 3, September 2022, pp. 977 985 ISSN: 2252 8938, DOI: 10.11591/ijai.v11.i3 pp977 985 977
Pipe leakage detection system with artificial neural network
Pipelines are becoming a more popular method for transporting bulk water in many nations since it is a safe and cost effective alternative. Each year, a large number of new pipelines are arranged and built in public and worldwide. Pipeline leaks can potentially cause a wide range of natural disasters and financial problems [1] [6]. The outflow of treated water from this country is higher than 4,27 billion liters due to ageing pipelines. A leading expert warns that unless reactionary measures are taken, more will be wasted. The consequences of this problem may also harm society [7] [11].
For example, a water treatment plant pipeline leak at Sungai Selangor caused a water cut in October 2020. In a statement, Air Selangor reported that more than 686 areas in Petaling Jaya, Gombak, Klang, Shah Alam, Kuala Lumpur, and Hulu Selangor districts were affected. Societies and economies are negatively impacted by this situation [12]. A pipeline can leak for various reasons, such as incorrect installation, the movement of soil around the pipeline, the depth of placement of the pipeline, and the material of the pipeline itself. In order to minimize damage, leak detection that is accurate and enables a quick response is imperative [13]
Article history: Received Jul 21, 2021 Revised Apr 8, 2022 Accepted May 7, 2022 This project aims to develop a system that can monitor to detect leaks in water distribution networks. It has been projected that leakage from pipelines may lead to significant economic losses and environmental damage. The loss of water from leaks in pipeline systems accounts for a large portion of the water supply. Pipelines are maintained throughout their lives span; however, it is difficult to avoid a leak occurring at some point. A tremendous amount of water could be saved globally if automated leakage detection systems were introduced. An embedded system that monitors water leaks can efficiently aid in water conservation. This project focuses on developing a real time water leakage detection system using a few types of sensors: water flow rate sensor, vibration sensor, and water pressure sensor. The data from the sensors is uploaded and stored by the microcontroller (NodeMCU V3) to the database cloud (Google Sheets). The data that is stored in the database is analyzed by artificial neural network (ANN) by using Matlab software. An application is developed based on results from ANN training to detect the leakage event. Implementing the proposed system can increase operations efficiency, reduce delay times, and reduce maintenance costs after leaks are detected.
Muhammad Iqmmal Rezzwan Radzman1, Abd Kadir Mahamad1 , Siti Zarina Mohd Muji1, Sharifah Saon1, Mohd Anuaruddin Ahmadon2, Shingo Yamaguchi2 , Muhammad Ikhsan Setiawan3 1Faculty of Electrical and Electronic Engineering, University of Tun Hussein Onn Malaysia, Johor, Malaysia 2Graduate School of Sciences and Technology for Innovation, Yamaguchi University, Ube, Japan 3Faculty of Civil Engineering, Narotama University, Surabaya, Indonesia
This is an open access article under the CC BY SA license. Corresponding Author: Abd Kadir DepartmentMahamadofElectrical and Electronic Engineering, Faculty of Electrical and Electronic Engineering, University Tun Hussein Onn Malaysia 86400 Parit Raja, Johor, Malaysia Email: kadir@uthm.edu.my
Keywords: Artificial neural networks Cloud ReGoogledatabasesheetsaltimewater leakages
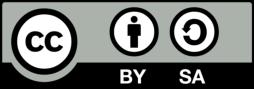
This project aims to develop a pipeline leak detection system that operates in real time, using the ANN [19] [23] and internet of things (IoT) [24] [26]. Wireless communication is possible with this system. A cloud server hosts the outputs data of the system, which is analyzed by ANN. As a result of the cost and instrumentation limitations, vibration sensors module (SW 420), flowrate sensors (YF 201), and water pressure sensors (SKU SEN0257) have been used to sense vibration, flowrate, and pressure from the pipeline. 2.1. Methods In this project, the vibration sensor, flow rate sensor, and pressure sensor has been used to detect water leaks. As a result, the sensor does not obstruct water flow but simply senses the vibration, flowrate, and pressure and collects the data. Microcontrollers are used in this project to read data from multiple sensors and monitor pressure, vibration, and flowrate. Data has been collected and uploaded to the cloud by the system. A neural network from MATLAB software has been used to analyze the stored data. Then, a minimalist application is developed based on ANN analysis results to detect leaks. The flowchart in Figure 1 provides an overview of the overall process.
2. RESEARCH METHOD
An artificial neural network (ANN) is a simulation model that simulates the way of the human brain analyses, and processes information. With ANN, problems that are impossible or difficult to solve by human or statistical standards are resolved. As a result of self learning capabilities, this computing system is able to produce better data analysis. ANNs consist of hundreds or thousands of artificial neurons, called processing units, interconnected by nodes. A processing unit consists of an input unit and an output unit. Input units receive information in a variety of forms and structures based on internal weightings, and neural networks are learning about information to produce an output. ANNs with feed forward connections have connections between processing units that do not form a cycle. The input layer, hidden layer, and the output layer of this ANN are all made up of layers. It is the first and most straightforward type of ANN and be implemented in this proposed system.
ISSN: 2252 8938 Int J Artif Intell, Vol. 11, No. 3, September 2022: 977 985 978
As per the specification report by Ranhill SAJ 2018, clean water shall be used for pipe leak testing, and air trapped inside the system shall be exhausted via a vent. A pressure test conducted at the end of 24 hours, and the rate of loss has been calculated by dividing it by time [14]. A long time is required for this method to yield a result, and it is less accurate. This project proposes using a piezoelectric sensor, flowrate sensor, and pressure sensor attached to a steel pipe to detect pipeline leaks. Any leakage detected will be sensed by the sensor, and data automatically been sent to the cloud (Google Sheets). Then, artificial neural networks (ANNs) has been used to analyze the data to determine the degree of leakage in the pipe. A pipe leak occurs when liquids and gases escape from the pipeline through a leak or crack. A relationship between leak outflows and flow conditions of water distribution systems must be defined to understand pipe leakage conditions. The relationship between these two variables is crucial in water distribution systems. The leak outflow, ����, depends mainly on the effective leak area �������� defined as the product of the discharge coefficient ����and of the leak area ���� and on the total head inside the pipe, H, or on the piezometric head, h. Other quantities can also be considered, as pipe thickness, discharge conditions (in air/submerged), ratio ����/���� (with ���� being the discharge upstream the leak), and, for large leaks, leak shape [15], [16]. In steady state conditions, the general equation ���� =������ (1) is often used [17], that includes the Torricelli’s equation ���� = ��������√2���� (2) when a= ��������(2��)1/2 and b=1/2. The (1) is used both at a global/district area scale, with H being a “mean pressure” over the district and ���� is the flow entering the district and at a local scale, considering a single leak. In both cases, on a local as well as on a global scale, the variation of �������� with H can be used to explain the increase of the b exponent with respect to Torricelli’s formula [18].
Figure 4 gives a comparison of vibrations based on two different data samples. Vibration data from pipeline systems that had a leakage and those without a leakage show significant differences. Vibration data for leakage events is very high compared to vibration data without leakage events. The pipe produced a force
�� = �� �� (3)
3.1. Flowrate
3. RESULTS AND DISCUSSION
Figure 1. The flowchart for overall process flow
According to Figure 2, two types of water flowrate data were taken. There were the flowrate data for the pipeline system with no leakage and with a leakage event. The unit of measurement for the above data distribution is liters per minute (L/min). The flowrate data for a pipeline system with no leakage event is higher than the flowrate data for a pipeline system with a leakage event. Due to no leaks in the pipeline, water flows in one way without being disturbed by changes in flow velocity (V). The water would flow outside the pipe if the leak occurred in the pipeline. This may affect the flow velocity of water when such an event occurs. When a leak has occurred, the flow of water becomes slower. Based on Figure 3, flowrate (Q) is defined to be the volume (v) of fluid passing by some location through an area during a period of time (t). Based on (3), the flowrate value (Q) decreased if the velocity of water decreases.
3.2. Vibration
Int J Artif Intell ISSN: 2252 8938 Pipe leakage detection system with artificial neural network (Muhammad Iqmmal Rezzwan Radzman) 979
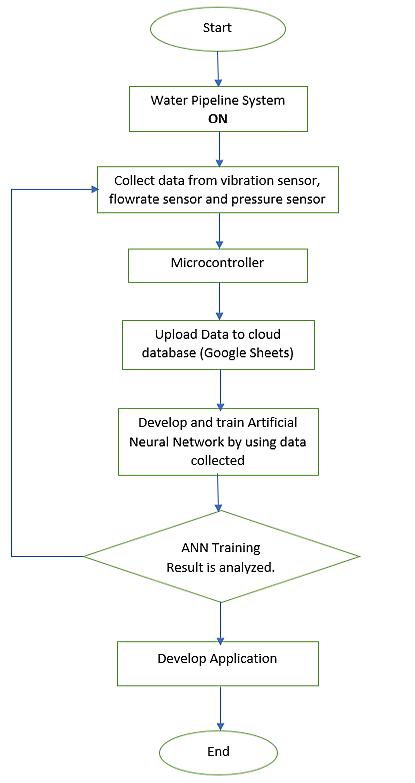
Figure 2. Flowrate result comparison
ISSN: 2252 8938 Int J Artif Intell, Vol. 11, No. 3, September 2022: 977 985 980that resulted in an unstable flow of water outside the pipe that caused this situation to occur. Despite the absence of leakage on this pipeline system, vibrations do occur. However, they are deficient in comparison with the vibration reading on the pipeline system with an event.
Figure 3. Flow rate and its relation to velocity
Figure 4. Vibration result comparison
This project finds vibration to be a particularly sensitive parameter because of many external disturbances that can prevent the data from being read properly from the vibration sensor, for example, the vibration from the water pump, or the presence of unwanted movement close to the pipeline system. Figure 5 shows the precautions taken while working on this project to obtain accurate data and reduce an external disturbance.
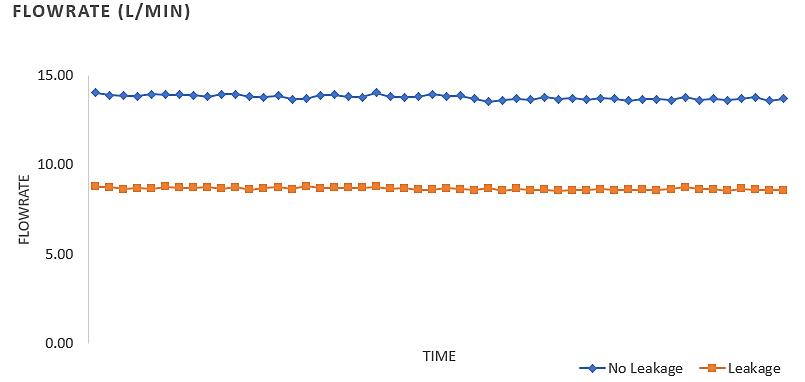
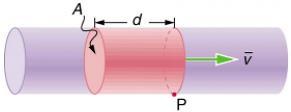
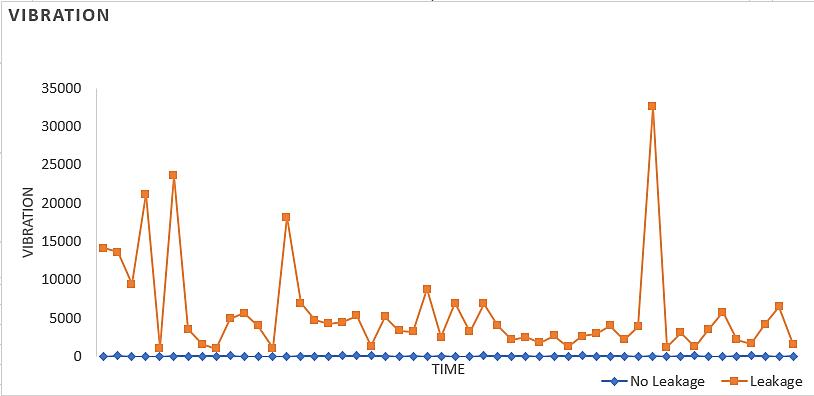
Figure 5. The frame that supports the pipeline system
3.4. Liquid output Figure 7 shows the results of the liquid output of the pipeline system for leakage event and no leakage event. From the results of both of the liquid output, the result is increased directly proportional over time. But there is a noticeable difference in term of the gradient of the results. The gradient liquid output results for no leakage event in the pipeline system is steeper than results for leakage event in the pipeline system. This is because of the difference in the volume of water flow through the pipeline. When the leakage has occurred, water flows outside the pipe and caused the loss of volume of water in the pipeline.
3.3. Pressure Figure 6 shows the different results of pressure reading data for pipeline systems with leakage and no leakage. Based on the results, the pressure data reading from the pipeline system shown in Figure 5, with a leakage event is lower than pressure data for pipeline systems with no leakage event. It is because the pressure in the pipeline system is decreased due to the existence of the leakage.
Int J Artif Intell ISSN: 2252 8938 Pipe leakage detection system with artificial neural network (Muhammad Iqmmal Rezzwan Radzman) 981
Figure 6. Pressure result comparison
3.5. Result of artificial neural network (ANN) In order to develop this ANN, it needs many data to develop a more stable and accurate ANN. In this project, the amount of data used to develop ANN is more than 3000 data for both types of data leakage and no leakage event. This ANN consists of a single layer and 10 nodes. Figure 8 to 11 show the result of ANN training, validation, testing and correlation coefficient R2 for this project respectively.
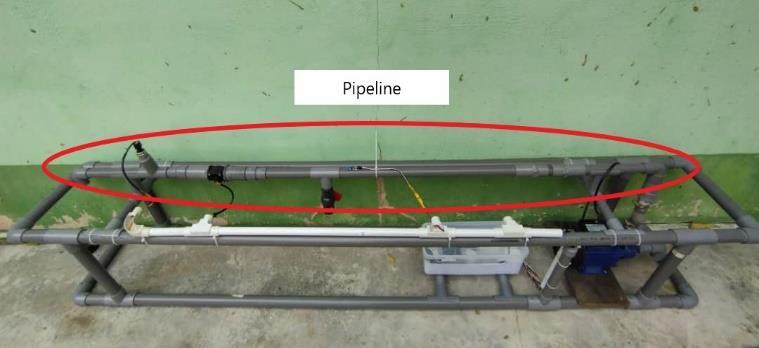
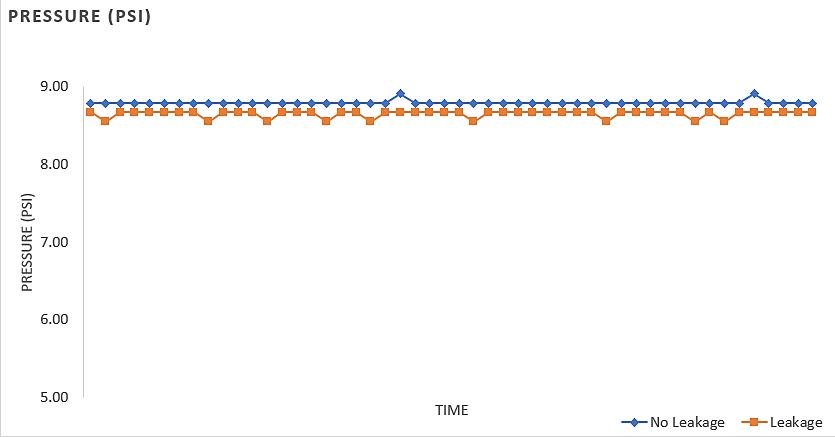
8
An application is developed to the interface for the user to key in the sensor reading. This application has been determined the existence of leakage events based on the data given by the user. This
Figure Results of ANN Results for output vs target of
3.6. Result
ISSN: 2252 8938 Int J Artif Intell, Vol. 11, No. 3, September 2022: 977 985 982 Figure 7. Liquid output result comparison
training Figure
10
application development
testing Figure 11
Figure Results of ANN 9 Results of ANN validation
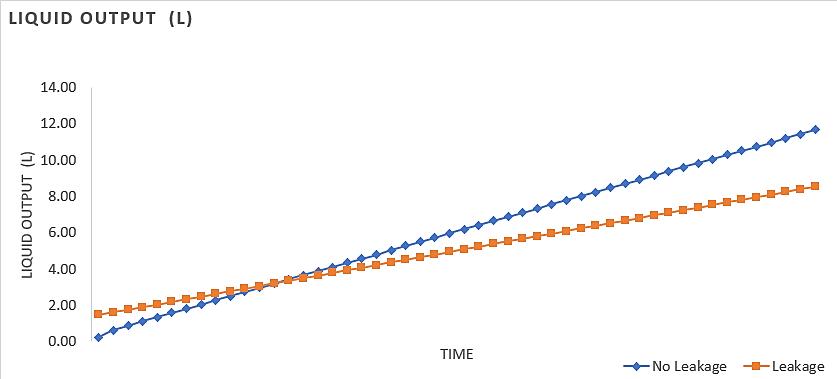
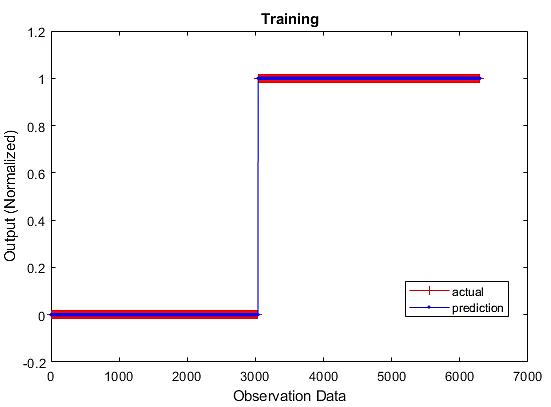
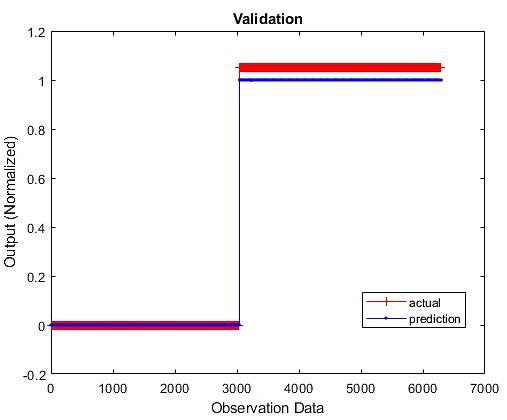
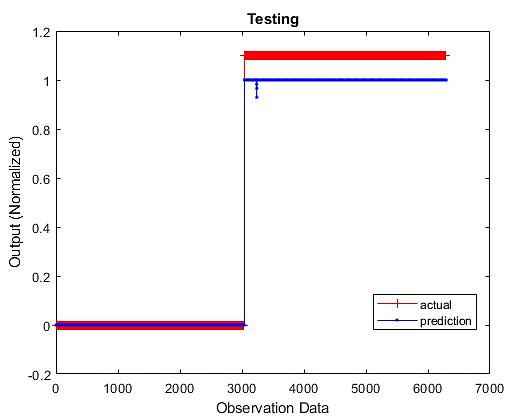
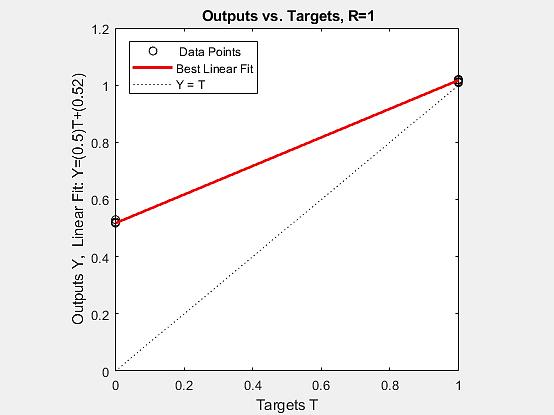
[7] S. S. Sufian, N. S. Romali, R. A. Rahman, and M. A. Seman, “Current practice in rehabilitating old pipes for water distribution network in Malaysia,” IOP Conf. Ser. Earth Environ. Sci., vol. 641, no. 1, p. 12011, Feb. 2021, doi: 10.1088/1755 1315/641/1/012011.
application interface is developed by using application designer on MATLAB Software, and the functionality of the application is determined based on ANN training results. The application is extracted from the MATLAB software and is a standalone application in extension format (exe). Figures 12 and 13, show the result “NO LEAKAGE” and “LEAKAGE ALERT!!!” when the random untrained data for no leakage event and leakage event on the pipeline system is key in by the user to the application. 12 Application result for no leakage event data Figure 13 Application result for leakage event data
Figure
[3] B. M. S. Arifin, Z. Li, S. L. Shah, G. A. Meyer, and A. Colin, “A novel data driven leak detection and localization algorithm using the Kantorovich distance,” Comput. Chem. Eng., vol. 108, pp. 300 313, Jan. 2018, doi: 10.1016/j.compchemeng.2017.09.022.
Int J Artif Intell ISSN: 2252 8938
In conclusion, this project is developed successfully. With an algorithmically programmed approach, it can determine leakage on pipelines based on real time data. Additionally, the data was uploaded to the cloud database, which can be used as a reference or record in the future. In addition, the pipe leakage detection system based on ANN application has been successfully developed and can detect the leakage event with real time data from the sensor. This project also includes an application representing the interface of the system. As well as being effective, easy to use, and compatible with a wide range of devices, this application also works properly.
Pipe leakage detection system with artificial neural network (Muhammad Iqmmal Rezzwan Radzman) 983
4. CONCLUSION
Communication of the research is made possible through monetary assistance by Universiti Tun Hussein Onn Malaysia, and this paper was partially supported by Faculty of Engineering, Yamaguchi University, Japan. REFERENCES [1] X. J. Wang, M. F. Lambert, A. R. Simpson, and J. P. Vítkovský, “Leak detection in pipeline systems and networks: A Review,” in Conference on Hydraulics in Civil Engineering, 2001, pp. 1 10. [2] I. A. Tijani, S. Abdelmageed, A. Fares, K. H. Fan, Z. Y. Hu, and T. Zayed, “Improving the leak detection efficiency in water distribution networks using noise loggers,” Sci. Total Environ., vol. 821, p. 153530, May 2022, doi: 10.1016/j.scitotenv.2022.153530.
ACKNOWLEDGEMENTS
[4] M. Liu, J. Yang, S. Li, Z. Zhou, E. Fan, and W. Zheng, “Robust GMM least square twin K class support vector machine for urban water pipe leak recognition,” Expert Syst. Appl., vol. 195, p. 116525, Jun. 2022, doi: 10.1016/j.eswa.2022.116525.
[5] M. J. Brennan, Y. Gao, P. C. Ayala, F. C. L. Almeida, P. F. Joseph, and A. T. Paschoalini, “Amplitude distortion of measured leak noise signals caused by instrumentation: Effects on leak detection in water pipes using the cross correlation method,” J. Sound Vib., vol. 461, p. 114905, Nov. 2019, doi: 10.1016/j.jsv.2019.114905.
[6] F. Piltan and J. M. Kim, “Leak detection and localization for pipelines using multivariable fuzzy learning backstepping,” J. Intell. Fuzzy Syst., vol. 42, no. 1, pp. 377 388, Dec. 2021, doi: 10.3233/JIFS 219197.
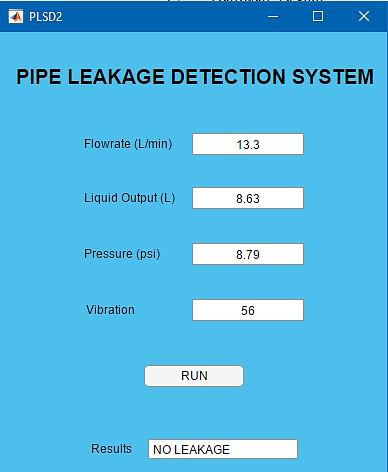
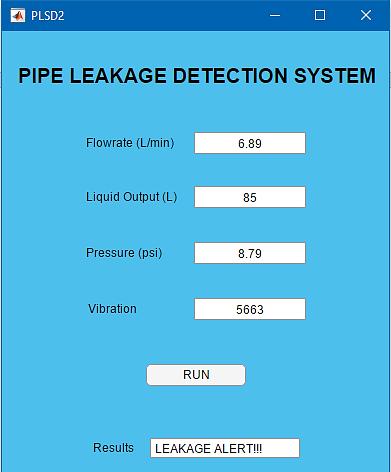
[15] A. Keramat, B. Karney, M. S. Ghidaoui, and X. Wang, “Transient based leak detection in the frequency domain considering fluid structure interaction and viscoelasticity,” Mech. Syst. Signal Process., vol. 153, p. 107500, May 2021, doi: 10.1016/j.ymssp.2020.107500.
[22] E. J. Pérez Pérez, F. R. López Estrada, G. Valencia Palomo, L. Torres, V. Puig, and J. D. Mina Antonio, “Leak diagnosis in pipelines using a combined artificial neural network approach,” Control Eng. Pract., vol. 107, p. 104677, Feb. 2021, doi: 10.1016/j.conengprac.2020.104677.
[23] T. Dawood, E. Elwakil, H. M. Novoa, and J. F. Gárate Delgado, “Toward urban sustainability and clean potable water: Prediction of water quality via artificial neural networks,” J. Clean. Prod., vol. 291, p. 125266, Apr. 2021, doi: 10.1016/j.jclepro.2020.125266.
[19] C. Ai, B. Wang, and H. Zhao, “Detection and fault diagnosis for acoustic vibration of pipes buried in soil,” in 2006 6th World Congress on Intelligent Control and Automation, 2006, pp. 5756 5759, doi: 10.1109/WCICA.2006.1714178.
[14] R. Beieler and A. Roshak, “Useful tips and tools for design of ductile iron and PVC pipelines,” in Pipelines 2014, Jul. 2014, pp. 594 604, doi: 10.1061/9780784413692.055.
[17] M. Ferrante, B. Brunone, and S. Meniconi, “Wavelets for the analysis of transient pressure signals for leak detection,” J. Hydraul. Eng., vol. 133, no. 11, pp. 1274 1282, Nov. 2007, doi: 10.1061/(ASCE)0733 9429(2007)133:11(1274).
[18] J. E. van Zyl and C. R. I. Clayton, “The effect of pressure on leakage in water distribution systems,” Proc. Inst. Civ. Eng. Manag., vol. 160, no. 2, pp. 109 114, Jun. 2007, doi: 10.1680/wama.2007.160.2.109.
BIOGRAPHIES OF AUTHORS Muhammad Iqmmal Rezzwan Radzman graduate from Johor Matriculation College for pre University Tertiary Education (2017), and Bachelor of Electronic Engineering at University Tun Hussein Onn Malaysia in 2021. Currently he is an engineer and independent reseacher. His research interests include automation, electronic, SMT, and embedded system. He can be contacted at email: iqmmalrezzwan@topempire.com.my
[11] S. K. Vandrangi, T. A. Lemma, S. M. Mujtaba, and O. T. N., “Developments of leak detection, diagnostics, and prediction algorithms in multiphase flows,” Chem. Eng. Sci.,vol. 248, p. 117205, Feb. 2022, doi: 10.1016/j.ces.2021.117205.
[12] T. Kawata, Y. Nakano, T. Matsumoto, A. Mito, F. Pittard, and H. Noda, “Challenge in high speed TBM excavation of long distance water transfer tunnel, Pahang Selangor raw water transfer tunnel, Malaysia,” 2014.
[25] Y. Kim, S. J. Lee, T. Park, G. Lee, J. C. Suh, and J. M. Lee, “Robust leak detection and its localization using interval estimation for water distribution network,” Comput. Chem. Eng., vol. 92, pp. 1 17, Sep. 2016, doi: 10.1016/j.compchemeng.2016.04.027.
[20] Q. Xu and W. Kong, “Research on forecast model based on BP neural network algorithm,” J. Phys. Conf. Ser., vol. 1982, no. 1, p. 12065, Jul. 2021, doi: 10.1088/1742 6596/1982/1/012065.
[24] B. Zhou, V. Lau, and X. Wang, “Machine learning based leakage event identification for smart water supply systems,” IEEE Internet Things J., vol. 7, no. 3, pp. 2277 2292, Mar. 2020, doi: 10.1109/JIOT.2019.2958920.
[26] S. T. N. Nguyen, J. Gong, M. F. Lambert, A. C. Zecchin, and A. R. Simpson, “Least squares deconvolution for leak detection with a pseudo random binary sequence excitation,” Mech. Syst. Signal Process., vol. 99, pp. 846 858, Jan. 2018, doi: 10.1016/j.ymssp.2017.07.003.
[9] X. Hu, Y. Han, B. Yu, Z. Geng, and J. Fan, “Novel leakage detection and water loss management of urban water supply network using multiscale neural networks,” J. Clean. Prod., vol. 278, p. 123611, Jan. 2021, doi: 10.1016/j.jclepro.2020.123611.
[21] R. B. Santos, M. Rupp, S. J. Bonzi, and A. M. F. Filetia, “Comparison between multilayer feedforward neural networks and a radial basis function network to detect and locate leaks in pipelines transporting gas,” Chem. Eng. Trans., vol. 32, pp. 1375 1380, 2013.
ISSN: 2252 8938 Int J Artif Intell, Vol. 11, No. 3, September 2022: 977 985 984 [8] Y. Gao, Y. Liu, Y. Ma, X. Cheng, and J. Yang, “Application of the differentiation process into the correlation based leak detection in urban pipeline networks,” Mech. Syst. Signal Process., vol. 112, pp. 251 264, Nov. 2018, doi: 10.1016/j.ymssp.2018.04.036
Abd Kadir Mahamad received his Bachelor of Science in Electrical Engineering (2002) and Master of Electrical Engineering (2005) from University Tun Hussein Onn Malaysia before pursuing Doctor of Philosophy (Computer Science and Electrical Engineering) at Kumamoto University, Japan (2010). He currently an Associate Professor at Faculty of Electrical and Electronic Engineering UTHM and registered as Professional Engineer. During the period of May 2015 through May 2016, he was doing industrial attachment at Melaka ICT Holdings Sdn Bhd, as Executive Assistant Manager. He was involved in the Smart City project in Melaka. He currently leads a research team in Video Analytic and internet of things (IoT). His research interests include Deep Learning, Smart City, Intelligent System applications and embedded system. He can be contacted at email: kadir@uthm.edu.my.
[10] B. C. Yalçın, C. Demir, M. Gökçe, and A. Koyun, “Water leakage detection for complex pipe systems using hybrid learning algorithm based on ANFIS method,” J. Comput. Inf. Sci. Eng., vol. 18, no. 4, Dec. 2018, doi: 10.1115/1.4040130.
[16] A. M. Cassa, J. E. van Zyl, and R. F. Laubscher, “A numerical investigation into the effect of pressure on holes and cracks in water supply pipes,” Urban Water J., vol. 7, no. 2, pp. 109 120, Apr. 2010, doi: 10.1080/15730620903447613.
[13] A. A. Lyapin and Y. Y. Shatilov, “Vibration based damage detection of steel pipeline systems,” in Non destructive Testing and Repair of Pipelines, 2018, pp. 63 72.
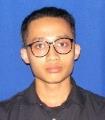



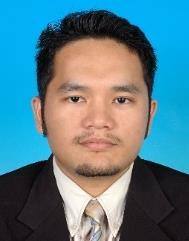



Int J Artif Intell ISSN: 2252 8938 Pipe leakage detection system with artificial neural network (Muhammad Iqmmal Rezzwan Radzman) 985
Sharifah Saon is currently a senior lecturer in the Faculty of Electrical and Electronic Engineering, Universiti Tun Hussein Onn Malaysia, Malaysia, and registered Professional Technologists. She received the Bachelor of Science in Electrical Engineering and Master of Electrical Engineering from Universiti Teknologi Malaysia, and Kolej Universiti Tun Hussein Onn Malaysia, Malaysia, in 2001, and 2004, respectively. Her research interest is in the area of theoretical digital signal processing, visible light communication, and digital and data communication, including the application to the internet of things (IoT) and big data analysis. She is a member of IEEE, Institute of Engineering Malaysia (IEM), Board of Engineering Malaysia (BEM), and Professional Technologist of Malaysia Board of Technologists (MBOT). She can be contacted at email: sharifa@uthm.edu.my Mohd Anuaruddin B. Ahmadon graduated from Kumamoto National College of Technology, Japan, in 2012. He received his B. Eng. (2014), M. Eng. (2015) and Dr. Eng. (2017) from Yamaguchi University, Japan. He is currently an Assistant Professor at Graduate School of Sciences and Technology for Innovation, Yamaguchi University, Japan. He was awarded IEEE Consumer Electronics Society East Japan Young Scientist Paper Award in 2016. His research interests include formal methodology in software and service engineering and its application to cyber security and Internet of Things. He is a member of IEEE and Consumer Technology Society. He can be contacted at email: anuar@yamaguchi u.ac.jp
Shingo Yamaguchi is currently a (full) professor in the Graduate School of Sciences and Technology for Innovation, Yamaguchi University, Japan. He received the B.E., M.E. and D.E. degrees from Yamaguchi University, Japan, in 1992, 1994 and 2002, respectively.
Muhammad Ikhsan Setiawan received his Bachelor of Civil Engineering (2002) from Universitas Merdeka, Malang, Indonesia, and Master of Civil Engineering (2005) from Universitas Indonesia before pursuing Doctor of Philosophy (Civil Engineering) at Universitas Tarumanagara, Indonesia (2010). He currently an Assistant Professor at Faculty of Civil Engineering, Narotama University, Indonesia and registered as Engineer Expert Certified. He currently leads a research team in Sustainable and Digital in Transportation, Tourism and Regional Economic, grant from Ministry of Education, Indonesia. His research interests include Smart City and Sustainability. He is also a Chairman of WORLDCONFERENCE.ID, IPEST commerce, SONGSONG ridt, member of IEEE, editors, and reviewers some Journal indexed in SCOPUS, DOAJ, CROSSREF and GOOGLE, also until now as Vice Rector of Narotama University, Indonesia. He can be contacted at email: Ikhsan.setiawan@narotama.ac.id.
He was an Assistant Professor in the Faculty of Engineering, Yamaguchi University, from 1997 to 2007. He was also a Visiting Scholar in the Department of Computer Science at University of Illinois at Chicago, United States, in 2007. He was an Associate Professor in the Graduate School of Sciences and Technology for Innovation, Yamaguchi University, from 2007 to 2017. His research interest is in the area of theoretical computer science and software engineering, including their application to business process management, IoT, bigdata analysis, AI, and cyber security. He is also a Senior Member of IEEE, Board of Governors of IEEE Consumer Electronics Society, Young Professionals Chair of IEEE Consumer Electronics Society, and a member of International Coordination Committee of ITC CSCC. In academic societies other than IEEE and IEICE, he plays many important roles, which is an Editorial Board Member of International Journal of Internet of Things and Cyber Assurance. He can be contacted at email: shingo@yamaguchi u.ac.jp
Siti Zarina Mohd Muji is Associate Professor at Department of Electronic Engineering in Computer field at Faculty of Electronic and Electrical Engineering, Universiti Tun Hussein Onn Malaysia (UTHM). Her research interest is in Embedded System and Application, Tomography, and Sensor and Application. She currently being a head of several grants includes Fundamental Research Grant Scheme (FRGS), Multidisciplinary Research (MDR) and MTUN Commercialization (Internal Grant). For FRGS grant, this is a collaboration with oil and gas industry that related to pipe corrosion. For MDR, this research focus on detection of pipe leakage at UTHM and for MTUN Commercialization is a grant to commercialize Jaundice Meter which is a device to detect the jaundice among babies. She also involves in publishing articles in journal (50), book chapter (10), technical papers in conferences (>30) and peer reviewed journal (>20) (IEEE sensor, Sensor and Actuator B Chemical and many more). She also won several medals in research and innovation showcases where a Jaundice Meter project won a gold medal in Pecipta 2017. She can be contacted at email: szarina@uthm.edu.my
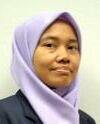







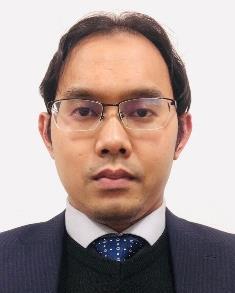



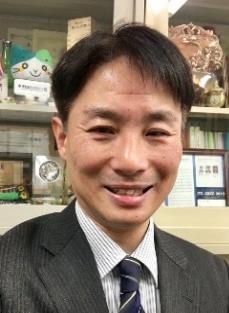



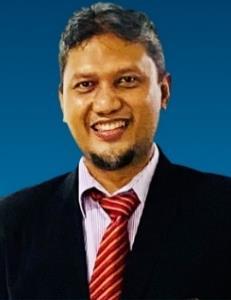



In the last few years, there has been a lot of research into the use of machine learning for speech recognition applications. However, applications to develop and evaluate air traffic controllers' communication skills in emergency situations have not been addressed so far. In this study, we proposed a new automatic speech recognition system using two architectures: The first architecture uses convolutional neural networks and gave satisfactory results: 96% accuracy and 3% error rate on the training dataset. The second architecture uses recurrent neural networks and gave very good results in terms of sequence prediction: 99% accuracy and �� 7% error rate on the training dataset. Our intelligent communication system (ICS) is used to evaluate aeronautical phraseology and to calculate the response time of air traffic controllers during their emergency management. The study was conducted at International Civil Aviation Academy, with third year air traffic control engineering students. The results of the trainees' performance prove the effectiveness of the system. The instructors also appreciated the instantaneous and objective feedback.
Artificial intelligence in a communication system for air traffic controllers' emergency training
Article Info ABSTRACT
This is an open access article under the CC BY SA license. Corresponding Author: Youssef Mnaoui, Signals, Distributed Systems and Artificial Intelligence Laboratory, ENSET Mohammedia, Hassan II University, Casablanca,Morocco Email: youssef.mnaoui etu@etu.univh2c.ma 1. INTRODUCTION Maintaining and improving human performance can only be achieved by focusing training on the skills needed to perform their duties safely and effectively, and if the training involves a variety of scenarios that expose people to the most relevant threats and errors in their environment. Human error is frequently cited in air accident investigation reports as a major cause of accidents and serious incidents, despite the evolution of the technologies and safety systems used [1]. Security is therefore only possible through practical training that makes error less probable and their consequences less serious. When an aviation emergency is declared, it is mandatory to think quickly and act immediately [2]. However, the need to communicate effectively and in a timely manner, as well as the lack of qualified personnel and time, causes stress that impairs the air traffic controller's situational awareness and decision making and can lead to serious incidents: 60% of communication errors between pilots and controllers are the cause of accidents or incidents [3] According to a study by NASA's aviation safety reporting systems (ASRS) database, Incorrect controller pilot communication is a causal factor in 80% of aviation incidents or accidents, while late communication accounts for 12% of the causes leading to incidents or accidents, as shown in Table 1 [4]
IAES International Journal of Artificial Intelligence (IJ AI) Vol. 11, No. 3, September 2022, pp. 986 994 ISSN: 2252 8938, DOI: 10.11591/ijai.v11.i3.pp986 994 986
Keywords: Air traffic recognition Convolutional neural networks
Youssef Mnaoui, Aouatif Najoua, Hassan Ouajji Signals, Distributed Systems and Artificial Intelligence Laboratory, ENSET Mohammedia University of Hassan II, Casablanca, Morocco
Automaticcontrolspeech
Article history: Received Aug 30, 2021 Revised May 26, 2022 Accepted Jun 9, 2022
Emergency services RecurrentPhraseologyneural networks
Journal homepage: http://ijai.iaescore.com
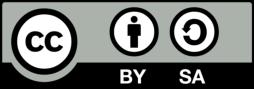
pilot communication [5] Voice Communication ControllerAtc TerminalPseudoPilote StructureAirspace FlightSimulationDisplayRadarATCPlansAircraftParametersControlInputs ControlInputs Pseudo Pilote
In order to reduce communication errors and ensure redundancy of communications between the controller and the pilot, the International Civil Aviation Organization (ICAO) has established a confirmation and correction process as a defence against communication errors [4], as shown in the Figure 1 However, in an abnormal or emergency situation (ABES), where every second counts, this communication loop can only be effective if the aeronautical phraseology used is correct and standard. Communication errors and the waste of time repeating messages in this kind of stressful situation can have tragic consequences.
Table 1. Communication factors [4] Factor Percentage of Reports communication 80% Absence of communication 33% Correct but late communication 12%
Int J Artif Intell ISSN: 2252 8938 Artificial intelligence in a communication system for air traffic … (Youssef Mnaoui) 987
Incorrect
Figure 1 . Controller pilot communication loop [4]
Traditionally, the performance of trainee air traffic controllers is assessed on simulators by requiring the presence of pseudo pilots. Figure 2 illustrates the whole controller/pseudo pilot communication process and associated devices [5]. However, the evaluation of performance during an emergency or abnormal situation (aircraft engine failure in our scenario) should not only determine whether the communication has been made but also whether the aeronautical phraseology is used correctly and in a timely manner. This can only be achieved by designing new systems that can perform an instantaneous and objective assessment of air traffic controllers' verbal communication.Figure2.Controller/Pseudo
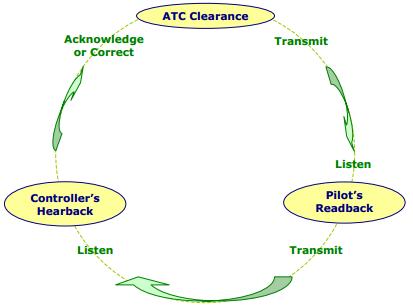
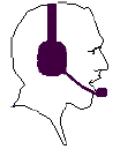
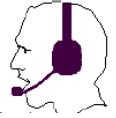
Utterance selection PhraseologyCORPUS UtteranceSpeechAcceptsignalcheckdeviation No Yes End Chronometercheck Emergency
Deep learning algorithms have mainly been used to improve the computer's capabilities to understand human behaviour, including speech recognition [6]. With the introduction of artificial intelligence [7], [8] speech recognition has in fact received a lot of attention in recent years and is proving to be an excellent tool for the analysis of instantaneous phraseology in an Air traffic controllers (ATC) simulator environment by replacing pseudo pilots with an automatic speech recognition device [9]. It is therefore interesting to implement new interactive systems based on automatic speech recognition that allow the evaluation of air traffic controllers' communication skills, especially when they are confronted with stressful situations [10]. Our present study thus aims to propose a new intelligent communication system based on automatic speech recognition that should recognise the phraseology errors made in real time by student air traffic controllers when faced with ABES. To achieve this, we organise our present paper as follows: section 1 gives an overview of the proposed system, while section 2 presents the creation of an intelligent speech recognition architecture using convolutional neural networks (CNN) and recurrent neural networks (RNN). The results and performance are described in section 3. Finally, section 4 concludes our research work.
Figure 3. Flowchart describing The following rules will be used to assess performance. It should be noted that this is an aircraft engine failure event: Each emergency activation is a consequence of detecting the term "Emergency". The "Emergency" expression is the event that triggers the emergency situation. Once an emergency has been activated, a chronometer is set up to calculate the time spent in the whole emergency exercise. Time pressure is an essential element of emergency management. Wasting time
ISSN: 2252 8938 Int J Artif Intell, Vol. 11, No. 3, September 2022: 986 994 988
2. RESEARCH METHOD 2.1. Overview of the proposed system
ATC are trained to use standard and correct International Civil Aviation Organisation (ICAO) phraseology. However, it has been observed that many air traffic controllers can work for long periods without being exposed to ABES. This lack of practice changes the aeronautical phraseology used without the air traffic controller being aware of it. To address this lack of practice, it was decided to develop a new system based on automatic speech recognition technology that allows interaction between the student and the machine without the need for a second person to perform the pseudo pilot task. The speech recognition function will serve as a basic tool for improving the quality of the evaluation. The user's willingness to move on to the next phase will be instantaneous. In addition, a time function has been integrated into the proposed system in order to determine the overall duration of the performance. The process chain can thus be described as illustrated Figure 3.
Int J Artif Intell ISSN: 2252 8938 Artificial intelligence in a communication system for air traffic … (Youssef Mnaoui) 989 repeating messages and finding the correct phraseology to be understood will reduce the efficiency of the air traffic controller, cause misunderstandings and will not ensure pilot confidence in the service
2.2.2. MFCC MFCC are cepstral coefficients calculated by a discrete cosine transformation applied to the signal's power spectrum. The frequency bands of this spectrum are logarithmically spaced along the Mel scale. The MFCC computation is the replication of the human auditory system that aims at an artificial implementation of the working principle of the ear, assuming that the human ear provides a reliable means of speaker recognition [17] In our research, these coefficients are obtained by the following stages as illustrated in Figure 4 [15]: - Cut the signal into "frames". Apply the Fourier transform to the acoustic signal corresponding to each frame to obtain the frequency spectrum of each signal Apply a logarithmic filter to the obtained spectrum as illustrated in (1) : ������(��)=2595������(1+��700) (1)
There are a variety of different options for representing the speech signal for the process of recognition, Mel frequency cepstral coefficient (MFCC) is the most popular [16] The particularity of the transformation into MFCC is is that even more accuracy is obtained by increasing the size of the acoustic characteristic vectors, or by increasing their number. That is by increasing the number of MFCC coefficients.
Reapply a Fourier transform to a Figurecosine.4.Mel frequency cepstral coefficient [15] Break the Signal into overlapping Frames Faster Fourier Transform Filter Bank LogFaster Fourier Transform Audio Signal coefficientsCepstral
The chronometer will always remain on while the practical exercise is in progress. The total time of the simulation will be a decisive factor in assessing the student's performance.
Theprovided.input to the system is the student's speech and a list of predicted phraseology from the newly designed phraseology corpus. The corpus contains (so far) thirty six transcripts from twelve students (seven female and five male) who were asked to participate in three different scenarios: i) loss of separation between two aircraft during initial climb due to engine failure; ii) failure of ground to air communication; and iii) overflying a restricted area due to bad weather conditions. The students' communications were conducted in English, the official and most widely used language in aviation [11]. The student's speech is compared to the word sequences in the corpus to detect errors. If the answer is not accepted, the student is invited to try again. Each passage between the phases is the consequence of detecting the term "check".
2.2. Data 2.2.1. Features extraction Poor quality or erroneous data can lead to difficulties in extracting information and making wrong predictions, so data must be properly prepared and collected Features extraction is generally referred to as front end signal processing [12] Feature extraction techniques typically produce a multidimensional feature vector for each speech signal [13] It is noted that speech features play an essential role in separating one speaker from another [14] The extraction of features reduces the magnitude of the speech signal in a way that does not damage its power [15] In our research, this is the first step that each of the recording files will go through. It consists of the transformation of one dimensional audio data into three dimensional spectrograms after extraction of vectors characteristic of each vocal signal.
During the project, we tested different architectures, which gave different results: the first architecture uses CNNs and gave satisfactory results: 96% accuracy and 3% error rate on the training dataset. The second is a recursive approach, using RNNs, which is notably good in terms of sequence prediction: 99% accuracy and �� 7 % error rate on the training dataset. The architectures used for the two kinds of models described are respectively as listed in Figure 5. 2.4. Training After creating the model's brain, the collected data will be used to find a value for the parameters of the model that will allow it to properly perform its recognition task. The training of the model is done in our case using a well known technique in optimization: the gradient descent [23], [24]. A neural network is a set of calculating stages where formatted data will enter and be transformed in order to extract characteristics .This transformation will be done by means of weights and by the successive application of two mathematical operations: a linearity and a non linearity [25]. The hyper parameters of a model are first initialised with random values, then during training the output of the model will be calculated and compared to the expected value (from the dataset). The (4) represents the error terms obtained by deriving the error function with respect to each weight [26].
∀��,�������� =|∆������|=| ���� ��������| (4) �� : Error function; ������ : Weights of the neural network; ��,�� : Error indices
Our training dataset is now complete. A formalisation of the dataset is presented as shown in (3): �������������� ={([[������(����)] ]��′0 �������� ~(����)) �������������� ∈[0;������������ 1]} (3) ���� : Frequency of frame �� ; �������� : Number of frames treated; ���� : Grammar corresponding to the �� element 2.3. Creation of an intelligent speech recognition architecture In the last few years, the performance of deep learning algorithms has surpassed that of traditional machine learning algorithms. The most commonly used deep learning algorithms in the field of speech recognition are RNN and CNN [18] CNNs have many applications in video and image recognition and recommendation systems [18], [19]. Mathematically, a convolution is the combination of two functions to obtain a third function. The inputs are reduced to a form without loss of features, thus reducing the computational complexity and increasing the success rate of the algorithm [20] RNN are a family of neural networks specialised in processing sequential data.They can remember the input data received and predict precisely what will follow. Due to their nature, RNNs are successfully applied to sequential data such as time series, speech, video, and text [21] Through the use of a long and short term memory architecture, the RNN is able to access long term memory. Long short term memory (LSTM) RNNs are a sort of gated RNNs which provide the most efficient models used in practical applications and solve the long term dependency problem of RNNs [22]
The idea is to have a correspondence between the set of transformed speech and the set of words that we want our system to be able to identify, in particular: the expression "emergency" to trigger the exercise of the emergency situation and the expression "check" for the inter passage between two successive steps of the emergency management procedure (engine failure in our example). In addition, in order to avoid making the system reactive to noise, a third class has been added, grouping a number of noise patterns that may exist in the working environment of an ATC simulator. This set could only be completed by a set of "Labels" consisting of the images of the words corresponding to each speech via the tilde application ~. The established bijection, of the set of "Labels" of: i) emergency, ii) control, and iii) noise, is represented in (2): ~:{(1 0 0),(0 1 0)(0 0 1)}→{emergency,check,noise} (2)
ISSN: 2252 8938 Int J Artif Intell, Vol. 11, No. 3, September 2022: 986 994 990
The
do: �� = prediction
�� = Label
Int J Artif Intell ISSN: 2252 8938 Artificial intelligence in a communication system for air traffic … (Youssef Mnaoui) 991
Err = ��(��,��) For ��,�� indices of the
do: ������ = |∆������|=| ���� ��������| �� = [������]��,�� # weight correction matrix Correct weights () Next batch Figure
For
Thus, the network adjusts its weights after each data sample until a value closer to the ideal is obtained. This learning process is in fact the gradient descent algorithm which works as [27]: each batch of data model(batch) correct(batch) Err matrix 5. respective architectures of the CNN and RNN
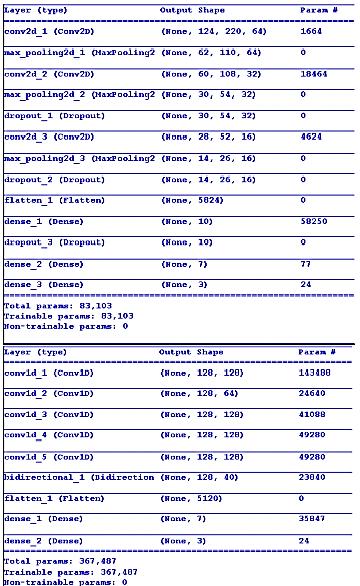
ISSN: 2252 8938 Int J Artif Intell, Vol. 11, No. 3, September 2022: 986 994 992
3.5
3. RESULTS AND DISCUSSIONS
Figure 6. Engine failure checklist [28]
6.33 7.550403020100 1 2 3 4 theofSteps checklist (x10) Repetition ScoreTotal
Figure 7. Performance monitoring
The different pattern in Figure 7 indicate the student's performance in the six steps of the engine failure management exercise The student's poor communication performance in the first two trials can be attributed to initial fear, use of incorrect aviation phraseology and unfamiliarity with the system. However, from the third repetition onwards, the student started to become familiar with the system and showed significantly better performance
The students find the ICS is an effective for developing the ability to produce correct aeronautical phraseology, even under stress. However, the assessment of nonverbal communication such as speaker's postures and voice was not possible. Some students stressed the relevance of nonverbal communication in the communication process. As there are few qualitative studies on the use of speech recognition to train soft skills, especially communication skills in the context of air traffic control, this study could add value to future research. However, one limitation of this study is that the speech recognition model will need even more data to achieve a higher accuracy value. Thus, our first recommendation for the future will be to spend much more time on data collection, data augmentation and data processing, in order to obtain a rich and high quality database. 4.5
Our system can be considered as a real learning environment for the development of communication competence, for two main reasons: firstly, the analysis of phraseology is instantaneous thanks to speech recognition and secondly, the integration of the temporal constraint of performance to allow the simulation of the temporal pressure present in an abnormal and emergency situation. The existence of such a model provides a safe and non threatening practice environment that tolerates trial and error. Most of the students did not perform well in communication in the first attempts: there were errors, disfluencies, hesitations in the messages conveyed and delays in communication. Some students reported feeling stressed at the beginning of the scenario because they had to use the correct phraseology and deal with an emergency situation at the same time. Figure 7 shows the average performance of a student for a single scenario repeated four times (engine failure in our example), in which they have to use correct aviation phraseology in a six step engine failure management checklist as illustrated in Figure 6 [28]
REFERENCES [1] D. Wiegmann, T. Faaborg, A. Boquet, C. Detwiler, and K. Holcomb, “Human error and general aviation accidents: a comprehensive, fine grained analysis using HFACS,” Washington, DC, 2005. [Online]. Available: https://commons.erau.edu/publication/1219.
[16] P. P. Kumar, K. Krishna, K. S. N. Vardhan, and K. S. Rama, “Performance evaluation of MLP for speech recognition in noisy environments using MFCC & wavelets,” International Journal of Computer Science & Communication (IJCSC), vol. 1, no. 2, pp. 42 45, 2010. [17] S. Chakroborty, A. Roy, and G. Saha, “Fusion of a complementary feature set with MFCC for improved closed set text independent speaker identification,” in 2006 IEEE International Conference on Industrial Technology, 2006, p. 387‑390, doi: 10.1109/icit.2006.372388.
[10] Y. Mnaoui, A. Najoua, and H. Ouajji, “Conception of a training system for emergency situation managers,” in 2020 1st International Conference on Innovative Research in Applied Science, Engineering and Technology (IRASET), Apr. 2020, pp. 1 4, doi: 10.1109/iraset48871.2020.9092222.
[12] S. A. A. Shah, A. ul Asar, and S. F. Shaukat, “Neural network solution for secure interactive voice response,” World Applied Sciences Journal, vol. 6, no. 9, pp. 1264 1269, 2009. [13] K. M. Ravikumar, R. Rajagopal, and H. C. Nagaraj, “An approach for objective assessment of stuttered speech using MFCC,” in The international congress for global science and technology, 2009, p. 19. [14] R. Kumar, R. Ranjan, S. K. Singh, R. Kala, A. Shukla, and R. Tiwari, “Multilingual speaker recognition using neural network,” in Proceedings of the Frontiers of Research on Speech and Music, 2009, pp. 1 8. [15] S. Narang and M. D. Gupta, “Speech feature extraction techniques: a review,” International Journal of Computer Science and Mobile Computing, vol. 4, no. 3, pp. 107 114, 2015.
[19] D. Rong, Y. Wang, and Q. Sun, “Video source forensics for iot devices based on convolutional neural networks,” Open Journal of Internet Of Things (OJIOT), vol. 7, no. 1, pp. 18 22, 2021. [20] Z. Zhang et al., “Downstream water level prediction of reservoir based on convolutional neural network and long short term memory network,” Journal of Water Resources Planning and Management, vol. 147, no. 9, p. 4021060, Sep. 2021, doi: 10.1061/(asce)wr.1943 5452.0001432. [21] L. Cai, K. Janowicz, G. Mai, B. Yan, and R. Zhu, “Traffic transformer: Capturing the continuity and periodicity of time series for traffic forecasting,” Transactions in GIS, vol. 24, no. 3, pp. 736 755, Jun. 2020, doi: 10.1111/tgis.12644.
[2] F. M. Amjad, A. Z. Sha’ameri, K. M. Yusof, and P. Eberechukwu, “Aircraft position estimation using angle of arrival of received radar signals,” Bulletin of Electrical Engineering and Informatics, vol. 9, no. 6, pp. 2380 2387, Dec. 2020, doi: 10.11591/eei.v9i6.2273.
[5] D. Schaefer, “Context sensitive speech recognition in the air traffic control simulation,” 2001.
[6] S. Singh and P. Singh, “High level speaker specific features modeling in automatic speaker recognition system,” International Journal of Electrical and Computer Engineering (IJECE), vol. 10, no. 2, pp. 1859 1867, Apr. 2020, doi: 10.11591/ijece.v10i2.pp1859 1867.
Int J Artif Intell ISSN: 2252 8938 Artificial intelligence in a communication system for air traffic … (Youssef Mnaoui) 993 4. CONCLUSION
[9] N. G. Çağin and Ö. Şenvar, “Anticipation in aviation safety management systems,” in YIRCoF’19, 2019, p. 9.
[23] J. Zhang, “Gradient descent based optimization algorithms for deep learning models training,” arXiv preprint, Mar. 2019, [Online]. Available: http://arxiv.org/abs/1903.03614.
[8] B. Al Braiki, S. Harous, N. Zaki, and F. Alnajjar, “Artificial intelligence in education and assessment methods,” Bulletin of Electrical Engineering and Informatics, vol. 9, no. 5, pp. 1998 2007, Oct. 2020, doi: 10.11591/eei.v9i5.1984.
ACKNOWLEDGEMENTS
We would like to thank the student air traffic controllers, without whom this research would not have been possible.
[11] R. Fowler, E. Matthews, J. Lynch, and J. Roberts, “Aviation English assessment and training,” Collegiate Aviation Review International, vol. 39, no. 2, p. 26, 2021, doi: 10.22488/okstate.22.100231.
[18] N. Sabharwal and A. Agrawal, “Neural networks for natural language processing,” in Hands on Question Answering Systems with BERT, Berkeley, CA: Apress, 2021, pp. 15 39.
[7] M. A. Anusuya and S. K. Katti, “Speech recognition by machine: A review,” International Journal of Computer Science and Information Security, vol. 6, no. 3, p. 25, Jan. 2009, [Online]. Available: http://arxiv.org/abs/1001.2267.
[22] R. F. Rojas, J. Romero, J. Lopez Aparicio, and K. L. Ou, “Pain assessment based on fNIRS using Bi LSTM RNNs,” in 2021 10th International IEEE/EMBS Conference on Neural Engineering (NER), May 2021, p. 399‑402, doi: 10.1109/ner49283.2021.9441384.
In order to ensure proper management of emergency situations, air traffic controllers must be prepared to deal with multiple information simultaneously by listening, understanding and using correct and standard aeronautical phraseology. Our study consists of proposing a ICS based on automatic speech recognition, allowing the interaction between the student and the machine without the need for a second student to perform the task of the pseudo pilot. In addition, the function of calculating the time taken to transmit instructions and clearances issued by student air controllers during emergency management was incorporated into the system. Through instant practice and repetition, the students were able to develop effective and efficient communication that facilitates emergency management. However, a limitation of this study is that the speech recognition model will still need more data. As the research is still in the development phase, future work is to develop a scalable training system that allows the injection of new scenarios.
[3] D. A. Wiegmann and S. A. Shappell, A human error approach to aviation accident analysis. London: Routledge, 2017. [4] “Effective pilot/controller communications,” in Flight Operations Briefing Notes, Human Performance, 2004, pp. 1 17.
Aouatif Najoua was born in Ouazzane, Morocco, on October 11, 1968. She is now a teacher at ENSET of various modules, such as Information Management, Administrative Management, Training Engineering, Educational Engineering. She holds a PhD since November 2012, in training engineering and didactics of science and technology, HDR in 2018 (Towards a method for evaluating science and technology education systems) from the Faculty of Sciences Ben M'sik, Hassan II University of Casablanca. Her field of research is the evaluation of science and technology teaching systems according to the semiotics of didactic environments. She is also a member of Cyber Physical Systems for pedagogical rehabilitation in special education (CyberSpeed) and a member of Association for the Development of Evaluation Methodologies in Education (ADMEE). She can be contacted at email: aouatif.najoua@gmail.com Hassan Ouajji was born in 1956 at Massa Agadir , Morocco. He is currently a Vice Director Research at the University Hassan II Casablanca, ENSET Institute. Since 2010, He is Head of the Electrical Systems Optimization Control and Management of Energy at the University Hassan II Casablanca, Electrical Engineering Mohammedia, Casablanca, Morocco. Since 1986, Full Time Faculty of Electrical Systems Optimization at the University Hassan II Casablanca, ENSET Institute. He is a Professor Researcher; his research is focused on Simulation, Electrical Systems, Plasma Physics, Control and Management of Energy. In 1986, Doctor of Engineering, Plasma Physics at the University Clermont Auvergne, Clermont Ferrand, Auvergne, France. He can be contacted at email: ouajji@hotmail.com.
ISSN: 2252 8938 Int J Artif Intell, Vol. 11, No. 3, September 2022: 986 994 994 [24] S. H. Haji and A. M. Abdulazeez, “Comparison of optimization techniques based on gradient descent algorithm: a review,” PalArch’s Journal of Archaeology of Egypt/Egyptology, vol. 18, no. 4, pp. 2715 2743, 2021. [25] C. Kim, K. Kim, and S. R. Indurthi, “Small energy masking for improved neural network training for end to end speech recognition,” in ICASSP 2020 2020 IEEE International Conference on Acoustics, Speech and Signal Processing (ICASSP), May 2020, p. 7684‑7688, doi: 10.1109/icassp40776.2020.9054382. [26] A. Mustapha, L. Mohamed, and K. Ali, “An overview of gradient descent algorithm optimization in machine learning: application in the ophthalmology field,” in Smart Applications and Data Analysis, Springer International Publishing, 2020, pp. 349 359 [27] S. Ruder, “An overview of gradient descent optimization algorithms,” arXiv preprint, Sep. 2016, doi: 10.48550/arXiv.1609.04747. [28] S. Malakis, T. Kontogiannis, and B. Kirwan, “Managing emergencies and abnormal situations in air traffic control (part I): Taskwork strategies,” Applied Ergonomics, vol. 41, no. 4, pp. 620 627, Jul. 2010, doi: 10.1016/j.apergo.2009.12.019.
BIOGRAPHIES OF AUTHORS Youssef Mnaoui was born in Mohammedia in 1980.He is a PhD student at the University Hassan II Casablanca, ENSET Institute since 2018 . Since 2004, he is an air traffic controller at the National Airports Office in Casablanca, Morocco. Since 2018, he is an Air Traffic Control Simulator Instructor at the International Civil Aviation Academy, Casablanca, Morocco. Since 2018, he is a teacher of the course Air Investigation and Accidents at the International Civil Aviation Academy, Casablanca, Morocco. His research is focused on Simulation, Technology teaching systems, Training System, Air Traffic Control Communication, Air Investigation and Accidents. He can be contacted at email: youssef.mnaoui etu@etu.univh2c.ma
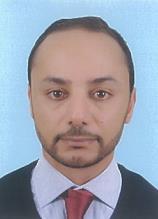



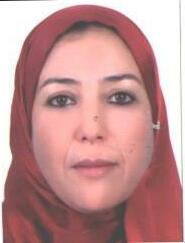



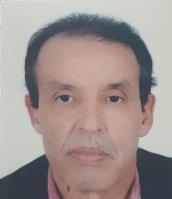



A crucial problem in deep learning and machine learning research areas is clustering. It is a technique that separates data points/samples into groups/clusters so that these data points/samples are more related to other data points/samples in the same groups/clusters than those in the other groups/clusters. Its applications are huge such as mobility pattern clustering [1], text clustering [2] [5], and customer segmentation [6] [9] It is worth to mention about the motivation of this one specific clustering problem, which is the text clustering problem of our company. In detail, we would like to partition Facebook users into their appropriate groups/clusters (i.e., depending on the contents that they are talking about). For example, there are groups of Facebook users talking about games, there are groups of Facebook users talking about music, and there are groups of Facebook users talking about religions.
Keywords:
Loc H. Tran1,2 , Nguyen Trinh1,2, Linh H. Tran1,2
The applications of this text clustering problem are huge such as: i) Our influencers/streamers can create the "appropriate" content fortheirfans/users; ii) This text clusteringproblem leadsto the implementation of the recommendation/matching systems (for example, fans/influencers, and fans/contents). We can employ the bi partite graph to implement these recommendation/matching systems (i.e., bi partite graph matching problem); and iii) Fake fans detection or anomaly/abnormal detection: for example, the fans/users belong to the group game, but they do not comment/feedback appropriately (i.e., they always talk about music)
Article history: Received Oct 4, 2021 Revised Jun 10, 2022 Accepted Jun 17, 2022 This paper constitutes the novel hypergraph convolutional neural network based clustering technique. This technique is employed to solve the clustering problem for the Citeseer dataset and the Cora dataset. Each dataset contains thefeaturematrix andtheincidencematrix ofthehypergraph (i.e., constructed from the feature matrix). This novel clustering method utilizes both matrices. Initially, the hypergraph auto encoders are employed to transform both the incidence matrix and the feature matrix from high dimensional space to low dimensional space. In the end, we apply the k means clustering technique to the transformed matrix. The hypergraph convolutional neural network (CNN) based clustering technique presented a better result on performance during experiments than those of the other classical clustering techniques.
Various clustering techniques are available from Python sklearn package [10] such as k means [1], hierarchical clustering technique, and affinity propagation. These techniques could be used to handle many clustering problems. For notation, however, they can only be applied to feature datasets. Regardless, in this paper, we only employ k means clustering technique as the "vanilla" or baseline technique because of three
NeuralHypergraphGraphClusteringAutoencodernetwork
Journal homepage: http://ijai.iaescore.com
1Department of Electronics, Ho Chi Minh City University of Technology (HCMUT), Ho Chi Minh City, Vietnam 2Department of Electronics, Vietnam National University Ho Chi Minh City (VNU HCM), Ho Chi Minh City, Vietnam Article Info ABSTRACT
IAES International Journal of Artificial Intelligence (IJ AI) Vol. 11, No. 3, September 2022, pp. 995 1003 ISSN: 2252 8938, DOI: 10.11591/ijai.v11.i3.pp995 1003 995
This is an open access article under the CC BY SA license. Corresponding Author: Linh H. DepartmentTranof Electronics, Ho Chi Minh City University of Technology Ho Chi Minh City, Vietnam Email: linhtran@hcmut.edu.vn
Hypergraph convolutional neural network-based clustering technique
1. INTRODUCTION
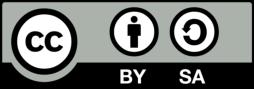
The low space complexity leads to less computational and training time of the classification/clustering techniques (i.e. the low time complexity).
2. GRAPH CONVOLUTIONAL NEURAL NETWORK BASED CLUSTERING TECHNIQUE 2.1. Problem formulation Given a set of samples {��1,��2, ,����}. n is the total number of samples. The pre defined number of clusters k. In detail, we have the adjacency matrix �� ∈����∗�� that: ������ ={1���������������������������������������������������������� 0����ℎ������������ (1)
ISSN: 2252 8938 Int J Artif Intell, Vol. 11, No. 3, September 2022: 995 1003 996main reasons: i) It's very simple to implement, ii) It scales to large datasets, and iii) It guarantees to converge to final solutions.Moreover, there are other clustering techniques (i.e. belong to different class of clustering techniques) that can also be employed to solve the clustering problem but can only be applied to network datasets such as spectral clustering [1], [11] [13], and maximum modularity approach [14] [20]. The weakness of these two classes of clustering techniques is obviously that they can only be applied to one type of dataset, which lead to information loss. About the case that we have both types of datasets such as the feature dataset and the network dataset, to assume that the samples in both datasets are the same, how can we apply the clustering technique to both datasets?Inthis study, we develop a novel clustering method. This method utilizes both the feature dataset and the network dataset. We discuss the details below. Initially, we employ the graph auto encoders proposed by Thomas Kipf [21] [25] to transform both the network dataset and the feature dataset from high dimensional space to low dimensional space. In the end, the k means clustering technique is applied to the transformed dataset. This novel clustering technique is called the graph convolutional neural network based clustering technique. There are some main advantages of this novel clustering technique such as: The memory/space required to store the data is lowered. This leads to the low space complexity.
The noises and redundant features are removed from the feature dataset and the network dataset. This leads to the high performance of the clustering technique. The claim is backed in section 4. Both network dataset and feature dataset are utilized. This leads to no information loss. However, there is oneweakness associated with the graph convolutional neural network (CNN) based clustering technique. In other words, assuming the pairwise relationships among the objects/entities/samples in this graph representation is not complete. Let's consider the case that we would like to partition/segment a set of articles into different topics [26], [27]. Initially, we employ the graph data structure to represent this dataset. The vertices of the graph are the articles. Two articles are connected by an edge (i.e., the pairwise relationship) if there is at least one author in common. Finally, we can apply clustering technique to this graph to partition/segment the vertices into groups/clusters.
Obviously, we ignore the information whether one specific author is the author of three or more articles (i.e., the co occurrence relationship or the high order relationship) in this graph data structure. This leads to information loss and eventually, poor performance (i.e., the low accuracy) of the clustering technique. To overcome this difficulty, we try to employ the hypergraph data structure to represent the above relational dataset. In detail, in this hypergraph data structure, the articles are the vertices, and the authors are the hyper edges. This hyper edge could connect more than two vertices (i.e., articles). Please note that if only the feature dataset is given, we need to construct the hypergraph from the feature dataset. The discussion on how to build the hypergraph from the feature vectors is presented in section 3.
In detail, initially, we develop the hypergraph auto encoders to transform both the hypergraph dataset (i.e., constructed from the feature dataset) and the feature dataset from high dimensional space to low dimensional space. In the end, the k means clustering technique is applied to the transformed dataset. This novel clustering technique is called the hypergraph CNN based clustering technique. For notation, these clustering techniques are un supervised learning techniques. Hence, in this paper, we do not need labeled datasets. The rest of the paper is organized as: section 2 defines the issue and presents the novel graph CNN based clustering technique. Section 3 presents the novel hypergraph CNN based clustering technique. Section 4 describes the Citeseer and the Cora dataset [28]. Then, section 4 compares the performance the hypergraph CNN based clustering technique and the performances of the graph CNN based clustering technique, the k means clustering technique, and the spectral clustering technique tested on these two Citeseer and Cora datasets. Section 5 is the conclusion.
Section 4 describes in detail the method of building the similarity graph from feature vectors. In the end, we get the similarity graph. This graph is represented by the adjacency matrix A Please note that this phase is required for spectral clustering techniques and graph CNN based clustering techniques if only the feature matrix is provided. ������ ={ 1���������������������������������������������������������� 0���������������������������������������������������������������� (3) 2.3 Graph convolutional neural network based clustering technique At present, the set of feature vectors {��1,��2, ,����} and the relationships among the samples (represented by the adjacency matrix A) in the dataset are available. For notation, ���� ∈��1∗��1,1≤�� ≤�� and �� ∈����∗�� We have �� ̂ =��+��, with I as the identity matrix. With �� ̂ as the diagonal degree matrix of �� ̂ , we have �� ̂ ���� =∑ �� ̂ ������ The result output (i.e., the embedding matrix) Z of the graph CNN could be defined as (4): �� =�� ̂ 1 2�� ̂ �� ̂ 1 2��������(�� ̂ 1 2�� ̂ �� ̂ 1 2����1)��2 (4) For notation, X is the input feature matrix and �� ∈����∗��1 ��1 ∈����1∗��2 and ��2 ∈����2∗�� are two parameter matrices to be learned during the training process. For notation, D is the dimensions of the embedding matrix Z.Next, we need to reconstruct the adjacency matrix �� from Z. We obtain the reconstruction ��′ representing the similarity graph using (5) ��′ =��������������(������) (5) The Rectified Linear Unit or the The ReLU operation is defined in (6): ��������(��)=max(0,��) (6) A sigmoid function can be defined in (7): ��������������(��)= 1 1+�� �� (7) For this graph auto encoder model, we need to evaluate the cross entropy error over all samples in the dataset: �� = 1 ��2 ∑ ∑ ������ ln(��������������(�������� ��))�� ��=1 �� ��=1 +(1 ������)(1 ������ ln(��������������(�������� ��)) (8) Please note that ���� is the row i vector of the embedding matrix Z and ���� ∈��1∗�� We train the two parameter matrices ��1 ∈����1∗��2 and ��2 ∈����2∗�� using the gradient descent method. Then, partition the samples (����)��=1, ,�� in ��1∗�� with the k means algorithm into k clusters/groups. In general, the graph CNN based clustering technique can be shown in Figure 1
Int J Artif Intell ISSN: 2252 8938
2.2 Adjacency matrix is not provided Suppose that we are given the feature matrix �� ∈����∗��1 but not the adjacency matrix �� ∈����∗��. In this case, the similarity graph could be constructed from these feature vectors using the k nearest neighbor (KNN) graph. To put it another way, sample i connects with sample j by an edge in a no direction: un directed graph if sample i is among the KNN of sample j or sample j is among the KNN of sample i.
2.4. Discussions about graph convolutional neural network based clustering technique From the section 2.3 , unlike other clustering techniques such as k means, we easily see that this proposed clustering technique (i.e., the graph CNN based clustering technique) utilizes both the feature dataset and the network dataset. This is a very strong argument of this proposed technique. Since no information are lost, the performance of this novel clustering technique is expected to be higher than the performance of the other classic clustering techniques such as k means. The claim is backed in section 4.
Hypergraph convolutional neural network based clustering technique (Loc H. Tran) 997 and the feature matrix �� ∈����∗��1 where ��1 is the dimensions of the feature vectors. Our objective is to output the clusters/groups ��1,��2, ,���� so that: ���� ={��|1≤��≤��������������������������������������������} (2)
Suppose
������ ={ 1������������������������������������ℎ������������������ 0������������������������������������������������ℎ������������������ (10) For
��
However, there is one major weakness of this proposed clustering technique. When new samples arrive, wecannotpredict which clusters these samplesbelong to(unlikek means clusteringtechnique).In other words, we have to update the adjacency matrix, and we have to re train our graph auto encoder. Thus, this technique can only be considered as the offline clustering technique although its performance is a lot higher than the performance of the other online clustering techniques. Figure 1. The graph convolutional neural network based clustering technique CONVOLUTIONAL NEURAL NETWORK BASED CLUSTERING Problem formulation Given a set of samples {��1,��2, ,����} k is the pre defined number of clusters. n is the total number of samples. In details, we are given the incidence matrix �� ∈����∗�� where: (9) and the feature matrix �� ∈����∗��1 where ��1 is the dimensions of the feature vectors. Our objective is to output the clusters/groups ��1,��2, ,���� where ���� ={��|1≤�� Incidence matrix of the hypergraph is not provided that we are given the feature matrix ∈�� 1 but not the incidence matrix �� ∈�� . In this case, using the KNN graph, we can create the incidence matrix H from these feature vectors. Particularly, sample i belongs to hyperedge j if sample i is among the KNN of sample j or sample j is among the KNN of sample i We have k set tobe 5in this paper. In the end, the incidence matrix H which represents the hypergraph is obtained. notation, this phase is required for hypergraph CNN based clustering techniques if only the feature matrix is provided.
TECHNIQUE 3.1.
3. HYPERGRAPH
≤��������������������������������������������} 3.2.
��∗��
��∗��
.
������ ={1������������������������������������ℎ������������������ 0����ℎ������������
ISSN: 2252 8938 Int J Artif Intell, Vol. 11, No. 3, September 2022: 995 1003 998
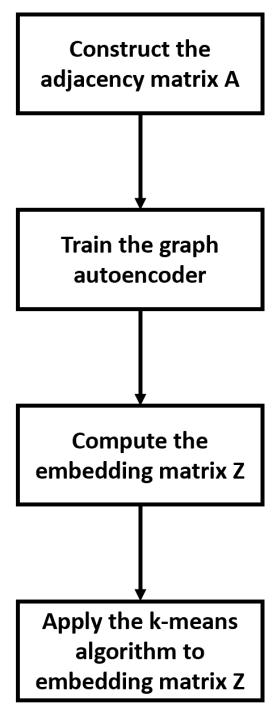
We already have the incidence matrix H of the hypergraph and the set of feature vectors {��1,��2, ,����} For notation, ���� ∈��1∗��1,1≤�� ≤�� and �� ∈����∗�� With w(e) as the weight of the hyper edge e, and the ����∗�� diagonal matrix that contains the weights of all hyper edges in its diagonal entries as W From the weight matrix W and the incidence matrix H, the degree of vertex v and the degree of hyper edge e can be defined in: ��(��)=∑��(��)∗ℎ(��,��) ��∈�� ��(��) =∑ℎ(��,��) ��∈�� Let the two diagonal matrices that contains the degrees of vertices and the degrees of hyper edges in their diagonal entries be ���� ����������, respectively. For additional notation, ���� is the ����∗�� matrix and ���� is the ����∗�� matrix. The final output (i.e., the embedding matrix) Z of the hypergraph CNN is defined in (11): �� =���� 1 2�������� 1�������� 1 2��������(���� 1 2�������� 1�������� 1 2����1)��2 (11) For notation, X is the input feature matrix and �� ∈����∗��1 ��1 ∈����1∗��2 and ��2 ∈����2∗�� are the two parameter matrices to be learned during the training process. For notation, the dimensions of the embedding matrix Z is D Next, we need to reconstruct the incidence matrix �� from Z. We obtain the reconstruction ��′ representing the hypergraph using (12) ��′ =��������������(������) (12)
Int J Artif Intell ISSN: 2252 8938 Hypergraph convolutional neural network based clustering technique (Loc H. Tran) 999 3.3. Hypergraph convolutional neural network based clustering technique
From the section 3.3, unlike the graph CNN based clustering techniques, instead of employing the pairwise relationships among objects/entities/samples, we easily see that this hypergraph CNN based clustering technique employs the high order relationship among objects/entities/samples. This leads to no information loss. Hence, this hypergraph CNN based clustering technique is expected to perform better than that of the graph CNN based clustering technique. The claim is backed in section 4. However, like the graph CNN based clustering technique, this hypergraph CNN based clustering technique is the offline clustering technique. In other words, when new samples arrive, we have to update the incidence matrix of the hypergraph, and we have to re train our hypergraph auto encoder.
The Rectified Linear Unit and the ReLU operation is defined in (13): ��������(��)=max(0,��) (13) A sigmoid function could be defined in (14): ��������������(��)= 1 1+�� �� (14) In the case of this hypergraph auto encoder model, we need to evaluate the cross entropy error over all samples in the dataset: �� = 1 ��2 ∑ ∑ ������ ln(��������������(�������� ��))�� ��=1 �� ��=1 +(1 ������)(1 ������ ln(��������������(�������� ��)) (15) Please note that ���� is the row i vector of the embedding matrix Z and ���� ∈��1∗�� We train the two parameter matrices ��1 ∈����1∗��2 and ��2 ∈����2∗�� using the gradient descent method. Then, partition the samples (����)��=1, ,�� in ��1∗�� with the k means algorithm into k clusters/groups. In general, the hypergraph CNN based clustering technique can be shown in Figure 2. 3.4. Discussion about hypergraph convolutional neural network based clustering technique
ISSN: 2252 8938 Int J Artif Intell, Vol. 11, No. 3, September 2022: 995 1003 1000 Figure 2. The hypergraph convolutional neural network based clustering technique
4. EXPERIMENTS AND RESULT
There are two publicly available dataset, the Citeseer dataset and the Cora dataset. We use them to test our novel clustering technique. For example, we test the graph CNN based clustering technique.
Please note that in the case that the adjacency matrices are not given, the similarity graph need to be built from the feature vectors of the datasets in the following ways: i) The fully connected graph: Connect all samples; ii) The �� neighborhood graph: All the samples whose pairwise distances are smaller than �� are connected; and iii) KNN graph: sample i connects with sample j by an edge in a no direction: un directed graph if sample i is among the k nearest neighbors of sample j or sample j is among the KNN of sample i. The KNN graph is used to build the similarity graph from feature vectors of the Cora dataset and the Citeseer dataset. For notation, we have k set as 5. Finally, the way showing how to construct the hypergraph (i.e., the incidence matrix H) from the feature vectors is discussed in detail in section 3. Please note that D, the dimensions of the embedding matrix Z, is set to be 16. 4.2. Experiment results We compare the performance of the hypergraph CNN based clustering technique with the performances of the graph CNN based clustering technique, the k means clustering technique, the spectral clustering technique for feature datasets, the spectral clustering technique for network datasets. Our model is tested (Python code) with NVIDIA Tesla K80 GPU (12 GB RAM option) on Google Colab. There are three performances of clustering techniques that we are going to employ in this paper which are: i) Silhouette coefficient, ii) Davies Bouldin score, and iii) Calinski Harabasz score
Cora: This dataset contains 2,708 scientific papers (i.e., nodes of the graph) linked by 5,429 edges representing one scientific paper citing to another. Individually, every paper (i.e., node of the graph) has a binary vector that describes the absence or presence of 1,433 unique words. This binary vector is called the feature vector of the scientific paper. The goal of the experiment is to classify each scientific paper into 7 categories which are Reinforce_Learning, Theory, Rule_Learning, Case_Based, Genetic_Algorithms, Probabilistic_Methods, Neural_Networks, and Theory.
Citeseer: The dataset contains 3,312 scientific papers (i.e., nodes of the graph) linked by 4,732 edges representing one scientific paper citing to another. Individually, every paper (i.e., node of the graph) has a binary vector that describes the absence or presence of 3,703 unique words. This binary vector is called the feature vector of the scientific paper. The goal of the experiment is to classify each scientific paper into one of 6 categories: Human computer interaction (HCI), machine learning (ML), database (DB), information retrieval (IR), artificial intelligence (AI), ML and Agents.
4.1. Preliminaries on dataset
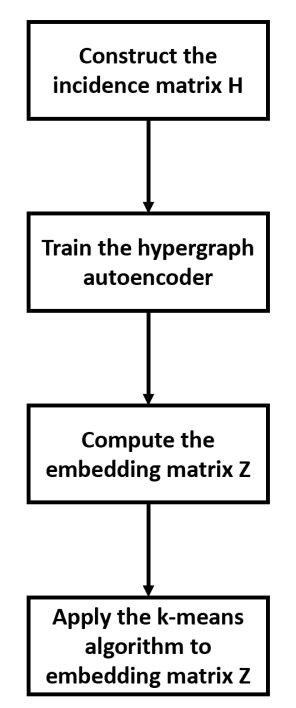
Each sample defines its Silhouette coefficient. The performance consists of two score: i) a: In the same groups/clusters, the mean distance between the sample and all other points/samples; and ii) b: In the next nearest cluster, the mean distance between the sample and all other points/samples. Then for a single sample, the Silhouette coefficient s could be computed in (16): �� = �� �� max(��,��) (16)
The hypergraph convolutional neural network based clustering technique 0.5034 0.4786 40369.4615
Table 1. Comparison of graph CNN based clustering technique with other techniques with the Citeseer dataset coefficientSilhouette DaviesscoreBouldin CalinskiscoreHarabasz
Int J Artif Intell ISSN: 2252 8938
The graph convolutional neural network based clustering technique 0.1203 2.0923 231.2733 The k means clustering technique 0.0007 7.3090 15.2688
Table 2. Comparison of graph CNN based clustering technique with other techniques with the Cora dataset coefficientSilhouette DaviesscoreBouldin CalinskiscoreHarabasz
The hypergraph convolutional neural network based clustering technique 0.3349 1.0471 1288.7860
The Silhouette coefficient for all samples is the silhouette coefficient of the dataset. From (16), we easily recognize that the higher the silhouette coefficient, the better the clustering results. The average similarity measure of each cluster with its most similar cluster, where the ratio of within cluster distances to between cluster distances is the similarity, is called The Davies Bouldin score. For that reason, clusters that are less dispersed and farther apart generate a better score. For notation, zero is the minimum score. From (16), we easily see that the lower the Davies Bouldin score, the better the clustering results. The variance ratio criterion or also called the Calinski Harabasz score is defined as the ratio of the sum of inter cluster dispersion for all clusters and between clusters dispersion. From the definition, we easily see that the higher the Calinski Harabasz score, the better the clustering results. For the Citeseer dataset, Table 1 presents the performances of the graph CNN based clustering technique, the k means clustering technique, the spectral clustering technique for feature vectors, the spectral clustering technique for adjacency matrix.
The spectral clustering technique for feature vectors 0.0047 5.9004 4.1290
The spectral clustering technique for feature vectors 0.0196 6.6584 20.4136
Hypergraph convolutional neural network based clustering technique (Loc H. Tran) 1001
Moreover, the hypergraph CNN based clustering technique is better than the CNN based clustering technique since the hypergraph data structure employs the high order relationships among the samples/entities/objects. This leads to no loss of information. However, when new samples arrive, for the (hyper) graph CNN based clustering techniques, we cannot predict which clusters these samples belong to. That is to say, we have to update the adjacency matrix or the incidence matrix, re train our (hyper) graph auto encoder, re compute the embedding matrix, and apply k means clustering technique to this embedding matrix again to get the final clustering result. Hence the (hyper) graph CNN based clustering technique can only be considered as the offline clustering technique.
Last but not least, please note that when new samples/data points arrive, weneedtore trainourmodel. For industrial projects, this novel model can be updated/re trained twice per month or once per month. Let’s
For the Cora dataset, Table 2 presents the performances many techniques. There is the graph CNN basedclusteringtechnique,thek meansclusteringtechnique,thespectralclusteringtechniqueforfeature vectors. There is also the spectral clustering technique for adjacency matrix.
The spectral clustering technique for adjacency matrix 0.0465 8.9685 2.0282
4.3 Discussions
The k means clustering technique 0.0149 5.5878 21.5432
With the results from the Tables 1 and 2, we acknowledge that the graph CNN based clustering technique is superior than the k means clustering technique, the spectral clustering technique for feature vectors, the spectral clustering technique for adjacency matrix since graph CNN based clustering technique utilize both the information from the feature vectors and the adjacency matrix of the dataset and noises and redundant features in the dataset (i.e., both in the feature vectors and the adjacency matrix) are removed.
The spectral clustering technique for adjacency matrix 0.0081 14.8230 2.1392
The graph convolutional neural network based clustering technique 0.1963 1.7312 322.3034
We would like to thank Ho Chi Minh City University of Technology (HCMUT), VNU HCM for the support of time and facilities for this study.
ISSN: 2252 8938 Int J Artif Intell, Vol. 11, No. 3, September 2022: 995 1003 1002consider the case when a lot of samples arrive, this event introduces a lot of novel patterns in the whole dataset. Model updating is a must. In the other words, we have to update all the models such as the hypergraph CNN based clustering technique, the graph CNN based clustering technique, the k means clustering technique, the spectral clustering technique for feature vectors, and the spectral clustering technique for adjacency matrix.
[11] U. von Luxburg, “A tutorial on spectral clustering,” Statistics and Computing, vol. 17, no. 4, pp. 395 416, Aug. 2007, doi: 10.1007/s11222 007 9033 z.
ACKNOWLEDGEMENTS
[9] J. J. Jonker, N. Piersma, and D. Van den Poel, “Joint optimization of customer segmentation and marketing policy to maximize long termprofitability,” ExpertSystems withApplications,vol.27,no.2,pp.159 168,Aug.2004,doi:10.1016/j.eswa.2004.01.010.
The main contributions of our paper are to develop the novel graph CNN based clustering technique and the novel hypergraph CNN based clustering technique. We then apply these novel clustering techniques to two Citeseer and Cora datasets and compare the performance of the hypergraph CNN based clustering technique with that of the graph CNN based clustering technique, the k means clustering technique, the spectral clustering technique for feature vectors, the spectral clustering technique for adjacency matrix. In this paper, we presented the (hyper) graph CNN based clustering technique to handle the clustering problem. The work is presumably, not complete. Many types of (hyper) graph CNN are available. In future research, the (hyper) graph CNN with/without attention could also be used to solve this clustering problem.
[6] C. Marcus, “A practical yet meaningful approach to customer segmentation,” Journal of Consumer Marketing, vol. 15, no. 5, pp. 494 504, Oct. 1998, doi: 10.1108/07363769810235974.
[20] S. Bhowmick and S. Srinivasan, “A template for parallelizing the louvain method for modularity maximization,” in Dynamics On and Of Complex Networks, Volume 2, Springer New York, 2013, pp. 111 124.
REFERENCES [1] L. H. Tran and L. H. Tran, “Mobility patterns based clustering: A novel approach,” International Journal of Machine Learning and Computing, vol. 8, no. 4, 2018, doi: 10.18178/ijmlc.2018.8.4.717. [2] J. Yi, Y. Zhang, X. Zhao, and J. Wan, “A novel text clustering approach using deep learning vocabulary network’,” Mathematical Problems in Engineering, vol. 2017, pp. 1 13, 2017, doi: 10.1155/2017/8310934.
5. CONCLUSION
[3] A. Hadifar, L. Sterckx, T. Demeester, and C. Develder, “A self training approach for short text clustering,” in Proceedings of the 4th Workshop on Representation Learning for NLP (RepL4NLP 2019), 2019, pp. 194 199, doi: 10.18653/v1/w19 4322.
[7] K. Tsiptsis and A. Chorianopoulos, Data mining techniques in CRM: inside customer segmentation. John Wiley $ Sons, Ltd, 2011.
[17] S. Wang and R. Koopman, “Clustering articles based on semantic similarity,” Scientometrics, vol. 111, no. 2, pp. 1017 1031, Feb. 2017, doi: 10.1007/s11192 017 2298 x. [18] S. Ghosh et al., “Distributed louvain algorithm for graph community detection,” in 2018 IEEE International Parallel and Distributed Processing Symposium (IPDPS), May 2018, pp. 885 895, doi: 10.1109/ipdps.2018.00098. [19] S. Emmons, S. Kobourov, M. Gallant, and K. Börner, “Analysis of network clustering algorithms and cluster quality metrics at scale,” PLOS ONE, vol. 11, no. 7, p. e0159161, Jul. 2016, doi: 10.1371/journal.pone.0159161.
[4] Y. Liu and X. Wen, “A short text clustering method based on deep neural network model’,” Journal of Computers, vol. 29, no. 6, pp. 90 95, 2018. [5] Z. Dai, K. Li, H. Li, and X. Li, “An unsupervised learning short text clustering method,” Journal of Physics: Conference Series, vol. 1650, no. 3, p. 32090, Oct. 2020, doi: 10.1088/1742 6596/1650/3/032090.
[12] A. Ng, M. Jordan, and Y. Weiss, “On spectral clustering: Analysis and an algorithm,” Advances in neural information processing systems, vol. 14, 2001. [13] L. H. Tran, L. H. Tran, and H. Trang, “Un normlized and Random Walk Hypergraph Laplacian Un supervised Learning,” in International Conference on Nature of Computation and Communication, Springer International Publishing, 2014, pp. 254 263. [14] D. Combe, C. Largeron, M. Géry, and E. Egyed Zsigmond, “I louvain: An attributed graph clustering method,” in International Symposium on Intelligent Data Analysis, Springer International Publishing, 2015, pp. 181 192. [15] P. Held, B. Krause, and R. Kruse, “Dynamic clustering in social networks using louvain and infomap method,” in 2016 Third European Network Intelligence Conference ({ENIC}), Sep. 2016, pp. 61 68, doi: 10.1109/enic.2016.017. [16] D. L. Sánchez, J. Revuelta, F. De la Prieta, A. B. Gil González, and C. Dang, “Twitter user clustering based on their preferences and the Louvain algorithm,” in International Conference on Practical Applications of Agents and Multi Agent Systems, Springer International Publishing, 2016, pp. 349 356.
[10] Scikit learn. "2.3. Clustering " scikit learn.org. https://scikit learn.org/stable/modules/clustering.html (accessed Jun. 1, 2022).
[8] J. Wu and Z. Lin, “Research on customer segmentation model by clustering,” in Proceedings of the 7th international conference on Electronic commerce ICEC05, 2005, pp. 316 318, doi: 10.1145/1089551.1089610.
[21] S. Pan, R. Hu, G. Long, J. Jiang, L. Yao, and C. Zhang, “Adversarially regularized graph autoencoder for graph embedding,” Jul. 2018, doi: 10.24963/ijcai.2018/362. [22] C. Wang, S. Pan, G. Long, X. Zhu, and J. Jiang, “Mgae: Marginalized graph autoencoder for graph clustering,” in Proceedings of the 2017 ACM on Conference on Information and Knowledge Management, Nov. 2017, pp. 889 898, doi: 10.1145/3132847.3132967.
Loc H. Tran completed his Bachelor of Science and Master of Science in Computer Science at University of Minnesota in 2003 and 2012 respectively. Currently, he’s a researcher at John von Neumann Institute, Vietnam. His research interests include spectral hypergraph theory, deep learning. He can be contacted at email: tran0398@umn.edu.
[28] LINQS "Datasets." linqs.soe.ucsc.edu https://linqs.soe.ucsc.edu/data (accessed Jun. 1, 2022).
Nguyen Trinh received the B.S. and M.S. degree in Electronics Engineering from Ho Chi Minh City University of Technology (HCMUT), Vietnam (2019, 2021). He is also working as lecturer at Faculty of Electrical Electronics Engineering, Ho Chi Minh City University of Technology VNU HCM. He can be contacted at email: nguyentvd@hcmut.edu.vn
[27] D. Zhou, J. Huang, and B. Schölkopf, “Learning with hypergraphs: Clustering, classification, and embedding,” Advances in Neural Information Processing Systems 19, vol. 19, 2006.
[26] D. Zhou, J. Huang, and B. Scholkopf, “Beyond pairwise classification and clustering using hypergraphs,” 2005.
BIOGRAPHIES OF AUTHORS
[23] S. Fan, X. Wang, C. Shi, E. Lu, K. Lin, and B. Wang, “One2multi graph autoencoder for multi view graph clustering,” in Proceedings of The Web Conference 2020, Apr. 2020, pp. 3070 3076, doi: 10.1145/3366423.3380079.
[25] L. H. Tran and L. H. Tran, “Applications of (SPARSE) PCA and LAPLACIAN EIGENMAPS to biological network inference problem using gene expression data,” International Journal of Advances in Soft Computing and its Applications,vol. 9, no. 2, 2017.
Linh H. Tran received the B.S. degree in Electrical and Computer Engineering from University of Illinois, Urbana Champaign (2005), M.S. and PhD. in Computer Engineering from Portland StateUniversity (2006, 2015). Currently, he is working as lecturer at Faculty of Electrical Electronics Engineering, Ho Chi Minh City University of Technology VNU HCM. His research interests include quantum/reversible logic synthesis, computer architecture, hardware software co design, efficient algorithms and hardware design targeting FPGAs and deep learning. He can be contacted at email: linhtran@hcmut.edu.vn.
Int J Artif Intell ISSN: 2252 8938 Hypergraph convolutional neural network based clustering technique (Loc H. Tran) 1003
[24] L. Tran, A. Mai, T. Quan, and L. Tran, “Weighted un normalized hypergraph Laplacian eigenmaps for classification problems,” International Journal of Advances in Soft Computing and its Applications, vol. 10, no. 3, 2018.
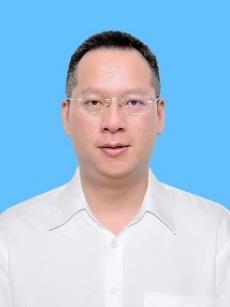



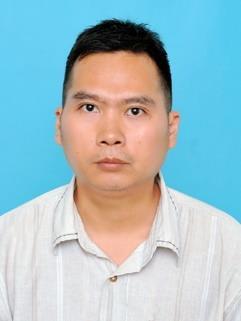



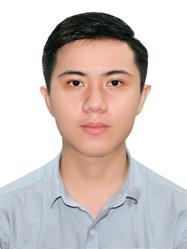



Keywords: Deep PowerNeuralForecastingEnsemblelearningmethodnetworksconsumption
Article history: Received Sep 5, 2021 Revised Apr 20, 2022 Accepted May 19, 2022
IAES International Journal of Artificial Intelligence (IJ AI) Vol. 11, No. 3, September 2022, pp. 1004 1018 ISSN: 2252 8938, DOI: 10.11591/ijai.v11.i3.pp1004 1018 1004
Smart power forecasting enables energy conservation and resource planning. Power estimation through previous utility bills is being replaced with machine intelligence. In this paper, a neural network architecture for demand side power consumption forecasting, called SGtechNet, is proposed. The forecast model applies ConvLSTM encoder decoder algorithm designed to enhance the quality of spatial encodings in the input feature to make a 7 day forecast. A weighted average ensemble approach was used, where multiple models were trained but only allow each model’s contribution to the prediction to be weighted proportionally to their level of trust and estimated performance. This model is most suitable for low powered devices with low processing and storage capabilities like smartphones, tablets and iPads. The power consumption comparison between a manually operated home and a smart home was investigated and the model’ s performance was tested on a time domain household power consumption dataset and further validated using a real time load profile collated from the School of Renewable Energy and Smart Grid Technology, Naresuan University Smart Office An improved root mean square error (RMSE) of 358 kwh was achieved when validated with holdout validation data from the automated office. Overall performance error, forecast and computational time showed a significant improvement over published research efforts identified in a literature review.
Journal homepage: http://ijai.iaescore.com
This is an open access article under the CC BY SA license. Corresponding Author: Yodthong Mensin School of Renewable Energy and Smart Grid Technology (SGtech) Naresuan University, 99 Moo 9. Tha Pho Sub district, Muang District, Phitsanulok 65000, Thailand Email: yodthongm@nu.ac.th 1. INTRODUCTION
Smart power consumption forecast model with optimized weighted average ensemble
Article Info ABSTRACT
Reliable forecasts enable tracking the loads relative to proper balancing and creation of a dynamic energy pricing model and trading opportunities for energy users, using the knowledge of their anticipated power needs. Load forecasting is very useful in scheduling of devices [1], and energy trading that is becoming the centerpiece of a developing energy revolution. To analyze power consumption trends and to characterize patterns and develop forecasts, various statistical and traditional methods [2], [3] are used. However, modeling a complex real world problem, such as power forecasting, with statistical linear models like autoregressive model (AR), autoregressive moving average (ARMA), autoregressive integrated moving average (ARIMA), and seasonal autoregressive integrated moving average (SARIMA), is often difficult. These types of models cannot determine non linear relationships in complex data, such as power consumption data with stochastic nature, therefore complex models, perhaps based on machine intelligence like neural networks, provide the analysis leverage necessary. Statistical tools from some industrial players like Prophet [4] from Facebook and Uber [5] that won the M4 Competition achieved some level of success
Alexander N. Ndife1, Wattanapong Rakwichian1, Paisarn Muneesawang2 , Yodthong Mensin1 1School of Renewable Energy and Smart Grid Technology, Naresuan University, Phitsanulok, Thailand 2Department of Electrical and Computer Engineering, Naresuan University, Phitsanulok, Thailand
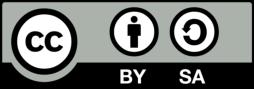
2. THE NETWORK ARCHITECTURE
Our proposed architecture as illustrated in Figure 1 is centered on optimizing neural networks learning process and mitigating its inherent challenges while achieving state of the art forecast model. A weighted average ensemble method using multiple models with similar configurations, but different initial random weights is proposed. Those various models were trained on 3 different datasets including two load demand datasets from a household in France and the one from the smart office of SGtech, Naresuan University Thailand. However, combining predictions from multiple models can also add a bias that can make the model less sensitive to specifics in the training data, choice of training scheme and the serendipity of single training. It has been observed over time that ensemble methods, if not properly checked, might not ensure that the best performing set of weights are used as a final model. So, our proposed method performed weighted average ensemble [8] as one of the ways of achieving a model ensemble in neural networks like voting [9] and stacking [10] and snapshot or checkpoint [11], among others, in a unique way. Here, instead of allowing equal contribution of all the models to the final prediction model, contributions were dependent on
Smart power consumption forecast model with optimized weighted … (Alexander N. Ndife) 1005 because of the methodology likened to the use of dropout and its invariants in approximating a well known probabilistic model, the gaussian process in neural networks In contrast to statistical modelling, neural network models formulate a model based on features learned from existing data and this dependency makes them data driven and self adaptive, essential aspects for time series forecasting and where Big Data is involved. Although neural networks are preferable in most time series problems, they are not without their limitations. Problem of large number of trainable parameters sometimes makes neural networks models unimplementable in low processing devices. For an instance, AlexNet, which won the 2012 ImageNet challenge, has about 60 million trainable parameters and VGGNet has a huge 138 million parameters. Although there has been continuous effort towards trainable set size reduction and overall performance optimization, more efforts are still needed. For instance, SqueezeNet was able to reduce its trainable parameters to 1 2 million while achieving a reasonable performance. These model size reduction efforts are important because real world problems, including power consumption forecasting, requires real time and on device processing It is not enough to have an accurate prediction model without ability to operate on resource constrained low power edge device without latency problem. Experimentally illustrated facts have shown that the model size affects its inference time [6], so the smaller the model size the faster the computational speed More recently, neural network methods have become very popular in time series forecasting due to the high performance achieved. Implementations in the form of deep learning algorithms have also become a turning point for both classification and regression tasks which, hitherto, have been difficult even on computers with excellent performance. Applying neural networks solution usually require training of large amounts of data to realize an appropriate machine learning model that can effectively be used in making projections. Given this, the model size obtained is normally big, requiring lengthy processing time. Therefore, a model compression technique is necessary to reduce the size and to expedite the computational process. Importantly, learning the arbitrary complex mapping from inputs to outputs has become the focus of research from which significant performance improvements have been achieved. However, a huge gap still exists between the methods of deployment and the implementation environment. Some of these gaps include a means to: capture the dominant factors in the data that need to be learned, as well as reducing the size of the model, increasing its inference time, and the selection of the model’s parameters. These are the major areas that the proposed forecast model, discussed in this paper, aims to optimize.
Complex models based on deep learning, such as SGtechNet proposed in this paper, stand a better chance of addressing most of the noted difficulties of a complex real world problem like power forecasting. It is intended that this model will be implemented in a low powered low memory on device mobile system, enabling smartphones to be used for demand side energy management and control It has been observed that the availability of high speed graphics processing units (GPUs) in labs gives greater performance for models with larger trainable parameters, but these models are unusable in many real world applications especially when implemented on resource constrained devices Achieving a lightweight model with a very high confidence in the predictions, was a major objective of our work. Based on this, an ensemble method together with advanced feature representation was used in combination with other improvement methods such as the layer compression technique to leverage improved forecast results. Many methods have yielded good model performance results but, in our work, we are more concerned on the scalable methods capable of optimizing the model training for quick convergence. aggregated deeep belief networks (DBNs) outputs using the support vector machine (SVM) algorithm, reported in [7], outperformed benchmark methods such as support vector regression (SVR), feedforward neural networks (FFNN), DBN and ensemble FFNN The model compression algorithm implemented in the current work addresses the challenges of cost, power, heat and other related issues, all of which will be elaborated in the methodology discussion
Int J Artif Intell ISSN: 2252 8938
A description of time series modeling methods used by deep neural networks, for power consumption forecasting, has been introduced previously, together with discussion of the various methods identified in the literature The organization of this paper includes, in section 2, related work, then the
Aside from model improvement, the design for SGtechNet feature learning made it adaptable to different datasets including augmented power consumption dataset [12] from an automated office in such a way that it detected and analyzed the atmospheric climate changes. In our development process we considered the weather conditions all year round. To ensure that the real time power consumption data used for both augmentation and validation of the model’s performance captures this fact, we juxtaposed the power generation capacity of test environment Thailand on the load factors based on urban and rural characterization discussed in [13] to test if climate changes have any effect on the characteristics of household electricity consumption. Load factors, seasonal factors, and utilizations factor are some of the usage characteristics relevant to the power consumption of air conditioners, fans, refrigerators, water heaters and even washing machines and clothes driers. For example, especially in the case of the latter three domestic appliances, heating water or drying laundry may not in fact be necessary in a climate such as is experienced in Thailand, whereas it could be a significant use of power in cold climates. Table 1 describes Thailand’s 2020 power statistics showing the monthly power generation capacity and load factors.
Table 1. Thailand power statistics 2020 [14] Jan. Feb. Mar. Apr. May Jun. Jul. Aug. Sep. Oct. Nov. Dec. GENERATION(GWh) 16,138 15,477 17,618 15,715 16,899 15,887 16,390 16,348 16,195 15,457 15,292 14,483 FACTORLOAD(%) 79.1 82.0 82.7 78.7 80.2 81.0 82.0 80.7 82.8 79.5 77.4 75.1
Juxtaposing the generation capacity with the load demand as illustrated in Figure 2, sourced from [14], showed that the load factor surpassed generation capacity in March and September. This indicated the need to ensure that the validation data for the proposed model was tested on across the different seasons of the year Also, the result of the preliminary analysis of weekday power consumption and generation/load demand discrepancy shown in Figure 3, emanating from data from the smart office that was used for the validation of the proposed model, showed the daily power consumption characteristics. These daily characteristics proved useful in determining the performance of the model.
ISSN: 2252 8938 Int J Artif Intell, Vol. 11, No. 3, September 2022: 1004 1018 1006thelevel of trust and estimated performance, to ensure the performance of poorly performed models do not affect the overall forecast result. This method not only reduces the variance of predictions, but also reduces the generalization error
Figure 1 Proposed neural network forecast model

Figure 3. Daily power consumption
1007
Figure
experimental and development methodology in section 3. section 4 presents the experimental results and discussion, and the paper is summarized in the conclusion. 2 Annual power generation against load
3. METHOD Modeling power consumption of a smart home is very challenging due to its stochastic nature and non linear relations over time. Given the sequence by sequence nature of the multivariate dataset used in this model, where an input sequence time (x = ��1, ��2,··· , ����) with ����∈ Rn, and n is the variable dimension. Our objective was to predict the corresponding outputs (y = ��1,�� 2,··· , ��ℎ) at each time step. The expected result of this type of sequential modeling network is to obtain a nonlinear mapping of input sequence (x) to the prediction sequence (y) through optimization from the current state as: (��1,��2, ,��ℎ)= ℎ 2�� (��1,��2,, ,����) (1) Also, considering the neural network and its weights, the distinct forecast output gives: ���� =��∑ ���� �� ��=1 ��1�� +���� (2) where ���� is the input to the neuron, ���� is the weight of the network, ���� is the bias in the network, ��() is the nonlinear function, while ���� is the output Therefore, our objective is to develop a network architecture capable of optimizing the mapping process. The development process started with the framing of the type of
Smart power consumption forecast model with optimized weighted … (Alexander N. Ndife)
Int J Artif Intell ISSN: 2252 8938
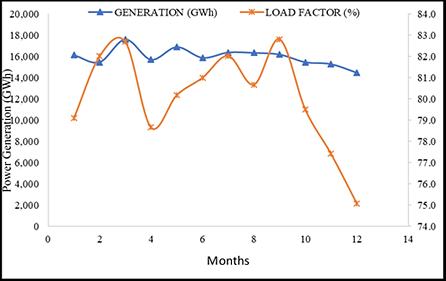
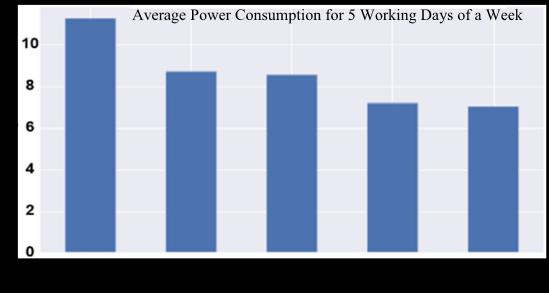
3.1. Dataset
Because of power consumption correlation to previous load consumption historical data and consumer behavior [20], this research leveraged secondary data from [12] that was augmented with remote sensing data acquired from SGtech Smart Office. This secondary data was a multivariate time series dataset containing 2,075,259 measurements gathered from a house located in Sceaux, France, between December 2006 and November 2010 (47 months), recorded in real time. The observations were made every minute and the temporal data captured the consumption behavior across different seasons of the year and weather conditions. Given that SGtechNet is interested in modeling power consumption behavior of a typical smart home, where all appliances are automated, we therefore validated the model performance with real time data from a smart office. Our Smart Office data were collected through a smart means where devices in the automated office were configured to transmit data in real time to a smart meter to enable
we are interested in, considering the available datasets, and proceeded to how the network could be trained and validated and finally ended with the performance evaluation. However, during the data preprocessing stage, we noticed non stationarity and seasonality trends due to the spatiotemporal factors contained in the power consumption datasets. This prompted the decision to apply a different approach to modeling power consumption behavior for reliable forecasting. Statistical methods and neural network combinations [15] [18] have been applied to regression problems of this nature with good results. Ordinarily, a stochastic method approach would have been the easiest to apply, particularly for power consumption forecasting, if not for its error susceptibility and inflexibility [1], [18], [19] implemented different types of neural networks for time series problems. However, as we are interested in predicting a week ahead horizon, we started our experimentation using the previous 7 days power demand as the input vector ����, and the next 7 steps ahead as ���� in our adaptive algorithm and continued varying the timesteps upwardly based on the hypothesis of the more the timesteps the better the prediction. Additionally, power consumption dependencies such as weather, calendar events (holidays, family social events, festival days and so on), other factors such as geographical locations, human comfortable temperature, heating/cooling technology, and type of consumers or purpose of electricity use industrial or residential, were included as additional lagged features to assist our model learn the data better This forecast method was implemented on deep learning encoder decoder networks. Dropout, which have been a common technique in model regularization, were used to block out a random set of unit cells during model training to avoid overfitting. In (3) expresses the way in which this proposed model accepts multi variant time series input variables and output 7 distinct forecasts ahead. The input parameters are the previously observed data at the scale time (t+y 1, t+y 2 … t). Therefore, the answer to finding the relationship between the input and output data) for the purpose of predicting the future data at the time (t+p) lies in the nonlinear functional mapping from the past observations of the time series to the future value, calculated in (3) and using (4) yt =�� ̃ (yt 1, yt 2,….,yt p,��)+ ԑ�� (3) where w is a vector of all parameters and f is the function determined by the network structure and the connectionUsingweightsasimple feed forward neural network architecture with 3 layers, for example, the output of the model can be computed as: ���� =α0 +∑ α�� �� ��=1 ��(β0j +∑ ������ �� ��=1 ���� 1)+ԑ��,Ɐ (4) At the instances ���� �� (�� =1,2,3, ,��) are the p inputs and ���� is the output and p, q are the integer values of the number of input nodes and hidden nodes respectively, while ���� (�� =0,1,2, ,��) and ������(�� = 0,1,2, ,��;�� =0,1,2, ,��) are the connection weights, and ԑ�� is the random shock, ��0 and ��0�� are the bias terms. For activation of this type of model, nonlinear activation functions such as the logistic sigmoid function or similar, such as linear, gaussian, hyperbolic tangent and so forth can be used However, the estimation of the connection weights as a measure for minimizing the error function in this network can be done using the nonlinear least square method of (5) ��(��)=∑ et 2 �� =∑ (���� �� ̂ ��)²�� (5) In (5) applies an optimization technique for error minimization, where �� is the space of all connection weights.
ISSN: 2252 8938 Int J Artif Intell, Vol. 11, No. 3, September 2022: 1004 1018
1008prediction
Smart power consumption forecast model with optimized weighted … (Alexander N. Ndife) 1009 profiling of each individual appliance, and their power consumption, and as well serving the purpose of power quality monitoring. Figure 4 showed the ditributions of the variables, and we later added one additional variable, Sub_metering_4 as shown to Figure 5, to the original 7 independent variables that comprised the original dataset secondary data from [12] This represents active energy for electric vehicle (EV) charging and other miscellaneous energy needs that were not accounted for in the original dataset In the model design, additional features like weekend and weekday as shown in Figure 3, were added because Total Active Power consumed changes very much for weekdays and weekends. Both datasets were split and 75% of each was used for model training, with the remaining 25% used for validation. The variables are obviously time dependent and can easily be influenced by changes in the weather. However, the unique characteristic of the weather suggests that location is an important determinant of a method to be applied in power forecasting. Location variation can invalidate the potency of a successful method when it is applied in another location with different weather and ambient characteristics. Therefore, a use case scenario [13] that characterizes power demand in urban areas, and rural areas across Thailand, was used for easy determination of likely energy demand in each category and to consider their various power consumption behavior. By this idea, the result of this predictions can therefore be compared with other predictions applicable to different locations and based on similar characterization 4 distributions
. Figure
Int J Artif Intell ISSN: 2252 8938
Plot of dataset variable
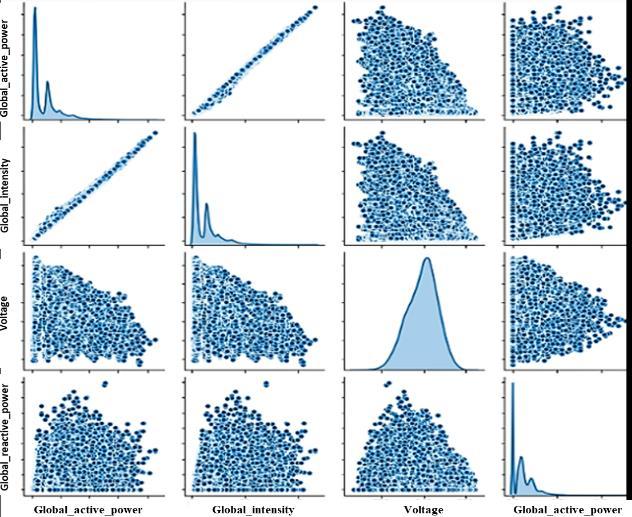
Since various factors including atmospheric climate domain factors are some of the determinants of power consumption differences experience across different locations, SGtechNet analyzed those factors. Diverse atmospheric climate differences across locations prompted the need to validate the performance of this model using multivariate datasets collated from different locations France and Thailand precisely. Basically, to determine the effect and influence of climatic factors relative to performance for proper comparison with other forecasting methods. Therefore, series of experiments is conducted at different timesteps with the same model configuration to increase the confidence in the prediction and validity for future studies To ascertain the effectiveness of this weighted average ensemble method against the backdrop
ISSN: 2252 8938 Int J Artif Intell, Vol. 11, No. 3, September 2022: 1004 1018 1010
This public dataset was cleaned, and imputation method used to fill all missing and corrupted values using a day wise last observation carried forward (LOCF) technique. This simply means carrying an observation from the same time the previous day. In a time series data of this nature with seasonality trend, other methods like linear interpolation, seasonal adjustment + linear interpolation could also be applied From Figure 4, it can be noticed that voltage seems to have a gaussian distribution where as rest of the data seems skewed (i.e., non symmetric), necessitating power transformation of the data before modelling. Exploratory analysis further showed that global active power expected to be predicted has strongest correlation with global intensity with a factor of 1 Therefore, this paper further investigates the extent each input variable affects the outcome of the prediction result of the global active power.
3.2. Model configuration
The network is trained to forecast the next consecutive 7 days a week ahead time steps using the learned features. Those additional features introduced during model design for the purposes of augmenting the data are concatenated to the vector and passed to the final prediction. Because ensemble method was used to ensure a better generalization, global optimization was consequently performed on the ensembled models to find the best coefficients for the weighted ensemble. The result of this optimization determines the individual contributions of the weight of each ensemble method to the final prediction 3.2.2. Prediction/evaluation
Network training
The architecture of the network has 7 input dimensions with 1 output layer, 3 convolutional and hidden layers each. This architecture consists of combination of convolutional neural network (CNN) and long sort term memory (LSTM) deep networks. While the input transformations and feature representation take place in the convolutional layers, the resulting output is convolved and read into fully connected LSTM unit. Since the input data is a 1 D sequence, it was easy for the interpretation over the number of time steps. The LSTM has 3 hidden layers with 4 gates that handles updates and memory functions of the network. As the gates receives both the input output from the last convolutional layer obtained at previous time step (ℎ�� 1) and the related current time (�� ��) the forget gate takes ���� and ℎ�� 1 as input to determine the information to be retained in cell state (Ct 1) using sigmoid layer ���� and ���� 1 denotes cell states at timesteps t and t −1 respectively. The value of Ct is therefore determined by the input gate ���� using ���� and ℎ�� 1 However, the function of the output gate is to regulate the output of LSTM cell based on ���� using both sigmoid layer and tanh layer 3.2.1.
3.1.1. Date pre processing
Figure 5. Individual distribution of the attributes
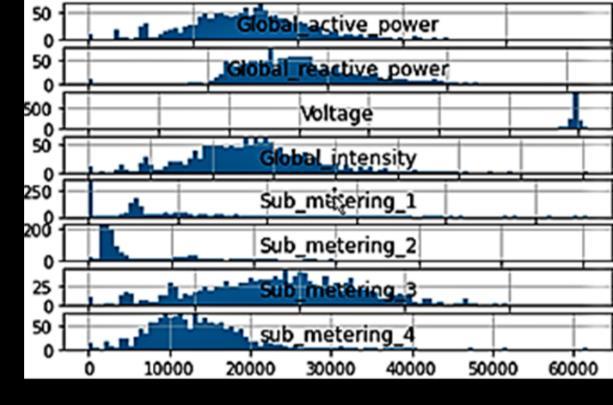
Int J Artif Intell ISSN: 2252 8938
Smart power consumption forecast model with optimized weighted … (Alexander N. Ndife) 1011 of the limitation of poor performance resulting from allowing equal contributions from ensemble members to the final prediction model especially when some of the models are bad. And mitigate against the drawback of lengthy preference ordering calculation of individual ensemble members which often results to higher computational complexity in some ensemble techniques like voting [21], two predictions were considered: using different numbers of ensemble members in increasing levels of complexity across different timesteps and model averaging method. We started with 10 ensemble members whose contributions to the final prediction model is based on their confidence level and kept varying the numbers until we reached a standalone. It was discovered that there were no discrepancies in error when the number of ensemble members were varied. However, a significant discrepancy is reported using model averaging method where equal contribution from ensemble members was allowed. The prediction performance of the proposed model is computed based on root mean square error (RMSE) and compared against mean absolute percentage error (MAPE) and mean absolute error (MAE) errors see Figure 6 over averaging ensemble method and standalone method These metrics are the most used performance measures for time series analysis because the error is of the same unit with the predictions and their errors can range from 0 to ∞. Figure 7 shows the validation loss across different timesteps (7, 14, 21 and 28). Walk forward validation scheme was implemented, where the model made 1 week prediction, then utilized the actual data for the week or 2 weeks as a basis for the predicting the subsequent week.Figure
6. Power consumption across day and time Figure 7. Validation loss across different timesteps 3.3. Encoder decoder network 05101520253035404550556065 40000 60000 80000 100000 120000 140000 160000 180000 200000 220000 1 2 3 4 TimestepsEpoch LossValidation
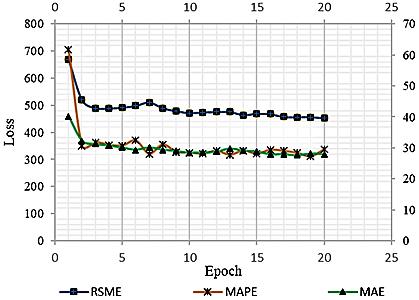
We considered advanced feature representation methods, such as encoder decoder, to preserve the hidden abstractions and invariant structures in the time series input. These have been previously applied in both reinforcement [22], supervised [23] and unsupervised learning This unsupervised neural network method is designed for the adaptive learning of the long term dependency and hidden correlation features of multivariate spatiotemporal data and was trained to reconstruct its own input in each layer as its output which is used as the inputs of the successive layer. In this paper, an encoder that extracts useful representative features from the time series input data was trained in such a way that the decoder could conveniently reconstruct those features from the encoded space. Specifically, the output of the convolutional layers is concatenated by Conv2D followed by LSTM layers, as achieved in [5], [24] to capture all the inherent spatiotemporal correlations in the time series input data. This proposed ConvLSTM encoder decoder architecture has 2 sub models: one for reading the input sequence and encoding (i e , mapping the variable length source sequence) this sequence into a fixed length vector, while the second part decodes the fixed length vector and outputs the predicted sequence (i e , mapping the vector representation back to a variable length target sequence). This output of the decoder represents the learned feature. Thereafter, a dense layer is used as the output for the network, and it uses the same weights by wrapping the dense layer in a time distributed wrapper function used in the network 3.4. Model compression On device systems are resource constrained, with limited memory and low computing power. However, deep learning algorithms are computational and memory intensive, so they cannot be implemented on real world applications or other resource constrained systems without difficulties As deep learning models goes deeper in layers their inference time increases along with the increase in number of trainable parameters; making it difficult to be deployed on resource constrained devices. By the parsimony concept, models with a smaller number of parameters are more likely to provide adequate representation of the underlying time series data, but models with a high number of trainable parameters requires more energy and space and are likely to overfit during training. Consequently, compression technique, as presented in [25], is required to allow the deployment of a large model on resource constrained devices Table 2 summarized the results from literature on the most recent efforts towards model size and trainable parameter reduction leveraging on different techniques in comparison with SGtechNet. This comparative analysis shows that SGtech has the least number of trainable parameters with a very considerate model size, hence the justification for its suitability for low power low memory devices. Model size is very important as far as performance optimization of on device system is concerned because larger models mean more memory reference and more energy [26]
Table 2 Model parameter comparison Model Parameters Size Training Time Inference Time ENet [27] 0.37 M 0.7 MB 15mins 383ms LEDNet [28] 1.856 M 3.8 MB SegNet [29] 29.46 M 56.2 MB 37mins 286ms AlexNet [30], [31] 60 M 232 MB 7,920mins VGG16 [31], [32] 138 M 528 MB SqueezNet [25] 0.66 M 4.8 MB ResNet152 [31] 232 M 60 MB GoogleNet [31] 6.8 M 28 MB SGtechNet (Proposed) 128K 4.93 MB 1.3mins 3ms
ISSN: 2252 8938 Int J Artif Intell, Vol. 11, No. 3, September 2022: 1004 1018 1012
Therefore, to fit the SGtechNet model on limited resourced devices, enabling the model to be usable in real world applications, the SqueezeNet [25] concept of was used, with the modification that the 1x1 and 1x3 convolution filters were used for feature representation. As each kernel receives an input time series, the corresponding outputs are concatenated and followed by convolutional LSTM layers which capture the long term spatial patterns in the electricity consumption data. This method not only reduces input data dimensionality but also reduces the complexity of the data [33] leading to an improved result even though a marginal cost burden is incurred due to a slight increase in number of parameters. However, the choice of a smaller filter reduces the models inference time. Also, SqueezeNet has almost the same accuracy of AlexNet with its compression of trainable parameters, but that accuracy is a little lower than GoogleNet. SeNet [34] developed an architecture that recalibrates channel wise feature responses and uses them to determine the interdependencies existing between two channels. Channel wise scale and element wise summation operations were combined into a single layer “AXPY ”using skip connections. This resulted in considerable
Figure
To address this limitation, an ensemble method of [7] with a weighted average of different trained models is used for prediction. Ordinarily, the model ensemble method allows each model an equal contribution to the final prediction which could sometimes be seen as a limitation when the contribution from poorly performed models to the final model jeopardizes the efforts of a well performed model. However, the contribution to the final model in this proposed model is purely dependent on the model’s trust and estimated performance, resulting to an improved overall prediction result. Sensitivity analysis was carried out to determine the number of ensemble members most appropriate for the forecasting problem and how impactful they could be to the test accuracy. To determine trustworthiness of ensemble models and to estimate performance, we need to find their weights. However, due to there being no analytical solution to estimation of values for the weights, we used gradient descent optimization with a unit norm weight constraint on the holdout validation set rather than on the training set. Ordinarily, a simpler way of finding each ensemble member’s weights would have been to grid search values but because our holdout validation is large enough, gradient descent optimization becomes the best option. This optimization procedure sums up all the model vector of weights to 1 i e , ��1, ��2,···, ���� = 1, also constrains them to positive values to allow weights to indicate the percentage of trust or expected performance of each model. The optimization process utilizes the set of information provided to it to search
The stochastic nature of power consumption varying with season and time necessitated the use of a stochastic learning algorithm for dataset training. However, the neural network algorithm has the inherent limitation of randomness which results in a different final model each time it is trained on the same dataset.
Feature learning or representation learning in machine learning is a set of techniques that allows a system to automatically discover the representations needed for feature detection or classification from raw data. Figure 8 shows the feature learning process This is a method of finding a representation in each data the features, the distance function, and the similarity function dictates how the predictive model will perform. Feature representation helps to reduce data complexity, so the anomalies and noise can be reduced. It also helps in reduction of the dimensional of input data, making it easier to find patterns, anomalies, and also provides a better understanding of the behavior of the data generally. Because our time series input data is 1D, a smaller kernel filters (1, 3) were used in the convolutional layers for feature learning.
Int J Artif Intell ISSN: 2252 8938
3.6. Ensemble method
Considering the spatiotemporal nature of power consumption variables, a state space representation of (6) represents the transition process expressing the discrete stochastic behavior of the variables and (7) represents the likelihood of the observations with the assumption that states are part of the model parameters.
φi+1 = φi+ut (6) yt =�� ̃ (xt, Ɵt)+ Vt (7) where ut is the process noise, vt isthemeasurednoise 8. Feature learning process
reductions in memory, cost, and computational burden It is imperative to note that the application environment of most of the state of the art models in Table 2 is image classification and detection, so for SGtechNet to achieve a RMSE Error of 358kwh in a regression task like power forecasting shows high level of robustness. Even though training and inference time for some of these models compared with SGtechNet was not reported in the literature, the few ones that were reported clearly put SGtechNet at advantage in terms of computational complexity.
3.5. Feature representation
Smart power consumption forecast model with optimized weighted … (Alexander N. Ndife) 1013
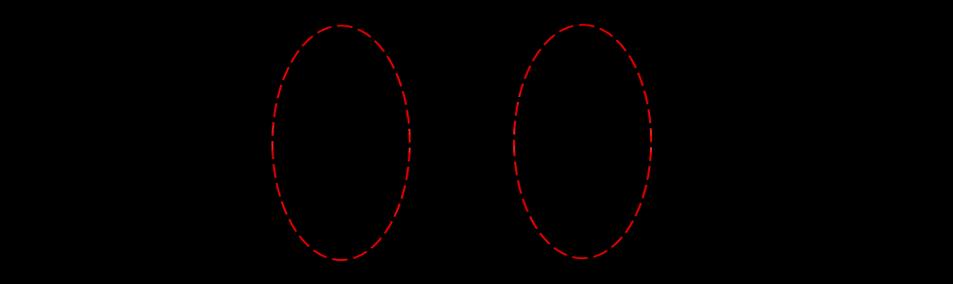
1014forweights with lower errors under defined bound (i e , 0 0 1.0) amongst 10 ensemble members until convergence. But, before performing weight optimization, 10 single models were created, and their individual performances were evaluated on the test dataset. For the optimization, a differential evaluation function was used to search and display the optimal sets of weights after several iterations which returned the score to be minimized and retrieved the best weights, with their performance being reported on the holdout validation data. Optimal weights of the base learners are aggregated to find the best tradeoff between bias and variance and minimize the prediction error. So, each base learner’s prediction (�� ̂ ��) on holdout validation set, therefore gives: ����������������(��1�� ̂ 1 +��2�� ̂ 2+···, ������ ̂ ��,��) (8) such that ∑ ��;�� ��=1 =1, when ��j ≥ 0 Ɐj =1, ��, where ��j represents the weights corresponding to base model j (j = 1,..,k), ŷ is the vector predictions of base model j, and y is the vector of true value. So, at any instance of training the base learner j, weights ��j is computed from optimization (4) on the assumption that n is the total number of instance, ���� as the true value of observation i, �� ̂ ���� as the prediction of observation i by base model j. ������ 1 ��(∑ ���� �� ��=1 ∑ ���� �� ��=1 �� ̂ ����) (9) Such that ∑ ��;�� ��=1 =��,��ℎ������j ≥ 0 Ɐj =1, �� The ensemble member contributions are evaluated based on those chosen weights. This process not only improve model performance but also saves time. Ordinarily, the search for such weights with lower error values would need to be done randomly and exhaustively, which is time demanding 3.6.1. Comparing weighted ensemble and model averaging method performance Table 3 shows the results produced by the weighted average ensemble method, which demonstrate that this method outperformed the model averaging method for individual ensemble members even though their processing time variation is insignificant Furthermore, the model’s performance is compared with baseline model see Table 4 using both secondary and primary datasets acquired from two different continents. The importance of this comparative analysis is to provide completeness of this study analysis as regards the major limitation of ensemble technique which is misleading assumption that all ensemble members are equally effective.
4. RESULTS AND DISCUSSION
A real time experimentation using Google Colab TPU and one of the finest neural network APIs, contained in Keras® with its backend TensorFlow produced the results shown in Table 3 and Table 4. Based on the performance evaluation of the model, this model significantly outperformed the baseline model. Though an unstable training trajectory was experienced during training, which could be likened to overfitting in the training data, the overall performance is good. In the model’s evaluation result of Figure 9, RMSE was found to statistically differ across the 7 days of the week as shown in Figure 9(a) while Figure 9(b) showed how the training error decreased sharply after commencement of training, before it became linear, due to the model’s complexity: likewise, the validation error. A squeeze layer technique, adopted from [35], reduced the size of the model to 4 9 M without affecting its performance, making it implementable in a low power low
ISSN: 2252 8938 Int J Artif Intell, Vol. 11, No. 3, September 2022: 1004 1018
Table 3 Comparative analysis of weighted ensemble models and model averaging method Statistics Weighted Ensemble Models Model Averaging Method Number of Iteration 1,000 1,000 Validation Time 2.053s 2 185s Average RMSE 358kwh 362 617kwh Table 4. Comparative analysis of weighted ensemble models and baseline model on different datasets Model Statistics Training on HHPC Dataset France Training on Real Time Dataset, SGtech, Thailand Propose Model Baseline Model Propose Model Persistence Model Hourly Daily Weekly Training Time 114 109s 78 916s Prediction Time 2 282s 2 053s RMSE 3 61 885kwh 465 294kwh 358kwh 480 246kwh 469 389kwh 465 294kwh
Comparative analysis
Smart power consumption forecast model with optimized weighted … (Alexander N. Ndife) 1015 memory device; Smartphone, iPad, Tablets. One of the limitations of the model performance enhancement method being discussed in this paper is that, as the model size is reduced, the number of parameters slightly increased, resulting in a marginal increase in resources usage relative to implementation. Therefore, further work is proposed to develop a systematic method of reducing the model size without necessarily increasing the number of model parameters 4.1.
and
Evaluation of this model’s performance was against the baseline model and other alternative forecasting methods even though some metrics, such as computational speed and prediction time, were not captured in all the literature reviewed. We also analyzed power consumption datasets used in validating SGtechNet along daily consumption cycles time and day as shown in Figure 10(a) and 10(b) respectively, for clearer understanding of residents habits. An experimental framework for the empirical comparison of different model performances, based on varying test conditions, was introduced. Uniqueness of weather characteristics in different locations indicated that there is no guarantee that a forecasting method that is successful at one location would be effective at a different location. The inclusion of this framework in the design accounts for diverse climatic conditions and created a valuable environment for future studies in emerging forecasting technologies. This increases the confidence in the observed results by allowing the validity of the forecasting algorithm to be tested on both the test set from France and the test set from SGtech Naresuan University Thailand, both of which are real time data. Alternatively, to prove that the improved processing time and other improvements achieved in this model are due to pure scientific contributions rather than software and hardware differences, we experimented on different technologies. We compared the results when using an NVIDIA GeForce GTX1080 TI GPU/TPU enabled TensorFlow against those achieved when using an NVIDIA Tesla K80 GPU running on the Ubuntu Server 16 04 3. The discrepancy in the results was found to be scientifically insignificant. The result of this model is further compared with model averaging and standalone methods as shown in Table 5. SGtechNet model size is 4.93 MB which means it can easily be put in an on chip Static random access memory (SRAM) cache. (a) (b) 9 (a) RMSE 7 days consecutive days forecasted (b) Model’s validation 935MB
SGtechNet performance evaluation result showing
across the
power
loss Table 5. Comparison of the experimental results of proposed model against some existing power forecast methods Model Statistics Models Propose Model Persistence Model Model A [36] Model B [37] Training Time 114 109s Prediction Time 2 282s Size 4.
Figure
Int J Artif Intell ISSN: 2252 8938
RMSE 358kwh 465 294kwh 530kwh 450 5kwh
training and
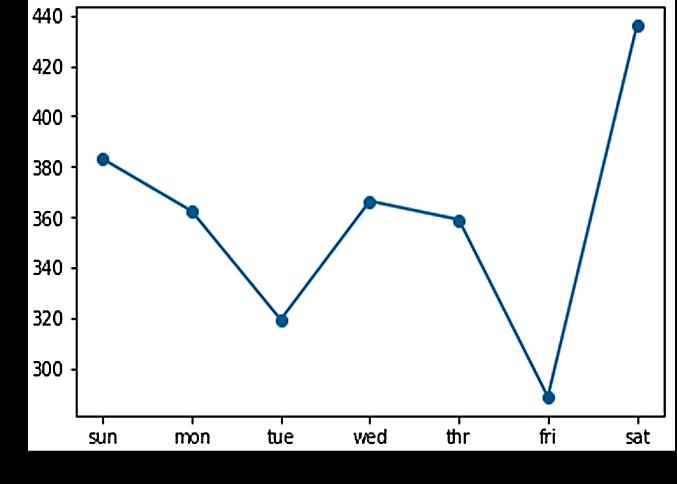
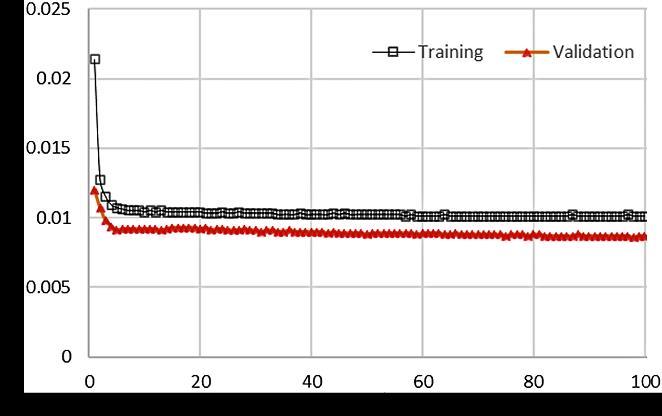
ISSN: 2252 8938 Int J Artif Intell, Vol. 11, No. 3, September 2022: 1004 1018 1016 (a) (b)
[5] L. Zhu and N. Laptev, “Deep and Confident Prediction for Time Series at Uber,” in 2017 IEEE International Conference on Data Mining Workshops (ICDMW), Nov. 2017, pp. 103 110, doi: 10.1109/icdmw.2017.19.
[1] K. M. U. Ahmed, M. Ampatzis, P. H. Nguyen, and W. L. Kling, “Application of time series and Artificial Neural Network models in short term load forecasting for scheduling of storage devices,” Sep. 2014, doi: 10.1109/upec.2014.6934761.
REFERENCES
[4] S. J. Taylor and B. Letham, “Forecasting at Scale,” The American Statistician, vol. 72, no. 1, pp. 37 45, Jan. 2018, doi: 10.1080/00031305.2017.1380080.
[6] R. Livni, S. Shalev Shwartz, and O. Shamir, “On the Computational Efficiency of Training Neural Networks,” dvances in neural information processing systems, vol. 27, 2014. [7] X. Qiu, L. Zhang, Y. Ren, P. Suganthan, and G. Amaratunga, “Ensemble deep learning for regression and time series forecasting,” Dec. 2014, doi: 10.1109/ciel.2014.7015739.
[2] G. K. F. Tso and K. K. W. Yau, “Predicting electricity energy consumption: A comparison of regression analysis, decision tree and neural networks,” Energy, vol. 32, no. 9, pp. 1761 1768, Sep. 2007, doi: 10.1016/j.energy.2006.11.010.
[10] J. Xie, B. Xu, and Z. Chuang, “Horizontal and Vertical Ensemble with Deep Representation for Classification,” Jun. 2013, [Online]. Available: http://arxiv.org/abs/1306.2759.
5. CONCLUSION Nationwide lockdown due to covid 19 pandemic is causing a rise in domestic power consumption, making energy conservation, and planning more relevant than ever. In our research, we demonstrated the effectiveness of combining atmospheric climate domain knowledge of factors determining power consumption differences based on location, with empirical data captured from automated systems for future energy forecasting This forecast model SGtechNet developed to optimize the data learning and prediction process leveraged on a multivariate dataset to make a multi step time series 7 days ahead forecast. SGtechNet, is based on ConvLSTM Encoder Decoder algorithm explicitly designed to optimize the quality of spatiotemporal encodings throughout the feature extraction process. The validation report of this model showed a significant improvement on the forecast result when a real time dataset from an automated office was used for model validation which was compared against a manually operated home/office represented by the secondary data. This implies, aside from the social behavioral factor that propels the users ’choice of time of use (ToU) electricity, that environmental and real time control factors are also contributory factors that determine the consumption rate and therefore cost of power that is consumed domestically or in an office workplace. The RMSE of 361 kwh recorded was compared with 465 kwh on the persistence model and an improved RMSE of 358 kwh was achieved when validated in holdout validation data from the automated office. Overall performance on error rate, forecast time and inference time were later compared with published research, and the comparison showed that our model, the SGtechNet, provided significant improvements in these factors One of the most significant achievements of SGtechNet is its adaptiveness to other forecast problems and different datasets in such a way that it detected and analyzed the atmospheric climate changes over different locations.
[11] G. Huang, Y. Li, G. Pleiss, Z. Liu, J. E. Hopcroft, and K. Q. Weinberger, “Snapshot Ensembles: Train 1, get M for free,” Mar.
Figure 10. Shows the plot of power consumption across different (a) Time and (b) Weekday
[3] H. K. Alfares and M. Nazeeruddin, “Electric load forecasting: Literature survey and classification of methods,” International Journal of Systems Science, vol. 33, no. 1, pp. 23 34, Jan. 2002, doi: 10.1080/00207720110067421.
[8] P. Izmailov, D. Podoprikhin, T. Garipov, D. Vetrov, and A. G. Wilson, “Averaging Weights Leads to Wider Optima and Better Generalization,” Mar. 2018, [Online]. Available: http://arxiv.org/abs/1803.05407.
[9] O. Gokalp and E. Tasci, “Weighted Voting Based Ensemble Classification with Hyper parameter Optimization,” in 2019 Innovations in Intelligent Systems and Applications Conference (ASYU), Oct. 2019, pp. 1 4, doi: 10.1109/asyu48272.2019.8946373.
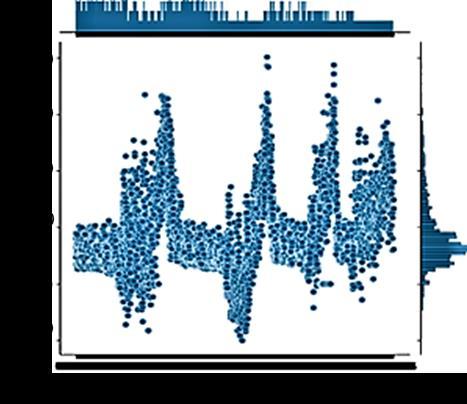
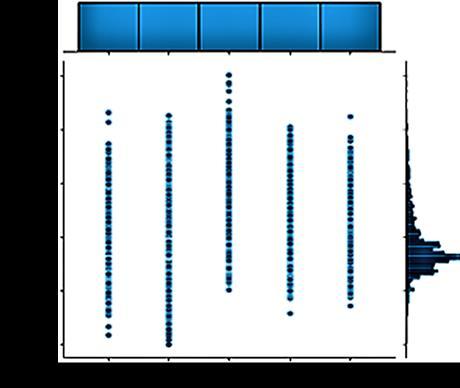
[23] S. Du, T. Li, Y. Yang, and S. J. Horng, “Multivariate time series forecasting via attention based encoder decoder framework,” Neurocomputing, vol. 388, pp. 269 279, May 2020, doi: 10.1016/j.neucom.2019.12.118.
[25] F. N. Iandola, S. Han, M. W. Moskewicz, K. Ashraf, W. J. Dally, and K. Keutzer, “SqueezeNet: AlexNet level accuracy with 50x fewer parameters and <0.5MB model size,” Feb. 2016, [Online]. Available: http://arxiv.org/abs/1602.07360.
[27] A. Paszke, A. Chaurasia, S. Kim, and E. Culurciello, “ENet: A Deep Neural Network Architecture for Real Time Semantic Segmentation,” Jun. 2016, [Online]. Available: http://arxiv.org/abs/1606.02147.
[12] D. D.a.K.T., “Household Electricity Consumption Dataset,” UCI Machine Learning Repository, 2017. http://archive.ics.uci.edu/ml.
[14] “Energy Statistics of Thailand,” Energy Policy and Planning Office, 2020. http://www.eppo.go.th/index.php/en/ (accessed Mar. 05, 2021).
[26] M. Horowitz, “1.1 Computing’s energy problem (and what we can do about it),” Feb. 2014, doi: 10.1109/isscc.2014.6757323.
[17] C. Nichiforov, I. Stamatescu, I. Fagarasan, and G. Stamatescu, “Energy consumption forecasting using ARIMA and neural network models,” Oct. 2017, doi: 10.1109/iseee.2017.8170657. [18] Z. Haydari, F. Kavehnia, M. Askari, and M. Ganbariyan, “Time series load modelling and load forecasting using neuro fuzzy techniques,” Oct. 2007, doi: 10.1109/epqu.2007.4424201.
[13] K. Poolsawat, W. Tachajapong, S. Prasitwattanaseree, and W. Wongsapai, “Electricity consumption characteristics in Thailand residential sector and its saving potential,” Energy Reports, vol. 6, pp. 337 343, Feb. 2020, doi: 10.1016/j.egyr.2019.11.085.
[28] N. Rangappa, Y. R. V. Prasad, and S. R. Dubey, “LEDNet: Deep Learning Based Ground Sensor Data Monitoring System,” IEEE Sensors Journal, vol. 22, no. 1, pp. 842 850, Jan. 2022, doi: 10.1109/jsen.2021.3129173.
[29] V. Badrinarayanan, A. Kendall, and R. Cipolla, “SegNet: A Deep Convolutional Encoder Decoder Architecture for Image Segmentation,” IEEE Transactions on Pattern Analysis and Machine Intelligence, vol. 39, no. 12, pp. 2481 2495, Dec. 2017, doi: 10.1109/tpami.2016.2644615.
[31] M. P. Véstias, “A Survey of Convolutional Neural Networks on Edge with Reconfigurable Computing,” Algorithms, vol. 12, no. 8, p. 154, Jul. 2019, doi: 10.3390/a12080154. [32] K. Simonyan and A. Zisserman, “Very Deep Convolutional Networks for Large Scale Image Recognition,” Sep. 2014, [Online]. Available: http://arxiv.org/abs/1409.1556. [33] N. Tishby and N. Zaslavsky, “Deep learning and the information bottleneck principle,” Apr. 2015, doi: 10.1109/itw.2015.7133169. [34] J. Hu, L. Shen, and G. Sun, “Squeeze and Excitation Networks,” Jun. 2018, doi: 10.1109/cvpr.2018.00745. [35] G. Nanfack, A. Elhassouny, and R. O. H. A. J. Thami, “Squeeze SegNet: a new fast deep convolutional neural network for semantic segmentation,” in Tenth International Conference on Machine Vision (ICMV 2017), Apr. 2018, doi: 10.1117/12.2309497. [36] E. Busseti, I. Osband, and S. Wong, “Deep learning for time series modeling,” Technical report, Stanford University, pp. 1 5, 2012. [37] H. Shi, M. Xu, and R. Li, “Deep Learning for Household Load Forecasting A Novel Pooling Deep RNN,” IEEE Transactions on Smart Grid, vol. 9, no. 5, pp. 5271 5280, Sep. 2018, doi: 10.1109/tsg.2017.2686012.
[15] A. Mellit, M. Benghanem, A. H. Arab, and A. Guessoum, “A simplified model for generating sequences of global solar radiation data for isolated sites: Using artificial neural network and a library of Markov transition matrices approach,” Solar Energy, vol. 79, no. 5, pp. 469 482, Nov. 2005, doi: 10.1016/j.solener.2004.12.006.
[24] R. Asadi and A. C. Regan, “A spatio temporal decomposition based deep neural network for time series forecasting,” Applied Soft Computing, vol. 87, p. 105963, Feb. 2020, doi: 10.1016/j.asoc.2019.105963.
[19] F. Fahmi and H. Sofyan, “Forecasting household electricity consumption in the province of Aceh using combination time series model,” Oct. 2017, doi: 10.1109/iceltics.2017.8253239.
BIOGRAPHIES OF AUTHORS Alexander N. Ndife is a Data Scientist and Doctorate degree holder in Smart Grid Technology from Naresuan University, Thailand. He obtained a bachelor’s degree in Electrical/Electronic Engineering, Anambra State University, Nigeria in 2008 with specialty in telecommunications and subsequently Masters’ degree in Electronics and Computer (Communications Engineering) from Nnamdi Azikiwe University, Nigeria in 2014. His research interests include Wireless Networks, Artificial Intelligent Systems, Deep Learning, Image Processing, and Cybersecurity & Smart Grid Networks. He is a registered engineer in Nigeria and CEO Ogugbalex Engineering limited. He has published numerous scientific papers and a member of various engineering organizations including Nigerian Society of Engineer (NSE), IEEE, IAENG and belongs to Society of Wireless Networks He can be contacted at email: alexandern60@email nu ac th
[20] B. Dhaval and A. Deshpande, “Short term load forecasting with using multiple linear regression,” International Journal of Electrical and Computer Engineering (IJECE), vol. 10, no. 4, pp. 3911 3917, Aug. 2020, doi: 10.11591/ijece.v10i4.pp3911 3917.
[30] A. Krizhevsky, I. Sutskever, and G. E. Hinton, “Imagenet classification with deep convolutional neural networks,” Advances in neural information processing systems, vol. 25, pp. 1097 1105, 2012.
[16] L. Marwala and B. Twala, “Forecasting electricity consumption in South Africa: ARMA, neural networks and neuro fuzzy systems,” Jul. 2014, doi: 10.1109/ijcnn.2014.6889898.
[21] F. Leon, S. A. Floria, and C. Badica, “Evaluating the effect of voting methods on ensemble based classification,” Jul. 2017, doi: 10.1109/inista.2017.8001122.
[22] X. Shi, Z. Chen, H. Wang, D. Y. Yeung, W. Wong, and W. Woo, “Convolutional LSTM Network: A Machine Learning Approach for Precipitation Nowcasting,” Advances in neural information processing systems, vol. 28, Jun. 2015, [Online]. Available: http://arxiv.org/abs/1506.04214.
Int J Artif Intell ISSN: 2252 8938 Smart power consumption forecast model with optimized weighted … (Alexander N. Ndife) 1017 2017, [Online]. Available: http://arxiv.org/abs/1704.00109.
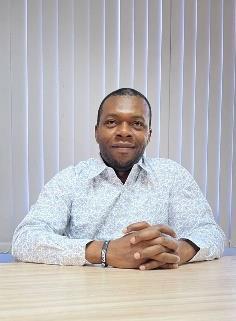



ISSN: 2252 8938 Int J Artif Intell, Vol. 11, No. 3, September 2022: 1004 1018 1018
Paisarn Muneesawang received a B Eng. Degree in Electrical Engineering from the Mahanakorn University of Technology, Bangkok, Thailand, in 1996, M.Eng. Sci. Degree in Electrical Engineering from the University of New South Wales, Sydney, NSW, Australia, in 1999, and Ph D Degree from the School of Electrical and Information Engineering, University of Sydney, Sydney. He was a Post Doctoral Research Fellow with Ryerson University, Toronto, ON, Canada, from 2003 to 2004, and an Assistant Professor with the College of Information Technology, University of the United Arab Emirates, Al Ain, UAE, from 2005 to 2006. He has been a Visiting Professor with Nanyang Technological University, Singapore, since 2012, and with Ryerson University, Toronto, Canada, since 2013. He was the Vice President for Administrative Affairs, Naresuan University, Phitsanulok, Thailand, where he is currently a Professor and Dean of the Graduate School. He co authored Multimedia Database Retrieval: A Human Centered Approach (Springer, 2006) and Unsupervised Learning A Dynamic Approach (Wiley IEEE Press, 2013). He co edited Advances in Multimedia Information Processing PCM 2009 (Springer, 2009). His current research interests include multimedia signal processing, computer vision, and machine learning. He has served as the Registration Co Chair of the International Conference on Multimedia and Expo 2006 and the Technical Program Co Chair of the Pacific Rim Conference on Multimedia 2009 He can be contacted at email: paisarnmu@nu.ac.th
Yodthong Mensin obtained bachelor’s degree (B .Sc) in Computer Science, and Master (M Sc) in Information Technology from Naresuan University, Thailand. He obtained Doctor of Philosophy degree in Energy, communities, and the environment from Chiang Mai Rajabhat University. He also received the Cert. in Renewable Energy Technology from the University of Applied Science, Stralsund, Germany. He is presently a deputy Director of research and academics affairs in School of Renewable Energy and Smart Grid Technology (SGtech), Naresuan University, Thailand. He is a permanent speaker for IEEE conference in the section of Power and Energy Society (PES) in Thailand. He has experience in the field of a microgrid system, automated demand response (ADR), virtual power plant (VPP), energy management system, smart grid data utilization, and energy trading platform with blockchain technology for more than 15 years. Currently, he is the advisory’s team member for the mega project implementation of Thailand utilities, ministry of energy and private sector He can be contacted at email: yodthongm@nu ac th
Wattanapong Rakwichian graduated from Srinakharinwirot University, Phitsanulok, Thailand in Physics (B Ed.). He obtained master’s degree in physics from Chiang Mai University, Thailand, and Doctor of Philosophy (Ph D) in Bioregulation Renewable Energy from Tokyo University of Agriculture, Tokyo, Japan. He is presently the Director of School of Renewable Energy and Smart Grid Technology (SGtech), Naresuan University, Thailand. He had served in various positions in recent times including Sub committee of the Royal Thai Project on Renewable Energy, Consultant of Ministry of Energy, Ministry of Science Technology and Energy. He has written several textbooks on Physics, Mathematics, Solar Energy, and Digital Systems. He has also written many research articles for international journals and proceedings. He has also hosted and participated in many national and international conference related to science, solar energy, and other energy sources He can be contacted at email: wattanapong.r@gmail.com




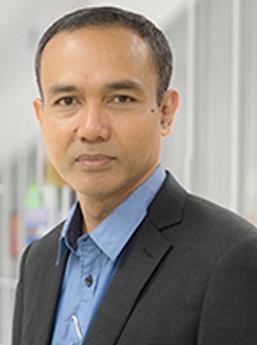



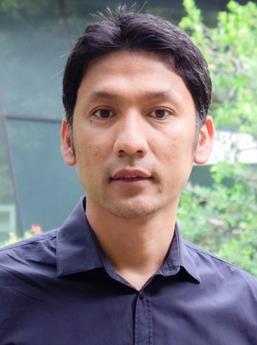



Department of Information Science and Engineering, BNM Institute of Technology, Bengaluru, India
Keywords: Convolutional neural network Interactive emotional dyadic motion capture Long short term memory Multimodal emotional lines
Qdatasetuantum conscious multimodal option mining framework Sentiment analysis This is an open access article under the CC BY SA license. Corresponding Author: Jamuna S DepartmentMurthyofComputer Science and Engineering, Ramaiah Institute of Technology Bengaluru, Karnataka, India Email: jamunamurthy.s@gmail.com
2Department of Information Science and Engineering, Ramaiah Institute of Technology, Bengaluru, India
1. INTRODUCTION Researches in the multimodal option mining happens to be a center exploration point in artificial intelligence linked regions such as emotionalprocessing, data combination and multimodal association. Apart from the conventional text based sentiment examination, the multimodal assumption investigation requires both the use of multimodality portrayal procedures and data combination strategies that are basically highlight level, choice level and hybrid combination methods. Most of the existing multimodal assumption investigation approaches center around distinguishing the extremity of individuals' conclusions which are affixed in online forums. The multimodal reports utilized in these examinations are mostly a part of individual accounts, without including collaborations among expounders or authors [1] [3]. These days, web based media, contacts the different areas of social action. Individuals share data to readily comprehend
Article Info ABSTRACT
1Department of Computer Science and Engineering, Ramaiah Institute of Technology, Bengaluru, India
Article history: Received Sep 22, 2021 Revised Jun 13, 2022 Accepted Jun 20, 2022 Option mining is an arising yet testing artificial intelligence function. It aims at finding the emotional states and enthusiastic substitutes of expounders associated with a discussion based on their suppositions, which are conveyed by various techniques of data. But there exist an abundance of intra and inter expression collaboration data that influences the feelings ofexpounders in a perplexing and dynamic manner. Step by step instructions to precisely and completely model convoluted associations is the critical issue of the field. To pervade this break, an innovative and extensive system for multimodal option mining framework called a “quantum conscious multimodal option mining framework (QMF)”, is introduced. This uses numerical ceremoniousness of quantum hypothesis and a long transientmemory organization. QMF system comprise of a multiple modal choice combination method roused by quantum obstruction hypothesis to catch the co operations inside every expression and a solid feeble impact model motivated by quantum multimodal (QM) hypothesis to demonstrate the communications between nearby expressions. Broad examinations are led on two generally utilized conversational assessment datasets: the multimodal emotional lines dataset (MELD) and interactive emotional dyadic motion capture (IEMOCAP) datasets. The exploratory outcomes manifest that our methodology fundamentally outflanks a broadscope of guidelines and best in class models.
A real-time quantum-conscious multimodal option mining framework using deep learning
Journal homepage: http://ijai.iaescore.com
IAES International Journal of Artificial Intelligence (IJ AI) Vol. 11, No. 3, September 2022, pp. 1019 1025 ISSN: 2252 8938, DOI: 10.11591/ijai.v11.i3.pp1019 1025 1019
3
Jamuna S. Murthy1 , Siddesh Gaddadevara Matt2 , Sri Krishna H. Venkatesh3, Kedarnath R. Gubbi3
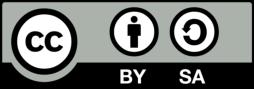
In [21], Liu et al. talked about, With the improvement of insightful dynamics, one sort of choice mode includes countless decision makings (DM), which is called large scale group decision making (LSGDM). In LSGDM,arrogance is one of the normal practices due to numerousDMs' support and the limited soundness of human choice. Carelessness normally contrarily affects LSGDM and can even prompt disappointment in the last decision(s). To accomplish agreement is vital for LSGDM. Henceforth, the reason for this paper is to propose an agreement model which thinks about carelessness practices, and the paper predominantly centers around LSGDM dependent on FRPs SC. In this, a DM bunching technique, which consolidates fluffy inclination esteems likeness and fearlessness similitude, is utilized to arrange the DMs with comparative suppositions into a subgroup. A gathering agreement list which considers both the fluffy inclination esteems and fearlessness is introduced to quantify the agreement level among DMs.
In [23], Zadeh et al represented the issue of multimodal supposition investigation as demonstrating intra methodology and inter methodology elements. They presented anovel model which learns both such
In [22], Qian, b et al talked about Recommender frameworks that propose things that clients may like as indicated by their unequivocal and verifiable input data, like appraisals, surveys, and snaps. Notwithstanding, most recommender frameworks center primarily around the connections among things and the client's last buying conduct while overlooking the client's enthusiastic changes, which assume a fundamental part in utilization movement. To address the test of working on the nature of recommender administrations, paper proposes a feeling mindful recommender framework dependent on half breed data combination in which three agent sorts of data are intertwined to extensively break down the client's highlights: client rating information as unequivocal data, client interpersonal organization information as verifiable data and notion from client audits as enthusiastic data. The test results confirm that the proposed method gives a higher expectation rating and altogether builds the suggestion precision. In this, an exact model dependent on certain criticism information is concentrated tentatively.
ISSN: 2252 8938 Int J Artif Intell, Vol. 11, No. 3, September 2022: 1019 1025 1020andadvise others identified with things they care for. A worldwide framework like Twitter permits individualsto communicate their sentiments in a generally short media message. Getting what individuals feel identified with items orservices is significant both for the leaders that control theindividual items/services and furthermore for their shoppers. Building total information for chiefs should be possible as markers. Markers are values that help chiefs to ground their choices [4] [8].
Multimodal option mining in discussion intends to recognize the emotional conditions of various expounders and study the nostalgic difference inevery expounder over the span of the collaboration. The past multimodal sentimental investigation drawsnear depicting the connections between various modalities, the association elements in discussions that are more intricate, including intra and inter expression collaborations. Intra expression connection alludes to the relationship between various modalities inside one expressionlike the shared impact, joint portrayal and choice combination. Inter expression communication includes rehashed connections among expounders, bringing about the trading of thoughts and affecting each other [9]There[12] has been a lot of research work carried out in the field of sentiment analysis and option mining. The authors in [13] [16] proposed novel multimodal system for rating forecast of buyer items by melding distinctive information sources, in particularphysiological signs and worldwide surveys obtained independently for the item and its brand. The surveys posted by worldwide watchers are recovered and prepared utilizing natural language processing (NLP) strategy to register compound scores considered as worldwide ratings. Additionally, electroencephalogram signs of themembers were recorded at the same time while watching various items on the PC's screen. From electroencephalogram (EEG) valence scores asfar as item evaluating are obtained utilizing self report towards each saw item for procuring nearby appraising. A higher valence score relates to the natural engaging quality of the member towards an item. Radio frequency based relapse procedures are utilized to display EEG information to assemble a rating forecast system considered as neighborhood rating. Moreover, artificial bee colony based streamlining calculation isutilized to help the general presentation of the structure by combining worldwide and neighborhood evaluations.
Affective computing is an arising interdisciplinary exploration field uniting specialists and professionals from different fields, going from artificial intelligence (AI), NLP, to psychological and sociologies [17] [20]. With theexpansion of recordings posted on the web for product surveys, film audits, political perspectives, and affective computing research has progressively advanced from customary unimodal investigation to more perplexing types of multimodal examination. This is the essential inspiration driving first of its sort, thorough writing survey of the assortedfield of affective computing. Besides, existing writing overviews come up short on a point by point conversation of best in class in multimodal influence investigation structures, which this audit intends to address. In this paper, they centeredfor the most part around the utilization of sound, visual and text data for multimodal influence examination. Following an outline of various strategies for unimodal influence examination, they diagram existing techniques for intertwiningdata from various modalities.
A real time quantum conscious multimodal option mining framework using … (Jamuna S Murthy) 1021 elements from start to finish. The approach is customized for the unpredictable idea of communicating in language in online recordings just as going with motions and voice. In the investigations, their model beats cutting edge approaches for both multimodal and unimodal supposition examination.
The proposed Making use of the quantum speculation ceremoniousness and the long short term memory (LSTM) designing, we put forward a novel and extensive quantum like multiple modal network structure, which commonly replicate the intra and inter articulation cooperation components by getting the associations betwixt various modalities and reasoning powerful effects among expounders. Regardless, the quantum conscious multimodal option mining framework (QMF) eliminates and addresses multiple modal highlights for all articulations in a solitary video utilizing a dense network based convolutional neural network (CNN) subnetwork and acknowledges them as data sources. Second, enlivened by the quantum estimation hypothesis, the QMF presents a solid feeble impact replica to quantify the impacts amid expounders across expressions and caters the subsequent impact grids into the QMF by fusing them into the yield door of every LSTM constituent. Third, with printed and visual highlights as information sources, the QMF utilizes 2 LSTM organizations to acquire their secret conditions, which are taken care of to the softmax capacities to get the neighborhoodslant examination results. At long last, a multimodal choice combination approach motivated by quantum obstruction is intended to infer an ultimate conclusion dependent on the neighborhood results. We have planned and done broad tests on two generally utilized conversational supposition datasets to exhibit the adequacy of the proposed QMF structure in examination with a wide scope of baselines, a component level combination approach and a choice level combination approach, and five best in class multimodal slant investigation models. The outcomes show that the QMF fundamentallyoutflanks this load of relative models. The significant developments of the work introduced in this are summed up: A quantum like multiple modal network structure, which uses the quantum likelihood hypothesis inside the LSTM engineering, to demonstrate both intra and inter expression collaboration elements for multimodal conclusion examination in discussions. A quantum obstruction propelled multimodal choice combination strategy to show the choice relationshipsbetween various modalities. Quantum estimation roused solid frail impact model to improve derivations about friendly impact among speakers than with past techniques. The proposed engineering for this framework is given above in Figure 1. It shows the manner in which this framework is planned and brief working of the framework. In the above figure of enthusiastic dataset is multiparty conversational slant examination dataset named interactive emotional dyadic motion capture (IEMOCAP), multimodal emotional lines dataset (MELD) and audio speech emotion This dataset is the biggest so far delivered for the expanded information with testing, preparing, and approval Applying arrangement calculation is profound learning CNN and LSTM with recurrent neural network (RNN) algorithm to characterize the presentation of enthusiastic outcome, after that preparation boundaries to track down the exact passionate classes execution of result check and get the end product like conversational opinion resultare constant perceive the distinctive enthusiastic with appropriate group of deep learning (DL) technique to precision score and execution methodology. In our work we are utilizing a few modules
Int J Artif Intell ISSN: 2252 8938
2. METHOD
Figure 1. Architecture of quantum conscious multimodal option mining framework
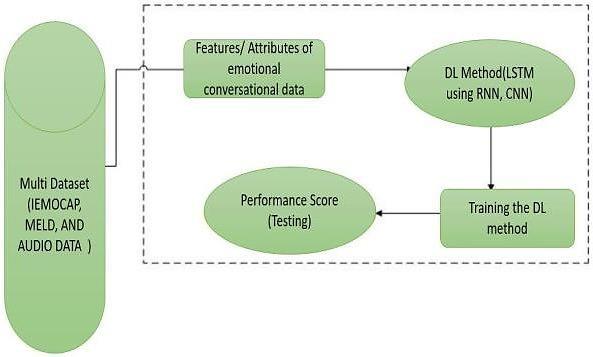
Multiple modal sentiment examination of discussionsis another region; the criterion datasets are somewhat restricted. We investigate the MELD1 and IEMOCAP2 datasets. MELD holds 13,708 expressions from 1433 exchanges of TV sequences. The expressions in every exchange are explained with one of three conclusions and one of seven feelings. The expressions in MELD are multiple modal, including sound and visual just as text based data. In this, we just utilize text based and visual data. IEMOCAP is a multiple modal data set of 10 expounders engaged with two way dyadic discussions. Every expression is explained utilizing one of the accompanying feeling classes: outrage, bliss, pity, unbiased, energy, disappointment, dread, shock, or others. We think about the initial four classifications and appoint different feelings to the fifth class to contrast our QMF and other cutting edge baselines in a reasonable way. One more dataset utilized Audio feeling discourse.
2.2. Feature selection and reduction From among the all ascribes of the informational collection are separated, all highlights of video edge and sound record utilizing image processing strategy asreferenced, a few DL procedures are utilized specifically, CNN, LSTM and RNN to extricate the highlights of all information documents. The examination was rehashed with all the DL procedures utilizing all credits.
2.1 Dataset collection and data pre-processing
Veracity is characterized as the grade of revisions. Since our methodology and baselines are regulated assumption investigation strategies, we embrace the exactness, review, F1 score, and precision as the assessment measurementsto assess the characterization execution of every strategy. Convolutional Neural Networks were utilized to fulfil certain evolution yield and secure remarkable challenges. The utilization of Convolutional layers embraces convolving an indication or a sketch with portions to acquire includesmaps. In this way, a unit in a component map is associated with the past layer through the loads of the parts. The loads of the bits are adjusted during the preparation stage by backproliferation, to upgrade certain qualities of the info. Since the portions are divided between all units of a similar elementmap, Convolutional layers have less loads to prepare than thickFC layers, making CNN simpler to prepare and less inclined toover fitting. Besides, since a similar bit is convolved over the entire picture, a similar element is recognized freely of the finding interpretation invariance. By utilizing pieces, data of the area is considered, which a helpful wellspring of setting data is. Generally, a non straight actuation work is applied on theyield of each neural unit. In the event that we stack a few Convolutional layers, the removed highlights become moretheoretical with the expanding profundity [24], [25]
A few quality demonstration quantifications like veracity, validity and blunder in arrangement are examined for the computation of implementation viability of this replica. Veracity in the present situation would mean the grade of occurrences effectively foreseeing from among every one of the attainable cases.
2.1.1 Dataset collection
This is a training graph that illustrates the training and testing loss, with the blue line denoting the training loss and the orange line represents the testing loss.
ISSN: 2252 8938 Int J Artif Intell, Vol. 11, No. 3, September 2022: 1019 1025 1022
The output for the training graph, which has 7560 training samples and an epoch value of 50, is shown in Figure 2 which turns out to provide training accuracy of 99.98% and testing accuracy as 98.81%.
2.1.2. Data pre processing For the picture data, the excessively huge pictures arere scaled to 360∗640. For literary data, we polish every writing by inspecting for unintelligible characters and revising spelling botches naturally. The stop terms are eliminated utilizing a grade prevent word list from Python's natural language toolkit (NLTK) bundle. We don't sift through the accentuation marks as certain accentuation marks, for example, question marks and interjection focuses, will in general convey abstract data. We run the tests utilizing five crease cross approval on every one of the similar models.
3. RESULTS AND DISCUSSION
Figure 3 shows the test results; it is built by taking into consideration 10 different datasets and printing the predicted and test values. The confusion matrix, sometimes known as the error matrix, is shown in Figure 4. It displays the algorithm's overall performance of specificity, accuracy, and precision of actual and forecasted values. The darker blue represents a significant correlation, while the lighter blue indicates a good affiliation. This is the final output shown in Figure 5, which indicates a 98% overall accuracy, along with precision, recall, f1 score, overall support.
Figure 4. Confusion matrix Figure 5. Over all classification accuracy
4. CONCLUSION
Conversational sentiment analysis is a significant and testing task. We proposed a “Quantum Conscious Multimodal Option Mining Framework”, which uses the numerical ceremoniousness of quantum hypothesis and a long momentary memory organization, to show both intra and inter expression connection elements and perceive expounders' feelings. The principle thought is to utilize a density matrix based CNN, a quantum estimation enlivened solid frail impact model and a quantum obstruction motivated multimodal choice combination approach. The exploratory outcomes on the MELD and IEMOCAP datasets show that our suggested QMF generally beats a broad scope of guidelines and best in class multimodal conclusion examination calculations, in this way confirming the adequacy of utilizing quantum hypothesis formalisms to demonstrate inter expression connection, the combination of multimodal substance and the combination of nearby choices. Since this QMF model is so based on the density matrix representation, in future work we
Int J Artif Intell ISSN: 2252 8938 A real time quantum conscious multimodal option mining framework using … (Jamuna S Murthy) 1023 Figure 2. Training and testing graph of dataset Figure 3. Testing performance of dataset
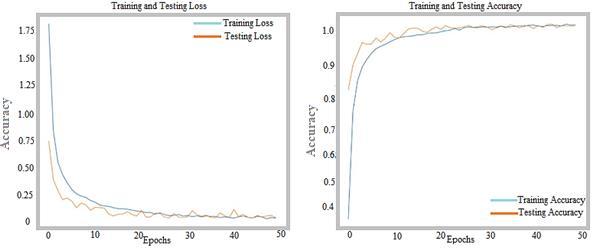
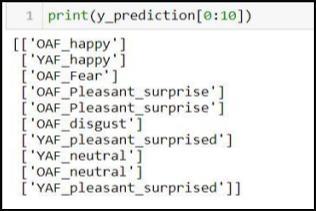
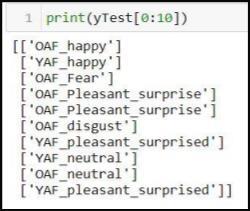
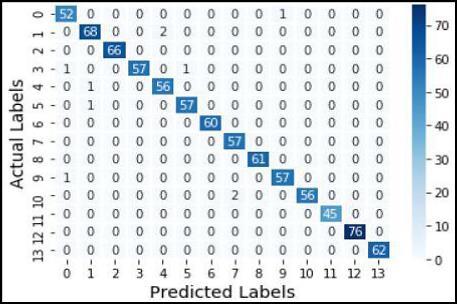
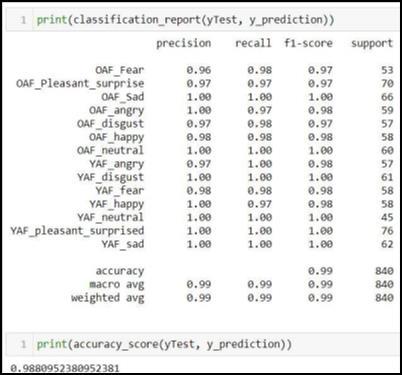
[1] E. Cambria, “Affective computing and sentiment analysis,” IEEE Intell. Syst., vol. 31, no. 2, pp. 102 107, Mar. 2016, doi: 10.1109/MIS.2016.31.
[22] Y. Qian, Y. Zhang, X. Ma, H. Yu, and L. Peng, “EARS: Emotion aware recommender system based on hybrid information fusion,” Inf. Fusion, vol. 46, pp. 141 146, Mar. 2019, doi: 10.1016/j.inffus.2018.06.004.
[12] E. Cambria, S. Poria, A. Gelbukh, and M. Thelwall, “Sentiment analysis is a big suitcase,” IEEE Intell. Syst., vol. 32, no. 6, pp. 74 80, Nov. 2017, doi: 10.1109/MIS.2017.4531228. [13] S. Kumar, M. Yadava, and P. P. Roy, “Fusion of EEG response and sentiment analysis of products review to predict customer satisfaction,” Inf. Fusion, vol. 52, pp. 41 52, Dec. 2019, doi: 10.1016/j.inffus.2018.11.001.
REFERENCES
[2] M. Dragoni, S. Poria, and E. Cambria, “OntoSenticNet: A commonsense ontology for sentiment analysis,” IEEE Intell. Syst., vol. 33, no. 3, pp. 77 85, May 2018, doi: 10.1109/MIS.2018.033001419.
[6] S. Poria, E. Cambria, N. Howard, G. Bin Huang, and A. Hussain, “Fusing audio, visual and textual clues for sentiment analysis from multimodal content,” Neurocomputing, vol. 174, pp. 50 59, Jan. 2016, doi: 10.1016/j.neucom.2015.01.095.
[23] A. Zadeh, M. Chen, S. Poria, E. Cambria, and L. P. Morency, “Tensor fusion network for multimodal sentiment analysis,” in Proceedings of the 2017 Conference on Empirical Methods in Natural Language Processing, 2017, pp. 1103 1114, doi: 10.18653/v1/D17 1115. [24] R. Girshick, “Fast R CNN,” in Proceedings of the IEEE International Conference on Computer Vision, Dec. 2015, vol. 2015 Inter, pp. 1440 1448, doi: 10.1109/ICCV.2015.169.
[18] S. Poria, D. Hazarika, N. Majumder, G. Naik, E. Cambria, and R. Mihalcea, “MELD: A multimodal multi party dataset for emotion recognition in conversations,” in Proceedings of the 57th Annual Meeting of the Association for Computational Linguistics, 2019, pp. 527 536, doi: 10.18653/v1/P19 1050. [19] B. Ojamaa, P. K. Jokinen, and K. Muischnek, “Sentiment analysis on conversational texts,” in Proceedings of the 20th Nordic Conference of Computational Linguistics, NODALIDA 2015, 2015, pp. 233 237. [20] J. Bhaskar, K. Sruthi, and P. Nedungadi, “Hybrid approach for emotion classification of audio conversation based on text and speech mining,” Procedia Comput. Sci., vol. 46, pp. 635 643, 2015, doi: 10.1016/j.procs.2015.02.112. [21] X. Liu, Y. Xu, and F. Herrera, “Consensus model for large scale group decision making based on fuzzy preference relation with self confidence: Detecting and managing overconfidence behaviors,” Inf. Fusion, vol. 52, pp. 245 256, Dec. 2019, doi: 10.1016/j.inffus.2019.03.001.
[11] Q. Yang, Y. Rao, H. Xie, J. Wang, F. L. Wang, and W. H. Chan, “Segment level joint topic sentiment model for online review analysis,” IEEE Intell. Syst., vol. 34, no. 1, pp. 43 50, 2019, doi: 10.1109/MIS.2019.2899142.
[3] M. Soleymani, D. Garcia, B. Jou, B. Schuller, S. F. Chang, and M. Pantic, “A survey of multimodal sentiment analysis,” Image Vis. Comput., vol. 65, pp. 3 14, Sep. 2017, doi: 10.1016/j.imavis.2017.08.003. [4] J. A. Balazs and J. D. Velásquez, “Opinion mining and information fusion: A survey,” Inf. Fusion, vol. 27, pp. 95 110, Jan. 2016, doi: 10.1016/j.inffus.2015.06.002.
[25] K. He, G. Gkioxari, P. Dollar, and R. Girshick, “Mask R CNN,” in Proceedings of the IEEE International Conference on Computer Vision, Oct. 2017, vol. 2017 Octob, pp. 2980 2988, doi: 10.1109/ICCV.2017.322.
[14] C. Welch, V. Perez Rosas, J. K. Kummerfeld, and R. Mihalcea, “Learning from personal longitudinal dialog data,” IEEE Intell. Syst., vol. 34, no. 4, pp. 16 23, Jul. 2019, doi: 10.1109/MIS.2019.2916965.
ISSN: 2252 8938 Int J Artif Intell, Vol. 11, No. 3, September 2022: 1019 1025 1024cansee how we will go even farther in accurately capturing speaker interactions and naturally incorporating them into an end to end architecture.
[10] V. Perez Rosas, R. Mihalcea, and L. P. Morency, “Multimodal sentiment analysis of spanish online videos,” IEEE Intell. Syst., vol. 28, no. 3, pp. 38 45, May 2013, doi: 10.1109/MIS.2013.9.
[5] I. Chaturvedi, E. Cambria, R. E. Welsch, and F. Herrera, “Distinguishing between facts and opinions for sentiment analysis: Survey and challenges,” Inf. Fusion, vol. 44, pp. 65 77, Nov. 2018, doi: 10.1016/j.inffus.2017.12.006.
[9] Y. Wang, C. von der Weth, Y. Zhang, K. H. Low, V. K. Singh, and M. Kankanhalli, “Concept based hybrid fusion of multimodal event signals,” in 2016 IEEE International Symposium on Multimedia (ISM), Dec. 2016, pp. 14 19, doi: 10.1109/ISM.2016.0013.
This research was supported by Ramaiah Institute of Technology (MSRIT), Bangalore 560054 and Visvesvaraya Technological University, Jnana Sangama, Belagavi 590018.
[15] S. Poria, N. Mazumder, E. Cambria, D. Hazarika, L. P. Morency, and A. Zadeh, “Context dependent sentiment analysis in user generated videos,” in ACL 2017 55th Annual Meeting of the Association for Computational Linguistics, Proceedings of the Conference (Long Papers), 2017, vol. 1, pp. 873 883, doi: 10.18653/v1/P17 1081. [16] D. Hazarika, S. Poria, A. Zadeh, E. Cambria, L. P. Morency, and R. Zimmermann, “Conversational memory network for emotion recognition in dyadic dialogue videos,” in NAACL HLT 2018 2018 Conference of the North American Chapter of the Association for Computational Linguistics: Human Language Technologies Proceedings of the Conference, 2018, vol. 1, pp. 2122 2132, doi: 10.18653/v1/n18 1193. [17] S. Poria, E. Cambria, R. Bajpai, and A. Hussain, “A review of affective computing: From unimodal analysis to multimodal fusion,” Inf. Fusion, vol. 37, pp. 98 125, Sep. 2017, doi: 10.1016/j.inffus.2017.02.003.
[7] S. Poria, N. Majumder, D. Hazarika, E. Cambria, A. Gelbukh, and A. Hussain, “Multimodal sentiment analysis: Addressing key issues and setting up the baselines,” IEEE Intell. Syst., vol. 33, no. 6, pp. 17 25, Nov. 2018, doi: 10.1109/MIS.2018.2882362.
[8] P. Zhang, Z. Su, L. Zhang, B. Wang, and D. Song, “A quantum many body wave function inspired language modeling approach,” in International Conference on Information and Knowledge Management, Proceedings, Oct. 2018, pp. 1303 1312, doi: 10.1145/3269206.3271723.
ACKNOWLEDGEMENTS
Dr. Siddesh G M is currently working as Associate professor in Department of Information Science and Engineering, M S Ramaiah Institute of Technology, Bangalore. He is the recipient of Seed Money to Young Scientist for Research (SMYSR) for FY 2014 15, from Government of Karnataka, Vision Group on Science and Technology (VGST). He has published a good number of research papers in reputed International Conferences and Journals. His research interests include Internet of Things, Distributed Computing and Data Analytics. He can be contacted at email: siddeshgm@gmail.com. Srikrishna V is a 2021 Information Science and Engineering graduate from B.N.M. Institute of Technology, Bengaluru. His areas of interest include Sentiment Analysis, Natural Language Processing, and Computer Vision under Machine Learning. Currently, Srikrishna is working as a server side developer at Altimetrik India Private Limited. He can be contacted at email: srikihv@gmail.com.
Int J Artif Intell ISSN: 2252 8938 A real time quantum conscious multimodal option mining framework using … (Jamuna S Murthy) 1025
Kedarnath R Gubbi has graduated from B.N.M. Institute of Technology in the field of Information science and engineering. His area of interest includes Sentiment Analysis and Natural Language Processing and is currently working as a front end web developer at NEC Technologies India Pvt. Ltd. He can be contacted at email: kedarnathrg.1999@gmail.com.
Jamuna S Murthy holds a Masters degree from M S Ramaiah Institutte of Technology, India in 2017. She is currently a Resaerch Scholar at M S Ramaiah Institute of Technology and currently working as an Assistant Professor in Department of ISE at B N M Institute of Technology, Bengaluru. Her research interests include Machine Learning, Natural Language Processing, Bigdata and Cloud Computing. She can be contacted at email: jamunamurthy.s@gmail.com.
BIOGRAPHIES OF AUTHORS
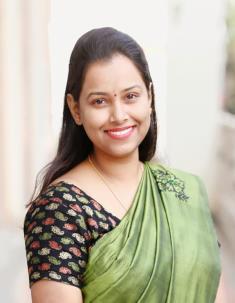



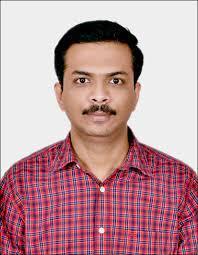



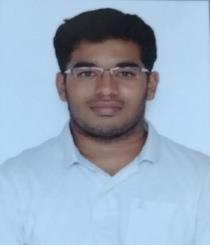



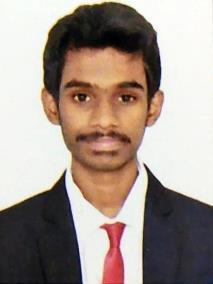



Article history: Received Oct 21, 2021 Revised Jun 9, 2022 Accepted Jun 18, 2022 Prediction of electrical load is important because it relates to the source of power generation, cost effective generation, system security, and policy on continuity of service to consumers. This paper uses Indonesian primary data compiled based on data log sheet per hour of transmission operators. In preprocessing data, detrending technique is used to eliminate outlier data in the time series dataset. The prediction used in this research is a long short term memory algorithm with stacking and time step techniques. In order to get the optimal one day forecasting results, the inputs are arranged in the previous three periods with 1, 2, 3 layers, 512 and 1024 nodes. Forecasting results obtained long short term memory (LSTM) with three layers and 1024 nodes got mean average percentage error (MAPE) of 8.63 better than other models.
Indonesian load prediction estimation using long short term memory Erliza Yuniarti1,4 , Siti Nurmaini2 , Bhakti Yudho Suprapto3 1Doctoral Program of Engineering Science, Faculty of Engineering Universitas Sriwijaya, Palembang, Indonesia
4Department of Electrical Engineering, Faculty of Engineering, Universitas Muhammadiyah Palembang, Palembang, Indonesia
Keywords: OneLLoadDetendringforecastingongshorttermmemorydayforecasting
2Intellignet Research Group, Universitas Sriwijaya, Palembang, Indonesia
IAES International Journal of Artificial Intelligence (IJ AI) Vol. 11, No. 3, September 2022, pp. 1026 1032 ISSN: 2252 8938, DOI: 10.11591/ijai.v11.i3.pp1026 1032 1026
Article Info ABSTRACT
3Department of Electrical Engineering, Universitas Sriwijaya, Palembang, Indonesia
1. INTRODUCTION
Corresponding Author: Erliza DoctoralYuniartiProgram
Electrical load forecasting in the electric power industry is important because it relates to the management of generating sources, cost effective power system planning, system security, customer service and policy making [1], [2]. Electrical load forecasting research is based on past load patterns [3] which may reappear in the future. Load forecasting can be classified based on the length of the horizon and the method used. Load forecasting can be classified based on the length of the horizon and the method used [4]. Based on the length of the forecasting horizon [5], [6] long term forecasts of 1 year or more, medium term 1 week to 1 year, and short term 1 hour to 1 week. Based on the load forecasting method, the statistical method is used with a mathematical approach based on correlation regression, while the machine learning and deep learning methods are built based on the training and testing process to train and test the performance of the model. The advantage of the deep learning model is that it is more efficient to train complex neural networks, with generalization capabilities that increase accuracy around 20 45% [7]. Among the three learning methods, deep learning techniques are the most successful methods in the field of image, text and data mining [8] Several studies using deep learning methods for load forecasting include using the convolutional neural network (CNN) neural network method, where the CNN layer is used to extract features from historical loads in homogeneous residential load clustering [9], [10]. Recurrent neural network (RNN) architecture in load forecasting supports non stationary discrete time input signals, making RNNs more suitable for sequential or sequential data [11] [13]. Long short term memory (LSTM) by combining
Journal homepage: http://ijai.iaescore.com
This is an open access article under the CC BY SA license.
of Engineering Science, Faculty of Engineering, Universitas Sriwijaya St. Palembang Prabumulih KM. 32 Kabupaten Ogan Ilir, Sumatera Selatan, Indonesia Email: erlizay69@gmail.com
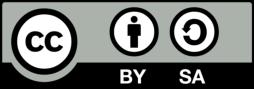
The research in this paper proposes to predict the short term load one day ahead with the stacking technique and the LSTM time step which is used for planning operations at the load control center one day ahead. This study uses a primary load dataset of Indonesia’s electrical energy consumption, which until now is still limited. The dataset is compiled from PT PLN’s daily logsheet based on the records of the transmission system operator every hour, for five years, 2013 2017. In preliminary research [24] our validation shows that LSTM based forecasting models outperform other alternative approaches. Based on this preliminary research, we developed an LSTM algorithm by setting the optimal lag time and time step which is integrated with 3 layers LSTM stacking. To get the predictive performance of the electrical load, it is measured using the mean absolute error (MAE) and the mean absolute percentage error (MAPE). The rest of this article is structured: Part 2 of the research methodology, provides an overview of building prediction techniques that we propose. Section 3 describes the experimental results, validation and comparing with other LSTM models and deep learning models. Section 4 draws conclusions from the research that has been done.
2. RESEARCH METHOD
To find out the pattern of electricity consumption contained in this dataset, it is shown in Figures 1 3, the graph depicts the electricity load per day, per month and per year. Due to it is sequentially every hour, the total data in each year consists of 365 days or 8670 data. The daily load profile for 24 hours is shown in Figure 1, load consumption has an upward trend starting from 08.00 hours to its peak at
Int J Artif Intell ISSN: 2252 8938 Indonesian load prediction estimation using long short term memory (Erliza Yuniarti) 1027 short term memory with long term memory, through gate control prevents signal loss during the prediction process, so as to a better accuracy [14] [16]. Gate recurrent unit (GRU) is applied in the demand side energy forecasting which is still limited [17], predict electrical power load [18], shows the prediction performance of GRU is still lower than LSTM, but better than traditional models. Modeling in particular with time series data sets using the LSTM technique is quite popular for solving complex sequence models such as electrical loads, by studying long term dependencies and tracing patterns that occurred far in the past. Several studies using the LSTM algorithm have been developed for short term electrical load forecasting and become the best algorithm for prediction for time series data [5], [16], [19] [22]. According to [16], [21] the vital problem in forecasting models using the LSTM structure is to choose the right sequence of lag times along with the right hyperparameter model for certain time series data. Electric load prediction based on one day ahead [19] using supervised learning k Means technique for the classification of consumer features based on the time sequence, namely adjacent times; adjacent day; and the same day of the adjacent week. The experimental result [19], [23] shows that the hyperparameter tuning of variations in the number of nodes in the parallel layer LSTM shows good accuracy performance, and overall LSTM performance can find energy consumption patterns from consumers. Kwon et al. [5] applying the short term forecasting method by extracting features from historical data, with the separation of features, namely the day of the week, the average load of the last two days, the hourly load and the hourly temperature so as to get good accuracy for forecasting the electricity load one day ahead in Korea. The recent research [20] uses a half hour period data set from 2008 2016 from metropolitan France for one day forecasting. Bouktif et al. [20] discusses the optimal configuration of the LSTM model by tuning the hyperparameter of the number of layers, the number of neurons in each layer, lag time, batch size, and type of activation so as to reduce the complexity of the dataset used. Abdel Nasser and Mahmoud [22] comparing the basic LSTM architecture, LSTM with sliding windows technique, LSTM with time steps and stacked LSTMs with other benchmark models in modeling temporal changes in photo voltaic (PV) output power per hour in Egypt. The architecture of all LSTMs outperforms benchmark models for one hour predictions ahead, and LSTM architectures with time steps get the lowest error compared to all other LSTM architectures.
Forecasting this electrical load uses sequential data, which is related to past values. Past data on the use of electrical energy represents trends and load patterns as well as anomalies that occur. An exploratory analysis of the electrical load time series can be useful for identifying trends, patterns, and anomalies. Consumption patterns are grouped into daily, monthly and yearly electrical load consumption so that a graphical correlation can be seen between time and electricity usage.
2.1. Data preparation and preprocessing
2.1.1 Dataset
In this section, a short term forecasting methodology for the next one day will be explained using the LSTM algorithm. We propose a three step framework, namely, dataset preparation and preprocessing, LSTM algorithm construction and comparison machine learning (ML) algorithm, LSTM algorithm training and validation. In the following, we present an overview of the methodology, and an explanation for each component of the methodology in detail from the Indonesian electricity consumption dataset in this case study.
Figure 1. Twenty four hour electrical load
Data pre processing The dataset used in this study comes from Indonesian data from 2013 2017, from operator data recording of the high voltage overhead line transmission system. In research using univariant datasets, we
4. The used of three historical data period to forcast one next period 2.1.2
ISSN: 2252 8938 Int J Artif Intell, Vol. 11, No. 3, September 2022: 1026 1032 102811.00 15.00 hours and decreases again along with reduced population activity. The peak load occurred at 18.00 20.00 with an increase in the load in housing and industry consisting of lighting and electronic equipment (65% of the load was housing load). Load consumption was reduced until the next following morning. From Figure 2, the hourly electricity consumption for one month obtained a lot of load outlier data in a few hours, this anomaly causes weather or humam errors, and will be overcome by preprocessing the load before being used as input for the maching learning model. In addition, Figure 3 illustrate the electrical consumption for one year. It infers that in the beginning of year, the electrical load slightly fluctuates. However, in the next following segment (middle to end of year) the electrical consumption relatively steady.
Figure 2. Monthly daily electrical load Figure 3. Yearly electrical load Forecasting electrical load data in this study is a short term forecast. The data used is the electrical load per hour on the same day. For example, to predict the electrical load on Monday, the model reference data from the previous Monday. In order to compose the input data using historical value, the previous three periods was used. The use of too much historical data can cause multicollinearity problems so that the prediction results of the model become less sensitive. Figure 4 shows the process of data reshaping as input for the LSTM model.Figure
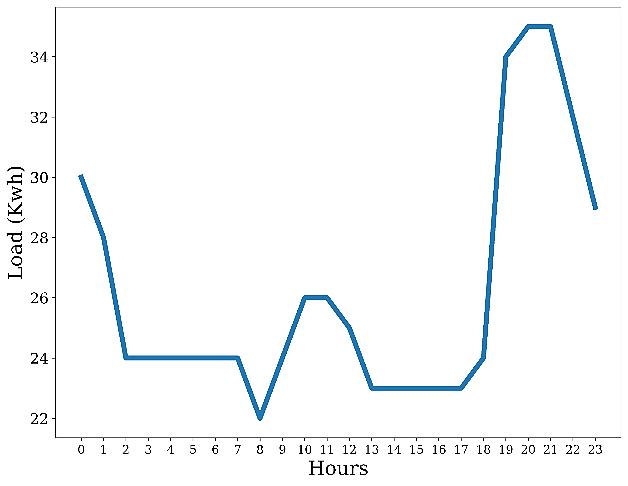
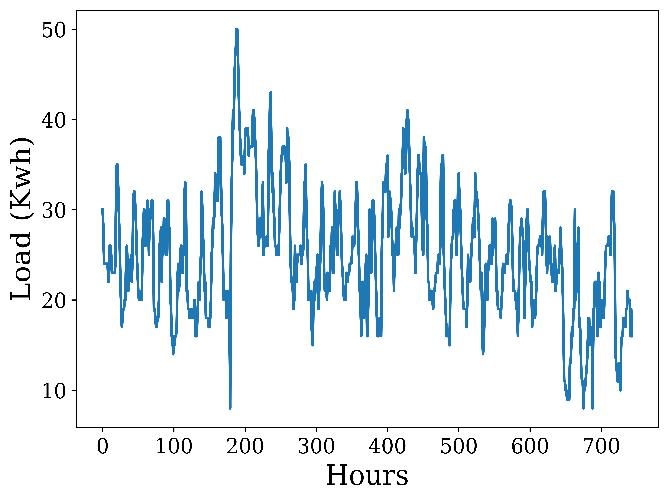

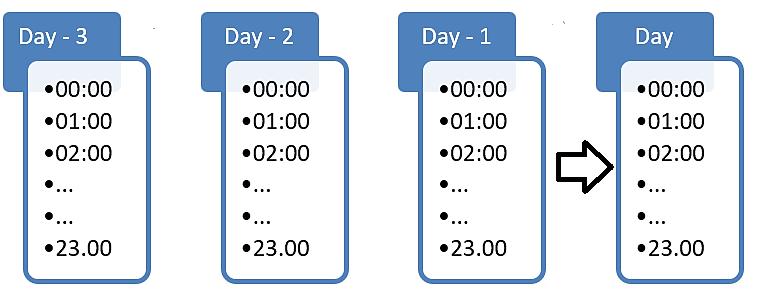
Indonesian load prediction estimation using long short term memory (Erliza Yuniarti) 1029
only use active power (MW) i.e. power usage or electricity consumption and reduce other data in the transmission operator’s records. Furthermore, data pre processing is consists of imputing missing value, detrending to remove distortion in the form of an increase or decrease from normalized time series data. Detrending is a process that aims to remove trends from the time series. The trend usually refers to the average change over time. When performing the detrending process, aspects that may distort the data will be removed. Distortion in time series data can be seen as fluctuations in time series graphs. By eliminating the distortion, the graph of the increase or decrease of the time series data can be seen. Figure 5(a) illustrate the raw data which contain an extreme fluctuation and may distor the training process. Thus, a detendring process was performed which illustrated in Figure 5(b). In this study normalization changes the feature range into the range [ 1, 1] because this range is suitable for use on data that has outliers. The results of the normalization stage are carried out in a pre processing stage, which is followed by data management, which includes data transformation and data splitting into training and testing data. Training and testing data are separated using an 80/20 ratio for training and testing respectively [25]
Figure 5. Illustrate (a) observed data (b) detendring result
(b)(a)
Int J Artif Intell ISSN: 2252 8938
To measure the model’s performance, an error rate was calculated using the MAPE. The lower the error rate, the higher the data accuracy. MAPE provides results in the form of absolute percentage averages with forecast errors compared to actual data.
2.2. Research methodology In this study, the electrical load forecasting process was carried out using the deep learning method. The deep learning method used is RNN with LSTM architecture. The LSTM network is a development of the RNN architecture. RNN architecture is a network that works for sequence or time series data by considering the output (information) of the previous process and storing the information for a short time (short term memory).The LSTM architecture accepts input in the form of an Xt feature, where this feature is the value of the electrical load. This feature is then entered into the LSTM block for processing. The LSTM block receives input ht 1 (hidden state from the previous LSTM block), Ct 1 (Cell state from the previous LSTM block), and Xt (feature input). The LSTM block also has outputs in the form of h¬t and Ct, namely the current hidden state and the current cell state (memory). In addition, in the LSTM block there are sigmoid and tanh activation functions, which act as input gates, forget gates and output gates. Several parameters are used to tune the LSTM model, such as the number of nodes and hidden layers. Figure 6 shows the research methodology of this research.
2.3 Evaluation metrics
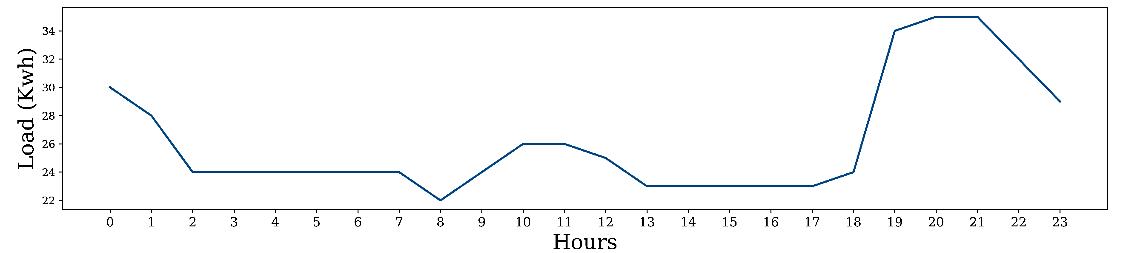
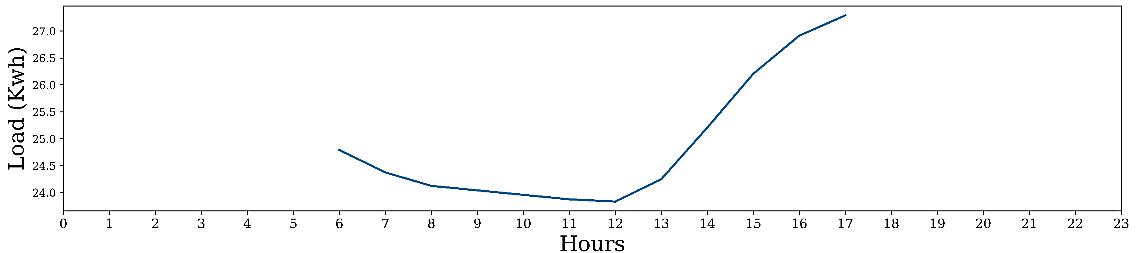
ISSN: 2252 8938 Int J Artif Intell, Vol. 11, No. 3, September 2022: 1026 1032 1030 �������� = 1 ��∑���� �� ̌ �� ���� �� ��=1 ��100 Where, N is number of samples, Yi is actual value, and �� ̌ �� is prediction value. Figure 6. Research methodology
In this study, testing was carried out with the distribution of the dataset of 80:20 for training data and validation data. Each dataset is trained using the LSTM model. The parameters used in this study include the number of hidden layers, the number of hidden nodes, the batch size of 4, epoch 100 and using the ADAM optimizer optimization method.
Table 1. LSTM one layer evaluation result Node MAPE Parameters 512 9.37 1,053,185 1024 9.29 4,207,617
3.1. LSTM 1 layer
Table 2. LSTM two layers evaluation result Node MAPE Parameters 512 9.28 3,156.481 1024 9.04 12,604,417
3.2. LSTM 2 layers In building 2 layers LSTM architecture, two LSTM blocks are stacked into one, thus forming a stack of LSTM blocks. The 2 layer LSTM architecture does not have much difference in the 1 layer LSTM architecture. Consists of 512 and 1024 hidden units, with Adam optimizer, and loss function MSE. The number of epochs and batch size for this layer is still the same, namely 100 and 4. Then the units in the input and output layers are 1 unit. However, because the number of layers is more, the parameters generated in the LSTM 2 layers architecture are also increased to 3,156.481 parameters for 512 nodes and 12,604,417 for 1024 nodes. The results of the two layer LSTM test are shown in Table 2.
In LSTM architecture, layer 1 has 512 and 1024 hidden units, with Adam optimizer, and loss function mean squared error (MSE). The number of epochs for this layer is 100 with a batch size of 4. Units in the input and output layers are 1 unit. While the number of parameters generated in the LSTM 1 layer architecture is 1,053,185 and 4,207,617 parameters for 512 and 1024 respectively. The results of the single layer LSTM test are shown in Table 1.
3. RESULTS AND DISCUSSIONS
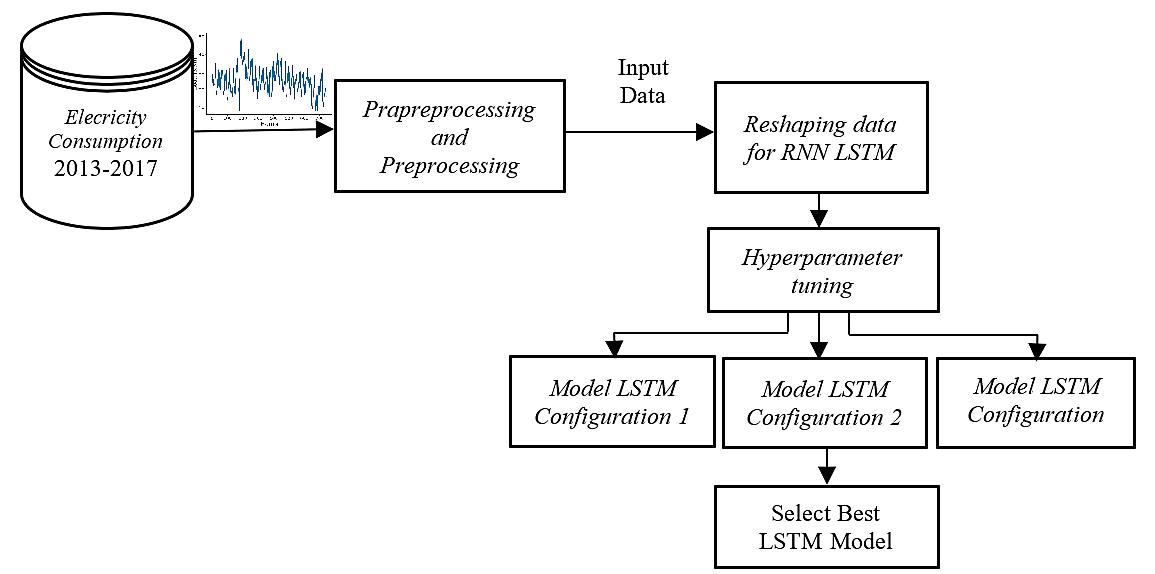
[3] H. Shi, M. Xu, and R. Li, “Deep learning for household load forecasting a novel pooling deep RNN,” IEEE Transactions on Smart Grid, vol. 9, no. 5, pp. 5271 5280, Sep. 2018, doi: 10.1109/TSG.2017.2686012.
[7] G. Chitalia, M. Pipattanasomporn, V. Garg, and S. Rahman, “Robust short term electrical load forecasting framework for commercial buildings using deep recurrent neural networks,” Applied Energy, vol. 278, p. 115410, Nov. 2020, doi: 10.1016/j.apenergy.2020.115410.
Layers LSTM 1 Layers MAPE % TechniqueForecasting Comparison with Other Method (Lower is Better)
This study applies LSTM to predict the electrical load energy. Based on the experimental result the best architecture was LSTM three layers with 1024 nodes. It reaches 8.63 of MAPE value. The historical features used in this study was three previous period.
The 3 layers LSTM architecture is the same as the 2 layers LSTM architecture, but the number of stacks of LSTM blocks is 3 stacks. This architecture consists of 512 and 1024 hidden units, with the Adam optimizer, and the loss function MSE. The number of epochs and batch size for this layer is still the same, namely 100 and 4. Then the units in the input and output layers are 1 unit. However, because the number of layers is more, the parameters generated in this 3 layers LSTM architecture also increase to 5,257,729 parameters. The results of the three layer LSTM test are shown in Table 3 In order to evaluate the model’s ability to predict the forecasting pattern that has been carried out, we compared it with other models from the state of the art research [7] and [19], in the MAPE evaluation metrics which is summarized in Figure 7. Table 3. LSTM three layers evaluation result Node MAPE Parameters 512 9.13 5,257,729 1024 8.63 21,001,217 Figure 7. Comparison of MAPE value with other forecasting techniques (lower is better)
REFERENCES
4. CONCLUSION
[1] F. Li and G. Jin, “Research on power energy load forecasting method based on KNN,” International Journal of Ambient Energy, vol. 43, no. 1, pp. 946 951, Dec. 2022, doi: 10.1080/01430750.2019.1682041.
[2] Z. Wang, B. Zhao, H. Guo, L. Tang, and Y. Peng, “Deep ensemble learning model for short term load forecasting within active learning framework,” Energies, vol. 12, no. 20, p. 3809, Oct. 2019, doi: 10.3390/en12203809.
0 5 10 15
Encoder-Decoder[19][7]CNN-LSTM[7] LSTM 3 Layers LSTM
Int J Artif Intell ISSN: 2252 8938 Indonesian load prediction estimation using long short term memory (Erliza Yuniarti) 1031
[5] B. S. Kwon, R. J. Park, and K. B. Song, “Short term load forecasting based on deep neural networks using LSTM layer,” Journal of Electrical Engineering and Technology, vol. 15, no. 4, pp. 1501 1509, Jul. 2020, doi: 10.1007/s42835 020 00424 7.
[9] K. Aurangzeb, M. Alhussein, K. Javaid, and S. I. Haider, “A pyramid CNN based deep learning model for power load forecasting of similar profile energy customers based on clustering,” IEEE Access, vol. 9, pp. 14992 15003, 2021, doi: 10.1109/ACCESS.2021.3053069.
Other
[8] Y. Zhu, R. Dai, G. Liu, Z. Wang, and S. Lu, “Power market price forecasting via deep learning,” in IECON 2018 44th Annual Conference of the IEEE Industrial Electronics Society, Oct. 2018, pp. 4935 4939, doi: 10.1109/IECON.2018.8591581.
3.3. LSTM 3 layers
[10] Acharya, Wi, and Lee, “Short term load forecasting for a single household based on convolution neural networks using data augmentation,” Energies, vol. 12, no. 18, p. 3560, Sep. 2019, doi: 10.3390/en12183560.
[11] M. N. Fekri, H. Patel, K. Grolinger, and V. Sharma, “Deep learning for load forecasting with smart meter data: online adaptive 22.45 12.86 15.62 8.639.049.29 20 25 LSTM 2
[4] A. Ahmad, N. Javaid, A. Mateen, M. Awais, and Z. A. Khan, “Short term load forecasting in smart grids: an intelligent modular approach,” Energies, vol. 12, no. 1, p. 164, Jan. 2019, doi: 10.3390/en12010164.
[6] Z. Guo, K. Zhou, X. Zhang, and S. Yang, “A deep learning model for short term power load and probability density forecasting,” Energy, vol. 160, pp. 1186 1200, Oct. 2018, doi: 10.1016/j.energy.2018.07.090.
[12] A. Rahman, V. Srikumar, and A. D. Smith, “Predicting electricity consumption for commercial and residential buildings using deep recurrent neural networks,” Applied Energy, vol. 212, pp. 372 385, Feb. 2018, doi: 10.1016/j.apenergy.2017.12.051.
[13] L. Sehovac and K. Grolinger, “Deep learning for load forecasting: sequence to sequence recurrent neural networks with attention,” IEEE Access, vol. 8, pp. 36411 36426, 2020, doi: 10.1109/ACCESS.2020.2975738.
[22] M. Abdel Nasser and K. Mahmoud, “Accurate photovoltaic power forecasting models using deep LSTM RNN,” Neural Computing and Applications, vol. 31, no. 7, pp. 2727 2740, Jul. 2019, doi: 10.1007/s00521 017 3225 z. [23] W. Kong, Z. Y. Dong, Y. Jia, D. J. Hill, Y. Xu, and Y. Zhang, “Short term residential load forecasting based on LSTM recurrent neural network,” IEEE Transactions on Smart Grid, vol. 10, no. 1, pp. 841 851, Jan. 2019, doi: 10.1109/TSG.2017.2753802.
[20] S. Bouktif, A. Fiaz, A. Ouni, and M. A. Serhani, “Multi sequence LSTM RNN deep learning and metaheuristics for electric load forecasting,” Energies, vol. 13, no. 2, pp. 1 21, 2020, doi: 10.3390/en13020391.
[19] R. Jiao, T. Zhang, Y. Jiang, and H. He, “Short term non residential load forecasting based on multiple sequences LSTM recurrent neural network,” IEEE Access, vol. 6, pp. 59438 59448, 2018, doi: 10.1109/ACCESS.2018.2873712.
[16] X. Tang, Y. Dai, T. Wang, and Y. Chen, “Short‐term power load forecasting based on multi‐layer bidirectional recurrent neural network,” IET Generation, Transmission and Distribution, vol. 13, no. 17, pp. 3847 3854, Sep. 2019, doi: 10.1049/iet gtd.2018.6687.
[17] A. M. N. C. Ribeiro, P. R. X. do Carmo, I. R. Rodrigues, D. Sadok, T. Lynn, and P. T. Endo, “Short term firm level energy consumption forecasting for energy intensive manufacturing: a comparison of machine learning and deep learning models,” Algorithms, vol. 13, no. 11, p. 274, Oct. 2020, doi: 10.3390/a13110274.
[18] K. Ke, S. Hongbin, Z. Chengkang, and C. Brown, “Short term electrical load forecasting method based on stacked auto encoding and GRU neural network,” Evolutionary Intelligence, vol. 12, no. 3, pp. 385 394, Sep. 2019, doi: 10.1007/s12065 018 00196 0.
[24] E. Yuniarti, N. Nurmaini, B. Y. Suprapto, and M. Naufal Rachmatullah, “Short term electrical energy consumption forecasting using RNN LSTM,” in 2019 International Conference on Electrical Engineering and Computer Science (ICECOS), Oct. 2019, pp. 287 292, doi: 10.1109/ICECOS47637.2019.8984496.
[21] S. Bouktif, A. Fiaz, A. Ouni, and M. A. Serhani, “Single and multi sequence deep learning models for short and medium term electric load forecasting,” Energies, vol. 12, no. 1, p. 149, Jan. 2019, doi: 10.3390/en12010149.
[15] J. Lago, F. De Ridder, and B. De Schutter, “Forecasting spot electricity prices: Deep learning approaches and empirical comparison of traditional algorithms,” Applied Energy, vol. 221, no. January, pp. 386 405, Jul. 2018, doi: 10.1016/j.apenergy.2018.02.069.
[25] P. Dangeti, Statistics for machine learning: techniques for exploring supervised, unsupervised, and reinforcement learning models with python and R. 2017.
ISSN: 2252 8938 Int J Artif Intell, Vol. 11, No. 3, September 2022: 1026 1032 1032 recurrent neural network,” Applied Energy, vol. 282, p. 116177, Jan. 2021, doi: 10.1016/j.apenergy.2020.116177.
[14] U. Ugurlu, O. Tas, and U. Gunduz, “Performance of electricity price forecasting models: evidence from Turkey,” Emerging Markets Finance and Trade, vol. 54, no. 8, pp. 1720 1739, Jun. 2018, doi: 10.1080/1540496X.2017.1419955.
BIOGRAPHIES OF AUTHORS Erliza Yuniarti currently is a PhD student in Facult of Engineering, Sriwijaya University. She gets a bachelor degree from electrical engineering major, Universitas Sriwijaya and get a master degree from Engineering Systems, Renewable Energy study program from Gadjah Mada University in 2012. Her research interest is electrical load study, renewable energy, distribution system, grounding and deep learning. She can contact at email: erlizay@yahoo.com Siti Nurmaini is currently a professor in the Faculty of Computer Science, Universitas Sriwijaya. She was received her Master's degree in Control system, Institut Teknologi Bandung Indonesia (ITB), in 1998, and the PhD degree in Computer Science, Universiti Teknologi Malaysia (UTM), at 2011. Her research interest, including Biomedical Engineering, Deep Learning, Machine Learning, Image Processing, Control Systems, and robotics. She can contact at email: siti nurmaini@unsri ac.id Bhakti Yudho Suprapto was born on February 11th 1975 in Palembang (South Sumatra, Indonesia). He is a graduate of Srwijaya University of Palembang in major of electrical engineering. His post graduate and doctoral program is in Electrical Enginering of Universitas Indonesia (UI). He is an academic staff of Electrical Engineering of Sriwijaya University of Palembang as a lecture. He concerns in major of control and intelligent system He can contact at email: bhakti@ft.unsri.ac.id
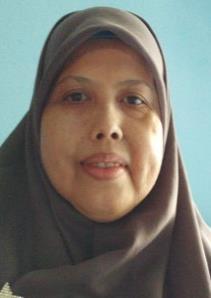



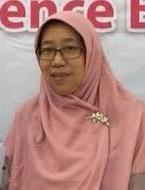



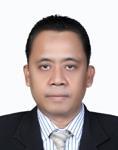



Binary spider monkey algorithm approach for optimal siting of the phasor measurement for power system state estimation
Article history: Received Aug 30, 2021 Revised Apr 22, 2022 Accepted May 21, 2022
Keywords: Binaryspider monkey
1. INTRODUCTION In power generation and distribution, the transmission and distribution network is crucial in transmitting electricity from power plants to customers. The power network provider must monitor and measure the different components of the power transmission network to avoid a loss of energy. Accurate measurement of the power system will provide more reliable and sustainable operation. Previously, supervisory control and data acquisition (SCADA) systems have been used to monitor the networks. The phasor measurement unit (PMU) is capable of measuring key network information including bus current, bus voltage, power angle, and generator speed, all using global position system (GPS) synchronized clocks. Operators in the control room may observe and evaluate the quality of the distribution network based on both dynamic and statistical operating circumstances by obtaining PMU measurement information from a wider area. Wide area monitoring system (WAMS) offers more advantages over SCADA in the form of better phasor measurement, increased sampling, and more precise measurement. The phasor measurement (PMUs) installation at all substations may greatly enhance the power network dependability, according to Phadke et al. [1], [2]. Regardless, the PMU device investment in all areas is economically undesirable owing to the high price of the device. By optimizing the quantity of PMU placement and using the full degree of observability, optimal PMU placement (OPP) is used to decrease maintenance fees and unit expenses. There
IAES International Journal of Artificial Intelligence (IJ AI) Vol. 11, No. 3, September 2022, pp. 1033 1040 ISSN: 2252 8938, DOI: 10.11591/ijai.v11.i3.pp1033 1040 1033
Journal homepage: http://ijai.iaescore.com
StatePhasorOptimalCompleteoptimizationobservabilityplacementmeasurementunitestimation
The phasor measurement unit (PMU) is an essential measuring device in current power systems. The advantage seems to be that the measuring system could simultaneously give voltages and currents phasor readings from widely dispersed locations in the electric power grid for state estimation and fault detection. Simulations and field experiences recommend that PMUs can reform the manner power systems are monitored and controlled. However, it is felt that expenses will limit the number of PMUs that will be put into any power system. Here, PMU placement is done using a binary spider monkey optimization (BSMO) technique that uses BSMO by simulating spider monkeys’ foraging behavior. Spider monkeys have been classified as animals with a fission fusion social structure. Animals that follow fission fusion social systems divide into big and tiny groups, and vice versa, in response to food shortage or availability. The method under development produced the optimum placement of PMUs while keeping the network fully observable under various contingencies. In the study published in IEEE14, IEEE24, IEEE30, IEEE39, IEEE57, and IEEE118, the proposed technique was found to reduce the number of PMUs needed.
This is an open access article under the CC BY SA license. Corresponding Author: Palepu Suresh Babu Department of Electrical and Electronics Engineering, Sri Venkateswara University College of Engineering Tirupati, Andhra Pradesh, 517502, India Email: sureshram48@gmail.com
Suresh Babu Palepu, Manubolu Damodar Reddy Department of Electrical and Electronics Engineering, Sri Venkateswara University College of Engineering, Tirupati, India Article Info ABSTRACT
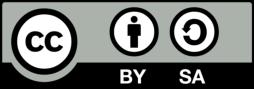
1034arethree
ISSN: 2252 8938 Int J Artif Intell, Vol. 11, No. 3, September 2022: 1033 1040 main types of OPP algorithms, and they may be broadly classified as deterministic, heuristic, and meta heuristic. The deterministic methods are implemented with the help of the mathematical programming method. The linear integer programming issue is the kind of problem where design variables can only take integer values. The strategic location of PMUs was addressed by Chen and Abur [3]. In Bei et al. the researchers suggested that PMUs be strategically placed for various budgets [4]. With respect to injection and power flow measurements, a specific form binary integer programming (BIP), a type of Integer linear programming (ILP), was employed to solve this problem. Additionally, the decision was made to sacrifice a single PMU to reduce the state estimation’s susceptibility to PMU failure. Some elements of PMU installation were discussed by Dua et al. [5]. Two indexes, via bus observability index (BOI) and system observability redundancy index (SORI), were developed in order to rate these numerous solutions even further. The optimal placement was made possible by using the capability of bus observability and zero injection and, therefore, the placement quality was enhanced by the use of BOI and SORI. In the Indian power grid’s Tamil Nadu State has benefited from the usage of ILP in determining the most advantageous PMU location, as it was demonstrated in [6]. The Chakrabarti and Kyriakides [7] suggested a binary search algorithm for determining the least quantity of PMU required. The Chakrabarti and Kyriakides [7] suggested a binary search algorithm for determining the least quantity of PMU required. A implicit data exclusion preprocessing technique [8] and a matrix decline algorithm were applied to make the placement form and the computational time smaller work required to determine the ideal placement set. In [9] proposes a heuristics based technique for ensuring a fully observable power system with the fewest feasible PMUs. According to Farsadi et al. [10], optimization techniques based on the sorting were employed to evaluate the lowest PMU required in IEEE57 bus and 14 bus systems. These graphs by Baldwin et al. [11] are a great way to build subgraphs that are measuring spanning measurements. The minimal spanning tree (MST) technique is adjusted depth first. By using the MST method, DFS is enhanced, which has rapid computation capabilities, and thus further enhances depth first search (DFS’s) weak and complicated convergence. Cai and Ai states this [12]. A superior approach to the heuristic method is meta heuristic. Meta heuristic search process uses intelligent approaches to find discrete variables and non continuous costs. In this study, we use the simulated annealing method, which has been implemented in [13], to determine the PMU location with respect to observability for the system. As it is used in [14], the modified simulated annealing (MSA) technique enables the search space to be much reduced, when compared to the simulated annealing (SA) method. The direct combination (DC) method heuristic rule is also used to decrease the searching spaces using the Tabu search technique. Simulated annealing has also been suggested by Abdelaziz et al. [15] for OPP. This method, which only requires a few stages, is very efficient in discovering optimum or near optimal solutions. Genetic algorithm (GA) is a natural selection inspired search technique. In [16], a genetic algorithm method for guaranteeing the smallest possible number and placement of PMUs involves calculating the lowest possible number of phasors measured.It is confirmed in [17] that the non dominated sorting algorithm (NSGA) reduces the quantity of PMUs and maximizes redundancy in measurement. Allagui et al. describe a method of monitoring network buses using implanted measuring devices in [18]. While flocking birds and schooling fish are social activity, Hajian et al. [19] statet that Drs. Eberhart and Kennedy developed particle swarm optimization (PSO) in 1995 as a socially influenced population based stochastic optimization approach. To calculate the optimal number of PMUs needed for complete observability, Hajian et al [19], Gao et al [20], Hajian et al [21], and Ahmadi et al. [22] used an adapted discrete binary version of the particle swarm optimization technique (BPSO). In [23], [24] used a similar approach like BPSO for location of PMUs in the improved binary flower pollination algorithm (IBFPA) algorithm. Binary search space only offers solutions with logic 0 or 1 values, and a new binary spider monkey optimization algorithm (BSMOA) is presented in this study based on [25] [27]. To optimize the placement of PMUs in the power system network for security, the basic SMO algorithm’s position updating equations have been altered using logical operators. This simplifies computation time, increases the robustness of the solution, and makes the solution applicable to other applications.
The ultimate focus of the PMU siting issue is to use the least quantity of PMUs needed. To attain total visibility of the power system while keeping total price to a minimum. The PMU Problem with a ‘m’ bus system is defined as shown in (1) Which includes cost of PMU installesd (1)
2. PROBLEM FORMULATION FOR OBSERVABILITY WITH OPTIMAL PMU SITING
��(��)=������∑ ���� �� ��=1 ∗����
An entry in the observability restraint vector function G(p) is non zero if the respective buses can be observed with regard to the specified measurement set, and zero otherwise. Vector constraint function provides observability of all network nodes in a complete manner. In order to fulfil the constraint, it is necessary to find a solution, that is, a minimal set of pi. In order to construct the restraint vector function, the binary connection matrix (AA) of the power system is used as input. It reflects the bus connection information of a power system, which may be derived from the line data of a power system’s underlying electrical network. The n mthelement of matrix AA corresponds to bus m and bus n is defined as: ������,�� ={1,������ =�� 1,����������′��′��������������������������′�� 0,�������� ′ (5) Let us consider IEEE 5 bus system as example shown in Figure 1 Figure 1 IEEE 5 bus test system Binary connectivity matrix (AA) for IEEE 5 bus test system is ���� = ( 11100 11111 11110 01111 01011) (6) For the system shown in Figure 1, the restraint vector task for the IEEE 5 bus test system was accomplished to achieve complete observability by (7) ��(��)=[��1��2��3��4��5]�� =��∗�� (7) where for buses ������1:��5 +��1 +��2 ≥1 ������2:��5 +��4 +��2 +��3 +��1 ≥1 ������3:��4 +��2 +��3 ≥1 ������4:��9 +��7 +��4 +��5 +��3 +��2 ≥1 ������5:��5 +��2 +��4 +��1 ≥1 } (8) In (8) ‘+’ works as logical operator ‘OR’. It can be stated from (8) that, to make bus 1 observable of the 5 bus test system, at least one PMU must be located at any of the buses (1, 2 or 3), if (8) is satisfied. Then the test system shown in Figure 1 is completely observable.
Subjected to restraint ��(��)≥�� (2) where binary variable ‘p’ is a vector for PMU, whose entry has as shown in (3) ���� ={1,��������������������������������ℎ������ 0,�������� (3) where i=1,2,….n bus number, wi is the cost of PMU sited at ithbus, b is a unit vector of length n �� =[11111]�� (4)
Int J Artif Intell ISSN: 2252 8938 Binary spider monkey algorithm approach for optimal siting of the phasor … (Suresh Babu Palepu) 1035
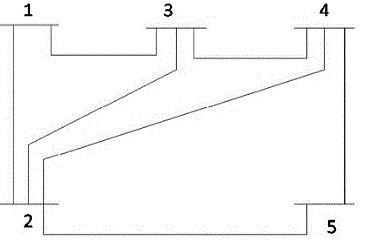
Step 1: During the initialization phase, the population size, global leader limit, and local leader limit, the most number of groups and rank of perturbation (pr) are all established. Generate random solutions using (9). Using this calculate fitness values and determine global and local leaders by comparison. Step 2: During the local leader phase, based on (10), generate a new solution. Calculate the best solution among the present and previous on the basis of the fitness.
A newly developed swarm intelligence meta heuristic algorithm, BSMOA [25], [26], it created to find the balance among local and global search abilities in order to achieve improved solution optimization [27]. As a result of the early stagnation, convergence and lack of investigation and development in prior algorithms, this method is designed to solve these issues and more. Each step of the SMO is: For binary optimization issues, BSMO is suggested, which is a generalization of the SMO method [25], [26]
The final positions of the spider monkey p modules are dependent on the knowledge acquired by the leader and group members’ experience in the local leader phase. When you acquire a new position, you compute the fitness value of that position. The employee’s new job offers a greater fitness value than his previous one, and so the employee changes his location. The location revise equation for ith p (which is a element of kth local group) in this phase i ������,�� ={����,�� ⊕((��⊗(������,�� ⊕))+ (��⊗(����,�� ⊕����,��))),������������ ≥���� ����,��,����ℎ������������ (10)
3.2. Global leader phase
Improved IBPSO algorithm described by Yuan et al. [28] [32] is the motivation for this method. They programmed IBPSO with the help of alogical operator, and utilised PSO’s velocity equations to implement it. Algorithm BSMO operates in binary space by applying logical operators to its basic equations. This method generates a random binary solution. This equation may be used to assist construct it: ����,�� ={0,�� <0.5 1,����ℎ������������ (9) where pi,jis the ith spider monkey of jth dimension, 0.5 is our probability value, and ‘r’ is a random value in the choice of [1,0]. A random value between 0.5 and 1 is used to determine the dimension. A dimension with a random number less than 0.5 will be set to 0, and vice versa. Spider monkeys' position calculations occur after initialization and use AND, OR, and XOR operations. The following updated equations are provided.
3. BINARY SPIDER MONKEY OPTIMIZATION ALGORITHM (BSMOA)
3.1. Local leader phase
All Solutions keep their locations update by accounting for group member knowledge and data from global leaders. According to the (11), the positions are always updated as ���� =09× (����������������) ������ �������������� +01 (11) where Pi denotes the probability, fitnessi denotes the fitness of the ith p, and min_fitness is the group’s minimum fitness. For this phase, if the Piless than random value then the position update equation is ������,�� ={����,�� ⊕((��⊗(������ ⊕)) +(��⊗(����,�� ⊕����,��))) (12) 3.3. Local leader decision phase
In local leader decision phase deals random solution ������,�� is the jth dimension of ith new position of p. Here pi,j is the previous dimension of jth p in the ith position. And glj is the global best in the jth element. Solution llk,j represent the local best of the jth dimension in the kth group, b and d are logical random numbers in the choice [1,0] and [1, 1] respectively, and +,⊕,⊗ are logical OR, AND, and XOR operators.
������,�� ={����,�� ⊕((��⊗(������,�� ⊕����,��))+ (��⊗(������ ⊕����,��))),�������� ≥���� ����������������������(9),����ℎ������������ (13)
ISSN: 2252 8938 Int J Artif Intell, Vol. 11, No. 3, September 2022: 1033 1040 1036
3.4. Binary spider monkey algorithm
Step 5: If the global leader is not updated, then divide into two groups and increased, if the most number of groups is reached, combine all groups into one. Local leader should be updated. If the convergence condition is met, the iterations are terminated.
Int J Artif Intell ISSN: 2252 8938
Step 4: In decision phase for the local leader; if the local leader is not modified, divert all members to forage using (13).
Binary spider monkey algorithm approach for optimal siting of the phasor … (Suresh Babu Palepu) 1037
Table 4 illustrates the quantity of PMUs needed for various systems and their bus positions. It also shows the maximum quantity of PMUs required when they are deployed under single PMU loss conditions when ZIBs are not enabled. For the IEEE 14bus system, nine PMUs are necessary. In this case number of PMUs is increased compare with base case.
4.3. Case 3: OPP without considering zero injections and one PMU loss
4.2. Case 2: OPP considering zero injections
Table 1. IEEE bus system data with no. of branches, zero injection buses and radial buses details SystemBus No. branchesof Total no. of InjectionZero Zero Injection Bus numbers Total no. of RadialBuses Radial Bus Numbers IEEE14 20 1 7 1 8 IEEE24 38 4 11, 12, 17, 24 1 7 IEEE30 41 6 6, 9, 22, 25, 27,28 3 11, 13, 26 IEEE39 46 12 1, 2, 5, 6, 9, 10, 11, 13, 14, 17,19, 22 9 30, 31, 32, 33, 34, 35, 36, 37, 38 IEEE57 78 15 4, 7, 11, 21, 22, 24, 26, 34, 36, 37, 39, 40, 45, 46, 48 1 33 IEEE118 179 10 5, 9, 30, 37, 38, 63, 64, 68, 71, 81 5 19, 73, 87, 111, 112
The findings for the number and placement of the buses for system observability considering zero injection buses, provided in Table 3. Three PMUs are required to implement an IEEE 14 bus system, and bus numbers are 2, 6, and 9. The number of PMUs is reduced considering zero injections compare with case 1. The cost of PMUs also reduced compare with case 1.
4.1. Case 1: OPP without considering zero injections
Step 3: Phase of global leadership, based on the (11), compute probabilities. Create a new population based on the (12). Determine the new solution fitness and choose the superior option based on the fitness of the new and old solutions. Update the position of the local and global leaders.
Proposed technique was simulated using MATLAB software. Table 1 showsthat number of zero injection buses and radial buses, for the six test systems [19], [28]
The information shown in Table 2 details the ideal number of PMUs needed for various systems. It also gives the places where they are required. 2, 6, 7, and 9 are necessary for placement in an IEEE 14 bus system, and without taking into consideration zero injection buses (ZIBs). In order to achieve full observability, 33 PMUs are needed for the 118 bus system. Number of PMUs required increases with system.
Table 2. No. of PMU’s required without considering zero injections for IEEE 14 bus system SystemBus Optimum no. of PMU’s needed Bus position of the PMU’s IEEE14 4 2, 6, 7, 9 IEEE24 7 2, 3, 8, 10, 16, 21, 23 IEEE30 10 2, 4, 6, 9, 10, 12, 15, 19, 25, 27 IEEE39 13 2, 6, 9, 10, 13, 14, 17, 19,20, 22, 23, 25, 29 IEEE57 17 1, 4, 6, 9, 15, 20, 24, 28, 30, 32, 36, 38, 41, 47,51,53,57 EEE118 32 3, 5, 9, 12, 15, 17, 21, 25, 28, 34, 37, 40, 45, 49, 52, 56, 62, 64, 68, 70,71, 76, 79, 85, 86, 89, 92,96, 100, 105, 110, 114
This technique was tested on IEEE 14, 24, 30, 39, 57, and 118 bus test systems and was also simulated for five different use cases. i) OPP without considering zero injections, ii) OPP considering zero injections, iii) OPP without considering zero injections and one PMU loss, iv) OPP considering zero injections and one PMU loss, and v) OPP considering zero injections and one PMU loss and a line outage.
Using the aforementioned values, it can be deduced to the basic scenario, the necessary quantity of PMUs for attaining full observability is about 1/3rd of the network dimension. This figure almost doubles when the scenario in which a single PMU outage or loss causes it is taken into consideration. It is expected that minimum of 2 PMUs will be in possession of each bus in a power system in order to maintain the power system observable.
4. RESULTS AND DISCUSSION
Table 6 No. of PMU’s required considering zero injections and one PMU loss and a line outage Bus System Optimum no. of PMU required Bus position of the PMU’s IEEE14 8 2, 4, 5, 6, 8, 9, 11, 13 IEEE24 11 1, 2, 7, 8, 9, 10, 16, 18, 20, 21, 23 IEEE30 13 1, 3, 5, 7, 10, 12, 13, 15, 16,17, 19, 20, 24 IEEE39 19 3, 6, 8, 13, 16, 20, 23, 25, 26, 29, 30, 31, 32, 33, 34, 35, 36, 37, 38 IEEE57 23 1, 3, 6, 9, 12, 14, 15, 18, 20, 25, 27, 29, 30, 32, 33, 36, 38, 41, 50, 51, 53, 54, 56 IEEE118 65 1, 3, 5, 7, 8, 10, 11, 12, 15, 17, 19, 21, 22, 24, 25, 27, 28, 29, 32, 34, 35, 37, 40, 41, 44, 45, 46, 49, 50, 51, 52, 54, 56, 59, 62, 66, 68, 72, 73, 74, 75, 76, 77, 78, 80, 83, 85, 86, 87, 89, 90, 92, 94, 96, 100, 101, 105, 107, 109, 110, 111, 112, 115, 116, 117
ISSN: 2252 8938 Int J Artif Intell, Vol. 11, No. 3, September 2022: 1033 1040 1038
Table 5 illustrates the quantity of PMUs needed for various systems and their bus positions. It also shows the maximum quantity of PMUs required when they are deployed under single PMU loss conditions when ZIBs are enabled. For the IEEE 14 bus system, seven PMUs are necessary. In this case number of PMUs is decreased compare with previous case because considering zero injections.
4.4. Case 4: OPP considering zero injections and one PMU loss
4.5. Case 5: OPP considering zero injections and one PMU loss or a line outage
The Table 6 illustrates the total number of PMUs desired for various systems. It also shows the various bus locations and the different situations where full observability maintained when one PMU fails or when the power line goes out. It is essential for IEEE14 bus structure 8 PMUs, and the bus information 2, 4, 5, 6, 8, 9, 11, and 13 are important for placing. The findings in Table 7 illustrate how many PMUs are needed for various systems, with their associated algorithms, and show the effectiveness of the proposed approach to find out the least quantity of PMU’s installations to attain a power system completely observable considering different algorithms.Table3 No. of PMU’s required considering zero injections for IEEE 14 bus system Bus System Optimum no. of PMU needed Bus position of the PMU’s IEEE14 3 2, 6, 9 IEEE24 6 2, 8, 10, 15, 20, 21 IEEE30 7 2, 4, 10, 12, 15, 19, 27 IEEE39 8 3, 8, 13, 16, 20, 23, 25, 29 IEEE57 11 1, 6, 13, 19, 25,29, 32, 38, 51, 54, 56 IEEE118 28 3, 8, 11, 12, 17, 21, 27, 31,32, 34, 37,40, 45, 49, 52, 56, 62, 72, 75, 77, 80, 85, 86, 90, 94, 102, 105, 110 Table 4 No. of PMU’s required considering zero injections and one PMU loss SystemBus Optimum no. of PMU required Bus position of the PMUs IEEE14 9 2, 4, 5, 6, 7, 8, 9, 10, 13 IEEE24 14 1, 2, 3, 7, 8, 9, 10, 11, 15, 16, 17, 20, 21, 23 IEEE30 21 2, 3, 4, 6, 7, 9, 10, 11, 12, 13, 15, 16, 18, 20, 22, 24, 25, 26, 27, 28, 30 IEEE39 28 2, 3, 6, 8, 9, 10, 11, 13, 14, 16, 17, 19, 20, 22, 23, 25, 26, 29, 30, 31, 32, 33, 34, 35, 36, 37, 38, 39 IEEE57 33 1, 3, 4, 6, 9, 11, 12, 15, 19, 20, 22, 24, 25, 26, 28, 29, 30, 32, 33, 34, 36, 37, 38, 41, 45, 46, 47, 50, 51, 53, 54, 56, 57 IEEE118 68 2, 3, 5, 6, 9, 10, 11, 12, 15, 17, 19, 21, 22, 24, 25, 27, 29, 30, 31, 32, 34, 35, 37, 40, 42, 43, 45, 46, 49, 51, 52, 54, 56, 57, 59, 61, 62, 64, 66, 68, 70, 71, 73, 75, 76, 77, 79, 80, 83, 85, 86, 87, 89, 90, 92, 94, 96, 100, 101, 105, 106, 108, 110, 111, 112, 114, 116, 117 Table 5. No. of PMU’s required considering zero injections and one PMU loss Bus System Optimum no. of PMU required Bus position of the PMUs IEEE14 7 2, 4, 5, 6, 9, 10, 13 IEEE24 11 1, 2, 7, 8, 9, 10, 16, 18, 20, 21, 23 IEEE30 12 2, 3, 4, 7, 10, 12, 13, 15, 16, 19, 20, 24 IEEE39 14 3, 12,15, 16, 20, 23, 25, 26, 29, 34, 35,36, 37, 38 IEEE57 21 1, 3, 9, 12, 14, 15, 18, 20, 25, 28, 29, 30, 32, 33, 38, 41, 50, 51, 53, 54, 56 IEEE118 64 1, 2, 5, 6, 8, 9, 11, 12, 15, 17, 19, 20, 21, 23, 25, 27, 28, 29, 32, 34, 35, 37, 40, 41, 43, 45, 46, 49, 50, 51, 52, 53, 56, 59, 62, 66, 68, 70, 71, 72, 75, 76, 77, 78, 80, 83, 85, 86, 87, 89, 90, 92, 94, 96, 100, 101, 105, 106, 108, 110, 111, 112, 114, 117
[1] A. G. Phadke, J. S. Thorp, and K. J. Karimi, “State estimlatjon with phasor measurements,” IEEE Trans. Power Syst., vol. 1, no. 1, pp. 233 238, 1986, doi: 10.1109/TPWRS.1986.4334878.
[9] B. K. S. Roy, A. K. Sinha, and A. K. Pradhan, “Optimal phasor measurement unit placement for power system observability A heuristic approach,” in 2011 IEEE Symposium on Computational Intelligence Applications In Smart Grid (CIASG), Apr. 2011, pp. 1 6, doi: 10.1109/CIASG.2011.5953335.
[7] S. Chakrabarti and E. Kyriakides, “Optimal placement of phasor measurement units for power system observability,” IEEE Trans. Power Syst., vol. 23, no. 3, pp. 1433 1440, Aug. 2008, doi: 10.1109/TPWRS.2008.922621.
[15] A. Y. Abdelaziz, A. M. Ibrahim, and R. H. Salem, “Power system observability with minimum phasor measurement units placement,” Int. J. Eng. Sci. Technol., vol. 5, no. 3, pp. 1 18, Mar. 2018, doi: 10.4314/ijest.v5i3.1.
[13] Z. Hong Shan, L. Ying, M. Zeng Qiang, and Y. Lei, “Sensitivity constrained PMU placement for complete observability of power systems,” in 2005 IEEE/PES Transmission and Distribution Conference and Exposition: Asia and Pacific, 2005, pp. 1 5, doi: 10.1109/TDC.2005.1547179.
This article presented a novel method called BSMO based on binary search space. Logic operators have been considered as the vital component of moving on to binary search room. In BSMO, the location of every spider monkey consists of 1 and 0 logic values, and these logical decision values are applied for optimal placement of PMUs in power system, which gives test systems a means of being topologically observable under consideration of ZIB, one PMU loss, and contingency of one line. The test results determined the effectiveness of the proposed approach to find out the least quantity of PMUs installations to attain a power system completely observable considering different operational aspects of the power system when compared to the GA, MBPSO and BFPA methods. This indicated that the proposed method is applicable for large systems.
[18] B. Allagui, I. Marouani, and H. H. Abdallah, “Optimal placement of phasor measurement units by genetic algorithm,” Int. J. Energy Power Eng., vol. 2, no. 1, pp. 12 17, 2013, doi: 10.11648/j.ijepe.20130201.12.
[11] T. L. Baldwin, L. Mili, M. B. Boisen, and R. Adapa, “Power system observability with minimal phasor measurement placement,” IEEE Trans. Power Syst., vol. 8, no. 2, pp. 707 715, May 1993, doi: 10.1109/59.260810.
[12] T. T. Cai and Q. Ai, “Research of PMU optimal placement in power systems,” in Proceedings of the 5th WSEAS/IASME Int. Conf. on Systems Theory and Scientific Computation, 2005, pp. 38 43, [Online]. Available: https://citeseerx.ist.psu.edu/viewdoc/download?doi=10.1.1.464.8571&rep=rep1&type=pdf.
Int J Artif Intell ISSN: 2252 8938 Binary spider monkey algorithm approach for optimal siting of the phasor … (Suresh Babu Palepu) 1039 Table 7 Assessment of PMU placements in IEEE bus system using BSMO algorithm with existing methods Bus System Optimal No. of PMUs for different systems GA [18] Modified binary PSO (MBPSO) [22] IBFPA [23] Proposed method IEEE14 3 3 3 IEEE24 6 6 6 6 IEEE30 7 7 7 7 IEEE39 8 IEEE57 13 13 11 IEEE118 29 29 29 28
5. CONCLUSION
[8] M. Zhou, V. A. Centeno, A. G. Phadke, Y. Hu, D. Novosel, and H. A. R. Volskis, “A preprocessing method for effective PMU placement studies,” in 2008 Third International Conference on Electric Utility Deregulation and Restructuring and Power Technologies, Apr. 2008, pp. 2862 2867, doi: 10.1109/DRPT.2008.4523897.
[14] K. S. Cho, J. R. Shin, and S. H. Hyun, “Optimal placement of phasor measurement units with GPS receiver,” in 2001 IEEE Power Engineering Society Winter Meeting. Conference Proceedings (Cat. No.01CH37194), 2001, vol. 1, pp. 258 262, doi: 10.1109/PESW.2001.917045.
[19] M. Hajian, A. M. Ranjbar, T. Amraee, and A. R. Shirani, “Optimal placement of phasor measurement units: particle swarm optimization approach,” in 2007 International Conference on Intelligent Systems Applications to Power Systems, Nov. 2007, pp. 1 6, doi: 10.1109/ISAP.2007.4441610.
REFERENCES
[2] A. G. Phadke and J. S. Thorp, Synchronized phasor measurements and their applications. Boston, MA: Springer US, 2008.
[16] F. J. Marín, F. García Lagos, G. Joya, and F. Sandoval, “Optimal phasor measurement unit placement using genetic algorithms,” in Computational Methods in Neural Modeling, Springer Berlin Heidelberg, 2003, pp. 486 493.
[5] D. Dua, S. Dambhare, R. K. Gajbhiye, and S. A. Soman, “Optimal multistage scheduling of PMU placement: an ILP approach,” IEEE Trans. Power Deliv., vol. 23, no. 4, pp. 1812 1820, Oct. 2008, doi: 10.1109/TPWRD.2008.919046.
[17] B. Milosevic and M. Begovic, “Nondominated sorting genetic algorithm for optimal phasor measurement placement,” IEEE Trans. Power Syst., vol. 18, no. 1, pp. 69 75, Feb. 2003, doi: 10.1109/TPWRS.2002.807064.
[10] M. Farsadi, H. Golahmadi, and H. Shojaei, “Phasor measurement unit (PMU) allocation in power system with different algorithms,” in 2009 International Conference on Electrical and Electronics Engineering ELECO 2009, 2009, pp. 396 400, doi: 10.1109/ELECO.2009.5355226.
[3] J. Chen and A. Abur, “Placement of PMUs to enable bad data detection in state estimation,” IEEE Trans. Power Syst., vol. 21, no. 4, pp. 1608 1615, Nov. 2006, doi: 10.1109/TPWRS.2006.881149.
[6] P. Gopakumar., G. S. Chandra, and M. J. B. Reddy, “Optimal placement of phasor measurement units for Tamil Nadu state of Indian power grid,” in 2012 11th International Conference on Environment and Electrical Engineering, May 2012, pp. 80 83, doi: 10.1109/EEEIC.2012.6221549.
[4] X. Bei, Y. J. Yoon, and A. Abur, “Optimal placement and utilization of phasor measurements for state estimation.” 2005, [Online]. Available: http://citeseerx.ist.psu.edu/viewdoc/download?doi=10.1.1.121.4322&rep=rep1&type=pdf.
ISSN: 2252 8938 Int J Artif Intell, Vol. 11, No. 3, September 2022: 1033 1040 1040 [20] Y. Gao, Z. Hu, X. He, and D. Liu, “Optimal placement of PMUs in power systems based on improved PSO algorithm,” in 2008 3rd IEEE Conference on Industrial Electronics and Applications, Jun. 2008, pp. 2464 2469, doi: 10.1109/ICIEA.2008.4582960.
[23] S. B. Palepu and M. D. Reddy, “Optimal PMU placement for power system state estimation using improved binary flower pollination algorithm,” in 2021 International Conference on Recent Trends on Electronics, Information, Communication and Technology (RTEICT), Aug. 2021, pp. 800 804, doi: 10.1109/RTEICT52294.2021.9573518.
[21] M. Hajian, A. M. Ranjbar, T. Amraee, and B. Mozafari, “Optimal placement of PMUs to maintain network observability using a modified BPSO algorithm,” Int. J. Electr. Power Energy Syst., vol. 33, no. 1, pp. 28 34, Jan. 2011, doi: 10.1016/j.ijepes.2010.08.007.
[27] U. Singh, R. Salgotra, and M. Rattan, “A novel binary spider monkey optimization algorithm for thinning of concentric circular antenna arrays,” IETE J. Res., vol. 62, no. 6, pp. 736 744, Nov. 2016, doi: 10.1080/03772063.2015.1135086.
[29] N. V. Phanendrababu, P. S. Babu, and D. V. S. SSivaSarma, “A novel placement of phasor measurement units using binary bat algorithm,” J. Electr. Eng., vol. 17, no. 8, 20117, [Online]. Available: http://www.jee.ro/index.php/jee/article/view/WS1477047046W5809f3063a15e. [30] M. L. Ramanaiah and M. D. Reddy, “Moth flame optimization method for unified power quality conditioner allocation,” Int. J. Electr. Comput. Eng., vol. 8, no. 1, pp. 530 537, Feb. 2018, doi: 10.11591/ijece.v8i1.pp530 537.
[32] P. S. Babu, P. B. Chennaiah, and M. Sreehari, “Optimal placement of svc using fuzzy and firefly algorithm,” IAES Int. J. Artif. Intell., vol. 4, no. 4, pp. 113 117, Dec. 2015, doi: 10.11591/ijai.v4.i4.pp113 117.
[26] A. H. Jabbar and I. S. Alshawi, “Spider monkey optimization routing protocol for wireless sensor networks,” Int. J. Electr. Comput. Eng., vol. 11, no. 3, pp. 2432 2442, Jun. 2021, doi: 10.11591/ijece.v11i3.pp2432 2442.
[28] X. Yuan, H. Nie, A. Su, L. Wang, and Y. Yuan, “An improved binary particle swarm optimization for unit commitment problem,” Expert Syst. Appl., vol. 36, no. 4, pp. 8049 8055, May 2009, doi: 10.1016/j.eswa.2008.10.047.
[24] Y. V. K. Reddy and M. D. Reddy, “Flower pollination algorithm to solve dynamic economic loading of units with piecewise fuel options,” Indones. J. Electr. Eng. Comput. Sci., vol. 16, no. 1, pp. 9 16, Oct. 2019, doi: 10.11591/ijeecs.v16.i1.pp9 16.
BIOGRAPHIES OF AUTHORS Palepu Suresh Babu received his B.Tech degree in Electrical and Electronics Engineering from Annamacharya Institute of Technology and sciences, Rajampet, Andhra Pradesh, India in 2006 and M. Tech degree in Power Systems from Sri Venkateswara University College of Engineering, Tirupati, and Andhra Pradesh, India in 2010. He is currently pursuing for Ph.D degree in Electrical Engineering at Sri Venkateswara University College of Engineering, Tirupati, and Andhra Pradesh, India. His research interests include capacitor, DG Placement and reconfiguration of distribution system, voltage stability studies, wide area monitoring system and smart grid. He can be contacted at email: sureshram48@gmail.com.
[31] A. V. S. Reddy, M. D. Reddy, and M. S. K. Reddy, “Network reconfiguration of primary distribution system using GWO algorithm,” Int. J. Electr. Comput. Eng., vol. 7, no. 6, pp. 3226 3234, Dec. 2017, doi: 10.11591/ijece.v7i6.pp3226 3234.
[25] J. C. Bansal, H. Sharma, S. S. Jadon, and M. Clerc, “Spider monkey optimization algorithm for numerical optimization,” Memetic Comput., vol. 6, no. 1, pp. 31 47, Mar. 2014, doi: 10.1007/s12293 013 0128 0.
Prof. Manubolu Damodar Reddy has 28 years of experience in teaching in Post Graduate level and 23 years of experience in Research. He has received M. Tech and Ph.D in Electrical Engineering from S. V. University College of Engineering, Tirupati, India, 1992 and 2008 respectively. He has a Life Member of ISTE. Presently he is working as a Professor in Electrical Engineering at S.V. University, Tirupati, India. He has published 1 Australian Patent, 63 Research (Intrnational Journals 48, Intrnational Conferences 32) Papers. Presently guiding 6 Ph.D. Scholars and 5 students are awarded. His Research area is Power system optimization and reactive power compensation. He can be contacted at email: mdreddy999@rediffmail.com.
[22] A. Ahmadi, Y. Alinejad Beromi, and M. Moradi, “Optimal PMU placement for power system observability using binary particle swarm optimization and considering measurement redundancy,” Expert Syst. Appl., vol. 38, no. 6, pp. 7263 7269, Jun. 2011, doi: 10.1016/j.eswa.2010.12.025.
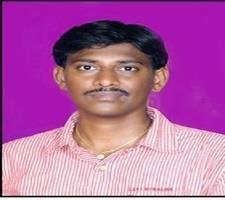




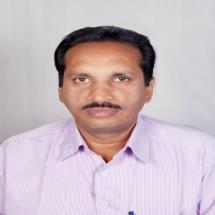




Article Info ABSTRACT
Based on past experience to recognize opportunities and predict the future by applying probability and statistics is the theorem of the Naïve Bayes method [1]. Strong or naive and assumptions, regardless of the condition or event of each is a character of Naïve Bayes [2]. Several data mining operations by applying Naïve Bayes with image data and numerical data from several diseases to obtain classification results [3]. In addition, classiying the behavior of web users applies naïve Bayes in the hope of obtaining optimal word segmentation results [4]. Many naïve Bayes applications classify both numerical data, images and web data with other things that are done by data crawling [5] It is because classification is a methodof usingdata todevelop a new computationalmodel in a certain area [6],[7]. The classification procedure employs a precise technique that differs from model to model, and a high level of accuracy is achieved when the accuracy reaches 100% [8]. It signifies that the final model produced good outcomes in terms of model creation using training and testing data. While classification by applying naïve Bayes is to detect hate speech on Twitter social media with the hope that the naïve Bayes method is able to study the previous data in Twitter and get accuracy in the carried out test [9], by a system applying the naïve Bayes classifier by 93%. Meanwhile AlSalman [10] also conducted research on the application of naïve Bayes with the topic of sentiment analysis on social media content to get opinions from several different applications and fields such as hobbies, activities and work carried out in Twitter which uses
Article history: Received Aug 15, 2021 Revised May 25, 2022 Accepted Jun 7, 2022 To classify Naïve Bayes classification (NBC), however, it is necessary to have a previous pre processing and feature extraction. Generally, pre processing eliminates unnecessary words while feature extraction processes these words. Thispaper focuses on featureextraction in which calculations and searches are used by applying word2vec while in frequency using term frequency Inverse document frequency (TF IDF). The process of classifying words on Twitter with 1734 tweets which are defined as a document to weight the calculation of frequency with TF IDF with words that often come out in tweet, the value of TF IDF decreases and vice versa. Following the achievement of the weight value of the word in the tweet, the classification is carried out using Naïve Bayes with 1734 test data, yielding an accuracy of 88.8% in the Slack word category tweet and while in the tweet category of verb 78.79% It can be concluded that the data in the form of words available on twitter can be classified and those that refer to slack words and verbs with a fairly good level of accuracy. so that it manifests from the habit of twitter social media user
The feature extraction for classifying words on social media with the Naïve Bayes algorithm
Arif Ridho Lubis, Mahyuddin Khairuddin Matyuso Nasution, Opim Salim Sitompul, Elviawaty Muisa Zamzami Fakultas Ilmu Komputer dan Teknologi Informasi, Universitas Sumatera Utara, Medan, Indonesia
IAES International Journal of Artificial Intelligence (IJ AI) Vol. 11, No. 3, September 2022, pp. 1041 1048 ISSN: 2252 8938, DOI: 10.11591/ijai.v11.i3 pp1041 1048 1041
Journal homepage: http://ijai.iaescore.com
This is an open access article under the CC BY SA license. Corresponding Author: Mahyuddin Khairuddin Matyuso Nasution Fakultas Ilmu Komputer dan Teknologi Informasi, Universitas Sumatera Utara Padang Bulan 202155 USU, Medan, Indonesia Email: mahyuddin@usu.ac.id 1. INTRODUCTION
Keywords: Feature extraction Naïve Bayes classification InverseTermWord2Vecfrequencydocument frequency
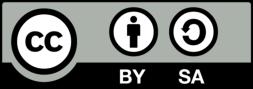
Data mining is a term that usually refers to knowledge findings in databases. It is a process that practices mathematical, statistical, artificial intelligence, and machine education methods that extract and recognize useful data and knowledge gathered from large databases [22]. Data mining, furthermore, is also referred to as the process of finding patterns, trends, and meaningful relationships [23]. Before carrying out the data mining process, it is better to know in advance what data mining can do, so that what is done later is suitable with what is needed and produces something that was previously unknown and is new and useful for its own users [24]. In principle, data mining has several tasks and must ensure that the pattern runs correctly in the process. There are 2 types of information mining tasks, namely [25]: a. EstimatingPredictive
2.2. Naïve Bayes Algorithm
One of the methods of classification is Naïve Bayes, this algorithm was invented by Thomas Bayes who is a scientist from England. Future opportunities can be predicted based on previous experience is the goal of Naïve Bayes [26]. This Naïve Bayes Classifier has the main characteristic of being very strong (naive) assumptions about each condition's self sufficiency. In compared to other classifier models, the Naïve Bayes Classifier performs quite well. One of the benefits of this method is that it just takes a little amount of training data to calculate the parameter estimation used in classification. The independent variable is the variation of a variable in a class that is designed to decide classification, not the whole covariance matrix [27]
The training stage and the classification stage are the stages of Naïve Bayes. The process of analyzing the document is carried out at the training stage where the vocabulary selection of the sample document is the word that appears in the sample document where the word is a representation of the document. The next step is to determine the probability for each category based on a sample document. Naïve Bayes built a probabilistic model from the term documents matrix data labeled. Document classification is done by first determining the
a certain attribute's value based on the values of other attributes. The dependent and target variables in such a case are called attributes, while the independent variable attribute is used to predict b. ObtainingDescriptivepatterns such as groups, trajectories, correlations, anomalies, and trends, which summarize the underlyingrelationshipsinthedataisthetaskofdescriptive.Descriptivedataminingtasksarealsoknown as investigations and often require post processing techniques for explanation and validation of the results.
2. MATERIAL AND METHOD 2.1. Data Mining in Social Network
ISSN: 2252 8938 Int J Artif Intell, Vol. 11, No. 3, September 2022: 1041 1048 Arabic,1042 the results of the experiment get useful from the proposed approach proposed to be continued. From these results were obtained comparisons showing this approach outperforms the field of work and it can increase the accuracy of 0.3%. Of various studies, naïve Bayes is often used in classification on social media to get sentiment analysis [11]. At this time, social media is one aspect that is very close to users, social media users are used to creating and sharing content any between users [12] [15]. Runining social media about 142 minutes a day [16], the initial low increases 100 minutes to get 142 minutes per day of use [17]. It is difficult to identify whether such platforms are profitable or detrimental to social media users, even though people around the world spend a large part of their days on social media platforms. This is related to the research conducted by Lubis et al [18] finding a framework for social media users, both the disclosure of words on social media is the keyword as the habit of social media users with initialized steps by reviewing current postings in order to have good data that is more particular and precise than those obtained from netizens [19] These exact keywords can then be used by the social media system to detect the profile of a certain user in the online domain in which a search engine can access. This certainly opens up insights that the behavior of social media users could be classified by applying the naïve Bayes method with training data in the form of words and keywords to classify words on social media [20] In matching and obtaining the frequency of the word data, nevertheless, a feature extraction stage is needed. So that in this study a comparison of several feature extraction techniques was carried out to obtain the optimal classification process. So many feature extractions are available that the research focuses on feature extraction on word classification on social media using naïve Bayes. In line with the development of data science applied in this paper, however, feature extraction uses term frequency Inverse document frequency (TF IDF) and Word2Vec. Where Word2Vec predicts the word given the surrounding context and after the occurrence of the model is created, what context vector operation is appropriate to perform the task for classification on the word in the new tweet [21]
The explanation of the general architecture in Figure 1 is:
Good research requires a research flow. The purpose of the research flow is to describe the stages that are carried out, where these stages are well explained. The research flow itself is used to ensure the research runs as expected. The flow of the research is drawn in a general structure as depicted in Figure 1: Figure 1. General Architecture
Int J Artif Intell ISSN: 2252 8938 The feature extraction for classifying words on social media with … (Arif Ridho Lubis) 1043 category c words in the document. The process of determining the categories of a document is done by calculating using equation (1) [28]: ��8 =����������������∈����(����|����)=����������������∈�� ∏��(������|����)����(����) (1) wwhere: kj is a feature or word of the document / tweet dj category to find out The value of p (wkj | ci) is known from the available training data.
a) Step 1. The crawling process using the API on Twitter makes it easy to get tweets that will be classified.
b) Step 2. The text preprocessing process is then continued with the feature extraction process where the feature extraction process is optimized by counting words assisted by Word2Vec then calculating the frequency with the IDF TF as well as contributing to this paper.
i) Making decomposition data ii) Reading the training data iii) For numeric data, how to calculate the number and probability is Each parameter is numeric, then the mean and standard deviation are calculated. The (2) to find the calculated average (mean) is as in (2):
3. GENERAL ARCHITECTURE
c) Step 3. The classification process applies NBC with the results, word classification and accuracy. The NBC procedure consists of the following steps:
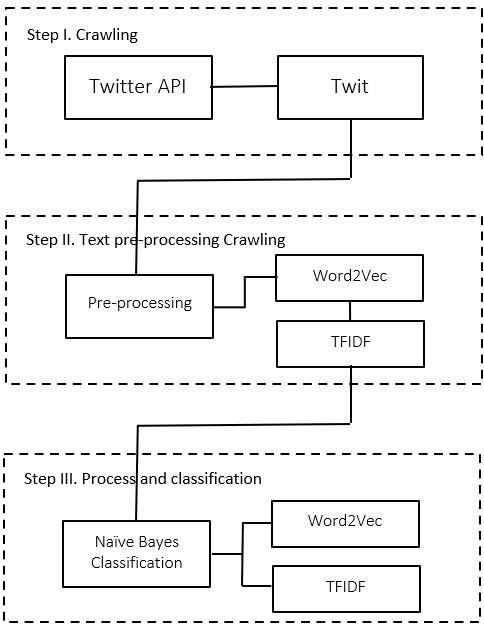
ISSN: 2252 8938 Int J Artif Intell, Vol. 11, No. 3, September 2022: 1041 1048 1044 �� = ∑ ���� �� �� �� (2) where μ : mean xi : the value of x to i n : Total samples While the (3) is to find the score/ value, Deviation Standard can be: �� =√∑ (���� ��) ��2 ��=1 �� 1 (3) where σ : deviation standard xi : the value of x to i μ : mean n : Total samples To get a probabilistic value, divide the amount of acceptable data from the same category by the amount of data in that category that is included. iv) Get value in word classification v) Produce accuracy with (4) ���������������� = ������������������������������������������������������������ �������������������������������� ��100% (4) 4. RESULT AND DISCUSSION
The data mining process in the form of classification techniques can be done using the Naïve Bayes Algorithm. Naïve Bayes generally are also often used in research that is sentiment analysis to get accuracy, patterns, human behavior and others available in cloud networks. The classification process with naïve Bayes cannot be separated from the process of training and testing data so that the correct data in this study use and collect data taken from social media. The data crawling is then performed feature extraction to facilitate the classification of words on social media. Several feature extractions will be tested to optimize the word classification using naïve Bayes on social media. Terms, which can be a sentence, word, or other indexing unit in a tweet that serves to establish the context, are things to considerwhile looking for informationfrom a collection of documents or tweets. Because each word has a distinct amount of relevance in the tweets on the tab, an indicator, specifically the term weight, is supplied for each word. When using word2vec to count and search for words, there are a few things to keep in mind. The results are shown in Figure 2: Figure 2. Results of Processing with Word2Vec 55% 19% 11% 8% 5% 2% Result of word count with Word2Vec Selamathappywkwkw pagi AnniversaryLovemakasih
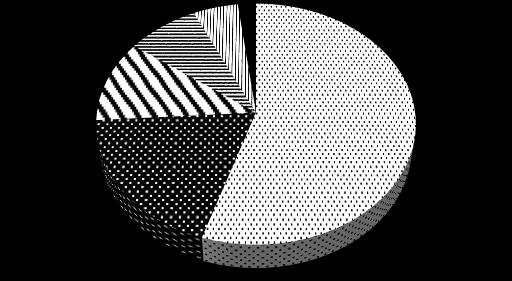






Next perform frequency calculations with TF IDF. Where, the term weighting method which is commonly used as a comparison method with the new weighting method is TF IDF. T term weight calculation of a document is done by multiplying the value of the Term Frequency Inverse Document Frequency. Some of the processes taken to compute the weight value using TF IDF Table 1 reveals that TF IDF calculations were performed using the frequency of tweets that frequently appear, namely the term "wkwk," which is slang for anyone who laughs or receives amusing things, containing the word happy, which is a verb in the form of joy. Where, the TF IDF calculation requires a frequency weighted value that has a function to calculate the best value, this is because the higher the number of words when calculating the TF IDF, the smaller the frequency. To find terms and assess the performance of documents which are based on tweets that appear, NBC is used. calculating the frequency of occurrence of words in the document is the first step taken. where the high frequency of repetition causes the greater the value of the word.
In the previous stage, Despite the fact that the generated text pattern was analysed by applying stopwords, the irregularity of the produced text pattern presents a challenge during identification. It appears that identifying the text was difficult and that careful examination was necessary. Because the patterns are irregular in their content arrangement, it is necessary to read the documents one at a time throughout the identification process to grasp the existing patterns in the text. The procedure to identify the tag on the training
In this study, data sources were employed from Twitter classified into documents as a reference to how papers would be classed. The targeted reference is document labeling based on expert domains. Twitter social media tweets are the type of document used. Twitter itself is unstructured content because there are things like mentions and HTML tags that cause the document to be meaningless. For classification accuracy, a structured document is needed so that it is easy to understand. The experimental document consists of 1734 characters from the @arfridho account.
Tokenization is first performed which aims to separate characters into tokens or words As certain characters could be used to separate tokens, tokenization is difficult for computer programs. To detect the pattern of the text which is going to be used for the categories that will be used as training data, so text identification is carried out.
Preprocessing andfeature extraction are the next steps in the classification process, which will be used to find meaning in tweets that will be trained or tested. This procedure must be followed since the document test data is in the form of paragraphs containing labels obscuring its content. Before the preparation process, it was difficult to understand the contents of the test text. Features that can potentially be affected by preprocessing so it is necessary to identify the text.
Table 1. Terms of Optimization Word2Vec and TF IDF Word (t) TF IDF happy 39 =log(112/39)= 0.4578818967 makasih 17 =log(112/17)= 0.8188854146 Anniversary 4 =log(112/4)= 1.447158031 wkwkwk 112 =log(112/112)=0 Love 9 =log(112/9)= 1.09482038 Selamat pagi 22 =log(112/22)= 0.7067177823
Int J Artif Intell ISSN: 2252 8938 The feature extraction for classifying words on social media with … (Arif Ridho Lubis) 1045
The NBC method requires two stages in the word classification process, namely the first stage is training where at this stage analysis are conducted on the documents of sample. They are in the format of social media data, namely tweets, words which might be shown up within a collection of documents of sample as well as determined from people's habits on social media reflect as many documents as possible, the documents used for training will be a reference in the testing process, as shown in Table 2. In the second stage is testing there is a training document that will be used as a reference for the testing process
Table 2. Data Decomposition Word (t) Training Testing happy 75% 25% makasih 75% 25% Anniversary 75% 25% wkwkwk 75% 25% Love 75% 25% Selamat pagi 75% 25%
ISSN: 2252 8938 Int J Artif Intell, Vol. 11, No. 3, September 2022: 1041 1048 document1046 is manually conducted. The data will be divided into two groups: slack words and verbs. Term frequency can be used to determine characteristics; however, experimental data indicated that only 25% of the chosen terms occurred frequently, which has little bearing on the categorization process. The data in Figure 3 are those having been achieved using the IDF TF. Then the document classification process requires a calculation involving the number of label documents n, m is the number of label documents, and the total number of training documents, which is called p (ci), namely the category x dividing the total documents in the category x the number of training data, similar to the category y is the division of the number of categorized documents y with the total number of training data, as shown in Table 3. In research with datawith words on Twitter that were tested as many as 531 twitting of verb categories and 1734 tweets referring to 1203 categories of Slack words as training data resulted in accuracy calculated based on (4) well in the tweet category of verbs 78.79% whereas on the Slack word category tweet of 88.8%.
120100806040200
IDFTFValue Word IDFTF
Figure 3. Classification features Table 3. Social Media Word Classifications by NBC Category Word Classification Verb Slack words p(ci) 0.50 0.50 P(wkj|ci) happy 15 24 makasih 17 0 Anniversary 2 2 wkwkw 0 112 love 9 0 Selamat pagi 20 2 5. CONCLUSION This paper draws the conclusion that data in the form of words available on twitter can be classified and those refering to the word slack and verb so as to manifest from the habit of the social media twitter users. In the process, word classifications in social media are conducted, beginning with data crawling on the Twitter API then carrying out preprocessing and feature extraction. Where there is interest in the feature extraction process with a combination of word2vec with TF IDF where the results of the TF IDF calculation result is possible to deduce that the TF IDF value with frequencies which frequently appear gets smaller frequency values and conversely with less frequency than the value of TF IDF is even bigger Following the TF IDF calculation, the classification is done using the Naïve Bayes approach, which divides the word classification into two categories, namely the Slack word category and the verb category. The test data consisted of 1734 twittes, with the results referring to 1203 Slack word categories and 531 twittens of verb categories as training data, resulting in good accuracy in the Slack word category twitt of 88.8% and 78.79% in the twitt verb categories.Where theresultsobtained fromthetest,obtainedafairlygoodaccuracyincategorizingslack words and verbs. happy makasih Anniversary wkwkw Love Selamat pagi
[2] H. Zhang, “Exploring Conditions for the Optimality of Naïve Bayes,” Int. J. Pattern Recognit. Artif. Intell., vol. 19, no. 02, pp. 183 198, Mar. 2005, doi: 10.1142/S0218001405003983.
[1] E. Sugiharti, S. Firmansyah, and F. R. Devi, “Predictive evaluation of performance of computer science students of unnes using data mining based on naÏve bayes classifier (NBC) algorithm,” J. Theor. Appl. Inf. Technol., vol. 95, no. 4, pp. 902 911, 2017.
[3] S. B. Özkan, S. M. F. Apaydin, Y. Özkan, and I. Düzdar, “Comparison of Open Source Data Mining Tools: NaiveBayes Algorithm Example,” in 2019 Scientific Meeting on Electrical Electronics & Biomedical Engineering and Computer Science (EBBT), 2019, pp. 1 4, doi: 10.1109/EBBT.2019.8741664.
[8] A. R. Lubis, M. Lubis, Al Khowarizmi, and D. Listriani, “Big Data Forecasting Applied Nearest Neighbor Method,” in ICSECC 2019 International Conference on Sustainable Engineering and Creative Computing: New Idea, New Innovation, Proceedings, 2019, pp. 116 120, doi: 10.1109/ICSECC.2019.8907010.
[14] E. B. Setiawan, D. H. Widyantoro, and K. Surendro, “Measuring information credibility in social media using combination of user profile and message content dimensions,” Int. J. Electr. Comput. Eng., vol. 10, no. 4, pp. 3537 3549, 2020, doi: 10.11591/ijece.v10i4.pp3537 3549.
[15] N. S. A. Rahman, L. Handayani, M. S. Othman, W. M. Al Rahmi, S. Kasim, and T. Sutikno, “Social media for collaborative learning,” Int. J. Electr. Comput. Eng., vol. 10, no. 1, pp. 1070 1078, 2020, doi: 10.11591/ijece.v10i1.pp1070 1078.
[22] A. Dogan and D. Birant, “Machine learning and data mining in manufacturing,” Expert Syst. Appl., vol. 166, p. 114060, 2021, doi: https://doi.org/10.1016/j.eswa.2020.114060.
[7] A. R. Lubis, M. Lubis, and Al Khowarizmi, “Optimization of distance formula in k nearest neighbor method,” Bull. Electr. Eng. Informatics, vol. 9, no. 1, pp. 326 338, 2020, doi: 10.11591/eei.v9i1.1464.
[18] A. R. Lubis, M. K. M. Nasution, O. S. Sitompul, and E. M. Zamzami, “A Framework of Utilizing Big Data of Social Media to Find Out the Habits of Users Using Keyword,” 2020, pp. 140 144. [19] A. R. Lubis et al., “Obtaining Value From The Constraints in Finding User Habitual Words,” pp. 8 11, 2020. [20] M. Asif, A. Ishtiaq, H.Ahmad, H.Aljuaid, and J.Shah,“Sentiment analysisof extremismin social mediafromtextual information,” Telemat. Informatics, vol. 48, p. 101345, 2020, doi: https://doi.org/10.1016/j.tele.2020.101345.
[23] S. Shirowzhan, S. Lim, J. Trinder, H. Li, and S. M. E. Sepasgozar, “Data mining for recognition of spatial distribution patterns of building heights using airborne lidar data,” Adv. Eng. Informatics, vol. 43, p. 101033, 2020, doi: https://doi.org/10.1016/j.aei.2020.101033.
[21] S. Lei, “Research on the Improved Word2Vec Optimization Strategy Based on Statistical Language Model,” in 2020 International Conference on Information Science, Parallel and Distributed Systems (ISPDS), 2020, pp. 356 359, doi: 10.1109/ISPDS51347.2020.00082.
[9] N. R. Fatahillah, P. Suryati, and C. Haryawan, “Implementation of Naive Bayes classifier algorithm on social media (Twitter) to the teaching of Indonesian hate speech,” in 2017 International Conference on Sustainable Information Engineering and Technology (SIET), 2017, pp. 128 131, doi: 10.1109/SIET.2017.8304122.
Int J Artif Intell ISSN: 2252 8938 The feature extraction for classifying words on social media with … (Arif Ridho Lubis) 1047
[10] H.AlSalman,“AnImproved Approach forSentimentAnalysisofArabicTweetsin TwitterSocialMedia,”in 20203rdInternational Conference on Computer Applications & InformationSecurity (ICCAIS),2020,pp.1 4,doi: 10.1109/ICCAIS48893.2020.9096850.
[25] J. Han, M. Kamber, and J. Pei, “1 Introduction,” in The Morgan Kaufmann Series in Data Management Systems, J. Han, M. Kamber, and J. B. T. D. M. (Third E. Pei, Eds. Boston: Morgan Kaufmann, 2012, pp. 1 38. [26] P.Phoenix,R.Sudaryono,and D.Suhartono,“ClassifyingPromotion ImagesUsing OpticalCharacterRecognitionand NaïveBayes Classifier,” Procedia Comput. Sci., vol. 179, no. 2020, pp. 498 506, 2021, doi: 10.1016/j.procs.2021.01.033. [27] S. Theodoridis, “Chapter 12 Bayesian Learning: Inference and the EM Algorithm,” S. B. T. M. L. (Second E. Theodoridis, Ed. Academic Press, 2020, pp. 595 646. [28] S. Theodoridis, “Chapter 13 Bayesian Learning: Approximate Inference and Nonparametric Models,” S. B. T. M. L. (Second E. Theodoridis, Ed. Academic Press, 2020, pp. 647 730.
[6] M. Morgan, C. Blank, and R. Seetan, “Plant disease prediction using classification algorithms,” IAES Int. J. Artif. Intell., vol. 10, no. 1, pp. 257 264, 2021, doi: 10.11591/ijai.v10.i1.pp257 264.
REFERENCES
[5] Q. Ye, Z. Zhang, and R. Law, “Sentiment classification of online reviews to travel destinations by supervised machine learning approaches,” Expert Syst. Appl., vol. 36, no. 3, Part 2, pp. 6527 6535, 2009, doi: https://doi.org/10.1016/j.eswa.2008.07.035.
[24] H. Thakkar, V. Shah, H. Yagnik, and M. Shah, “Comparative anatomization of data mining and fuzzy logic techniques used in diabetes prognosis,” Clin. eHealth, vol. 4, pp. 12 23, 2021, doi: 10.1016/j.ceh.2020.11.001.
[13] N. S. Shaeeali, A. Mohamed, and S. Mutalib, “Customer reviews analytics on food delivery services in social media: A review,” IAES Int. J. Artif. Intell., vol. 9, no. 4, pp. 691 699, 2020, doi: 10.11591/ijai.v9.i4.pp691 699.
[4] D. Bai, L. Zeng,and M. Feng,“Naivebayes for web penetrationbehavior classification based on word segmentation improvement,” in 2019 2nd International Conference on Information Systems and Computer Aided Education (ICISCAE), 2019, pp. 419 422, doi: 10.1109/ICISCAE48440.2019.221666.
[17] E. J. Ivie, A. Pettitt, L. J. Moses, and N. B. Allen, “A meta analysis of the association between adolescent social media use and depressive symptoms,” J. Affect. Disord., vol. 275, pp. 165 174, 2020, doi: https://doi.org/10.1016/j.jad.2020.06.014.
[16] T. Roshini, P. V Sireesha, D. Parasa, and S. Bano, “Social Media Survey using Decision Tree and Naive Bayes Classification,” in 2019 2nd International Conference on Intelligent Communication and Computational Techniques (ICCT), 2019, pp. 265 270, doi: 10.1109/ICCT46177.2019.8969058.
[11] C. Fiarni, H. Maharani, and R. Pratama, “Sentiment analysis system for Indonesia online retail shop review using hierarchy Naive Bayes technique,” in 2016 4th International Conference on Information and Communication Technology (ICoICT), 2016, pp. 1 6, doi: 10.1109/ICoICT.2016.7571912.
[12] K. Subrahmanyam, S. M. Reich, N. Waechter, and G. Espinoza, “Online and offline social networks: Use of social networking sites by emerging adults,” J. Appl. Dev. Psychol., vol. 29, no. 6, pp. 420 433, 2008, doi: https://doi.org/10.1016/j.appdev.2008.07.003.
Arif Ridho Lubis He got master from Universiti Utara Malaysia in 2012 and graduate from Universiti Utara Malaysia in 2011, both information technology. He is a lecturer in Department of Computer Engineering and Informatics, Politeknik Negeri Medan in 2015. His research interest includes computer science, network, science and project management. He can be contacted at email: arifridho.l@students.usu.ac.id
AUTHORS
ISSN: 2252 8938 Int J Artif Intell, Vol. 11, No. 3, September 2022: 1041 1048 1048 BIOGRAPHIES OF
Mahyuddin Khairuddin Matyuso Nasution Professor from Universitas Sumatera Utara, Medan Indonesia. Mahyuddin K. M. Nasution was born in the village of Teluk Pulai Dalam, Labuhan Batu Regency, North Sumatera Province. Worked as a Lecturer at the Universitas Sumatera Utara, fields: Mathematics, Computer and Information Technology. Education: Drs. Mathematics (USU Medan, 1992); MIT, Computers and Information Technology (UKM Malaysia, 2003); Ph D in Information Science (Malaysian UKM). He can be contacted at email: mahyuddin@usu.ac.id Opim Salim Sitompul Received the Ph.D. degree in information science from Universiti Kebangsaan Malaysia, Selangor, in 2005. He is currently a Professor with the Department of Information Technology, Universitas Sumatera Utara, Medan, Indonesia. His skill and expertise are in AI, data warehousing, and data science. His recent projects are in natural language generation (NLP) and AIoT. The result of his work can be seen in his most recent publication is ‘‘Template Based Natural Language Generation in Interpreting Laboratory Blood Test.’ He can be contacted at email: opim@usu.ac.id Elviawaty Muisa Zamzami Graduated from Bandung Institute of Technology (Indonesia), magister of informatics, 2000 and awarded Doctoral in Computer Science from the University of Indonesia in 2013. She is a lecturer at Department of Computer Science, Universitas Sumatera Utara, Indonesia. Currently her research interests are reverse engineering, requirements recovery, software engineering, requirements engineering and ontology. She can be contacted at email: elvi_zamzami@usu.ac.id
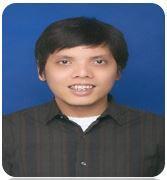



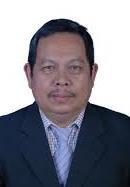



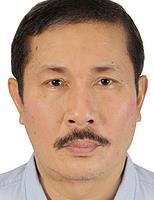



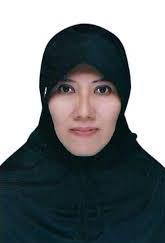



Thispaperpresentsmodelingofpowerdeliverynetwork(PDN)impedancewithvaryingdecouplingcapacitorplacementsusingmachinelearningtechniques.Theuseof multilayerperceptronartificialneuralnetworks(ANN)andgaussianprocessregression(GPR)techniquesareexplored,andtheeffectsofthehyperparameterssuchas thenumberofhiddenneuronsintheANN,andthechoiceofkernelfunctionsinthe GPRareinvestigated.Thebestperformingnetworksineachcaseareselectedand comparedintermsofaccuracyusingtestdataconsistingofPDNimpedanceresponses thatwereneverencounteredduringtraining.ResultsshowthattheGPRmodelswere significantlymoreaccuratethantheANNmodels,withanaveragemeanabsoluteerror of5.23mΩ comparedto11.33m
TominimizetheimpedanceofaPDN,oneofthemostcommonapproachistoplacedecoupling capacitorsonthePDN,especiallyatlocationsclosetothepowerpinsofthedevicesonthenetwork[4],[5].
Powerintegrity(PI)isafieldofengineeringwhichstrivestoensurethequalityofpowerdelivered tointegratedcircuitcomponentsthroughanetworkknownasthepowerdeliverynetwork(PDN)[1],[2].
PatrickGoh SchoolofElectricalandElectronicEngineering,UniversitiSainsMalaysia 14300,NibongTebal,Penang,Malaysia Email:eepatrick@usm.my 1. INTRODUCTION
Manypreviousworkexistsonoptimizingtheplacement,sizing,andselectionofdecouplingcapacitors onaPDN[6]-[9].However,theseworkarebasedontheoriesandruleofthumbswhichcanvaryfromdesign Journalhomepage: http://ijai.iaescore.com
PoorlydesignedPDNcaninduceseriousnoiseproblemstotheboardandaffectthesignalquality,whichcan impairdatathroughputandresultsinreducedperformances.GoodPDNdesignsshouldsatisfythegiventarget impedancesinacircuitinordertominimizedirectcurrent(DC)effectssuchasIRdrop,andalsoalternating current(AC)effectssuchassimultaneousswitchingnoiseandgroundbounce[3].
Thedecouplingcapacitorsareusedtoprovidelowimpedancepathsfromthepowersourcetothepowersinks andalsotofilterorguidethenoiseawaytotheground.However,thedesignandplacementsofthesedecoupling capacitorsonaPDNisnotatrivialtask.ToofeworpoorlyplaceddecouplingcapacitorswouldresultinPDNs whichfailtomeetthetargetspecifications,whiletoomanydecouplingcapacitorswouldincreasethesize andcostofthedesign.Inaddition,allphysicalcapacitorshaveassociatedparasiticinductances,whichare negligeableatlowerfrequencies,butcandominatetheperformanceathigherfrequencies,dependingonthe valueofthecapacitors.Thus,propersizingofthecapacitorsalsoplayanimportantroleinsatisfyingthetarget impedanceovertherangeoftheoperatingfrequencyinthedesign.
CorrespondingAuthor:
IAESInternationalJournalofArtificialIntelligence(IJ-AI) Vol.11,No.3,September2022,pp.1049∼1056 ISSN:2252-8938,DOI:10.11591/ijai.v11.i3.pp1049-1056 ❒ 1049 Machinelearningmodelingofpowerdeliverynetworks withvaryingdecouplingcapacitors YeongKangLiew1,NurSyazreenAhmad2,AznizaAbdAziz2,PatrickGoh2 1IntelCorporation,Penang,Malaysia 2SchoolofElectricalandElectronicEngineering,UniversitiSainsMalaysia,NibongTebal,Malaysia ArticleInfo Articlehistory: ReceivedJul8,2021 RevisedMar8,2022 AcceptedApr5,2022 Keywords: Artificialneuralnetwork Gaussianprocessregression Powerdeliverynetwork ABSTRACT
Ω fortheANN. Thisisanopenaccessarticleunderthe CCBY-SA license.
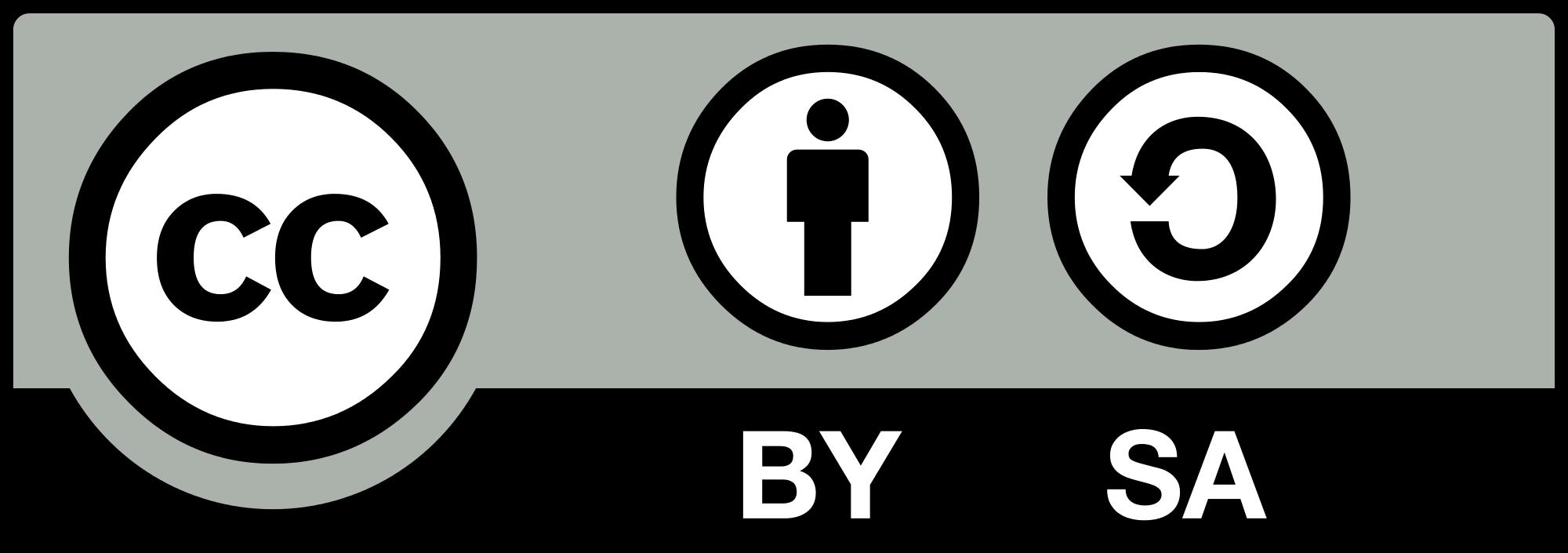
1050 ❒ ISSN:2252-8938 todesignandareusuallynoteasytogeneralizeandimplementpractically,withoutanexpertknowledgein thefield.Inaddition,theyrequireextensivetimeandefforttostudyandoptimizethedesignrepeatedly,often relyingoncomputationallyexpensivecomputer-aideddesignsimulations[10]-[12].
ThispaperpresentsastudyofMLtechniquestomodelandpredicttheimpedanceresponsecurvesof PDNswithvaryingdecouplingcapacitorplacements.Artificialneuralnetwork(ANN)andGaussianprocess regression(GPR)areappliedtomodelthePDNfromaphysicaldesignwithdifferentdecouplingcapacitor modelandvalues.EffectsofdifferenttrainingmethodsintheANNandkernelfunctionsintheGPRarealso investigated,andtheaccuracyofthevariousnetworksinbothmethodsarecompared.Thefundamentalsof ANNandGPRareassumedtobewellknowntothereader,andarenotrepeatedinthispaperforthebenefitof brevity. 2.
RESEARCHMETHOD 2.1. Datageneration
Recently,machinelearning(ML)techniqueshaveseenconsiderablesuccessinvariousengineering fields[13],[14],particularlyalsointhecloselyrelatedsignalintegrity(SI)field[15]-[18].Asaresult,researchershaveexploredtheuseofMLtechniquesforthesolutionofPIandPDNproblems[19]-[21].This includesworkutilizingparticleswarmoptimization,geneticalgorithm,andQ-learningtooptimizetheplacement,sizing,andselectionofthedecouplingcapacitorsonaPDN[22]-[26].Thegeneralpurposeofthese researchworksistooptimizethedesigntolowerdowntheinputimpedancesuchthatitislowerthanthetarget ordesiredimpedancevaluewiththeleastnumberofdecouplingcapacitorsusedinPDN.However,whilethese researchesfocusontheoptimizationofthePDN,theydonothelpintheimpedanceresponsepredictionof thesedesigns.Thisisimportantaswellasdesignersoftenprefertolookattheactualimpedanceresponseplots ofthePDNstogaininsightintotheirperformances.Theworkin[27]appliesarecurrentneuralnetworkto predicttheimpedanceresponsecurvebutitisrelatedtoextrapolationsoftheimpedanceresponsebeyondthe trainingfrequencybylearningthecorrelationoftheexistingimpedancevalues.Thus,thismethodcannotbe applieddirectlytoobtaintheimpedanceresponsefromaphysicaldesign.
Thedatausedinthisworkisobtainedbysimulationofactualimpedanceprofilesofpowerdelivery networksobtainedfromIntelCorporation.ThePDNisdrivenbyavoltageregulatormodule(VRM)andhas atotalof17locationsfordecouplingcapacitorplacements.Fourdifferentdecouplingcapacitormodelsare consideredandthisistabulatedinTable1.DuetothedifferentmodelsandlocationproximitytotheVRM onthePDN,eachdecouplingcapacitorlocationisrestrictedtoonlyreceiveonetypeofcapacitor.However, notethatitisnotnecessaryforalocationtohaveacapacitor,andinstead,itcanbeleftemptyifnecessary. Table2tabulatesthepossiblelocationsforeachofthedecouplingcapacitor,wherethe17locationsarelabeled fromAtoQ.Next,theimpedanceprofilesofthePDNwithvaryingdecouplingcapacitorplacementsare simulatedinCadenceAllegroSigrityOptimizePI.
Table1.Decouplingcapacitormodelsusedinthiswork IDModelnameManufacturerModeltypeCapacitance(µF)Area(mil2)RatedSelfresonance voltage(V)freq.(MHz) 1GRM155R61E104KA87MurataSPICE0.18002526.0 2LMK105BBJ475MVLFTaiyoYudenSPICE4.7800103.98 3CL05A106MQ5NUNSamsungSPICE108006.32.51 4GRM21BR60J476ME15MurataSPICE4740006.31.00 Table2.Possiblelocationsforeachofthecapacitormodel CapacitorIDPossiblelocations 1D,F,H,I,J,K,L,M,N,O 2E,G 3A 4B,C,P,Q Twodifferentsamplingmethodsareconsideredinthiswork.Inthefirstmethod,afinesamplingis performedwithatotalof236pointsoverthefrequencyrangeof10kHzto200MHz,whileinthesecond method,acoarsesamplingisperformedwithatotalof29pointsoverthefrequencyrangeof100kHzto IntJArtifIntell,Vol.11,No.3,September2022:1049–1056
❒ 1051
2.3. GPRmodelingmethod
No.ofhiddenneuronsLevenberg-marquardtMAE(mΩ)BayesianregularizationMAE(mΩ) 1015.8014.83 1515.9714.93 2015.3014.60 2515.3714.80 3014.9314.93 3515.2714.43 4015.9714.77 4514.9714.90 5014.7714.80 Machinelearningmodelingofpowerdeliverynetworkswithvaryingdecoupling...(YeongKangLiew)
TheGPRmodelhasthesamemodelinggoals,andinputandoutputtargetsastheANNintheprevioussubsection.ThemainhyperparameterintheGPRisthechoiceofkernelorcovariancefunction.For that,10differentkernelfunctionsareconsidered,rangingfromexponential,squaredexponential,Mat´ernwith parameters3/2and5/2,andrationalquadratickernels,andallareconsideredwiththesameoraseparatelength scaleperpredictor.Thus,10separateGPRmodelsaretrained,oneforeachkernel.Ineachcase,Bayesian optimizationisusedtooptimizetheremaininghyperparametersbyexploringthesearchspaceandminimizing thecross-validationloss.Finally,thebestperformingGPRmodelisselectedbasedonthelowestmeanabsolute error.
AthreelayeredmultilayerperceptronneuralnetworkisusedmodelthePDNtopredicttheimpedance responseoverthefrequencyrangeofinterest.Theinputsarethedecouplingcapacitorplacementsandcombinations,whiletheoutputsaretheimpedanceresponseofthePDN.Ahyperbolictangentsigmoidfunctionis usedastheactivationfunctionfortheinputlayer,whilealinearactivationfunctionisusedfortheoutputlayer. Toavoidoverweightingthelargerdatavalues,theinputandoutputdatavaluesarenormalizedtobebetween-1 and1.Twodifferentbackpropagationtrainingalgorithmsareconsidered,whicharetheLevenberg-Marquardt andtheBayesianregularizationtrainingfunctions.Earlystoppingisusedtopreventoverfitting.Inorderto determinetheoptimalnumberofhiddenneurons,alinearsweepisperformedfrom10-50hiddenneurons,with anincrementof5neuronsperiteration.Finally,thebestperformingneuralnetworkisselectedbasedonthe lowestmeanabsoluteerror.
IntJArtifIntellISSN:2252-8938
RESULTANDDISCUSSION
3.
200MHz.Thefinesamplingisnaturallymoreaccurate,butisalsocomputationallymoreexpensiveinboth thetrainingdatagenerationandmachinelearningtrainingprocesses.Acomparisonwillbedrawnbasedonthe resultsinbothsamplingmethodsinthenextsection.Oncethetrainingdatahasbeengeneratedbasedonthe twosamplingmethods,twomachinelearningmodelingtechniquesareinvestigatedtomodeltheimpedance profilesofthePDN.Thefirstutilizesamultilayerperceptronneuralnetwork,whilethesecondutilizesa Gaussianprocessregressionmodel.Thefollowingsubsectionsdescribetheprocessandparameterusedinboth methods. 2.2. ANNmodelingmethod
3.1. ANNmodelingresults
Themeanabsoluteerror(MAE)fortheANNtrainedusingtheLevenberg-MarquardtandBayesian regularizationtrainingalgorithmswithvaryingnumberofhiddenneuronsareshowninTable3forthecaseof afinesamplingwith236pointsoverthefrequencyrangeofinterest,andinTable4forthecaseofacoarse samplingwith29pointsoverthefrequencyrangeofinterest.Itcanbeseenthatthefinesamplingproduces amoreconsistentresultacrossallthenumberofhiddenneurons,whiletheresultsfromthecoarsesampling showsmorefluctuations.Thisisduetothefactthatthecoarsesamplingdatahaslessoverallpointsacross thefrequencyrange,andthusmorevariabilitybetweenthepoints.However,thecoarsesamplingdatamodels canyieldbetterresultsthanthefinesamplingdatamodelsattheoptimalnumberofhiddenneurons.Thebest performingnetworkisselectedastheANNwith35hiddenneuronstrainedwithBayesianregularizationforthe finesamplingmodel,andtheANNwith20hiddenneuronstrainedwithLevenberg-Marquardtforthecoarse samplingmodel.
Table3.MAEfortheANNmodelswithvaryingnumberofhiddenneuronsforfinesampling
❒
KernelfunctionLengthscaleperpredictorMAE(mΩ
Table4.MAEfortheANNmodelswithvaryingnumberofhiddenneuronsforcoarsesampling 1035.211.4 159.111.0 205.68.6 2522.69.2 3016.630.3 3531.19.5 4041.987.1 4510.134.7 5021.424.2 GPRmodelingresults TheMAEfortheGPRtrainedusingdifferentkernelfunctionsareshowninTable5forthecaseofthe finesamplingandTable6forthecaseofthecoarsesampling.ItcanbeseenthattheGPRmodelsshowless fluctuationswithvaryingkernelfunctions,comparedtotheANNswithvaryingnumberofhiddenneurons.In addition,usingasamelengthscaleperpredictorproducesbetterresultsonaveragethanusingaseparatelength scaleperpredictor.ThebestperformingmodelisselectedastheGPRwithanexponentialkernelfunctionand asamelengthscaleforeachpredictor,inbothcases.
Table5.MAEfortheGPRmodelswithvaryingkernelfunctionsforfinesampling )
Ω)
RationalquadraticSame15.50 SquaredexponentialSeparate58.00 ExponentialSeparate58.00 Matern3/2Separate58.00 Matern5/2Separate58.00 RationalquadraticSeparate58.00
3.2.
KernelfunctionLengthscaleperpredictorMAE(m
SquaredexponentialSame5.07 ExponentialSame4.80 Matern3/2Same5.00 Matern5/2Same5.03
RationalquadraticSame5.00 SquaredexponentialSeparate24.37 ExponentialSeparate24.47 Matern3/2Separate21.50 Matern5/2Separate24.57 RationalquadraticSeparate23.80
SquaredexponentialSame15.27 ExponentialSame12.07 Matern3/2Same15.63 Matern5/2Same15.60
3.3. ComparisonbetweenANNandGPR
Fromthefigures,itcanbeseenthattheGPRmodelsproducethemoreaccuratepredictiononthe testingdatasets,forboththefineandcoarsesamplingdata.WhiletheperformanceofboththeANNand IntJArtifIntell,Vol.11,No.3,September2022:1049–1056
1052 ISSN:2252-8938
Table6.MAEfortheGPRmodelswithvaryingkernelfunctionsforcoarsesampling
No.ofhiddenneuronsLevenberg-marquardtMAE(mΩ)BayesianregularizationMAE(mΩ)
InordertoperformanunbiasedtestingandcomparisonbetweentheANNandGPRmodels,two additionalPDNimpedanceprofilesaresimulatedusingCadenceAllegroSigrityOptimizePIwithdecoupling capacitorplacementcombinationsthatwereneverseenduringtraining.Thebestperformingmodelsofthe ANNandGPRdepictedintheprevioussubsectionswereselected,andthemodelswereusedtopredictthe impedanceprofilesinboththefineandcoarsesamplingfrequencyrange.Figure1showsacomparisonofthe predictedresultsfromtheANNandGPRmodelsfortestcase1,whereFigure1(a)showstheresultusingfine sampling,whileFigure1(b)showstheresultusingcoarsesampling.Similarly,Figure2showsacomparisonof thepredictedresultsfromtheANNandGPRmodelsfortestcase2,whereFigure2(a)showstheresultusing finesampling,whileFigure2(b)showstheresultusingcoarsesampling.
IntJArtifIntellISSN:2252-8938 ❒ 1053 GPRmodelsduringtrainingwerecomparabletoeachother,theGPRisabletogeneralizemuchbetterwhen presentedwithnewdatasetsthatwerenotencounteredduringtraining.ThiscanbeattributedtothenonparametricnatureoftheGPRthatpredictsoutcomesinaprobabilisticmanner,comparedtotheparametric ANNs.TheMAEvaluesforbothmodelsonthetestingdatasetsaresummarizedinTable7. 0.010.1110100 Frequency (MHz) 1 10 100 1000 )(mImpedance ANN GPR Actual (a) 0.1110100 Frequency (MHz) 1 10 100 1000 )(mImpedance ANN GPR Actual (b) Figure1.ComparisonoftheimpedanceprofilepredictedresultsfromtheANNandGPRmodelsfor testcase1(a)finesamplingand(b)coarsesampling 0.010.1110100 Frequency (MHz) 1 10 100 1000 )(mImpedance ANN GPR Actual (a) 0.1110100 Frequency (MHz) 1 10 100 1000 )(mImpedance ANN GPR Actual (b) Figure2.ComparisonoftheimpedanceprofilepredictedresultsfromtheANNandGPRmodelsfor testcase2(a)finesamplingand(b)coarsesampling Table7.MAEfortheANNandGPRmodelsforthetestingdata ModelTestcase1MAE(mΩ)Testcase2MAE(mΩ) FinesamplingCoarsesamplingFinesamplingCoarsesampling ANN14.112.45.813.0 GPR8.32.56.23.9 4. CONCLUSION Inthispaper,machinelearningmethodsareexploredforthemodelingofpowerdeliverynetwork impedancewithvaryingdecouplingcapacitorplacements.Theapplicationsofartificialneuralnetworksand Machinelearningmodelingofpowerdeliverynetworkswithvaryingdecoupling...(YeongKangLiew)
IEEETransactionsonElectromagneticCompatibility
[15] T.Nguyen etal.,“Comparativestudyofsurrogatemodelingmethodsforsignalintegrityandmicrowavecircuit applications,” IEEETransactionsonComponents,PackagingandManufacturingTechnology,vol.11,no.9,pp.
1054 ❒ ISSN:2252-8938
Ozbayat,T.Michalka,andJ.L.Drewniak,“Fastalgorithmforminimizingthe numberofdecapinpowerdistributionnetworks,”
[5] T.Wu,H.Chuang,andT.Wang,“OverviewofpowerintegritysolutionsonpackageandPCB:decouplingand EBGisolation,”
IAESInternationalJournalofArtificialIntelligence(IJ-AI),vol.10,no.1,pp.215–223, 2021,doi:10.11591/ijai.v10i1.pp215-223.
ACKNOWLEDGEMENT
ThisworkissupportedbytheMinistryofHigherEducation,Malaysia,undertheFundamentalResearchGrantScheme(FRGS)grantnumberFRGS/1/2020/TK0/USM/02/7. REFERENCES [1]
[13] A.Cabani,P.Zhang,R.Khemmar,andJ.Xu,“Enhancementofenergyconsumptionestimationforelectricvehicles byusingmachinelearning,”
[9] J.Xu etal.,“Anovelsystem-levelpowerintegritytransientanalysismethodologyusingsimplifiedCPMmodel, physics-basedequivalentcircuitPDNmodelandsmallsignalVRMmodel,”in 2019IEEEInternationalSymposiumonElectromagneticCompatibility,SignalandPowerIntegrity(EMC+SIPI),2019,pp.205–210,doi: 10.1109/ISEMC.2019.8825256. [10] B.Zhao etal.,“Systematicpowerintegrityanalysisbasedoninductancedecompositioninamulti-layeredPCBPDN,” IEEEElectromagneticCompatibilityMagazine,vol.9,no.4,pp.80–90,2020,doi:10.1109/MEMC.2020.9327998.
,vol.52,no.2,pp.346–356,May2010,doi: 10.1109/TEMC.2009.2039575. [6] K.Koo,G.R.Luevano,T.Wang,S.
IEEETransactionsonComputer-AidedDesignofIntegratedCircuitsandSystems,vol.22,no.4,pp. 428–436,2003,doi:10.1109/TCAD.2003.809658. [8] S.M.Vazgen,H.S.Karo,V.A.Avetisyan,andA.T.Hakhverdyan,“On-chipdecouplingcapacitoroptimization technique,” 2017IEEE37thInternationalConferenceonElectronicsandNanotechnology(ELNANO),2017,pp. 116–118,doi:10.1109/ELNANO.2017.7939729.
[11] B.Zhao etal.,“Physics-basedcircuitmodelingmethodologyforsystempowerintegrityanalysisanddesign,” IEEETransactionsonElectromagneticCompatibility,vol.62,no.4,pp.1266–1277,Aug.2020,doi: 10.1109/TEMC.2019.2927742. [12] I.ErdinandR.Achar,“FastpowerintegrityanalysisofPDNswitharbitrarilyshapedpower-groundplane pairs,”in 2020IEEEElectricalDesignofAdvancedPackagingandSystems(EDAPS),2020,pp.1–3,doi: 10.1109/EDAPS50281.2020.9312920.
Gaussianprocessregressiontechniquesweredemonstrated,andtheeffectsofthenumberofhiddenneurons inANN,andthekernelfunctioninGPRwereinvestigated.Thebestperformingnetworkswerecomparedin termsoftheaccuracyinmodelingarealPDNforbothafineandacoarsesamplingfrequencyrange.Itis foundthattheGPRgreatlyoutperformstheANNinthisregardwithanaverageMAEof5.23mΩ comparedto 11.33mΩ fortheANN,onnewtestdatathatwereneverencounteredduringtraining.Futureworkwillfocus onoptimizationofsearchspaceexplorationtechniquestoimprovethetrainingdatagenerationprocess,such thatitcanbetterrepresentthePDNandfrequencyrangeofinterest.Inaddition,improvedhyperparameter optimizationtechniquescanalsobepursuedtofurtherimprovethegeneralizationabilityofthemodels.
[3] M.Swaminathan,D.Chung,S.Grivet-Talocia,K.Bharath,V.Laddha,andJ.Xie,“Designingandmodelingfor powerintegrity,”
IEEETransactionsonElectromagneticCompatibility,vol.60,no. 3,pp.725–732,June2018,doi:10.1109/TEMC.2017.2746677.
IAESInternationalJournalofArtificial Intelligence(IJ-AI),vol.9,no.3,pp.429–438,2020,doi:10.11591/ijai.v9.i3.pp429-438.
[14] H.Ohmaid,S.Eddarouich,A.Bourouhou,andM.Timouyas,“ComparisonbetweenSVMandKNNclassifiersfor irisrecognitionusinganewunsupervisedneuralapproachinsegmentation,”
IntJArtifIntell,Vol.11,No.3,September2022:1049–1056
[7] H.Su,S.S.Sapatnekar,andS.R.Nassif,“Optimaldecouplingcapacitorsizingandplacementforstandard-cell layoutdesigns,”
J.Fan,“Signalintegrityandpowerintegrity,” IEEEElectromagneticCompatibilityMagazine,vol.9,no.2,pp.60–60, 2020,doi:10.1109/memc.2020.9133245. [2] Z.Yang,“Fundamentalsofpowerintegrity,”in 2018IEEESymposiumonElectromagneticCompatibility,Signal IntegrityandPowerIntegrity(EMC,SIandPI),2018,pp.1–50,doi:10.1109/EMCSI.2018.8495347.
IEEETransactionsonElectromagneticCompatibility,vol.52,no.2,pp.288–310,May2010,doi: 10.1109/TEMC.2010.2045382. [4] J.N.Tripathi,J.Mukherjee,P.R.Apte,N.K.Chhabra,R.K.Nagpal,andR.Malik,“Selectionandplacement ofdecouplingcapacitorsinhighspeedsystems,” IEEEElectromagneticCompatibilityMagazine,vol.2,no.4,pp. 72–78,2013,doi:10.1109/MEMC.2013.6714703.
[20] F.D.J.Leal-Romo,J.L.Ch´avez-Hurtado,andJ.E.Rayas-S´anchez,“Selectingsurrogate-basedmodelingtechniques forpowerintegrityanalysis,”in 2018IEEEMTT-SLatinAmericaMicrowaveConference(LAMC2018),2018,pp. 1–3,doi:10.1109/LAMC.2018.8699021.
Electronics,vol.8,no.7,2019,Art.no.737,doi:10.3390/electronics8070737. [23] K.Bharath,E.Engin,andM.Swaminathan,“Automaticpackageandboarddecouplingcapacitorplacementusing geneticalgorithmsandM-FDM,”in 200845th ACM/IEEEDesignAutomationConference,2008,pp.560–565,doi: 10.1145/1391469.1391611. [24] H.Park etal.,“Reinforcementlearning-basedoptimalon-boarddecouplingcapacitordesignmethod,”in 2018IEEE 27th ConferenceonElectricalPerformanceofElectronicPackagingandSystems(EPEPS),2018,pp.213–215,doi: 10.1109/EPEPS.2018.8534195.
BIOGRAPHIESOFAUTHORS
IntJArtifIntellISSN:2252-8938 ❒ 1055 1369–1379,2021,doi:10.1109/TCPMT.2021.3098666.
[27] O.W.BhattiandM.Swaminathan,“Impedanceresponseextrapolationofpowerdeliverynetworksusingrecurrent neuralnetworks,”in 2019IEEE28thConferenceonElectricalPerformanceofElectronicPackagingandSystems (EPEPS),2019,pp.1–3,doi:10.1109/EPEPS47316.2019.193198.
YeongKangLiew receivedtheB.Eng.inElectricalandElectronicEngineeringfrom theAsianInstituteofMedicine,ScienceandTechnology(AIMST)Universityin2018andtheM.Sc. degreeinElectronicSystemsDesignEngineeringfromUniversitiSainsMalaysia(USM)in2020.His researchinterestsareinthefieldsofelectronics,signalandpowerintegrity,andmachinelearning.He iscurrentlyahardwaredesignengineerinIntelMicroelectronic(M)SdnBhd.Hecanbecontacted atemail:yeong.kang.liew@intel.com.
[19] M.Swaminathan,H.M.Torun,H.Yu,J.A.Hejase,andW.D.Becker,“Demystifyingmachinelearningforsignalandpowerintegrityproblemsinpackaging,”
NurSyazreenAhmad receivedtheB.Eng.(Hons)degreeinElectricalandElectronic EngineeringfromtheUniversityofManchester,UnitedKingdomin2009,andPh.D.degreeinControlSystemsfromthesameuniversityin2012.SheiscurrentlywiththeSchoolofElectricaland ElectronicEngineering,UniversitySainsMalaysia.Hercurrentresearchinterestrevolvesaroundrobustconstrainedcontrol,intelligentcontrolsystems,computer-basedcontrolandautonomousmobile systemsinwirelesssensornetwork.Shecanbecontactedatemail:syazreen@usm.my. Machinelearningmodelingofpowerdeliverynetworkswithvaryingdecoupling...(YeongKangLiew)
[21] K.T.Chang etal.,“UltrahighdensityIOfan-outdesignoptimizationwithsignalintegrityandpowerintegrity,”in 2019IEEE69thElectronicComponentsandTechnologyConference(ECTC),2019,pp.41–46,doi: 10.1109/ECTC.2019.00014. [22] S.Piersanti,R.Cecchetti,C.Olivieri,F.dePaulis,A.Orlandi,andM.Buecker,“Decouplingcapacitorsplacement atboardleveladoptinganature-inspiredalgorithm,”
NeuralComputingandApplications,vol.32,no.11,pp.7311–7320,2020, doi:10.1007/s00521-019-04252-3.
[17] K.S.Ooi,C.L.Kong,C.H.Goay,N.S.Ahmad,andP.Goh,“Crosstalkmodelinginhigh-speedtransmissionlinesby multilayerperceptronneuralnetworks,”
[25] H.Park etal.,“Deepreinforcementlearning-basedoptimaldecouplingcapacitordesignmethodforsiliconinterposerbased2.5-D/3-DICs,”in IEEETransactionsonComponents,PackagingandManufacturingTechnology,vol.10,no. 3,pp.467–478,2020,doi:10.1109/TCPMT.2020.2972019. [26] L.Zhang etal.,“DecouplingcapacitorselectionalgorithmforPDNbasedondeepreinforcementlearning,” 2019 IEEEInternationalSymposiumonElectromagneticCompatibility,SignalandPowerIntegrity(EMC+SIPI),2019, pp.616–620,doi:10.1109/ISEMC.2019.8825249.
[16] C.H.Goay,N.S.Ahmad,andP.Goh,“Transientsimulationsofhigh-speedchannelsusingCNN-LSTMwith anadaptivesuccessivehalvingalgorithmforautomatedhyperparameteroptimizations,” IEEEAccess,vol.9,pp. 127644–127663,2021,doi:10.1109/ACCESS.2021.3112134.
[18] C.K.Ku,C.H.Goay,N.S.Ahmad,andP.Goh,“Jitterdecompositionofhigh-speeddatasignalsfromjitterhistogramswithapole–residuerepresentationusingmultilayerperceptronneuralnetworks,” IEEETransactionson ElectromagneticCompatibility,vol.62,no.5,pp.2227–2237,Oct.2020,doi:10.1109/TEMC.2019.2936000.
IEEETransactionsonComponents,PackagingandManufacturing Technology,vol.10,no.8,pp.1276–1295,Aug.2020,doi:10.1109/TCPMT.2020.3011910.
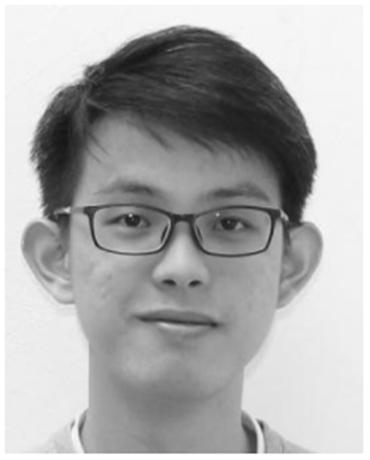



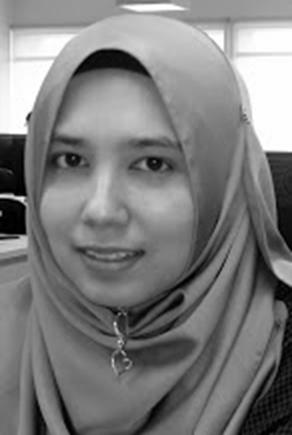



PatrickGoh receivedtheB.S.,M.S.,andPh.D.degreesinelectricalengineeringfromthe UniversityofIllinoisatUrbana-Champaign,Urbana,IL,USAin2007,2009,and2012respectively. Since2012,hehasbeenwiththeSchoolofElectricalandElectronicEngineering,UniversitiSains Malaysia,wherehecurrentlyspecializesinthestudyofsignalintegrityforhigh-speeddigitaldesigns. Hisresearchinterestincludesthedevelopmentofcircuitsimulationalgorithmsforcomputer-aided designtools.HewasarecipientoftheRajMittraAwardin2012andtheHaroldL.OlesenAward in2010,andhasservedonthetechnicalprogramcommitteeandinternationalprogramcommittee invariousIEEEandnon-IEEEconferencesaroundtheworld.Hecanbecontactedatemail:eepatrick@usm.my. IntJArtifIntell,Vol.11,No.3,September2022:1049–1056
1056 ❒ ISSN:2252-8938
AznizaAbdAziz receivedthePh.D.degreefromtheUniversityofSouthCarolina, Columbia,SC,USA.ShewasanAdvancedSignalIntegrityEngineerwithIntelCorporation,Penang, Malaysia,andaSeniorSignalIntegrityEngineerwithHewlettPackardEnterprise,PaloAlto,CA, USA,withtenyearsofexperienceindesigningandvalidatingdesktop,mobile,andserverplatforms.SheiscurrentlywiththeSchoolofElectricalandElectronicEngineering,UniversitiSains Malaysia,NibongTebal,Malaysia.Hercurrentresearchinterestsincludesignalintegritysolutions forhigh-speeddatadesign,electromagnetic,communicationsystems,machinelearning,andRFand microwaveengineering.Shecanbecontactedatemail:azniza@usm.my.
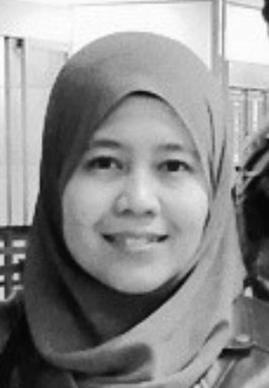



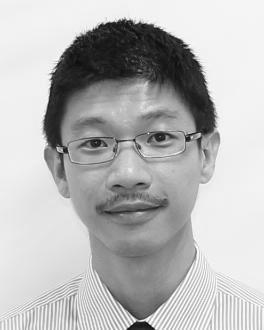



Corresponding Author: Pachisa KhunyingKulkanjanapibanLongAthakravisunthorn
Journal homepage: http://ijai.iaescore.com
Learning Resources Center, Prince of Songkla University 15 Karnjanavanich Rd., Hat Yai, Songkhla 90110, Thailand Email: pachisa.ku@psu.ac.th
Keywords: LatentBibliometricsdirichlet allocation Research trends Text Topicminingmodeling This is an open access article under the CC BY SA license.
This study investigates the evolution of information science research based on bibliometric analysis and semantic mining. The study discusses the value and application of metadata tagging and topic modeling. Forty two thousand seven hundred thirty eight articles were extracted from Clarivate Analytic's Web of Science Core Collection 2010 2020. This study was divided into two phases. Firstly, bibliometric analyzes were performed with VOSviewer. Secondly, the topic identification and evolution trends of information science research were conducted through the topic modeling approach latent dirichlet allocation (LDA) is often used to extract themes from a corpus, and the topic model was a representation of a collection of documents that is simplified using topic modeling toolkit (TMT). The top 10 core topics (tags) were information research design, information health based, model data public, study information studies, analysis effect implications, knowledge support web, data research, social research study, study media information, and research impact time for the studied period. Not only does topic modeling assist in identifying popular topics or related areas within a researcher's area, but it may be used to discover emerging topics or areas of study throughout time
1. INTRODUCTION
Article Info ABSTRACT
Tipawan Silwattananusarn1, Pachisa Kulkanjanapiban2 1Faculty of Humanities and Social Sciences, Prince of Songkla University, Mueang Pattani, Thailand
2Khunying Long Athakravisunthorn Learning Resources Center, Prince of Songkla University, Hat Yai, Thailand
A text mining and topic modeling based bibliometric exploration of information science research
Article history: Received Oct 1, 2021 Revised May 19, 2022 Accepted Jun 17, 2022
Several techniques for building knowledge models based on topics extracted using text mining procedures have been developed in recent years. Moro et al. [1] describes that latent semantic analysis and topic modeling are two of the most used techniques. The former is a natural language processing technique that analyzes relationships between textual terms and documents founded on the notion that words with similar meanings will appear incomparable material. At the same time, the latter takes as input the structure obtained by text mining, with the relevant terms and their frequency gathered into an orderly structure in which the documents are split into subjects [2]. Both techniques generate themes that summarize the body of
IAES International Journal of Artificial Intelligence (IJ AI) Vol. 11, No. 3, September 2022, pp. 1057 1065 ISSN: 2252 8938, DOI: 10.11591/ijai.v11.i3.pp1057 1065 1057
Many businesses generate large amounts of text and image data, which they store. This makes it challenging to manage large amounts of data and extract relevant information for decision making. New tools and techniques are required to manage this explosion of electronic documents better. Topic modeling is one of the new techniques for finding patterns of words in many documents developed in the last decade by machine learning and statistics for effective information retrieval. Numerous topic modeling applications include tag recommendation, text categorization, keyword extraction, and similarity search. Text mining, information retrieval, and statistical language modeling are just a few applications.
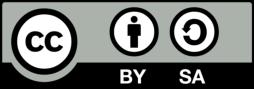
2. RESEARCH METHOD
Critical studies on applying latent dirichlet allocation (LDA), topic modeling, and text mining have been reviewed. Text mining enables the identification and retrieval of high quality new semantic information through the automated assessment of textual patterns and trends in the literature under review, which provides a more in depth understanding of the contents than a fundamental word count analysis [3]. A topic model is a valuable tool for text mining to identify research topics and hotspots in scientific and technological papers. The LDA model is popular in various fields. Some articles apply LDA, topic modeling, and text mining, such Lee and Cho [4] proposed a web document ranking method using topic modeling for effective information collection and classification. Allahyari et al. [5] described several of the most fundamental text mining tasks and techniques in biomedical and health care domains. The paper aims to identify major academic branches and detect research trends in design research using text mining techniques. Lubis et al. [6] proposed the topic modeling approach on helpful subjective reviews. Subeno et al. [7] aimed to determine the optimal number of corpus topics in the LDA method. The proposed approach in [8] can cluster the text documents of research papers into meaningful categories which contain a similar scientific field using a title, abstract, and keywords of the paper to the categories topics. Chauhan and Shah [9] introduced the preliminaries of the topic modeling techniques and reviewed its extensions and variations. The research in [10] is to survey the body of research revolving around big data and analytics in hospitality and tourism using bibliometric techniques, network analysis, and topic modeling. Chen et al. [11] used the LDA model to extract the subject of each paper published by authors in 239 educational journals from China and the United States during 20 years (2000 2019). Suominen et al. [12] uses LDA to create topic based linkages between publications and patents based on the semantic content in the documents. In empirical investigations [13], topic modeling has analyzed textual data. Between 2009 and 2020, the study analyzed subject modeling in 111 publications from the top ten ranked software engineering journals. The most common topic modeling techniques are LDA and LDA based strategies. The importance and complexity of information science issues have drawn scholars from various disciplines, including bibliometrics, social media analytics, text mining, machine learning, knowledge management, knowledge sharing, qualitative research, social science. Although information science has received much attention in recent years, few studies have attempted to conduct a large scale evaluation of academic literature on the subject. One of the essential functions of information science research is that it aids in identifying a variety of current public policy issues. This function responds to the growing need for information science in rational decision making. Building conceptual frameworks of relevant information science research are required to make more reasonable policies. In order to assist in the deployment of a rational information science development plan, a topic modeling based bibliometrics examination of peer reviewed literature representing information science research with 42,738 target articles published between 2010 and 2020 was conducted. This study combines the bibliometric method and LDA model to analyze the development trend of information science research from statistical analysis and text mining. This research fills in the blanks of existing literature by employing a technique that examines disciplines and applies them as tags to information science journals. This research benefits information retrieval, the semantic web, and linked data. This publication will be helpful to researchers, documentation and information professionals, students, and others interested in the field.
ISSN: 2252 8938 Int J Artif Intell, Vol. 11, No. 3, September 2022: 1057 1065 1058information included in the documents, resulting in a literature synthesis.
This study considers core journals in information science and library science from 2010 to 2020 and provides a method to identify the disciplinary identity in information science research. Each document's title, abstract, and keywords were used for the topic analysis. Forty two thousand seven hundred thirty eight articles published from 2010 2020 were collected as shown in Table 1. LDA topic modeling was used to further process and analyze the data sets. The topics were modeled using the LDA modeling technique.
Recently, the availability of accessible software allows researchers to make use of topic modeling and other text mining methodologies, making these methods more approachable. This study's modeling process is based on the topic modeling toolkit (TMT) package [14]. Text mining front end additions, such as the R package and VOSviewer [15], are required by the topic models package. The use of Microsoft Excel and PowerBI to aid in the processing and plotting of statistical data.
2.1. Data collection
The data used in this investigation was obtained from the Science Citation Index Expanded (SCI Expanded), Social Sciences Citation Index (SSCI), and Arts and Humanities Citation Index (AHCI) databases in June 2021, provided by the Institute for Scientific Information (ISI). The search time frame is set
Int J Artif Intell ISSN: 2252 8938 A text mining and topic modeling based bibliometric exploration of … (Tipawan Silwattananusarn) 1059 from 2010 to 2020. The following search query selected: SU=Information Science* AND DT=(Article) AND PY=2010 2020 Refined By: Web of Science Index: Social Sciences Citation Index (SSCI) or Science Citation Index Expanded (SCI EXPANDED) or Arts and Humanities Citation Index (A&HCI) to search articles published between 2010 and 2020 in the online SCI Expanded, SSCI, and AHCI databases. The study was restricted to research papers only articles. Proceedings papers, early access, book chapters, retracted publications were excluded. A total of 42,738 publications were collected.
Figure 1 demonstrates the LDA model as a probabilistic graphical model. The LDA representation has three layers. The variables shown in the figure are defined [2], [21]:
θ topic distribution for the document, d z the topic for the nth word in the document, d w is the specific word N total number of words M total number of documents in the corpus
Table 1. Year wise distribution of information science articles from 2010 2020 Year of publication Number of articles 2010 3292 2011 3498 2012 3548 2013 3684 2014 3855 2015 3933 2016 4126 2017 4129 2018 4086 2019 4149 2020 4438 Total 42738 2.2. Latent dirichlet allocation (LDA) LDA is a legal term that refers to (latent dirichlet allocation) [2]. In a text collection, topic modeling is a method for analyzing the distribution of semantic word clusters or "topics." It can explore a corpus' content and generate content related features for computational text classification. Topic modeling is thus largely independent of language and orthographic convention because it relies solely on the analyzed texts; it does not use additional sources of information such as dictionaries or external training data. It is solely based on a statistical analysis of symbol co occurrence (at the word level), then translated into possible semantic relationships [2], [16] [20] This paper focuses on applying LDA [2] to model the subjects from the corpus of Information Science articles based on dirichlet distribution. Each article is represented in this study as a pattern of LDA topics. LDA automatically infers the topic mentioned in a collection of articles, and these topics can be used to summarize and organize the articles. Bags of words per article are the variables observed, while the hidden random variables are the topic distribution of each article. The observable variables in LDA are: i) the bags of words per article based on probabilistic modeling. LDA's fundamental purpose is to compute the posterior of hidden variables given the observable variables' values. Articles with similar themes will employ similar groupings of words, ii) articles are a probability distribution over latent topics, and iii) topics are probability distributions over words [21] as shown in Figure 1. Figure 1. LDA is represented graphically in this model (source [2])
α parameter of Dirichlet prior on the per document topic distribution β parameter of Dirichlet prior on per topic word distribution
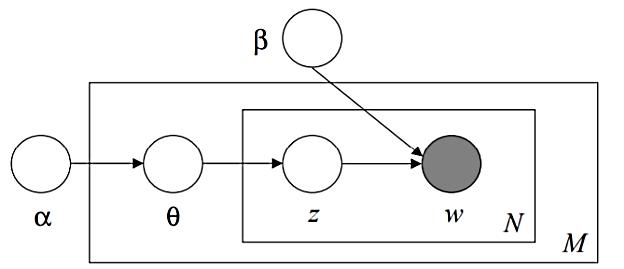
The outside box in Figure 1 represents documents in the LDA model, while the inner box represents the documents' repeated selection of subjects and terms. The alpha (α) and the beta (β) are corpus level parameters assumed to be sampled once during the corpus generation process. The variables θd are document level variables sampled only once for each document. The word level variables zdn and wdn are sampled once for each word in each document.
3. RESULTS AND DISCUSSION
VOSviewer is a software application that creates maps based on network data and then visualizes and explores these maps [26], [27] The VOSviewer functionality can create maps based on network data and visualize and explore maps. VOSviewer can be used to create, visualize, and explore maps based on any network data, in addition to analyzing bibliometric networks. Van Eck and Waltman [15] presents the text mining functionality of VOSviewer supports the creation of term maps from a corpus of texts. A term map is a two dimensional map in which words are arranged so that the distance between two terms may be read to measure their relatedness. The closer two terms are related to each other, the smaller the distance between them. The co occurrences of terms in documents are used to determine their relatedness. Titles, abstracts, and full texts in publications, patents, and newspaper articles are examples of these documents. To create a term map based on a corpus of documents, VOSviewer identifies the following processes:Identification of noun phrases by: i) performing part of speech tagging, ii) using a linguistic filter to identify noun phrases, and iii) converting plural noun phrases into singular ones. Selection of the most relevant noun phrases by: i) determining the distribution of co occurrences overall noun phrases, ii) comparing this distribution with the overall distribution of co occurrences over noun phrases, iii) grouping noun phrases in co occurrence with a high relevance together into clusters. Each cluster can be viewed as a separate topic
3.1. Bibliometric analysis
Mapping and clustering of the terms
2.3. Topic modeling toolkit (TMT) Finding topics in a document is known as topic modeling [22]. The co occurrence of terms in a document is one method of detecting the presence of a topic in a document. Topic models make analyzing large amounts of unlabeled text simple. A topic comprises a group of words that appear together regularly. Using contextual signals, topic models can connect words with similar meanings and discriminate between words with various meanings. A topic model is a representation of a collection of documents that is simplified. TMT [14], [23], [24] is a topic modeling software that associates words with subject labels so that words that frequently appear in the same documents are more likely to have the same label applied to them. It can find similar themes in a collection of documents and trends in discourse through time and across borders. TMT is a graphical interface tool for LDA topic modeling [25]. All 42,738 articles were converted into text format and then processed using TMT. In the toolkit, the following parameters were being fixed for the study: i) number of topics: 20, ii) number of iterations: 400, iii) number of topic words to print: 20, iv) interval between hyperprior optimizations: 10, and v) number of training threads: 4.
3.1.1. Publication analysis The number of publications in information science has increased significantly over the last decade, and in the coming years, this pattern is expected to continue. As illustrated in Figure 2(a), there is a noticeable increase from 2010 2015 due to growing concerns about information science research. The difference in trends between the two sub periods, 2010 2015 and 2016 2020, is notable. As a result, the relationship between the annual cumulative number of articles and the publication year for the two sub
The results of the mapping and clustering are visualized.
2.4. Text mining functionality in VOSviewer
ISSN: 2252 8938 Int J Artif Intell, Vol. 11, No. 3, September 2022: 1057 1065 1060
LDA is a corpus based generative probabilistic model [2]. The core idea is that documents are represented as random mixes over latent topics, with each subject defined by a distribution of words. LDA assumes the following generative process for each document w in a corpus D: Choose N ~ Poisson(ξ) Choose θ ~ Dir(α) For each of the N words wn: i) choose a topic zn ~ Multinomial(θ) and ii) choose a word wn from p(wn | zn, β), a multinomial probability conditioned on the topic zn
(b)(a)
Figure 2. The trend of (a) number of articles and (b) cumulative number of articles from 2010 to 2020 The trend also spans many general information science and technology journals and more specialized outlets. As Table 2 shows, the most significant number of relevant articles published by general information science and technology appears in Scientometrics [28] (3175), followed by Journal of the American Medical Informatics Association [29] (1853), Qualitative Health Research [30] (1645), and Journal of Health Communication [31] (1273). Among the top five publication outlets emphasizes the importance of health for domain specific informatics scholars. Furthermore, publications in Telecommunications Policy [32], Government Information Quarterly [33], and Journal of Documentation [34] suggest context specific considerations of information science research.
Int J Artif Intell ISSN: 2252 8938 A text mining and topic modeling based bibliometric exploration of … (Tipawan Silwattananusarn) 1061 periods was described using linear and power models, respectively. Figure 2(b) depicts the overall trend in the number of articles. The linear curve fitting result is y = 3953.2x 1417.6, and the power curve fitting result is y = 3230.8x1.0707, where y stands for the cumulative number of articles and x stands for the publication year. Both curves fit the observed data points well with high correlation coefficients (R²=0.9989 for 2010 2015, and R²=0.9998 for 2016 2020). Since 2015, the power model has shown a rapid increase in the number of articles in information science.
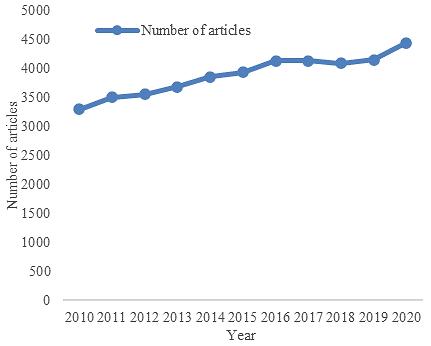
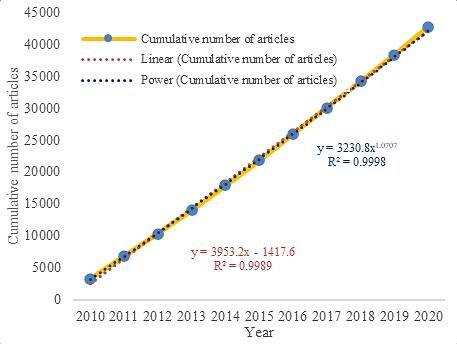
20
3.1.2.
ISSN: 2252 8938 Int J Artif Intell, Vol. 11, No. 3, September 2022: 1057 1065 1062 Table 2. Top 20 publication outlets and their respective number of articles on information science No Name of Journal Year of Publication 10 11 12 13 14 15 16 17 18 19 20 Total 1 Scientometrics 207 215 214 249 299 343 291 357 350 265 385 3175 2 Journal of the American Medical Informatics Association 107 144 172 200 200 157 169 148 182 165 209 1853 3 Qualitative Health Research 137 133 137 140 143 136 162 172 166 155 164 1645 4 Journal of Health Communication 93 107 111 114 112 180 155 118 105 85 93 1273 5 International Journal of Geographical Information Science 89 96 110 122 125 102 120 111 106 108 98 1187 6 Journal of the Association for Information Science and Technology 184 185 215 191 114 101 103 1092 7 Professional De La Information 74 86 82 66 71 88 90 113 113 120 168 1071 8 International Journal of ManagementInformation 55 59 58 87 75 70 99 85 100 142 203 1033 9 Information Processing and Management 56 64 80 89 50 63 72 73 72 140 237 996 10 Telematics and Informatics 23 37 38 53 78 93 185 172 93 91 863 11 Journal of Informetrics 59 60 70 93 82 80 79 83 82 71 77 836 12
16
18 Government
Journal of Academic Librarianship 56 56 43 71 73 95 84 63 96 78 104 819 of Knowledge Management 57 57 54 53 62 68 64 78 81 100 95 769 & Management 41 44 36 60 86 75 65 80 75 78 102 742 15 Library Journal 59 72 63 79 70 74 68 78 78 54 43 738 Telecommunications Policy 60 76 61 72 62 60 65 74 61 55 84 730 of the American Society for Information Science and Technology 176 184 178 180 718 Information Quarterly 47 50 70 60 70 46 67 53 67 67 71 668 19 Electronic Library 56 50 48 48 51 69 59 69 66 63 51 630
13 Journal
Keyword search terms are vocabularies that can locate an article in abstracting and indexing databases. Trends and topics of interest can be discovered using keyword analysis. The VOSviewer analyzed all keywords in documents, including author's and index keywords, at a five frequently used keywords threshold. The most used keywords were mapped out as shown in Figure 3. Keywords with similar colors were grouped. The size of each circle in the cluster represented the proportion of citations for that subject's keywords. Larger circles and map labels signified greater relevance and significance.
Figure 3. Co occurrence network map of most frequently used keywords in information science research
17 Journal
14 Information
Journal of Documentation 39 43 41 40 53 61 59 65 69 75 67 612 Co occurrence keywords analysis
As shown in Figure 3, clusters were differentiated by five colors: red, green, blue, yellow, and purple. The central cluster in red included the keywords "knowledge management," "innovation,"
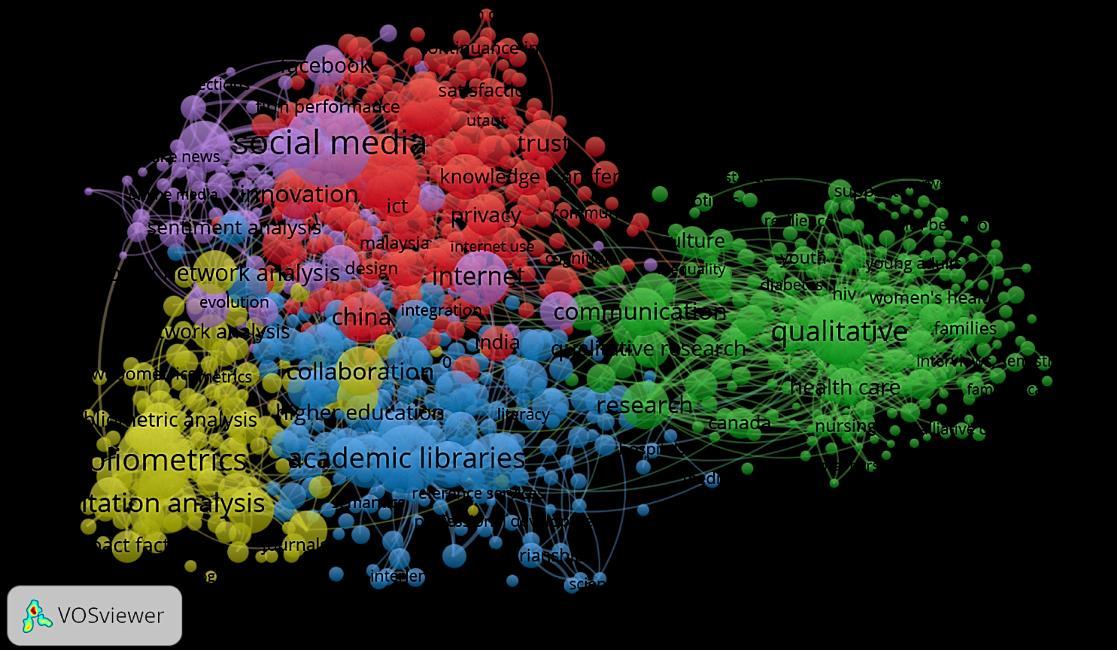
Int J Artif Intell ISSN: 2252 8938
0
researchInformationdesign information research design based paper process findings show international study data results in level related language government network implementation potential evaluate
Socialstudyresearch social research study characteristics result in experience software performance resources education media organizational assessment understand journals studies patterns institutional citation reference
Topic Potential topic List of topics
The following are some examples of interpretations from Table 3:
Analysisimplicationseffect analysis effect implications information services study case provide future article individual libraries business online applied social records citation theoretical 5
It also includes words like "language," "government," "network." Indicating that novel information science technology, such as natural language processing (NLP), data governance, social media analysis, text mining, social media mining, becomes more critical in the development research strategy of information science.
Model data public model data public library methodology system paper survey context factors mobile information approaches academic sharing methods order risk analyzed results
Topic 9 also addresses social approach analysis with the highest frequent term of "analysis effect implications." However, unlike topic 4, topic 9 focuses on the research impact time and social approach analysis findings in analysis effect implications. Terms like "research impact," "data method," "social approach," "journal knowledge" are all research impact time. An essential issue in this topic is the analysis findings set trust service level.
Table 3. Top 10 frequent terms according to their probability values
Study informationmedia study media information control processes article sources examined behavior librarians increase quality knowledge significant dimensions documents published factors paper collection
8
Topic 3 and topic 6 contain words like "paper," "research," "data," "tools source," "online analysis," "topic results," "users," "methods," and "performance." Topics 3 and 6 discuss paper research data issues related to information science. However, different from topic 3, topic 6 focuses on management purpose and online analysis development significant study. An essential issue in this topic is the study results in performance influence users.
Topic 4 refers to the effects of information analysis on information services and contains words like "analysis," "implications," "information service," and "business online." Topic 4 frequently uses terms like "social records," "social approach analysis," and "citation theoretical."
Researchtimeimpact research impact time data method social approach analysis findings number information collected results journals knowledge set trust service level
Informationbasedhealth information health based digital library systems result in authors related found knowledge articles research examine attention discussed patient publications community aims 2
Table 3 summarizes the LDA results generated by the TMT. Each topic's top 10 frequent terms for the ten years are organized in descending order according to their probability values.
A text mining and topic modeling based bibliometric exploration of … (Tipawan Silwattananusarn) 1063
"knowledge sharing," "information technology," and "big data." The keywords made up the second cluster, highlighted in green "qualitative," "communication," "decision making," "health care," and "culture." The most commonly used keywords in the third blue cluster were "academic libraries," "information literacy," "machine learning," "information retrieval," and "natural language processing." The fourth cluster in yellow included the keywords of "bibliometrics," "citation analysis," "social network analysis," "h index," and "research evaluation." The fifth cluster shown in purple consisted of the keywords "social media," "social networks," "content analysis," "web 2.0," and "Twitter."
Paper data research paper data research management purpose online analysis development libraries significant study years work results performance users two authors decision user
3.2.1. Topic identification Statistics of terms that frequently appear in a collection of abstracts can provide a primary picture of a research field. The latent intellectual concepts in the literary corpus were discovered using the LDA model.
3.2. Topic modeling analysis
Topic 1 focuses on information health related concerns in information science and the effects of information health based digital library systems on patient community knowledge and well being.
7
Knowledgewebsupport knowledge support web information research data practical models based analysis scientific role practice conducted quality significantly rights higher limited cited 6
1
4
9
3
Topic 0 contains words like "information," "research," "design," "findings," "results," and thus apparently discusses the role of information research design in increasing collaboration implementation.
Studystudiesinformation study information studies paper research data Elsevier technology tools source topic results users high methods influence groups measure common capital
ISSN: 2252 8938 Int J Artif Intell, Vol. 11, No. 3, September 2022: 1057 1065 1064Topics 7 and 8 discuss social research study issues related to study media information. Topic 7 contains words like "characteristics," "social," "research," "study," "education," "media," "experience," "software," "performance," "organizational," "assessment," "patterns," "institutional," "citation," "reference.", and refers to the influences of social research projects on the organizational assessment and pattern institutional citation reference. Topic 8 focuses more on information control and knowledge quality level, thus, employs words like "control," "processes," "sources," "examined," "behavior," "paper collection," "factors," and "document published."
4. CONCLUSION
ACKNOWLEDGEMENTS
[11] S. Chen, S. Ren, L. Zheng, H. Yang, W. Du, and X. Cao, “A comparison study of educational scientific collaboration in China and the USA,” Phys. A Stat. Mech. its Appl., vol. 585, p. 126330, Jan. 2022, doi: 10.1016/J.PHYSA.2021.126330.
A topic modeling based bibliometric exploration of information science research uses the 42738 articles collected from the SCI Expanded, SSCI, and A&HCI databases. This investigation's findings provide a comprehensive overview, focusing on information science research topics from 2010 to 2020. From 2020 to 2020, linear and exponential relationships between the annual cumulative number of articles and publication year were obtained for the collected articles, revealing that annual article publications grow constantly. The findings of the co occurrence map based on the author keywords of the information science research, the keywords knowledge management, innovation, qualitative, decision making, academic libraries, machine learning, bibliometrics, citation analysis, social media, social networks were the most co occurrences and the hot topics in the information science research. This topic analysis shows that information research design issues appeal to scholars more than information studies themselves and that an interdisciplinary trend of information health based research is emerging from the convergence of health science, social science, and media information. This research contributes to our understanding of information science's academic concerns over the last ten decades. It can be said that information science research is the core of current knowledge and powerfully connects with much other research in related fields. The study's findings have implications for future information policy. The rapid increase in publications indicates a significant demand for information related research. In addition, the government should provide more funding for this research field in conjunction with the accelerated information development process. Second, because large projects in emerging economics and health science will account for most of the growth in information generation, these should learn from the experience of information science development in these areas. Furthermore, this research provides a comprehensive overview for this purpose.
This study received no specific funding from the public, private, or non profit sectors. The authors are grateful to thank the anonymous reviewers for their careful reading of our manuscript and their many insightful comments and suggestions.
[7] B. Subeno, R. Kusumaningrum, and Farikhin, “Optimisation towards Latent Dirichlet Allocation: Its Topic Number and Collapsed Gibbs Sampling Inference Process,” Int. J. Electr. Comput. Eng., vol. 8, no. 5, pp. 3204 3213, 2018, doi: 10.11591/IJECE.V8I5.PP3204 3213. [8] A. A. Jalal and B. H. Ali, “Text documents clustering using data mining techniques,” Int. J. Electr. Comput. Eng., vol. 11, no. 1, pp. 664 670, 2021, doi: 10.11591/IJECE.V11I1.PP664 670. [9] U. Chauhan and A. Shah, “Topic Modeling Using Latent Dirichlet allocation: A Survey,” ACM Comput. Surv., vol. 54, no. 7, 2022, doi: 10.1145/3462478.
[2] D. M. Blei, A. Y. Ng, and J. B. Edu, “Latent Dirichlet Allocation Michael I. Jordan,” J. Mach. Learn. Res., vol. 3, pp. 993 1022, 2003, doi: 10.5555/944919.944937. [3] V. Gupta and G. S. L. Professor, “A Survey of Text Mining Techniques and Applications,” 2009, [Online]. Available: www.alerts.yahoo.com [4] Y. Lee and J. Cho, “Web document classification using topic modeling based document ranking,” Int. J. Electr. Comput. Eng., vol. 11, no. 3, pp. 2386 2392, 2021, doi: 10.11591/IJECE.V11I3.PP2386 2392. [5] M. Allahyari et al., “A Brief Survey of Text Mining: Classification, Clustering and Extraction Techniques,” vol. 13, 2017, [Online]. Available: http://en.wikipedia.org/wiki/Statistics [6] F. F. Lubis, Y. Rosmansyah, and S. H. Supangkat, “Topic discovery of online course reviews using LDA with leveraging reviews helpfulness,” Int. J. Electr. Comput. Eng., vol. 9, no. 1, pp. 426 438, 2019, doi: 10.11591/IJECE.V9I1.PP426 438.
[10] M. Mariani and R. Baggio, “Big data and analytics in hospitality and tourism: a systematic literature review,” Int. J. Contemp. Hosp. Manag., vol. 34, no. 1, pp. 231 278, Jan. 2022, doi: 10.1108/IJCHM 03 2021 0301/FULL/PDF.
[12] A. Suominen, S. Ranaei, and O. Dedehayir, “Exploration of Science and Technology Interaction: A Case Study on Taxol,” IEEE Trans. Eng. Manag., vol. 68, no. 6, pp. 1786 1801, Dec. 2021, doi: 10.1109/TEM.2019.2923634.
[1] S. Moro, G. Pires, P. Rita, and P. Cortez, “A text mining and topic modelling perspective of ethnic marketing research,” J. Bus. Res., vol. 103, pp. 275 285, 2019, doi: 10.1016/j.jbusres.2019.01.053.
REFERENCES
[31] A. F. Hannawa, L. García Jiménez, C. Candrian, C. Rossmann, and P. J. Schulz, “Identifying the field of health communication,” J. Health Commun., vol. 20, no. 5, pp. 521 530, May 2015, doi: 10.1080/10810730.2014.999891.
[34] D. Ivanović and Y. S. Ho, “Highly cited articles in the Information Science and Library Science category in Social Science Citation Index: A bibliometric analysis,” J. Librariansh. Inf. Sci., vol. 48, no. 1, pp. 36 46, Mar. 2016, doi: 10.1177/0961000614537514.
[25] “senderle (Jonathan Scott Enderle) · GitHub.” https://github.com/senderle (accessed Mar. 12, 2022). [26] N. van Eck and L. Waltman, “VOSviewer Visualizing scientific landscapes,” VOSviewer. Accessed: Feb. 12, 2022. [Online]. Available: https://www.vosviewer.com// [27] N. J. van Eck and L. Waltman, “Software survey: VOSviewer, a computer program for bibliometric mapping,” Scientometrics, vol. 84, no. 2, pp. 523 538, Dec. 2010, doi: 10.1007/S11192 009 0146 3/FIGURES/7. [28] E. Coşkun, G. Özdağoğlu, M. Damar, and B. A. Çallı, “Scientometrics based study of computer science and information systems research community macro level profiles,” Proc. 12th IADIS Int. Conf. Inf. Syst. 2019, IS 2019, pp. 180 188, 2019, doi: 10.33965/IS2019_201905L023.
Pachisa Kulkanjanapiban is an Academic Librarian with a Professional Level in the Khunying Long Athakravisunthorn Learning Resources Center, Prince of Songkla University, Thailand. She holds an M.Sc. degree in Management Information Technology (MIT) from the Prince of Songkla University. She is a specialist in scientometrics, bibliometrics, database and information retrieval, and predictive modeling. Her expertise is Institutional Repository (IR), especially running the "PSU Knowledge Bank." Also, she is responsible for IT project and IT service management, knowledge and data management in her organization. She is fascinated by data science, data mining and machine learning, digital management systems, data analytics and visualization, business intelligence, digital transformation, database analysis and design, computing in social sciences, arts and humanities, and data management topics. She can be contacted at email: pachisa.ku@psu.ac.th.
[20] M. H. Hwang, S. Ha, M. In, and K. Lee, “A Method of Trend Analysis using Latent Dirichlet Allocation,” Int. J. Control Autom., vol. 11, no. 5, pp. 173 182, 2018, doi: 10.14257/ijca.2018.11.5.15.
[18] Z. Hu, S. Fang, and T. Liang, “Empirical study of constructing a knowledge organization system of patent documents using topic modeling,” Scientometrics, vol. 100, no. 3, pp. 787 799, 2014, doi: 10.1007/s11192 014 1328 1.
[30] N. Mays and C. Pope, “Qualitative research in health care: Assessing quality in qualitative research,” BMJ Br. Med. J., vol. 320, no. 7226, p. 50, Jan. 2000, doi: 10.1136/BMJ.320.7226.50.
Tipawan Silwattananusarn is an Assistant Professor in the Information Management Program, Faculty of Humanities and Social Sciences, Prince of Songkla University, Thailand. She has received her Ph.D. degree in Information Studies from Khon Kaen University. She is involved in research and teaching in Data Mining and Machine Learning Tools, Data Analytics and Visualization, Information Management Systems and Technologies, Information Security Management, Digital Information Management. She is a specialist in data mining and analytics, data mining applications for knowledge management, and IT applications in academic libraries. She is an author and published research and textbooks in Data Mining and Data Analytics & Visualization. Her latest book, "Practical Data Mining with WEKA" (written in Thai), was published in June 2021. She can be contacted at email: tipawan.s@psu.ac.th.
[29] T. A. Morris and K. W. McCain, “The structure of medical informatics journal literature,” J. Am. Med. Informatics Assoc., vol. 5, no. 5, pp. 448 466, Sep. 1998, doi: 10.1136/JAMIA.1998.0050448/2/JAMIA0050448.F08.JPEG.
[33] F. Bannister and Å. Grönlund, “Information Technology and Government Research: A Brief History,” Hawaii Int. Conf. Syst. Sci. 2017, Jan. 2017, Accessed: Apr. 06, 2022. [Online]. Available: https://aisel.aisnet.org/hicss 50/eg/transformational_government/4
[15] N. J. van Eck and L. Waltman, “Text mining and visualization using VOSviewer”, [Online]. Available: www.vosviewer.com.
[17] X. Han, “Evolution of research topics in LIS between 1996 and 2019: an analysis based on latent Dirichlet allocation topic model,” Scientometrics, vol. 125, no. 3, pp. 2561 2595, 2020, doi: 10.1007/S11192 020 03721 0/FIGURES/2.
Int J Artif Intell ISSN: 2252 8938 A text mining and topic modeling based bibliometric exploration of … (Tipawan Silwattananusarn) 1065
BIOGRAPHIES OF AUTHORS
[32] Y. Kwon and J. Kwon, “An overview of Telecommunications Policy’s 40 year research history: Text and bibliographic analyses,” Telecomm. Policy, vol. 41, no. 10, pp. 878 890, Nov. 2017, doi: 10.1016/J.TELPOL.2017.07.012.
[16] T. Jiang, X. Liu, C. Zhang, C. Yin, and H. Liu, “Overview of Trends in Global Single Cell Research Based on Bibliometric Analysis and LDA Model (2009 2019),” J. Data Inf. Sci., vol. 6, no. 2, pp. 163 178, 2021, doi: 10.2478/JDIS 2021 0008.
[23] “Projects senderle/topic modeling tool GitHub.” https://github.com/senderle/topic modeling tool/projects (accessed Mar. 12, 2022). [24] “Quickstart Guide | Topic Modeling Tool Blog.” https://senderle.github.io/topic modeling tool/documentation/2017/01/06/quickstart.html (accessed Mar. 12, 2022).
[13] C. C. Silva et al., “Topic modeling in software engineering research,” Empir. Softw. Eng. 2021 266, vol. 26, no. 6, pp. 1 62, 2021, doi: 10.1007/S10664 021 10026 0. [14] “GitHub senderle/topic modeling tool: A point and click tool for creating and analyzing topic models produced by MALLET.” https://github.com/senderle/topic modeling tool (accessed Mar. 12, 2022).
[21] M. Lamba and M. Madhusudhan, “Mapping of topics in DESIDOC Journal of Library and Information Technology, India,” Scientometrics, vol. 120, no. 2, pp. 477 505, 2019, doi: 10.1007/S11192 019 03137 5. [22] G. Shobha and S. Rangaswamy, “Computational analysis and understanding of natural languages: Principles, methods and applications Chapter 8 Machine learning,” Handb. Stat., vol. 38, pp. 197 228, 2018.
[19] A. R. Destarani, I. Slamet, and S. Subanti, “Trend Topic Analysis using Latent Dirichlet Allocation (LDA) (Study Case: Denpasar People’s Complaints Online Website),” J. Ilm. Tek. Elektro Komput. dan Inform., vol. 5, no. 1, pp. 50 58, 2019, doi: 10.26555/JITEKI.V5I1.13088.
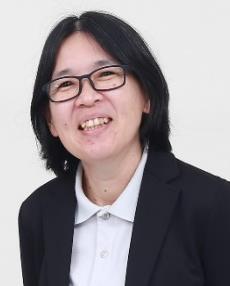




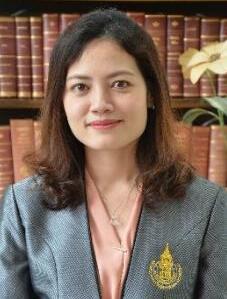




Tsehay Admassu Assegie1, Prasanna Kumar Rangarajan2, Napa Komal Kumar3 , Dhamodaran Vigneswari4 1
Journal homepage: http://ijai.iaescore.com
Department of Computer Science, College of Natural and Computational Science, Injibara University, Injibara, Ethiopia 2
Department of Computer Science and Engineering, St. Peter’s Institute of Higher Education and Research, Chennai, India 4Department of Information Technology, KCG College of Technology, Chennai, India
Article history: Received Apr 25, 2021 Revised May 24, 2022 Accepted Jun 12, 2022
IAES International Journal of Artificial Intelligence (IJ AI) Vol. 11, No. 3, September 2022, pp. 1066 1073 ISSN: 2252 8938, DOI: 10.11591/ijai.v11.i3 pp1066 1073 1066
Article Info ABSTRACT
Keywords: Decision tree Heart disease prediction Random Recursiveforestfeature elimination Support vector machine
This is an open access article under the CC BY SA license. Corresponding Author: Tsehay Admassu Assegie Department of Computer Science, Injibara University P.O.B: 40, Injibara, Ethiopia Email: tsehayadmassu2006@gmail.com
1. INTRODUCTION
In recent years, machine learning is attaining higher precision and accuracy in clinical heart disease dataset classification. However, literature shows that the quality of heart disease feature used for the training model has a significant impact on the outcome of the predictive model. Thus, this study focuses on exploring the impact of the quality of heart disease features on the performance of the machine learning model on heart disease prediction by employing recursive feature elimination with cross validation (RFECV). Furthermore, the study explores heart disease features with a significant effect on model output. The dataset for experimentation is obtained from the University of California Irvine (UCI) machine learning dataset. The experiment is implemented using a support vector machine (SVM), logistic regression (LR), decision tree (DT), and random forest (RF) are employed. The performance of the SVM, LR, DT, and RF models. The result appears to prove that the quality of the feature significantly affects the performance of the model. Overall, the experiment proves that RF outperforms as compared to other algorithms. In conclusion, the predictive accuracy of 99.7% is achieved with RF
In the last few years, the implementation and adoption of a machine learning algorithm for heart disease diagnosis have been the major focus of researchers [1]. The reason behind the wider adoption and application of machine learning and predictive model to heart disease prediction include the promising accuracy of the learning model compared to a human expert, the speed, and the cost expenditure spent for heart disease prediction or detection. Despite the wider adoption and application of the predictive model to heart disease diagnosis and their promising result on heart disease prediction, the performance of the machine learning model has still scope for improvement. In the literature, the impact of heart disease feature quality on the learning model is largely focused on. Hence, this study is aimed to further investigate the impact of heart disease feature quality and explores the most important or informative heart disease features that represent the heart disease patient resulting in better predictive outcomes This research focuses on the application of recursive feature elimination with the cross validation (RFECV) method to process heart disease data before the model is trained using a support vector machine
An empirical study on machine learning algorithms for heart disease prediction
Department of Computer Science and Engineering, Amrita School of Engineering, Amrita Vishwa Vidyapeetham, Chennai, India 3
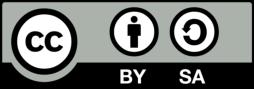
An empirical study on machine learning algorithms for heart disease prediction (Tsehay Admassu Assegie) 1067 (SVM), logistic regression (LR), decision tree (DT), and random forest (RF). The RFECV method is used to determine the most relevant risk factor heart disease features that are important for improving the prediction outcome of SVM, LR, DT, and RF. Generally, the goal of this research is to investigate the number of heart disease features required to develop a more accurate and computationally efficient model for heart disease prediction. In addition, the variability of the performance of SVM, LR, DT, and RF models for heart disease prediction is explored using the implemented RFECV method. This research follows an empirical methodology to experiment using RFECV for feature selection and SVM, LR, DT, and RF for model development using real world data obtained from the University of California Irvine (UCI) heart disease data repository. The objectives of this research are to answer the questions shortlisted: i) what is the optimal number of heart disease feature that maximizes the performance of the SVM, DT, RF and LR model for heart disease prediction?; ii) what is the impact of varying the number of features, on the performance of SVM, DT, RF and LR model for heart disease prediction?; and iii) among SVM, LR, DT and RF which predictive model has high variability of cross validation score for varying number of heart disease features?
Despite the wide application of supervised machine learning algorithms to heart disease datasets for implementation of an automated intelligent model for heart disease prediction [8] [10], literature shows that heart disease feature has an impact on heart disease prediction performance of the predictive model and feature selection also reduces the computational cost such as time and memory space. Thus, heart disease symptom or feature, which is important to represent a heart disease sample, has to be determined to improve the performance of the machine learning model for heart disease prediction. Thus, this research focused on the implementation automation of heart disease diagnosis with the RFECV method to obtain a better result on heart disease prediction using SVM, LR, DT, and RF models.
Heart disease is a cardiovascular disease that causes death all over the world [2]. The identification of heart disease is difficult using common heart disease risk factors such as high blood pressure, high cholesterol level, age, sex, and serum cholesterol. Overall, the characteristics of heart disease are complex and some of the heart diseases features overlap with other diseases such as chronic kidney disease. Thus, the identification of heart disease requires caution and heart disease treatment requires highly experienced cardiologists which is usually costly and requires much time and human effort
3. METHOD To conduct this study, the authors reviewed recently published articles in reputed international scholarly journals indexed in Scopus. Then we collected clinical heart disease records and conducted exploratory data analysis using statistical methods such as correlation analysis and descriptive statistics. Much of the research work in the literature [11] [16] has employed RF, SVM, LR, and DT to predict the framework of heart disease diagnosis. Based on the literature survey, we have selected the four most popular supervised machine learning algorithms namely, SVM, DT, RF, and LR to conduct experimental research on the performance of SVM, LR, DT, and RF The heart disease dataset is collected from the UCI machine
2. LITERATURE REVIEW
Int J Artif Intell ISSN: 2252 8938
Recently, machine learning is gaining importance in the health care industry as one of the means to combat the long term effect of heart disease on society [3] [5]. The higher precision, high performance, and cost effectiveness is the major advantage of the predictive model in heart disease identification. With more and more patients admitted to hospitals, the diagnosis of heart disease is becoming more challenging. One of the major challenges in heart disease identification is that highly experienced cardiologists are required to identify heart disease accurately. However, training humans requires much effort and time usually, many years for healthcare clinicians to gain the necessary skill and experience in heart disease identification. Thus, machine learning has become not only an alternative solution to replace human experts in heart disease identification, but also a necessity to aid the decision making process during heart disease identification. In this study, the authors developed a predictive model for heart disease diagnosis using supervised learning algorithms specifically, SVM, LR, DT, and RF. The authors have also experimented on the developed model using the heart disease dataset collected from the UCI data repository. In [6], the researchers evaluated the performance of DT, RF, and artificial neural network (ANN) for heart disease diagnosis using the UCI heart disease data repository. The experimentation on DT, RF, and ANN shows that artificial neural network outperforms as compared to DT and RF model for heart disease detection. Overall, the predictive performance of 85.03%, 79.93%, and 79.93% is achieved with an artificial neural network, DT, and RF model respectively. Thus, the performance of an artificial neural network is better compared to DT and RF models. Moreover, in another study [7], the researchers applied RF to develop a predictive model that predicts heart disease. In addition, the authors experimented on the model with a heart disease test set and the result shows that the performance of the model achieved an accuracy of 94.03%.
ISSN: 2252 8938 Int J Artif Intell, Vol. 11, No. 3, September 2022: 1066 1073 1068learning data repository, which is one of the most popular machine learning data repositories for conducting experimental research in machine learning research [17] [21]. The heart disease dataset employed in this study is demonstrated in Table 1.Table 1. Heart disease dataset characteristics Data source No. of instances No. of patients No. of non patients No. of classes UCI data repository 1025 526 498 2 (patient and healthy)
Table 2. Heart disease dataset description
Feature Data type Description Value Age Numeric Age of patient Mean=54, Max=77, Min=29 Sex Nominal Patient’s gender (1=male,0=female) 1=Male, 0=Female restecg Numeric Blood pressure in mmHg 0=Normal, 1=Having ST T, 2=Showing probability Cholesterol (chol) Numeric Continuous value in mm/dl Mean=246, Max=564, Min=126 Fasting blood pressure (fbs) Nominal Level of sugar in blood >120 mg/dl (1=yes, 0=no) >120 mg/dl, 1=Yes, 0=No Heart rate achieved (thalach) Numerical Heart rate in mmHg Mean=149, Max=202, Min=71 Thallium scan(thal) Nominal Nominal (3=Normal,6=fixed defect,7=Reversible defect) Exerciseangina(exang)induced Nominal Nominal (presence of exercise angina,1=present,0=absent)induced 1=Yes, 0=No Slope (slope) Nominal Nominal (1=Up slopping,2=Flat,3=Down slopping) 1=Up slopping, 2=Flat, 3=Down slopping Status of fluoroscopy (ca) Nominal Nominal (number of vessels colored through X ray (0 to 3)) Continuous values (0 3) Chest pain (cp) Nominal Nominal (With chest pain=1, no chest pain=0) 1=Typical, 2=Atypical, 3=Non angina, 4=Asymptomatic oldpeak S T depression induced by exercise relative to rest nominal (0 to 6) Mean=1.07, Max=6.2, Min=0 Resting blood (tresbps)pressure Nominal Blood pressure at rest Mean=131, Max=200, Min=94 Target Nominal Predicted class 1=Patient, 0=Healthy or not patient 3.2. Correlation model To get insight into the heart disease dataset and explore the dependency or collinearity that exists among heart disease features we have employed Pearson correlation to the heart disease dataset for exploratory data analysis. Figure 1 demonstrates the correlation circle for heart disease features. Pearson correlation among each heart disease feature is determined by using the Pearson’s correlation formula given in (1) [22] [24]: �� = ∑(���� ��)(���� ��) √∑(���� ��)(���� ��) (1) where r denotes Pearson correlation coefficient, xi denotes values of the variable x in the heart disease dataset, Yi denotes values of the variable y in the heart disease dataset, x denotes the mean of variable x and y denotes the mean of the values of variable y in the heart disease dataset. Figure 1, shows the correlation of heart disease dataset features. Total resting blood pressure, age, fasting blood sugar, cholesterol, and quantity of main vessels colored by fluoroscopy have a positive correlation. In addition, sex, heart rate, exercise induced angina, oldpeak, and heart rate have a positive correlation to each other. Similarity, chest pain, slope and maximum heart rate achieved has a positive correlation to each other. In contrast, chest pain, maximum heart rate, and slope are negatively correlated to sex, heart rate, exercise induced angina, oldpeak, and heart rate. Similarly, resting electrocardiography has a
The UCI heart disease dataset consists of 1,025 sample data points, each data point or sample is described by 13 heart disease features described in Table 2. The authors have considered 70% of the dataset or 717 samples and the remaining 30% or 308 data samples are used for testing. In addition, the dataset consists of balanced observations of the patient and non patient class distribution.
3.1. UCI heart disease feature description
Figure
Figure 2 proves that the performance of the SVM and RF model is highly affected by the heart disease feature used for model training. Figures 2(a) and 2(b) and Figures 3(a) and 3(b) show the RFECV curve of the proposed model, the SVM achieves a higher accuracy when 11 informative heart disease features are used as shown in Figures 2(a), then gradually decreases the accuracy as the non informative heart disease features are added into the model. Similarly, RF achieves a higher accuracy when 11 informative heart disease features are used as shown in Figure 2(b). In addition, the shaded region represents the variability of cross validation or standard deviation above and below the mean accuracy score drawn by the cross validation curve. We see from Figures 2(a) and 2(b) that there is a high variability of cross
4. RESULTS AND DISCUSSION This section presents the results such as accuracy and cross validation variability between SVM, LR, DT, and RF for varying features. The performance of SVM, DT, RF, and LR is evaluated using accuracy, receiver operating characteristics the area under the curve (AUC), and average precision. In experimentation, the model is tested against a varying number of input features. Cross validated accuracy is computed for different input feature sizes and the result is compared.
Int J Artif Intell ISSN: 2252 8938 An empirical study on machine learning algorithms for heart disease prediction (Tsehay Admassu Assegie) 1069 negative correlation to total resting blood pressure, age, fasting blood sugar, cholesterol, and quantity of main vessels colored X ray. 1. Correlation graph for heart disease features
3.3. RFECV Recursive feature elimination (RFE) is a feature selection technique that fits the model and eliminates less important features (or features) until the specified number of features is selected. Features are ranked by their important characteristics, and by recursively removing a small number of features per loop, RFE eliminates dependencies or collinearity that exists between the features [25]. To determine the relevant number of heart disease features, we have employed RFE with cross validation or RFECV to compute the cross validation score on the selected heart disease feature.
4.1. The effect of heart disease input feature size on classifier performance To determine the optimal number of heart disease features, cross validation is used with RFE to score different feature subsets and select the best performing collection of heart disease features. The RFECV is demonstrated in Figures 2(a) and 2(b) and Figures 3(a) and 3(b) show the number of heart disease features in the SVM, R, DT, and LR models along with their cross validated test score and variability respectively. Moreover, Figures 2(a) and 2(b) and Figures 3(a) and 3(b) demonstrate the selected number of heart disease features for each model.
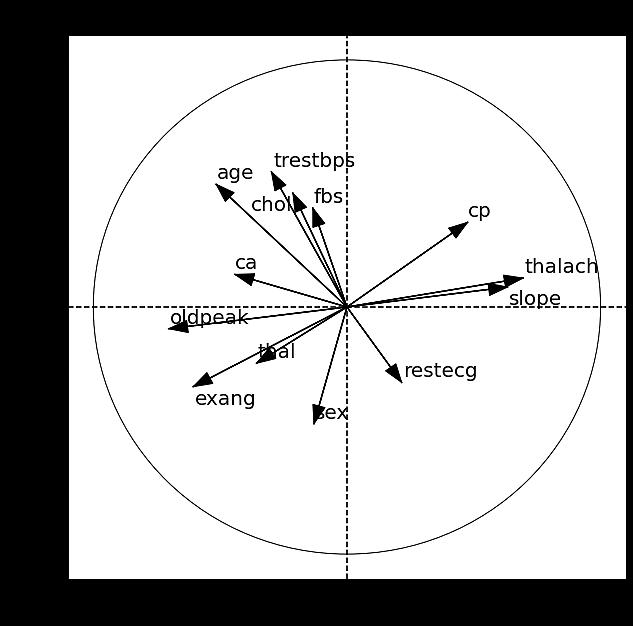
scores with varying heart disease features using SVM as compared to the RF model on heart disease prediction.
(b)(a)
Figure 2 Effects of selecting heart disease feature on the performance of classier (a) SVM and (b) RF We see from Figures 3(a) and 3(b) that DT achieves a higher accuracy when 8 informative heart disease features are used as shown in Figure 3(a), then remained constant accuracy as the non informative heart disease features are added into the model. Similarly, LR achieves a higher accuracy when 10 informative heart disease features are used as shown in Figure 3(b). In addition, we see from Figures 3(a) and Figure 3(b) that there is a high variability of cross validation scores with varying heart disease features using LR as compared to the DT model on heart disease prediction.
ISSN: 2252 8938 Int J Artif Intell, Vol. 11, No. 3, September 2022: 1066 1073
1070validation
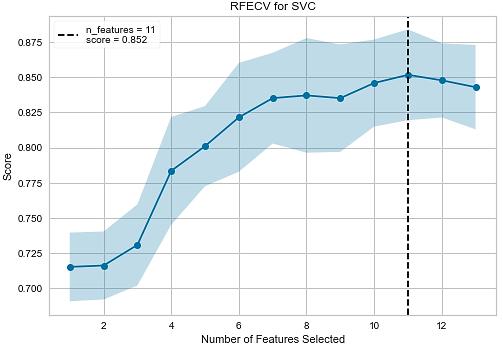

Model No. of features Highest accuracy Avg. AUC Avg. precision LR 10 85.8% 0.88 0.94 SVM 11 85.2% 0.84 0.86 DT 8 99.6% 0.96 1.00 RF 11 99.7% 1.00 1.00
(b)(a)
Int J Artif Intell ISSN: 2252 8938 An empirical study on machine learning algorithms for heart disease prediction (Tsehay Admassu Assegie) 1071
Figure 3 Effects of selecting heart disease feature on the performance of classier (a) DT and (b) LR
4.2. Comparison between the accuracy of the model
The authors employed accuracy as a performance metric to evaluate and compare the performance of SVM, DT, LR, and RF models. In comparison, the highest accuracy achieved by each model is different. In addition to accuracy variation across the different models, the experimental result appears to prove that the model performance varies for different features. Table 3 illustrates the variation in the performance of the model for a varying input feature.Table 3. Heart disease dataset feature description
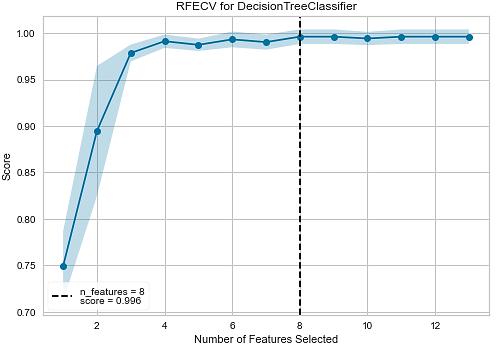
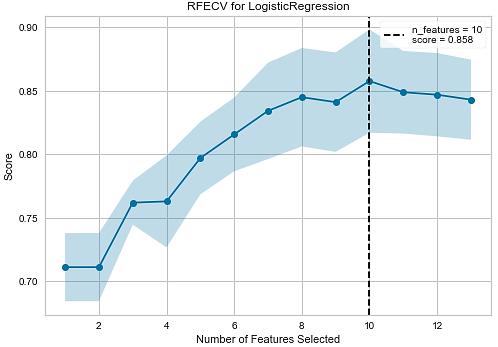
[4] S. Sun, Z. Cao, H. Zhu, and J. Zhao, “A survey of optimization methods from a machine learning perspective,” IEEE Trans. Cybern., vol. 50, no. 8, pp. 3668 3681, Aug. 2020, doi: 10.1109/TCYB.2019.2950779.
[14] T. A. Assegie, R. L. Tulasi, and N. K. Kumar, “Breast cancer prediction model with decision tree and adaptive boosting,” IAES Int. J. Artif. Intell., vol. 10, no. 1, pp. 184 190, Mar. 2021, doi: 10.11591/ijai.v10.i1.pp184 190. [15] C. A. Cheng and H. W. Chiu, “An artificial neural network model for the evaluation of carotid artery stenting prognosis using a national wide database,” in 2017 39th Annual International Conference of the IEEE Engineering in Medicine and Biology Society (EMBC), Jul. 2017, pp. 2566 2569, doi: 10.1109/EMBC.2017.8037381.
[11] R. Sigit, A. Basuki, and Anwar, “A new feature extraction method for classifying heart wall from left ventricle cavity,” Int. J. Adv. Sci. Eng. Inf. Technol., vol. 10, no. 3, p. 964, Jun. 2020, doi: 10.18517/ijaseit.10.3.12152.
[17] F. S. Alotaibi, “Implementation of machine learning model to predict heart failure disease,” Int. J. Adv. Comput. Sci. Appl., vol. 10, no. 6, pp. 261 268, 2019, doi: 10.14569/IJACSA.2019.0100637.
ACKNOWLEDGEMENTS
[20] S. J. Sushma, T. A. Assegie, D. C. Vinutha, and S. Padmashree, “An improved feature selection approach for chronic heart disease detection,” Bull. Electr. Eng. Informatics, vol. 10, no. 6, pp. 3501 3506, Dec. 2021, doi: 10.11591/eei.v10i6.3001.
[18] A. M. Alqudah, M. AlTantawi, and A. Alqudah, “Artificial intelligence hybrid system for enhancing retinal diseases classification using automated deep features extracted from OCT images,” Int. J. Intell. Syst. Appl. Eng., vol. 9, no. 3, pp. 91 100, Sep. 2021, doi: 10.18201/ijisae.2021.236.
In this study, the authors conducted an empirical study on the performance of machine learning for heart disease prediction using SVM, LR, DT, and RF. Furthermore, we have employed RFECV to select optimal input features to obtain better heart disease diagnosis outcomes. With RFECV, we have determined the optimal number of heart disease features that maximize the heart disease diagnosis outcome of the proposed model. In addition, the proposed model is compared to the existing model and the experimental result shows that the RF model outperforms as compared to DT, SVM, and LR. Overall, a random forest model was performed with a classification accuracy of 99.7%. The performance of RF, DT, SVM, and LR models is 99.7% and 99.6%. 85.2% and 85.8% respectively
[8] J. H. Joloudari et al., “Coronary artery disease diagnosis; ranking the significant features using a random trees model,” Int. J. Environ. Res. Public Health, vol. 17, no. 3, p. 731, Jan. 2020, doi: 10.3390/ijerph17030731.
[10] R. C. Chen, C. Dewi, S. W. Huang, and R. E. Caraka, “Selecting critical features for data classification based on machine learning methods,” J. Big Data, vol. 7, no. 1, Dec. 2020, doi: 10.1186/s40537 020 00327 4.
[1] T. A. Assegie, R. L. Tulasi, V. Elanangai, and N. K. Kumar, “Exploring the performance of feature selection method using breast cancer dataset,” Indones. J. Electr. Eng. Comput. Sci., vol. 25, no. 1, pp. 232 237, Jan. 2022, doi: 10.11591/ijeecs.v25.i1.pp232 237. [2] T. A. Assegie, “An optimized k nearest neighbor based breast cancer detection,” J. Robot. Control, vol. 2, no. 3, pp. 115 118, 2021, doi: 10.18196/jrc.2363. [3] R. Spencer, F. Thabtah, N. Abdelhamid, and M. Thompson, “Exploring feature selection and classification methods for predicting heart disease,” Digit. Heal., vol. 6, p. 205520762091477, Jan. 2020, doi: 10.1177/2055207620914777.
[5] R. Aggrawal and S. Pal, “Sequential feature selection and machine learning algorithm based patient’s death events prediction and diagnosis in heart disease,” SN Comput. Sci., vol. 1, no. 6, Nov. 2020, doi: 10.1007/s42979 020 00370 1.
REFERENCES
[6] A. Niakouei, M. Tehrani, and L. Fulton, “Health disparities and cardiovascular disease,” Healthcare, vol. 8, no. 1, Mar. 2020, doi: 10.3390/healthcare8010065.
[9] T. R. S. Mary and S. Sebastian, “Predicting heart ailment in patients with varying number of features using data mining techniques,” Int. J. Electr. Comput. Eng., vol. 9, no. 4, p. 2675, Aug. 2019, doi: 10.11591/ijece.v9i4.pp2675 2681.
ISSN: 2252 8938 Int J Artif Intell, Vol. 11, No. 3, September 2022: 1066 1073 1072 5. CONCLUSION
The authors would like to thank Inijbara University for providing internet facilities and laboratory equipment (Laptop) for conducting this work.
[7] L. J. Muhammad, I. Al Shourbaji, A. A. Haruna, I. A. Mohammed, A. Ahmad, and M. B. Jibrin, “Machine learning predictive models for coronary artery disease,” SN Comput. Sci., vol. 2, no. 5, Sep. 2021, doi: 10.1007/s42979 021 00731 4.
[12] C. R, “Heart disease prediction system using supervised learning classifier,” Bonfring Int. J. Softw. Eng. Soft Comput., vol. 3, no. 1, pp. 1 7, Mar. 2013, doi: 10.9756/BIJSESC.4336.
[13] D. Khanna, R. Sahu, V. Baths, and B. Deshpande, “Comparative study of classification techniques (SVM, logistic regression and neural networks) to predict the prevalence of heart disease,” Int. J. Mach. Learn. Comput., vol. 5, no. 5, pp. 414 419, Oct. 2015, doi: 10.7763/IJMLC.2015.V5.544.
[21] K. Budholiya, S. K. Shrivastava, and V. Sharma, “An optimized XGBoost based diagnostic system for effective prediction of heart disease,” J. King Saud Univ. Comput. Inf. Sci., Oct. 2020, doi: 10.1016/j.jksuci.2020.10.013. [22] T. Suresh, T. A. Assegie, S. Rajkumar, and N. Komal Kumar, “A hybrid approach to medical decision making: diagnosis of heart disease with machine learning model,” Int. J. Electr. Comput. Eng., vol. 12, no. 2, pp. 1831 1838, Apr. 2022, doi: 10.11591/ijece.v12i2.pp1831 1838. [23] I. Javid, A. Khalaf, and R. Ghazali, “Enhanced accuracy of heart disease prediction using machine learning and recurrent neural networks ensemble majority voting method,” Int. J. Adv. Comput. Sci. Appl., vol. 11, no. 3, pp. 540 551, 2020, doi: 10.14569/IJACSA.2020.0110369.
[16] E. Nikookar and E. Naderi, “Hybrid ensemble framework for heart disease detection and prediction,” Int. J. Adv. Comput. Sci. Appl., vol. 9, no. 5, pp. 243 248, 2018, doi: 10.14569/IJACSA.2018.090533.
[19] Z. Khandezamin, M. Naderan, and M. J. Rashti, “Detection and classification of breast cancer using logistic regression feature selection and GMDH classifier,” J. Biomed. Inform., vol. 111, Nov. 2020, doi: 10.1016/j.jbi.2020.103591.
[25] R. Naseem et al., “Performance assessment of classification algorithms on early detection of liver syndrome,” J. Healthc. Eng., vol. 2020, pp. 1 13, Dec. 2020, doi: 10.1155/2020/6680002.
Mr. Tsehay Admassu Assegie holds a Master of Science degree from Andhra University, India 2016. He also received his B.Sc. (Computer Science) from Dilla University, Ethiopia in 2013. His research includes machine learning, data mining, bioinformatics, network security and software defined networking. He has published over 33 papers in international journals and conferences. He can be contacted at email: tsehayadmassu2006@gmail.com.
Int J Artif Intell ISSN: 2252 8938 An empirical study on machine learning algorithms for heart disease prediction (Tsehay Admassu Assegie) 1073
BIOGRAPHIES OF AUTHORS
Mr. Prasanna Kumar Rangarajan is presently serving as Faculty in the Department of Computer Science and Engineering, Amrita Vishwa Vidyapeetham Chennai. He has 20 years of teaching experience. His area of interest includes the theory of computation, compiler design, machine learning and data science. He can be contacted at email: r_prasannakumar@ch.amrita.edu.
[24] P. C. Kaur, “A study on role of machine learning in detectin heart diseas,” in 2020 Fourth International Conference on Computing Methodologies and Communication (ICCMC), Mar. 2020, pp. 188 193, doi: 10.1109/ICCMC48092.2020.ICCMC 00037.
Mr. Napa Komal Kumar is currently working as an Assistant Professor in the Department of Computer Science and Engineering at St. Peter’s Institute of Higher Education and Research, Avadi, Chennai. His research interests include Machine Learning, Data Mining, and Cloud Computing. He can be contacted at email: komalkumarnapa@gmail.com.
Vigneswari Dhamodaran is currently working as an Assistant Professor in the Department of Information Technology, KCG College of Technology, Chennai, Tamil Nadu, India. Her research interests include data mining, and machine learning. She can be contacted at email: vigneswari121192@gmail.com.
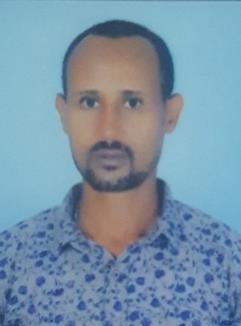



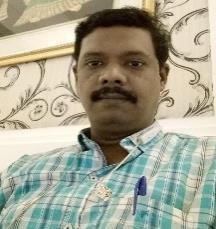



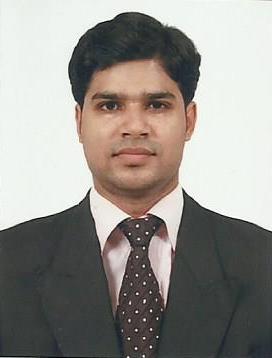



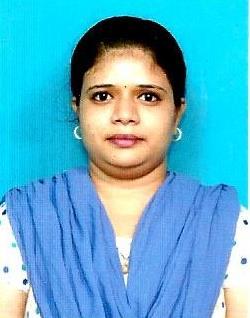



IAES International Journal of Artificial Intelligence (IJ AI) Vol. 11, No. 3, September 2022, pp. 1074 1084 ISSN: 2252 8938, DOI: 10.11591/ijai.v11.i3.pp1074 1084 1074
Computer Science, BINUS Graduate Program Master of Computer Science Bina Nusantara University, Jakarta, Indonesia 11480
Article Info ABSTRACT
Journal homepage: http://ijai.iaescore.com
Hardware sales forecasting using clustering and machine learning approach
Rani Puspita, Lili Ayu Wulandhari Department of Computer Science, BINUS Graduate Program Master of Computer Science, Bina Nusantara University, Jakarta, Indonesia
This is an open access article under the CC BY SA license. Corresponding Author: Rani DepartmentPuspitaof
Article history: Received Nov 2, 2021 Revised Jun 1, 2022 Accepted Jun 20, 2022 This research is a case study of an information technology (IT) solution company. There is a problem that is quite crucial in the hardware sales strategy which makes it difficult for the company to predict the number of various items that will be sold and also causes the excess or shortage in hardware stocking. This research focuses on clustering to group various of items and forecast the number of items in each cluster using a machine learning approach. The methods used in clustering are k means clustering, agglomerative hierarchical clustering (AHC), and gaussian mixture models (GMM), and the methods used in forecasting are autoregressive integrated moving average (ARIMA) and recurrent neural network long short term memory (RNN LSTM). For clustering, k means uses two attributes, namely "Quantity and Stock" as the best feature in this case study. Using these features the k means obtain silhouette results of 0.91 and davies bouldin index (DBI) values of 0.34 consisting of 3 clusters. While for forecasting, RNN LSTM is the best method, where it produces more cost savings than the ARIMA method. The percentage of the difference in saving costs between ARIMA and RNN LSTM to the actual cost is 83%.
MachineHardwareForecastingEvaluationClusteringsaleslearning
Email: rani.puspita@binus.ac.id 1. INTRODUCTION Business lately has become very fast and growing. This makes companies compete with each other [1] Many companies are engaged in information technology (IT) Solutions. One of the businesses whose development will always increase is the hardware sales business. With the help of sales in marketing products, both hardware and software, it really helps the company financially. But so far, the company has had quite a crucial problem in its hardware sales strategy which makes it difficult for the company to predict the number of items to be sold and also sometimes causes the company to experience excess or shortage in hardwareAinventory.ccordingto [2] sales forecasting can be used for companies or other to anticipate things that will come. If the company does wrong in forecasting sales, things can happen that are not desirable. For example, the company cannot meet the sudden increase in consumer demand. Or maybe consumer demand is not in accordance with the company's estimates so that the existing goods are not sold. In other words, the company may experience excess stock of goods. This of course can bring losses to the company. This is in line with the opinion [3]. According to him, sales forecasting is very important to do. Sales forecasting refers to predicting the future by assuming factors in the past (in this case it can be data from the past) that will have an influence in the future. Clustering also very much needed to see hardware cluster which one is classified
Keywords:
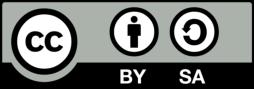
Nadeak and Ali [16] Apriori Data mining, association, Apriori algorithm, testing. Successfully utilize artificial intelligence techniques in drug sales Edastama et al. [17] Apriori Data cleaning, data integration, data selection. Succeeded in obtaining information on the most in demand items so that it can be used to increase sales growth and marketing of eyewear.
Based on Table 1, it can be concluded that in the sale of goods, analysis needs to be carried out to assist the company in managing sales strategies and of course helping the company in increasing revenue.
Researchers will use clustering and forecasting. In this study, researchers will use k means clustering, AHC and GMM in clustering and use ARIMA and RNN LSTM methods for forecasting. This research will certainly be able to increase profits for the company and can also help the company to find out the sales strategy for the following year.
Int J Artif Intell ISSN: 2252 8938
Successfully implemented the k means clustering algorithm for the sales cluster. The results of testing using DBI values produces a value of 0.2.
Researchers read and conduct literature studies in journals related to the chosen research topic. It aims to learn all things related to research and to assist researchers in identifying research problems so that this will support the course of research. The summary of the related works to data mining in sales is shown in Table 1, the summary of the related works to clustering is shown in Table 2 and the summary of the related works related to forecasting is shown in Table 3. Table 1 Related works for data mining in sales References Method Process Result Fithri Wardhanaand [13] k means clustering Data collection, data mining, perform analysis with k Means clustering, testing with davies bouldin index (DBI) values
Johannes Alamsyahand[14] Decision tree Cross industry standard process for data mining (CRISP DM) Managed to determine the prediction of the number of items sold by the viewers, the price, and the type of shoes.
Soepriyanto et al. [15] k nearest neighbor (k NN) and Naïve Bayes Data collection, implementation,analysis,testing Successfully predict stock prices. Naïve Bayes produces an accuracy value of 69.38, and the k NN method produces an accuracy value of 67.25%.
2. RELATED WORKS
Hardware sales forecasting using clustering and machine learning approach (Rani Puspita) 1075 as high, medium, or low according to the previously determined characteristics that become the reference for doing forecasting. In addition to using data on goods sold and existing stock, other characteristics in the data can also be used to analyze clusters of the hardware data sold. It aims for a promotional strategy that can be used by the company [4] The clustering method that is most widely used is k means clustering method [5]. K means is an algorithm used in grouping which separates data into different clusters. Basically the use of this algorithm depends on the data obtained and the conclusions to be reached at the end of the process. K means clustering is a method for performing clusters that are affected by the selection of the initial cluster centroid [6]. So that in the use of the k means clustering algorithm, there are two rules. The first rule is to determine the number of clusters that need to be included and the second is to have attributes of type numeric because clustering can only process numerical data [7]. Besides k means clustering, there is also agglomerative hierarchical clustering (AHC). AHC is a clustering technique that forms a hierarchy so as to form a tree structure. Thus, the grouping process is carried out in stages or stages. There are 2 methods in the hierarchical clustering algorithm, namely agglomerative (bottom up) and divisive (top down) [8]. In addition there are also gaussian mixture models (GMM). GMM is a model consisting of components of gaussian functions [9]. Then one method that is very popular and can be used for forecasting is using the recurrent neural network long short term memory (RNN LSTM) method. LSTM is a method that can be used to study a pattern in time series data. LSTM is a type of RNN [10]. This is in line with the opinion [11] which states that the LSTM performs better in practice. LSTM is universal in other words LSTM provides enough network units to be able to calculate whether a conventional computer can calculate, as long as it has the right weight matrix, which can be viewed as a program. There is also another algorithm with basic time series data, namely ARIMA. Autoregressive Integrated Moving Average (ARIMA) is a forecasting technique that uses a correlation technique between a time series. Then, the model finds patterns of correlation between series of observations [12]. Based on the background, a research focuses on the implementation of data mining with machine learning methods as a solution to sales problems that often experience excess or lack of stock in warehouses and to find out hardware sales strategies and increase turnover in the company.
It can be seen that GMM can help analyze the timing and energy signals of each sub pattern.
Rani et al. [19] k means gpatternfrequentandclustering,(FP)rowth Data collection, k means algorithm and FP Growth algorithm, analyzing data, implementing data
Table 3. The summary of the related works in forecasting References Method Process Result Xu et al. [23] ARIMA, and deep belief network (DBN) Data collection, training data, prediction, testing. Successfully demonstrated that the model has a high predictive accuracy and may be a useful tool for time series forecasting.
3. THEORY AND METHODS In clustering, researchers will use the k means clustering, AHC and GMM methods. Then for forecasting, researchers will use the ARIMA and RNN LSTM methods. The following is an explanation of the theory and methods for clustering and forecasting.
Successfully implemented the k means algorithm in classifying tourist visits to the city of pagar alam to increase visitors.
3.1. K means clustering K means clustering is one of the techniques of clustering in the data mining modeling process without supervision and method of grouping data by partition. The data are grouped into several groups and each group has characteristics that are similar to or the same as the others but with other groups having different characteristics [27]. In other words, k means clustering is a similar container of objects. If objects whose behavior is closer, they will be grouped in one class and those that are far or not similar are grouped in clusters different [27]. The clustering steps with the k means algorithm are: i) Define K/N clusters; ii) Initialize the centroid randomly; iii) Find the nearest object using Euclidean distance; iv) Recalculate the data in each cluster to get the mean; and v) Restore data and put it back it to centroid. If the data in the cluster does not change, then the step cluster stops but if the center cluster is still changing, then it must return to number 3 until the cluster does not change anymore. Steps of k means clustering can be seen in the process flow Figure 1. The formula Euclidean distance according to [28] is:
Successfully applied data mining techniques with the k means clustering method which aims to help students determine the correct course according to the established criteria
Rashid et al. [22] k andClustering,meansGMM Adding peripheral cluster, dataset description, conditioning on previous frames, adding constraints, combinatorial clustering (k means and GMM), determine K, data processing k means and GMM. Successfully applied the k means and GMM methods and tested the method using sparse multidimensional data obtained from the use of video game sales all around the world
Alabdulrazzaq et al. [26] ARIMA Analysis, ARIMAparametersARIMAoptimization,modelvalidation. Managed to apply the Arima model for the prediction of Covid 19 in Kuwait and get precise and good accuracy.
Succeeded in grouping student score data to make it easier for students to take expertise courses in the next semester
ISSN: 2252 8938 Int J Artif Intell, Vol. 11, No. 3, September 2022: 1074 1084 1076
Puspita Sasmitaand[18] k means clustering Determine the number of clusters, determine the centroid value of each cluster, calculate the distance between data, and calculate the minimum object distance
Irawan [20] k means clustering CRISP DM
Gupta et al. [24] Support vector machine (SVM), forecastingprophetmodel, and linear regression Data collection, perform SVM, perform linear regression, perform prophet forecasting model, train and test models.
Shen et al. [21] GMM Description of the operation dataset, analysis of heating load patterns, GMM clustering for heating load patterns, prediction model, dan evaluation of the proposed models.
Table 2. The summary of the related works in clustering References Method Process Result
The study predicts that there could be a second rebound of the pandemic within one year. Based on this research, this helps the government to act quickly.
Successfully predict active rate, death rate, and cured rate in India by analyzing COVID 19 data Malki et al. [25] ARIMA Dataset description, perform ARIMA models, model selection, data normalization, experimental result, and evaluation.
3.2. AHC Agglomerative hierarchical grouping is a hierarchical grouping method with approach bottom up. The grouping process starts from each data as a group, then recursively looks for the closest group as a pair to join as a large group. This is in line with the opinion of Krisman et al. that hierarchical clustering is a technique of clustering that forms a hierarchy so as to form a tree structure. Thus, the grouping process is carried out in stages or stages. There are 2 methods in the algorithm, hierarchical clustering namely Agglomerative (bottom up) and Divisive (top down) [29]. The steps of the AHC method are: i) Calculate euclidien distance; ii) Merge the two closest clusters; iii) Update the distance matrix according to the agglomerative clustering method. For example, using single linkage, average linkage, or complete linkage; iv) Repeat steps 2 and 3 to get define number of clusters; and v) Output of clustering.
3.3. GMM Gaussian mixture models is a type of density model consisting of components of functions Gaussian [9]. GMM is applied to study the distribution parameters based on the optimal threshold that corresponds to the minimum calculated error probability [21]. GMM is an accurate method and the number of clusters is predetermined [30] This is in line with the opinion [31] that GMM is a method that can be used for data clustering. GMM is a mathematical model that attempts to estimate the probability density of a data
Int J Artif Intell ISSN: 2252 8938 Hardware sales forecasting using clustering and machine learning approach (Rani Puspita) 1077 ��(����,����)=√∑ (���� ����) ��2 ��=1 (1) dWhere: = Distance i = Number of data y = Centroid x = Data Start Determinethenumberofclusters Determinethecenterofthecluster Groupobjectsbasedontheclosestdistance Recalculatecentroidstogetnewcentroids ClusterFinishChanging? No Yes Figure 1. Flow process k means clustering
ISSN: 2252 8938 Int J Artif Intell, Vol. 11, No. 3, September 2022: 1074 1084 1078distribution using a mixed finite distribution gaussian. Gaussian is the most widely used distribution. When GMM is used as a method clustering, then it will determine the number of clusters [32]. Expectation maximization (EM) algorithm is a method used for maximum likelihood to estimate distribution parameters. EM algorithm is an iterative method. EM consists of two steps: expectation (E step) and maximization (M step) [33]. The following is the formula for EM Algorithm: (��|��)=∑ ���� ��(��|����)�� ��=1 (2) The steps Input:are:Training dataset Initialize: j, j, ∑j for each j distribution function Repeat: • E Step: ������ =��(��|�� )= ��(��)��(�� |��) ��(�� ) (3) • M Step (update parameter): ���� = 1 ∑ ������,�� = ∑ ����1 ∑ ������ ,∑�� = ∑ ������ (���� ����)(���� ����) ���� ��=1 ∑ is an explanation of = covariance matrix = = proportion 3.4. RNN-LSTM RNN is a machine learning architecture that has a combination of networks in loops. Networks loop allow information to remain [34]. The method RNN method is known to be able to process sequential text data. RNN has three layers, namely input layer, output layer and hidden layer [35]. Figure 2 is an illustration of the RNN architecture. The LSTM method was first proven by Hochreiter and Schmidhuber in 1997. LSTM is a method that can be used to study a pattern in time series data. LSTM is a type of Recurrent Neural Network [34]. In LSTM architecture, content cells are more complex than RNN. In LSTM there are three gates, including input, forget and output gates. The input gate aims to enter new data, while erasing unimportant information contained in the forget gate and affecting the output at the same time is the task of the output gate. Figure 3 is an illustration of the LSTM architecture.
Mixture ratio p(x|θj) = Mixture components xi = Random variable π
��
��
��
��
the symbols: ∑
Variance
Mixture
�� ��=1
��
������ �� ��=1 �� ��=1 (4) Do this until the parameters do not change Below
���� �� ��
The following is the formula for ARIMA: Autoregressive (AR): ���� = ��0 +��1 ���� 1 +��2 ���� 2 + + ���� ���� �� +∈�� (5) Moving Average (MA): ���� = +∈�� ��1 ∈�� 1 ��2 ∈�� 1 ���� ∈�� �� or Y AR (6)
j = Mean wij
��
3.5. ARIMA Autoregressive Integrated moving average is a model that ignores the variables independent as a whole in forecasting [36]. The ARMA model is a combination of the autoregressive (AR) and moving average (MA). The AR model is a method to see the movement of a variable through the variable itself while the MA model is used to find out the movement of a variable with its residuals in the past [37]. ARIMA is also known as the time series method Box Jenkins. ARIMA is well known in forecasting time series [38]
The framework is a logical sequence to solve a research problem as outlined in a diagram flow from beginning to end, so that research can run systematically according to the concepts that have been made. The research framework for implementing data mining in clustering and forecasting will be outlined in Figure 4. Based on the framework in Figure 4, the first stage in the research that must be carried out is a literature review. Literature review is very important to identify problems and determine the objectives of the research.
Int J Artif Intell ISSN: 2252 8938 Hardware sales forecasting using clustering and machine learning approach (Rani Puspita) 1079 Autoregressive and moving average: ���� = ��0 +��1 ���� 1 +��2 ���� 2+....+���� ���� �� +∈�� ��1 ∈�� 1 ��2 ∈�� 1 ���� ∈�� �� (7) The symbols are described: Yt 1 = Time series data in the time period (t 1) θ1, 2, p = Coefficient Y = True value in period t Figure 2. RNN architecture Figure 3. LSTM architecture
4. RESEARCH METHODOLOGY
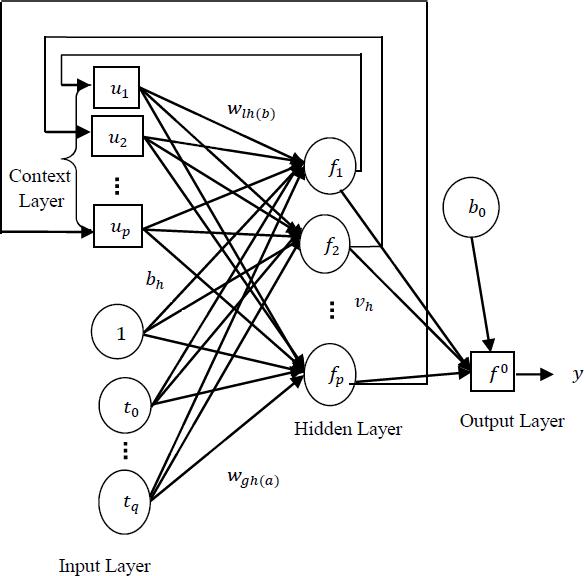
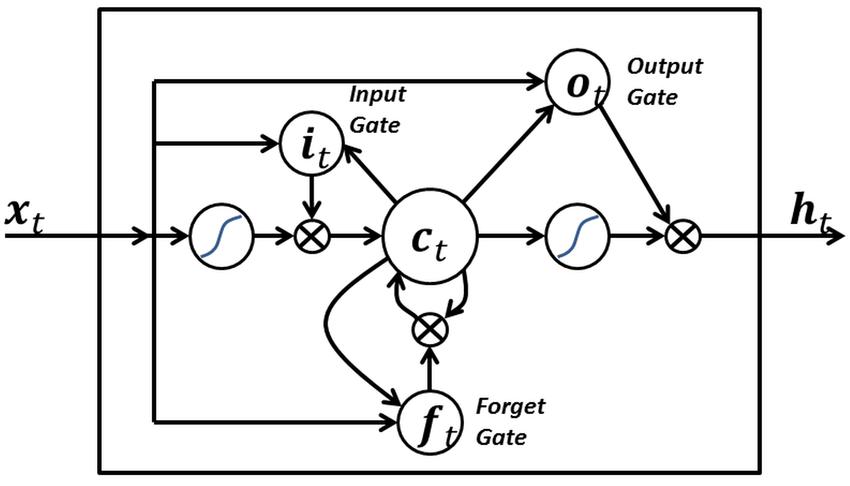
LiteratureReview Identificationof Problem DataCollectionandDataAnalysis ClusteringModel ClusteringModelEvaluation Qualify? ForecastingModel ForecastingModel Evaluation Qualify? End No Yes No Yes Figure 4. Research methodology 5.
Start PROPOSED METHODS
review was conducted to collect previous research journals on sales and to collect journals on methods of machine learning in the form of clustering and forecasting. From the literature review, the researcher concludes that for clustering, the researcher will use the k means clustering, AHC and GMM methods where these methods are frequently used methods and produce an accurate evaluation in terms of clustering. Then for forecasting, the methods used are ARIMA and RNN LSTM methods. Where the method is a method that is very often used and produces an accurate evaluation in terms of forecasting. After conducting a literature review, it is continued by identifying problems in the research and proceeding to the stage of data collection and analysis. Then proceed with modeling. In modeling, the first thing to do is clustering using k means clustering, AHC and GMM. After that, evaluate with each method. After evaluating clustering, the next step is to determine model clustering which is the best. After that, it is continued by doing forecasting using ARIMA and RNN LSTM based on the results of clustering. Then evaluate the forecasting and determine the model forecasting best based on the evaluation that has been done. When the best method for has been selected clustering and forecasting, and the research objectives have been achieved, the process is complete.
ISSN: 2252 8938 Int J Artif Intell, Vol. 11, No. 3, September 2022: 1074 1084
1080Literature
Proposed methods aims to describe what solutions are proposed to the problems that have been described in the background section. There are five activities in this sub chapter. For more details, in this session, the design and manufacture of solutions will be carried out which is illustrated in Figure 5. The first step to do is to prepare the dataset. Then do pre processing, for example by normalizing the data. After the data is deemed sufficient for modeling, the next step is to do clustering using the methods
The Following is a summary obtained from several clustering scenarios that have been carried out. There is some information such as Method, Number of Clustering, Attribute, Silhouette, and DBI Values. A summary of clustering is in Table 4.
Hardware sales forecasting using clustering and machine learning approach (Rani Puspita) 1081 k means clustering, AHC and GMM and then train the data. After that, the results will be obtained clustering from each method and the next step is to evaluate the model of each method. The evaluation will use silhouettes and DBI values. Silhouette refers to a method of validation of consistency within clusters of data. Then the results of the evaluation of the two methods are compared and seen which method clustering is better. When the results are obtained clustering best, the next step is forecasting with ARIMA and RNN LSTM. The first stage in forecasting is to train the data. After that, the results will be obtained forecasting sales of hardware from each method. The RNN LSTM method uses Python. The first step is to define a library. In this method, Scikit learn is used. Then there is the sequential class which is part of the Keras library which aims to connect between layers. This method activates the LSTM and dense layer. The dense layer output is 1 neuron. The hidden layer is in the form of a 3D input layer using numpy reshape. The activation function uses ReLU, optimization uses Adam, and the number of epochs is 50. Then evaluate the model of each method and compare the evaluation results. Evaluation forecasting using root mean square error (RMSE), and calculate the amount of saving cost from each forecasting model. RMSE is a calculation between actual and predicted. RMSE which has a small value is more accurate than RMSE which has a large value. Then when the evaluation results are visible, the next step is to compare which method is better for forecasting. After comparing and choosing the method, the researcher will know which method is the best for clustering and forecasting. In addition, after determining the best method from the evaluation results, the main objective in this research will also be achieved, namely developing and implementing data mining sales of hardware with methods of machine learning to determine clustering of hardware sold and developing and implementing data mining for forecasting sales of Hardware based on clustering that has been made with methods of machine learning to determine stock hardware as a sales strategy for the company.
Int J Artif Intell ISSN: 2252 8938
ProposedMethods onDataTestingonDataTrainingPreparationDataClusteringClusteringTrainingDataonForecastingTestingDataonForecasting Start PrepareDataset PreProcessingand ExperimentDataAnalysiswithThreeClusteringMethodsDataTraining HardwareSales ClusteringResultsfor EachMethod EvaluationoftheModel ofEachMethod Comparisonof EvaluationResultsof EachMethod AlreadyGetting theBestClustering Method? ExperimentwithTwo ForecastingMethodsDataTraining HardwareSales ForecastingResultsfor EachMethod EvaluationoftheModel ofEachMethod Comparisonof EvaluationResultsof EachMethod AlreadyGetting theBestForecasting Method? End No Yes No Yes Figure 5. Flow diagram proposed methods
6. RESULTS AND DISCUSSION
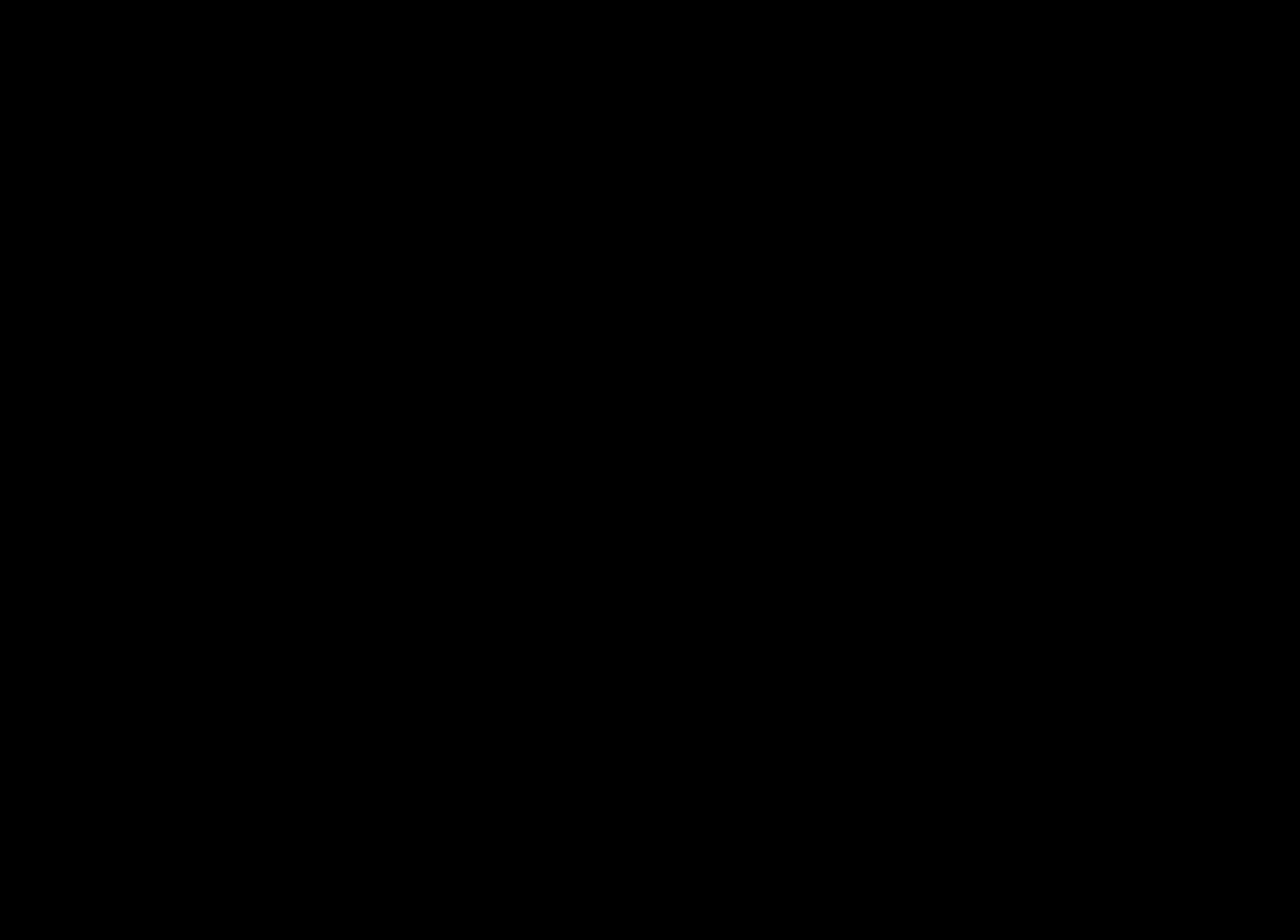
The authors thank to Bina Nusantara University for the research grant and supporting this research.
Table 5. Evaluation result for forecasting Method Detail Attribute Saving Cost ARIMA Forecasting Cluster 1 (above 10) Date, Quantity, Stock 100% ARIMA Forecasting Cluster 1 (below 10) Date, Quantity, Stock 85% ARIMA Forecasting Cluster 2 (above 10) Date, Quantity, Stock 64% ARIMA Forecasting Cluster 2 (below 10) Date, Quantity, Stock 99% ARIMA Forecasting Cluster 3 (above 10) Date, Quantity, Stock 88% ARIMA Forecasting Cluster 3 (below 10) Date, Quantity, Stock 100% RNN LSTM Forecasting Cluster 1 (above 10) Date, Quantity, Stock 100% RNN LSTM Forecasting Cluster 1 (below 10) Date, Quantity, Stock 86% RNN LSTM Forecasting Cluster 2 (above 10) Date, Quantity, Stock 64% RNN LSTM Forecasting Cluster 2 (below 10) Date, Quantity, Stock 99% RNN LSTM Forecasting Cluster 3 (above 10) Date, Quantity, Stock 80% RNN LSTM Forecasting Cluster 3 (below 10) Date, Quantity, Stock 100%
ACKNOWLEDGEMENTS
Clustering is done using three methods, namely k means clustering, AHC, and GMM. In each method three experiments were carried out. The first experiment uses the “Quantity and Stock” attribute, the second experiment uses the “Quantity, Stock and Price” attribute, then the third experiment uses the “Quantity, Stock, Price, and Customer” attribute. The best method for clustering is k means clustering using 2 attributes. with a silhouette of 0.91 and DBI values of 0.34. Then, forecasting is done using two methods including ARIMA and RNN LSTM. In each method, six experiments were carried out. The first experiment using training data and testing cluster 1 above 10, the second experiment uses training data and testing cluster 1 below 10, the third experiment uses training data and testing cluster 2 above 10, the fourth experiment uses training data and testing cluster 2 under 10, the fifth experiment uses training data and testing cluster 3 above 10 and the sixth experiment using training data and testing cluster 3 below 10. The best method for forecasting is RNN LSTM because it produces more cost savings than the ARIMA method. Percentage of saving cost for ARIMA against actual cost is 83% and percentage of saving cost for RNN LSTM against actual cost is 84%. The percentage of the difference in saving costs between ARIMA and RNN LSTM to the actual cost is 83%.
Based on the results of the experiment clustering and based on the summary of Table 4, k means clustering using two attributes, namely "Quantity and Stock", is the best method for the case study in this study. Evaluation for the k means clustering method obtained results silhouette of 0.91 and DBI values of 0.34 consisting of 3 clusters, namely 146 data for cluster 1, 3 data for cluster 2 and 3 data for cluster 3. And Table 5 is a summary of forecasting.Table
7. CONCLUSION
4. Evaluation result for clustering Method Number of Clustering Attribute Silhouette DBI Values k means clustering 3 Quantity, Stock 0.91 0.34 k means clustering 3 Quantity, Stok, Price 0.80 0.37 k means clustering 3 Quantity, Stok, Price, Customers 0.79 0.32 AHC 3 Quantity, Stock 0.87 0.50 AHC 3 Quantity, Stok, Price 0.80 0.37 AHC 3 Quantity, Stok, Price, Customers 0.79 0.28 GMM 3 Quantity, Stock 0.68 0.72 GMM 3 Quantity, Stok, Price 0.001 1 GMM 3 Quantity, Stok, Price, Customers 0.74 0.55
Based on Table 5, it can be seen the overall results of the forecasting evaluation. In addition, it is also known the amount of saving cost based on experiments using the ARIMA and RNN LSTM methods, it can be seen that the RNN LSTM method is better because it produces more cost savings than the ARIMA method. Percentage of saving cost against actual cost based on these two methods is 83%.
ISSN: 2252 8938 Int J Artif Intell, Vol. 11, No. 3, September 2022: 1074 1084 1082
[9] J. Gu, J. Chen, Qiming Zhou, and H. Zhang, “Gaussian mixture model of texture for extracting residential area from high resolution remotely sensed imagery,” ISPRS Work. Updat. Geo spatial Databases with Imag. 5th ISPRS Work. DMGISs, no. 1973, pp. 157 162, 2007. [10] M. Elsaraiti and A. Merabet, “A Comparative Analysis of the ARIMA and LSTM Predictive Models and Their Effectiveness for Predicting Wind Speed,” Energies, vol. 14, no. 20, 2021, doi: 10.3390/en14206782.
[8] R. T. Adek, R. K. Dinata, and A. Ditha, “Online Newspaper Clustering in Aceh using the Agglomerative Hierarchical Clustering Method,” Int. J. Eng. Sci. Inf. Technol., vol. 2, no. 1, pp. 70 75, 2021, doi: 10.52088/ijesty.v2i1.206.
[18] D. Puspita and S. Sasmita, “Application of K Means Algorithm in Grouping of City Tourism City Pagar Alam,” Sinkron, vol. 7, no. 1, pp. 28 32, 2022, doi: 10.33395/sinkron.v7i1.11220.
[7] R. D. Dana, D. Soilihudin, R. H. Silalahi, D. . Kurnia, and U. Hayati, “Competency test clustering through the application of Principal Component Analysis (PCA) and the K Means algorithm,” IOP Conf. Ser. Mater. Sci. Eng., vol. 1088, no. 1, p. 012038, 2021, doi: 10.1088/1757 899x/1088/1/012038.
[2] V. Sohrabpour, P. Oghazi, R. Toorajipour, and A. Nazarpour, “Export sales forecasting using artificial intelligence,” Technol. Forecast. Soc. Change, vol. 163, no. November, p. 120480, 2021, doi: 10.1016/j.techfore.2020.120480.
[13] F. A. Fithri and S. Wardhana, “Cluster Analysis of Sales Transaction Data Using K Means Clustering At Toko Usaha Mandiri,” J. PILAR Nusa Mandiri, vol. 17, no. 2, pp. 113 118, 2021. [14] R. Johannes and A. Alamsyah, “Sales prediction model using classification decision tree approach for small medium enterprise based on indonesian e commerce data,” Mar. 2021, doi: 10.48550/arXiv.2103.03117.
[1] O. Saritas, P. Bakhtin, I. Kuzminov, and E. Khabirova, “Big data augmentated business trend identification: the case of mobile commerce,” Scientometrics, vol. 126, no. 2, pp. 1553 1579, 2021, doi: 10.1007/s11192 020 03807 9.
[15] B. Soepriyanto, P. Studi, and S. Informasi, “Comparative analysis of K NN and naïve bayes methods to predict stock prices,” Int. J. Comput. Inf. Syst., vol. 2, no. 2, pp. 49 53, May 2021, doi: 10.29040/ijcis.v2i2.32.
Int J Artif Intell ISSN: 2252 8938 Hardware sales forecasting using clustering and machine learning approach (Rani Puspita) 1083
[6] D. A. N. Wulandari, R. Annisa, and L. Yusuf, “an Educational Data Mining for Student Academic Prediction Using K Means Clustering and Naïve Bayes Classifier,” Semin. Nas. Apl. Teknol. Inf., pp. 155 160, 2020, doi: 10.33480/pilar.v16i2.1432.
[11] J. Zhao, D. Zeng, S. Liang, H. Kang, and Q. Liu, “Prediction model for stock price trend based on recurrent neural network,” J. Ambient Intell. Humaniz. Comput., vol. 12, no. 1, pp. 745 753, 2021, doi: 10.1007/s12652 020 02057 0.
[22] S. Rashid, A. Ahmed, I. Al Barazanchi, and Z. A. Jaaz, “Clustering Algorithms Subjected to K Mean and Gaussian Mixture Model on Multidimensional Data Set,” Period. Eng. Nat. Sci., vol. 7, no. 2, pp. 448 457, 2019.
[24] A. K. Gupta, V. Singh, P. Mathur, and C. M. Travieso Gonzalez, “Prediction of COVID 19 pandemic measuring criteria using support vector machine, prophet and linear regression models in Indian scenario,” J. Interdiscip. Math., vol. 24, no. 1, pp. 89 108, 2021, doi: 10.1080/09720502.2020.1833458.
[3] H. Wei and Q. Zeng, “Research on sales Forecast based on XGBoost LSTM algorithm Model,” J. Phys. Conf. Ser., vol. 1754, no. 1, 2021, doi: 10.1088/1742 6596/1754/1/012191.
[25] Z. Malki et al., “ARIMA models for predicting the end of COVID 19 pandemic and the risk of second rebound,” Neural Comput. Appl., vol. 33, no. 7, pp. 2929 2948, 2021, doi: 10.1007/s00521 020 05434 0. [26] H. Alabdulrazzaq, M. N. Alenezi, Y. Rawajfih, B. A. Alghannam, A. A. Al Hassan, and F. S. Al Anzi, “On the accuracy of ARIMA based prediction of COVID 19 spread,” Results Phys., vol. 27, p. 104509, 2021, doi: 10.1016/j.rinp.2021.104509. [27] S. A. Fahad and M. M. Alam, “A modified K means algorithm for big data clustering,” Int. J. Sci. Eng. Comput. Technol., vol. 6, no. 4, pp. 129 132, Apr. 2016. [28] M. Faisal, E. M. Zamzami, and Sutarman, “Comparative Analysis of Inter Centroid K Means Performance using Euclidean Distance, Canberra Distance and Manhattan Distance,” J. Phys. Conf. Ser., vol. 1566, no. 1, 2020, doi: 10.1088/1742 6596/1566/1/012112. [29] M. Roux, “A Comparative Study of Divisive and Agglomerative Hierarchical Clustering Algorithms,” J. Classif., vol. 35, no. 2, pp. 345 366, 2018, doi: 10.1007/s00357 018 9259 9. [30] S. Kannan, “Intelligent object recognition in underwater images using evolutionary based Gaussian mixture model and shape matching,” Signal, Image Video Process., vol. 14, no. 5, pp. 877 885, 2020, doi: 10.1007/s11760 019 01619 w.
[5] S. Huang, Z. Kang, Z. Xu, and Q. Liu, “Robust deep k means: An effective and simple method for data clustering,” Pattern Recognit., vol. 117, p. 107996, 2021, doi: 10.1016/j.patcog.2021.107996.
[16] S. I. Nadeak and Y. Ali, “Analysis of Data Mining Associations on Drug Sales at Pharmacies with APRIORI Techniques,” IJISTECH (International J. Inf. Syst. Technol., vol. 5, no. 1, p. 38, 2021, doi: 10.30645/ijistech.v5i1.113.
[4] M. Iqbal, J. Ma, N. Ahmad, K. Hussain, and M. S. Usmani, “Promoting sustainable construction through energy efficient technologies: an analysis of promotional strategies using interpretive structural modeling,” Int. J. Environ. Sci. Technol., vol. 18, no. 11, pp. 3479 3502, 2021, doi: 10.1007/s13762 020 03082 4.
[17] P. Edastama, A. S. Bist, and A. Prambudi, “Implementation Of Data Mining On Glasses Sales Using The Apriori Algorithm,” Int. J. Cyber IT Serv. Manag., vol. 1, no. 2, pp. 159 172, 2021, doi: 10.34306/ijcitsm.v1i2.46.
[19] L. N. Rani, S. Defit, and L. J. Muhammad, “Determination of Student Subjects in Higher Education Using Hybrid Data Mining Method with the K Means Algorithm and FP Growth,” Int. J. Artif. Intell. Res., vol. 5, no. 1, pp. 91 101, 2021, doi: 10.29099/ijair.v5i1.223. [20] Y. Irawan, “Implementation Of Data Mining For Determining Majors Using K Means Algorithm In Students Of SMA Negeri 1 Pangkalan Kerinci,” J. Appl. Eng. Technol. Sci., vol. 1, no. 1, pp. 17 29, 2019, doi: 10.37385/jaets.v1i1.18.
[12] N. Talkhi, N. Akhavan Fatemi, Z. Ataei, and M. Jabbari Nooghabi, “Modeling and forecasting number of confirmed and death caused COVID 19 in IRAN: A comparison of time series forecasting methods,” Biomed. Signal Process. Control, vol. 66, no. February, p. 102494, 2021, doi: 10.1016/j.bspc.2021.102494.
REFERENCES
[21] X. Shen, Y. Zhang, K. Sata, and T. Shen, “Gaussian Mixture Model Clustering Based Knock Threshold Learning in Automotive Engines,” IEEE/ASME Trans. Mechatronics, vol. 25, no. 6, pp. 2981 2991, 2020, doi: 10.1109/TMECH.2020.3000732.
[23] W. Xu, H. Peng, X. Zeng, F. Zhou, X. Tian, and X. Peng, “A hybrid modelling method for time series forecasting based on a linear regression model and deep learning,” Appl. Intell., vol. 49, no. 8, pp. 3002 3015, 2019, doi: 10.1007/s10489 019 01426 3.
[31] Z. Wang, C. Da Cunha, M. Ritou, and B. Furet, “Comparison of K means and GMM methods for contextual clustering in HSM,” Procedia Manuf., vol. 28, pp. 154 159, 2019, doi: 10.1016/j.promfg.2018.12.025.
[32] A. Mirzal, “Statistical Analysis of Microarray Data Clustering using NMF, Spectral Clustering, Kmeans, and GMM,” IEEE/ACM Trans. Comput. Biol. Bioinforma., vol. 5963, no. c, pp. 1 1, 2020, doi: 10.1109/tcbb.2020.3025486.
[33] S. Srivastava, G. DePalma, and C. Liu, “An Asynchronous Distributed Expectation Maximization Algorithm for Massive Data: The DEM Algorithm,” J. Comput. Graph. Stat., vol. 28, no. 2, pp. 233 243, 2019, doi: 10.1080/10618600.2018.1497512.
[36] S. Noureen, S. Atique, V. Roy, and S. Bayne, “Analysis and application of seasonal ARIMA model in Energy Demand Forecasting: A case study of small scale agricultural load,” Midwest Symp. Circuits Syst., vol. 2019 Augus, pp. 521 524, 2019, doi: 10.1109/MWSCAS.2019.8885349.
[38] Ü. Ç. Büyükşahin and Ş. Ertekin, “Improving forecasting accuracy of time series data using a new ARIMA ANN hybrid method and empirical mode decomposition,” Neurocomputing, vol. 361, pp. 151 163, 2019, doi: 10.1016/j.neucom.2019.05.099.
BIOGRAPHIES OF AUTHORS Rani Puspita is a master’s student at BINUS Graduate Program Master of Computer Science, Bina Nusantara University with a focus on data science. Her undergraduate education background is Informatics Engineering at UIN Syarif Hidayatullah Jakarta. She is also a system analyst. She can be contacted at email: rani.puspita@binus.ac.id.
Lili Ayu Wulandhari is a lecturer at BINUS Graduate Program Master of Computer Science, Bina Nusantara University. She is also a data scientist. She can be contacted at email: lili.wulandhari@binus.ac.id.
ISSN: 2252 8938 Int J Artif Intell, Vol. 11, No. 3, September 2022: 1074 1084 1084 [34] A. A. Ningrum, I. Syarif, A. I. Gunawan, E. Satriyanto, and R. Muchtar, “Deep learning lstm algorithm for power transformer lifetime,” JTIIK, vol. 8, no. 3, pp. 593 548, 2021, doi: 10.25126/jtiik.202184587.
[37] A. Saikhu, C. V Hudiyanti, J. L. Buliali, and V. Hariadi, “Predicting COVID 19 Confirmed Case in Surabaya using Autoregressive Integrated Moving Average, Bivariate and Multivariate Transfer Function,” IOP Conf. Ser. Mater. Sci. Eng., vol. 1077, no. 1, p. 012055, 2021, doi: 10.1088/1757 899x/1077/1/012055.
[35] D. Li and J. Qian, “Text sentiment analysis based on long short term memory,” IEEE Int. Conf. Comput. Commun. Internet, 2016, doi: 10.1109/CCI.2016.7778967.
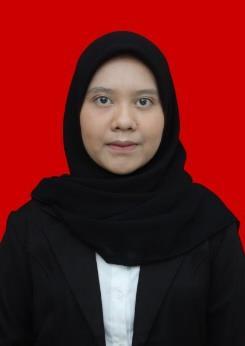



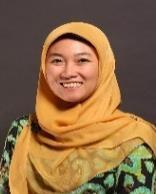



Article history: Received Jul 15, 2021 Revised Jun 2, 2022 Accepted Jun 20, 2022
Keywords: Artificial intelligence E TwitterSentimentalMachinecommercelearninganalysisdataset
Journal homepage: http://ijai.iaescore.com
An exponential growth posting on the web about the product reviews on social media, there has been a great deal of examination being done on sorting out the purchasing behaviors of the client. This paper depends on utilizing twitter for sentiment analysis to comprehend the customer purchasing behavior. There has been a significant increase in e commerce, particularly in persons purchasing products on the internet. As a result, it becomes a fertile hotspot for opinion analysis and belief mining. In this investigation, we look at the problem of recognizing and anticipating a client's purchase goal for an item. The sentiment analysis helps to arrive at a more indisputable outcome. In this study, the support vector machine, naive Bayes, and logistic regression methods are investigated for understanding the customer's sentiment or opinion on a specific product. These strategies have been demonstrated to be genuinely for making predictions using the analysis models which examine the client's conclusion/sentiment the most precisely. The exactness for each machine learning algorithm will be analyzed and the calculation which is the most precise would be viewed as ideal.
IAES International Journal of Artificial Intelligence (IJ AI) Vol. 11, No. 3, September 2022, pp. 1085 1093 ISSN: 2252 8938, DOI: 10.11591/ijai.v11.i3.pp1085 1093 1085
This is an open access article under the CC BY SA license. Corresponding Author: Vigneshwaran Pandi Department of Networking and Communications, School of Computing, College of Engineering and Technology, SRM Institute of Science and Technology SRM Nagar, Kattankulathur 603 203, Chengalpattu District, Chennai, India Email: vigenesp@srmist.edu.in
Social media has become one of the most important channels for communication and content generation. It fills in as a bound together stage for clients to communicate their contemplations on subjects going from their day by day lives to their sentiment on organizations and items. This, thus, has made it a significant asset for digging client feelings for errands going from anticipating the exhibition of films to aftereffects of stock market exchanges and races. Even though the vast majority is reluctant to answer reviews about items or administrations, they express their considerations unreservedly via online media and employ a huge impact in molding the assessments of different buyers. These customer voices can impact brand recognition, brand dedication and brand support. Therefore, it is basic that big companies give more consideration to mining client assessment identified with their brands and items from web based media. With web based media checking, they will have the option to take advantage of shopper bits of knowledge to
A comprehensive analysis of consumer decisions on Twitter dataset using machine learning algorithms
Vigneshwaran Pandi1, Prasath Nithiyanandam1, Sindhuja Manickavasagam2 , Islabudeen Mohamed Meerasha3, Ragaventhiran Jaganathan4 , Muthu Kumar Balasubramanian4 1Department of Networking and Communications, School of Computing, SRM Institute of Science and Technology, Kattankulathur, Chennai, India 2Department of Information Technology, Rajalakshmi Engineering College, Thandalam, Chennai, India 3Department of Computer Science and Engineering, School of Engineering, Presidency University, Bengaluru, India 4School of Computing and Information Technology, REVA University, Bengaluru, India Article Info ABSTRACT
1. INTRODUCTION
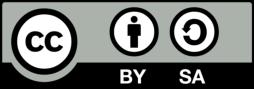
Sentiment analysis of user posts is required to help take business decisions. It is a cycle which extricates notions or suppositions from audits which are given by clients over a specific subject, zone, or item on the web. Estimation may be divided into two types: i) good or ii) negative that determines an individual's overall attitude toward a given subject. Predicting the sentiment of a tweet is our main priority. Purchasing objectives are often assessed and used by advertising executives as a contribution to decisions regarding new and current goods and administrations. Until date, many businesses have used client overview frameworks in which they offer questions such, "How likely are you to buy an item in a certain time span?" and then use that data to calculate the buy goal. We need to see whether we can use Twitter tweets to train a model that can differentiate tweets that indicate a purchase intention for a product.
ISSN: 2252 8938 Int J Artif Intell, Vol. 11, No. 3, September 2022: 1085 1093 1086improve their item quality, offer better assistance, drive deals, and even recognize new business openings. What is more, they can lessen client care costs by reacting to their clients through these web based media channels, as half of clients incline toward arriving at specialist organizations via online media as opposed to a call place.It is a phenomenal device for undertakings to dissect clients' communicated conclusions via online media without expressly posing any inquiries as this methodology frequently mirrors their actual sentiments.
2. RELATED WORKS Tan et al [1] proposed interpreting public sentiment variation to be able to further understand the reason behind the shift of public opinion on product or even people. In this case, they proposed using two models: one foreground and background latent dirichlet allocation (LDA) to filter out background topics that have no significance in the most recent public sentiment variation, and the other reason candidate and background LDA to rank the various reasons based on their "popularity" in the given period. It also employed Gibb's sampling since it was simple to expand and shown to be a successful approach. A sentiment analysis tool for slang word translation was also used, which could translate slangs into legitimate terms, which may be beneficial for more accuracy. They used data from the Stanford Network Analysis Platform. The suggested approach outperformed previous models in terms of accuracy and might be used for product evaluations, scientific publications, and many other applications; it is also the first effort to assess public sentiment changes. Xia et al. [2] developed dual sentiment analysis to solve the polarity shift problem in sentiment analysis, which affects the entire order but is otherwise treated the same in a typical model. So, in order to address the polarity shift, they offer dual training and dual prediction algorithms to assess both original and reversed data in order to comprehend not only how positive or negative the original data is, but also how positive or negative the reversed data is. They also expanded their polarity paradigm to a three class structure that includes neutral data. They created language independent pseudo antonym dictionaries to lessen their reliance on external antonym dictionaries. Support vector machine (SVM), naive Bayes, and logistic regression classifiers were used, and it was discovered that they exceed the baseline by 3.0 and 1.7% on average, respectively. Hamroun et al [3] advocated using latent semantics instead of current models that employ polarity terms and matching phrases and may fail when views are stated using latent semantics, which is known as customer intents analysis. They combined OpenNLP, W3C Web Ontology Language (OWL) ontologies, and WordNet natural language processing processes with additional meanings. Their strategy was to automatically extract patterns from Twitter for consumer intention research. The idea is to use domain ontology for two key purposes: creating ontology representations and using ontology representations in pattern learning. They utilized five distinct datasets, with the continuous integration (CI) pattern outperforming the baseline by 3 6% on average. Li et al [4] proposed combining two models: Sentiment specific word embeddings and Weighted text feature modal. Because the majority of conventional models are either lexicon based or machine learning based. Instead of immediately using the word embeddings approach, it will be done by first constructing vectors in order to avoid missing out on semantic hints and to enhance semantic categorization. weighted text feature model that generates two sort of features: the first is a negation feature based on negation terms, and the second is generated by computing the similarity of tweets and their polarity. The suggested strategy outperformed the previous model and separated sentiment specific word embeddings (SSWE) and (weighted text feature model (WTFM); moreover, when SSWE + word2vec was used, the
In spite of the fact that it has disadvantages with respect to the populace examined, it very well may be utilized to surmise general assessment. The objective of this exploration is to manufacture a framework that can give exact outcomes, helping brands to see how the clients are responding to the specific item. Nowadays interpersonal organizations, web journals, and other media produce an enormous measure of information on the Internet. This tremendous measure of information contains pivotal sentiment related data that can be utilized to profit organizations and different parts of business and logical ventures. Manual following and separating this valuable data from this monstrous measure of information is practically inconceivable.
Jose and Chooralil [12] evaluated and tried to address the problem with selecting just one algorithm for sentiment analysis, so they came up with the solution of combining machine learning algorithms along with lexicon based algorithms which would choose the appropriate algorithm for its use so as to remove the risk of selecting inappropriate classifiers. They chose SentiWordNet classifier, naive Bayes classifier, and Hidden Markov model classifier, which showed to be more accurate. So, after analyzing sentiment classification on numerous tweets, they concluded that their ensemble technique produced an accuracy of roughly 71.48%, which was higher than all three classifiers combined. Kouloumpis et al [13] recommended using Twitter hashtags to achieve even more accurate sentiment analysis since hashtags and emoticons may occasionally add significantly to model accuracy. In contrast to basic sentiment or non sentiment analysis, they would employ a three way classifier. To work on the datasets, they concentrated on n gram features, lexicon features, and part of speech features. They employed three datasets for development and training: hashtagged dataset from Edinburgh Twitter Corpus, emoticon dataset from twittersentiment.appspot.com, and iSieve company for assessment. After doing their investigation, they discovered that combining the n gram, lexicon, and microblogging features resulted in an accuracy of 74 75%. Park and Seo [14] used sentiment analysis to rank the three AI assistants, Siri from Apple, Cortana from Microsoft, and Google Assistant from Google, based on user feedback. They evaluated tweets using valence aware dictionary and
Chen et al [6] evaluated a very hard constraint project which only focused on engineering students' difficulties faced during their program. Naive Bayes and multi label classification algorithms were employed in the technique. The method used was a combination of qualitative analysis and large scale data mining approaches. It is a machine learning method that is also language dependent. It was founded on the notion that informal social media data might give additional information about students' experiences. Purdue University provided the tweets, which included subjects ranging from sleep deprivation to food. The dataset was taken from twitter API Tweepy. Bollegala et al [7] looked to address the mismatch problem arising in trained dataset and target dataset that is when the trained dataset has been for selected words and the test data does not contain those words, it creates a mismatch. In order to overcome this mismatch problem, they came up with a cross domain sentiment classifier where they used already extracted sentiment sensitive words and were able to determine that the existing models such as SentiWordNet, which is a lexical resource were outperformed by cross domain classifier. It also uses a lexical based approach and is a language dependent model aimed mainly at product reviews and the dataset was taken from amazon.com.
Lin et al [8] presented a joint sentiment analysis model as well as a reparametrized version of supervised joint sentiment topic because it was frequently observed that the weakly supervised joint sentiment topic, which is a component of LDA, failed to produce acceptable performance when shifting to new domains. As a result, our model can now recognize both sentiment and the subject of a certain data set. It is a machine learning method that is also language dependent. The dataset came from Amazon.com and IMDB.com and was based on product or movie reviews. Wang et al [9] proposed that for complete sentiment analysis of a tweet, we should also consider hashtags as complete words, and that three types of information are required to generate the complete sentiment polarity for hashtag, which differs from sentence and document level sentiment analysis. They also suggested using improved boosting classification, which would allow us to use the literal meaning of hashtags as a semi supervised training set. To construct the hashtag sentiment, they utilized an SVM classifier; it was a language dependent model for the Twitter dataset. Mudinas et al. [10] assessed both lexicon only and learning only approaches and presented a hybrid strategy that takes the best of both worlds from lexicon and learning only algorithms. When they ran the experiment, they discovered that the sentiment polarity classification and sentiment strength detection values in their pSenti system were higher, which is very near to the pure learning model and higher than the pure lexicon model. It was language specific and used both machine learning and lexical models. This model was created for software and movie reviews, including data from computer network (CNET) and internet movie database (IMDB). Yu et al [11] built their whole research around a movie domain case study and assessed the difficulty of forecasting sales using sentiment analysis. They investigated several hidden sentiment components in order to use sentiment Probabilistic Latent Sentiment Analysis (PLSA) to evaluate complicated forms of sentiment. They then suggested an updated version of the auto regressive sentiment aware model to boost accuracy. It was a language dependent, machine learning based model that focused on sales prediction in a movie based case study. The dataset was derived from the Twitter API, Tweepy, and was created exclusively for Twitter.
Int J Artif Intell ISSN: 2252 8938 A comprehensive analysis of consumer decisions on Twitter dataset … (Vigneshwaran Pandi) 1087 performance was extremely near to SSWE. Tweepy, a Twitter Application Program Interface (API), was utilized to generate the dataset. Ren and Wu [5] created a lexicon based learning method that is also language dependent to anticipate unknown user subject opinions. They attempted to include topical and social information into the current prediction model mathematically. They understood the association between social and topical context after applying an appropriate hypothesis and also utilized topic content similarity (TCS) to quantify the same. The findings revealed that the suggested ScTcMF framework was really superior to the existing one. The scope of the project was just for twitter and the dataset was also from twitter API.
ISSN: 2252 8938 Int J Artif Intell, Vol. 11, No. 3, September 2022: 1085 1093 1088sentiment reasoner (VADER), the Kruskal Wallis test, and the Mann Whitney test to determine statistical significance between groups. They employed null hypotheses and the t test to determine how the similarity of various aides varied over time. Prakruthi et al. [15] assess people's feelings towards a person, trend, product, or brand. The Twitter API is used to directly retrieve tweets from Twitter and construct sentiment classifications for the tweets. The data are categorized and represented using a Histogram and a Pie Chart. The pie chart depicts the%age of positive, negative, and neutral attitude, which is believed to be roughly 65% positive, 20% negative, and 15% neutral. The histograms below depict positive, negative, and neutral emotion. Go et al. [16] tested many models and performed trials to identify the best classifier for organizations who wish to analyze the sentiment of their products. Twitter tweets with emoticons serve as training data. Three classifiers were used: naive Bayes, maximum entropy, and SVM; all methods had an accuracy of more than 80% when trained using emoticon data. However, the SVM was the most accurate, with an accuracy of 85% Trupthi et al [17] want to do real time sentiment analysis on tweets retrieved from Twitter and present the results to the user. The tools and processes used here are natural language processing. Naïve Bayes and Twitter API. Natrual Language Processing (NLP) is used to remove the words with tags which is not helpful for the building of the classifier. The tweets removed by the Streaming API are then arranged into positive, negative, or unbiased tweets. The analytics for word nepotism from twitter is evident that Twitter verse feels mostly negative about nepotism. The results for the word education were mostly positive. Karthika et al [18] evaluated different models and the experiments were conducted to find the best classifier to analyze the reviews from shopping site amazon. Based on those reviews the product is classified as positive, negative, or neutral. Algorithms used here are random forest and SVMs. Random forest gave the best accuracy showing 84% while SVM showed 81% accuracy. Dataset contains reviews from 7 different products. Ramalingam et al [19] tested numerous models and performed trials to discover the best classifier for identifying similar qualities among depressed persons and identifying them using various machine learning methods. The algorithms are intended to examine tweets for emotion detection as well as the identification of suicide ideation among social media users. logistic regression, SVM, and Random Forest are the algorithms employed here. The goal of these strategies is to leverage data accessible on Twitter and other social media to forecast people's mindsets by studying their numerous social media posts. When compared to logistic regression and random forest, SVM has the highest accuracy of 82.5% Singh and Kumar [20] analyzed numerous models and conducted trials to determine the best method for predicting cardiac disease using various machine learning techniques. K nearest neighbor, decision tree, linear regression, and SVM are the approaches. Jupyter notebook is employed as the simulation tool in this case. The dataset contains 14 variables such as sex, age, blood sugar, and so on. We discovered that the accuracy of each algorithm was 87%, 79%, 78%, and 83%, respectively. As a result, k nearest neighbor (KNN) is the most precise. Sujath et al [21] tested many models and performed tests to determine the optimal method for analyzing the impact of COVID 19 on the stock market. Using several algorithms, we attempt to determine which method provides the best accurate prediction of the impact of COVID 19 on the stock market. The algorithms are random forest, linear regression, and SVM. The dataset was discovered on Kaggle. We discovered that SVM had the highest accuracy of 82% Mujumdar and Vaidehi [22] analyzed different models and experiments were conducted to find the best algorithm to predict diabetes among patients. The dataset contains 800 records and 10 attributes. Algorithms used here are decision tree, logistic regression and KNN. Logistic regression shows the most accuracy with 96% compared to the other two which shows only 90% and 86% accuracy. Huq et al. [23] examined many models and performed tests to determine the best algorithm to predict the sentiment of a tweet on social media, i.e., whether it is good, negative, or neutral. It generally focuses on the tweet's wording and sentiment. KNN and SVM are the algorithms applied in this case. The dataset was obtained from the website Kaggle. According to the research, KNN is the most accurate, with an accuracy rate of 84% Lassen et al. [24] examined many models and performed trials to determine the best algorithm to forecast iPhone sales based on tweets. The tweets are categorized as good, negative, or neutral. The dataset utilized here contains 400 million tweets from 2007 2010. Predictions are performed using linear regression and multiple regression models. Multiple regression has the smallest gap between anticipated and actual sales (5 10%), making it the most accurate. Dhir and Raj [25] examined many models and performed trials to determine the best algorithm for predicting movie performance. In this section, we analyze the internet movie database (IMDB) and estimate the IMDB score, as well as how it influences the movie collection. Logistic regression decision tree and random forest are the methods employed in this case. With 61% accuracy, random forest is the best. It demonstrates that social media likes, the number of voted users, and the length all have a significant impact on the IMDB score. Labib et al [26] used machine learning methodologies to examine multiple models and perform tests to discover the optimal algorithm to analyze traffic incidents to predict the intensity of accidents. The algorithms employed in this case include naive Bayes, decision trees,
KNN, and AdaBoost. It classifies the severity of incidents as deadly, serious, or minor harm. AdaBoost has the highest accuracy rate of 80%. It also revealed that accidents are more common at no joint exits and T intersections. Wongkar and Angdresey [27] created this model for the 2019 presidential election using Python and the naive Bayes, SVM, and K NN classifiers. Crawlers were employed to get tweets from Twitter, which were then tokenized to discover significant terms. They discovered that naive Bayes was more accurate, with an accuracy of 75 76%, after extensive study.
Gamon [28] proposed to perform sentiment analysis on even noisy data by the use of large feature vectors with feature reduction. As customer feedback are received at a very large volume, to be able to react to it quickly there has to be an efficient model to class the tweets into positive, negative, and neutral. They used NLPW in natural language processing for linguistic analysis. The accuracy at the end was 85.47%.
Int J Artif Intell ISSN: 2252 8938
3. COMPARISON ANALYSIS Table 1 (as seen in Appendix) shows the comparison of existing systems. To summarize all the existing works on sentiment analysis, we’ve gone through, we can divide it categorically into four types, which are document level, sentence level, phrase level, and aspect level. These existing papers tried to either tackle any one of the four types or even clubbed them, some tried to incorporate hashtags, some even tried to incorporate emoticons, some had language dependency, and some even had language independency. Some had greater accuracy but could tackle only one of the types, where some even had lesser accuracy but could incorporate a lot, some even tried building a complete corpus based antonym dictionary. Overall, we have a lot to dig in to use opinion mining to its fullest potential. What we will be doing in our model is, we will be taking the three best performing algorithms which are SVM, naive Bayes, and logistic regression to build a model which would allow enterprises to actually understand how well their products are performing, what shortcomings did customers feel, what could be better and many more. The proposed system will be much more efficient.
4. CONCLUSION AND FUTURE WORK
This article addresses a number of machines learning methods, including naive Bayes, SVM, logistic regression, and random forest. After extensive research, we discovered that SVM, naive Bayes, and logistic regression may be utilized to develop a model for our project that will provide a more accurate model than the present one, as demonstrated in the publications above. As we all know how analysis of twitter is being done to mine the opinions of users or customers in order to bring in potential customers or to enhance their products or services. Hence, it has become very important to constantly evolve and bring out even more accurate models. This work will help enterprises to draw out a basic idea on how the customers are reacting to the products which will then help them to make the product even better. This may help enterprises to leave behind the traditional methods of feedback forms which anyways is not very accurate. People now have the option to organize the unrelenting rise of knowledge from interpersonal organizations. Because virtually all actual complicated concerns ranging from natural to mechanical in nature may be addressed via social media, its challenges should be heard. Rumor detection, evaluation repetition, patterns of online conversations resulting in riotous circumstances, and online shaming, all shift assumptions, allowing us to understand social pervasiveness in the form of preferences, shares, and retweets. Finding the right content and the right time to publish are two of the most important difficulties that need be addressed in interpersonal organizations before fully integrating into people's life. Indeed, even the detection of fraudulent remarks should be attended to at the tiniest level of social places like Twitter to avoid unnecessary badgering from spammers. Medical issues of genuine concern should be addressed in additional study so that they have a strong impact via web based media clients. It would be appropriate at this point to prepare a tied up unified model that comprehends the assessments of the clientele when she/he is making remarks on social media.
A comprehensive analysis of consumer decisions on Twitter dataset … (Vigneshwaran Pandi) 1089
Kusrini and Mashuri [29] proposed two classifiers SVM and naive Bayes and compared both classifiers to understand which classifier gives the best result. It first takes the dataset, uses tokenization to segregate the words, removes various slangs and then uses stemming using python to reduce the volume of data. The accuracy at the end was around 82 83%. Mandloi and Patel [30] proposed using three different classifiers namely SVM, naive Bayes and maximum entropy classification to understand the user’s sentiment towards the following product, movie, and the people’s alignment towards the political parties. To extract the data, they used three features namely unigram, bi gram and n gram features and the accuracy came out to be 85% for naive Bayes.
Xia et al. [2] 2015 Dual Training and Dual Prediction along with corpus based dictionaryantonym Naive Bayes, SVM, logistic regression 85 87% Hamroun et al. [3] 2015 OPEN NLP, WordNet, OWL ontology CI patterns 72% Li et al. [4] 2016 LibLinear Model and RNDN N gram, SSWE, WTFM 66.8 Ren and Wu [5] 2013 The social and topical contexts Factorization of Matrixes (ScTcMF) Breadth first search, user topic labellingopinion 60.35 Chen et al. [6] 2014 Use informal social medial data to insightsprovide Naive Bayes, classificationmultilevel 61% Bollrgala et al. [7] 2013 SentiWordNet lexica classifier, corpus based Cross domain classificationsentiment 80% Lin et al. [8] 2012 To identify sentiment and topic from text at the same time Joint sentiment topic (JST) model with weak supervision based on latent Dirichlet allocation (LDA, Reverse JST). 71.20% Wang et al. [9] 2011 To automatically create the overall sentiment polarity for a specific hashtag during a specified time period, which significantlydiffersfrom the typical sentence level and document level sentiment polarities. SVM classifier 76% Mudinas et al. [10] 2012 To classify polarity and detectstrengthsentiment A hybrid strategy (lexicon based + M/c learning) was used. 77% Yu et al. [11] 2012 To Predict PerformanceSales Sentiment S PLSA (an SentimentAutoregressiveandQualityAwaremodel) 73%
ISSN: 2252 8938 Int J Artif Intell, Vol. 11, No. 3, September 2022: 1085 1093 1090
Name of the authors Year of publication Methodology Algorithms used Accuracy Tan et al. [1] 2014 Foreground and Background LDA, Reason Candidate and Background LDA Gibbs AverageParametersampling,estimation,wordentropy 69.70%
Jose and Chooralil [12] 2016 Three way classifier unlike simple sentiment or nonanalysissentiment n gram feature, lexicon feature and part of speech feature 75% Kouloumpis et al. [13] 2011 Three way classifier unlike simple sentiment or nonanalysis.sentiment n gram feature, lexicon feature and part of speech feature 75% Park and Seo [14] 2018 Three AI assistants namely Siri by Apple, Cortana by Microsoft and Google Assistant by Google using analysissentiment VADER, Kruskal Wallis test and Mann Whitney test 71% Prakruthi et al. [15] 2018 Sentiment classification for the tweets using Histogram and Pie Chart. Bag of Words algorithm 68% Go et al. [16] 2009 Unigrams, Bi grams, and parts of speech to use emoticons Naive Bayes, SVM 81% Trupthi et al. [17] 2017 Natural ProcessingLanguageNLTK Naive classificationBayes 74%
APPENDIX
Table 1 Performance analysis comparison of existing systems (continue)
[6] X. Chen, M. Vorvoreanu, and K. P. C. Madhavan, “Mining social media data for understanding students’ learning experiences,” IEEE Trans. Learn. Technol., vol. 7, no. 3, pp. 246 259, Jul. 2014, doi: 10.1109/TLT.2013.2296520. [7] D. Bollegala, D. Weir, and J. Carroll, “Cross domain sentiment classification using a sentiment sensitive thesaurus,” IEEE Trans. Knowl. Data Eng., vol. 25, no. 8, pp. 1719 1731, Aug. 2013, doi: 10.1109/TKDE.2012.103.
Logistic regression, SVM, and random forest 82.50% Singh and Kumar [20] 2020 Machine algorithms'learningaccuracy in predicting heart disease k nearest neighbor, decision tree, linear regression, and support vector machine 87% Sujath et al. [21] 2020 forecasting model for COVID 19 pandemic decision tree, logistic regression and KNN. 96% Mujumdar and Vaidehi [22] 2019 best algorithm to predict diabetes among patients. decision tree, logistic regression and KNN 96% Huq et al. [23] 2017 To predict the sentiment of a tweet on social media KNN and classifiersSVM 84% Lassen et al. [24] 2014 predict iPhone sales using tweets based on iPhone linear regression and multiple regression models 70% Dhir and Raj [25] 2018 movie predictionsuccess logistic randomdecisionregressiontreeandforest 61% Labib et al. [26] 2019 determine the intensity of accidents naïve bayes, decision trees, KNN and AdaBoost 80% Wongkar Angdreseyand[27] 2019 Data collection utilizing Python libraries, text processing, testing training data, and text categorization Naive Bayes classifier, SVM classifier and K NN classifier 76% Gamon [28] 2004 Train linear SVMs to obtain classificationhigh accuracy on difficult to classify data. NLPW in natural language processing for linguistic analysis. 85% Kusrini and Mashuri [29] 2019 Lexicon Based and Polarity Multiplication SVM, naive Bayes 83% Mandloi and Patel [30] 2020 Three features namely unigram, bi gram and n gram features SVM, naive Bayes and Maximum Entropy 85% REFERENCES
[1] S. Tan et al., “Interpreting the public sentiment variations on Twitter,” IEEE Trans. Knowl. Data Eng., vol. 26, no. 5, pp. 1158 1170, May 2014, doi: 10.1109/TKDE.2013.116.
Int J Artif Intell ISSN: 2252 8938 A comprehensive analysis of consumer decisions on Twitter dataset … (Vigneshwaran Pandi) 1091
Table 1 Performance analysis comparison of existing systems
[5] F. Ren and Y. Wu, “Predicting user topic opinions in Twitter with social and topical context,” IEEE Trans. Affect. Comput., vol. 4, no. 4, pp. 412 424, Oct. 2013, doi: 10.1109/T AFFC.2013.22.
[8] C. Lin, Y. He, R. Everson, and S. Ruger, “Weakly supervised joint sentiment topic detection from text,” IEEE Trans. Knowl. Data Eng., vol. 24, no. 6, pp. 1134 1145, Jun. 2012, doi: 10.1109/TKDE.2011.48.
[2] R. Xia, F. Xu, C. Zong, Q. Li, Y. Qi, and T. Li, “Dual sentiment analysis: considering two sides of one review,” IEEE Trans. Knowl. Data Eng., vol. 27, no. 8, pp. 2120 2133, Aug. 2015, doi: 10.1109/TKDE.2015.2407371.
[4] Q. Li, S. Shah, R. Fang, A. Nourbakhsh, and X. Liu, “Tweet sentiment analysis by incorporating sentiment specific word embedding and weighted text features,” in 2016 IEEE/WIC/ACM International Conference on Web Intelligence (WI), Oct. 2016, pp. 568 571., doi: 10.1109/WI.2016.0097.
[10] A. Mudinas, D. Zhang, and M. Levene, “Combining lexicon and learning based approaches for concept level sentiment analysis,” in Proceedings of the First International Workshop on Issues of Sentiment Discovery and Opinion Mining, 2012, pp. 1 8., doi: 10.1145/2346676.2346681.
[9] X. Wang, F. Wei, X. Liu, M. Zhou, and M. Zhang, “Topic sentiment analysis in twitter: a graph based hashtag sentiment classification approach,” in Proceedings International Conference on Information and Knowledge Management, 2011, pp. 1031 1040., doi: 10.1145/2063576.2063726.
Name of the authors Year of publication Methodology Algorithms used Accuracy Karthika et al. [18] 2019 Receiver classifiercurveCharacteristicOperating(ROC)toevaluateoutput Random algorithm,forestSVM 84% Ramalingam et al. [19] 2019 Machine learning and lexicon assessmentmining,techniquesbasedtoopinionaswellasmetrics
[3] M. Hamroun, M. S. Gouider, and L. Ben Said, “Lexico semantic patterns for customer intentions analysis of microblogging,” in 2015 11th International Conference on Semantics, Knowledge and Grids (SKG), Aug. 2015, pp. 222 226., doi: 10.1109/SKG.2015.40.
[23] M. R. Huq, A. Ali, and A. Rahman, “Sentiment analysis on Twitter data using KNN and SVM,” Int. J. Adv. Comput. Sci. Appl., vol. 8, no. 6, 2017, doi: 10.14569/ijacsa.2017.080603.
[29] Kusrini and M. Mashuri, “Sentiment analysis in Twitter using lexicon based and polarity multiplication,” in 2019 International Conference of Artificial Intelligence and Information Technology (ICAIIT), Mar. 2019, pp. 365 368., doi: 10.1109/ICAIIT.2019.8834477.
[16] A. Go, R. Bhayani, and L. Huang, “Twitter sentiment classification using distant supervision,” 2009.
[27] M. Wongkar and A. Angdresey, “Sentiment analysis using naive bayes algorithm of the data crawler: Twitter,” in 2019 Fourth International Conference on Informatics and Computing (ICIC), Oct. 2019, pp. 1 5., doi: 10.1109/ICIC47613.2019.8985884.
[18] P. Karthika, R. Murugeswari, and R. Manoranjithem, “Sentiment analysis of social media network using random forest algorithm,” in 2019 IEEE International Conference on Intelligent Techniques in Control, Optimization and Signal Processing (INCOS), Apr. 2019, pp. 1 5., doi: 10.1109/INCOS45849.2019.8951367.
[28] M. Gamon, “Sentiment classification on customer feedback data,” 2004., doi: 10.3115/1220355.1220476.
[22] A. Mujumdar and V. Vaidehi, “Diabetes prediction using machine learning algorithms,” Procedia Comput. Sci., vol. 165, pp. 292 299, 2019, doi: 10.1016/j.procs.2020.01.047.
[17] M. Trupthi, S. Pabboju, and G. Narasimha, “Sentiment analysis on Twitter using streaming API,” in 2017 IEEE 7th International Advance Computing Conference (IACC), Jan. 2017, pp. 915 919., doi: 10.1109/IACC.2017.0186.
[12] R. Jose and V. S. Chooralil, “Prediction of election result by enhanced sentiment analysis on twitter data using classifier ensemble approach,” in 2016 International Conference on Data Mining and Advanced Computing (SAPIENCE), Mar. 2016, pp. 64 67., doi: 10.1109/SAPIENCE.2016.7684133.
[11] X. Yu, Y. Liu, X. Huang, and A. An, “Mining online reviews for predicting sales performance: a case study in the movie domain,” IEEE Trans. Knowl. Data Eng., vol. 24, no. 4, pp. 720 734, Apr. 2012, doi: 10.1109/TKDE.2010.269.
[20] A. Singh and R. Kumar, “Heart disease prediction using machine learning algorithms,” in 2020 International Conference on Electrical and Electronics Engineering (ICE3), Feb. 2020, pp. 452 457., doi: 10.1109/ICE348803.2020.9122958.
[30] L. Mandloi and R. Patel, “Twitter sentiments analysis using machine learninig methods,” in 2020 International Conference for Emerging Technology (INCET), Jun. 2020, pp. 1 5., doi: 10.1109/INCET49848.2020.9154183.
ISSN: 2252 8938 Int J Artif Intell, Vol. 11, No. 3, September 2022: 1085 1093 1092
[26] M. F. Labib, A. S. Rifat, M. M. Hossain, A. K. Das, and F. Nawrine, “Road accident analysis and prediction of accident severity by using machine learning in Bangladesh,” in 2019 7th International Conference on Smart Computing & Communications (ICSCC), Jun. 2019, pp. 1 5., doi: 10.1109/ICSCC.2019.8843640.
[13] E. Kouloumpis, T. Wilson, and J. Moore, “Twitter sentiment analysis: the good the bad and the OMG!,” Proc. Fifth Int. AAAI Conf. Weblogs Soc. Media, vol. 5, no. 1, pp. 538 541, 2011 [14] C. W. Park and D. R. Seo, “Sentiment analysis of Twitter corpus related to artificial intelligence assistants,” in 2018 5th International Conference on Industrial Engineering and Applications (ICIEA), Apr. 2018, pp. 495 498., doi: 10.1109/IEA.2018.8387151.
[25] R. Dhir and A. Raj, “Movie success prediction using machine learning algorithms and their comparison,” in 2018 First International Conference on Secure Cyber Computing and Communication (ICSCCC), Dec. 2018, pp. 385 390., doi: 10.1109/ICSCCC.2018.8703320.
[24] N. B. Lassen, R. Madsen, and R. Vatrapu, “Predicting iPhone sales from iPhone tweets,” in 2014 IEEE 18th International Enterprise Distributed Object Computing Conference, Sep. 2014, pp. 81 90., doi: 10.1109/EDOC.2014.20.
[19] D. Ramalingam, V. Sharma, and P. Zar, “Standard multiple regression analysis model for cell survival/ death decision of JNK protein using HT 29 carcinoma cells,” Int. J. Innov. Technol. Explor. Eng., vol. 8, no. 10, pp. 187 197, Aug. 2019, doi: 10.35940/ijitee.H7163.0881019.
BIOGRAPHIES OF AUTHORS Vigneshwaran Pandi has obtained his Doctoral Degree in Anna University Chennai in 2016 and Master of Engineering under Anna University Chennai in June 2005. He is having 20 years of experience and specialization in Cybersecurity. Presently, He is working as Associate Professor at the SRM Institute of Science and Technology, Chennai. He has published more than 30 papers in various international journals and 10 in International Conferences. His area of interest includes Security, Routing, and Intelligent Data Analysis. He can be contacted at email: vigenesp@srmist.edu.in Prasath Nithiyanandam has obtained his Doctoral Degree from Anna University, Chennai in 2017 and Master of Technology from SASTRA University in 2009 and Undergraduate degree under Anna University Chennai in 2006. He is having 13+ years of experience in teaching and Industry. He has published 4 patents and published more than 15 research papers in refereed conferences and in journals. He is a member many professional societies. His research interests include MANET, Sensor Networks, IoT and Cloud, Cyber Physical System and Machine Learning. He can be contacted at email: prasathn@srmist.edu.in, prasath283@gmail.com
[21] R. Sujath, J. M. Chatterjee, and A. E. Hassanien, “A machine learning forecasting model for COVID 19 pandemic in India,” Stoch. Environ. Res. Risk Assess., vol. 34, no. 7, pp. 959 972, Jul. 2020, doi: 10.1007/s00477 020 01827 8.
[15] V. Prakruthi, D. Sindhu, and D. S. Anupama Kumar, “Real time sentiment analysis of Twitter posts,” in 2018 3rd International Conference on Computational Systems and Information Technology for Sustainable Solutions (CSITSS), Dec. 2018, pp. 29 34., doi: 10.1109/CSITSS.2018.8768774.
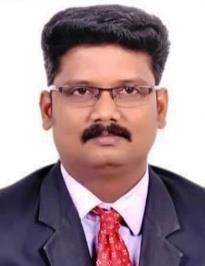



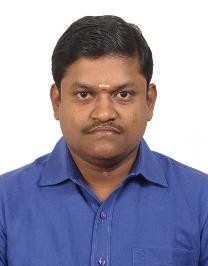



Int J Artif Intell ISSN: 2252 8938
Dr. Islabudeen Mohamed Meerasha received his B.E. Degree in Computer Science and Engineering from Madurai Kamaraj University, Madurai, India in 2001 and M.E. Degree in Computer Science and Engineering and Ph.D. Degree in Information and Communication Engineering from Anna University, Chennai, India in 2008 and 2021, respectively. He is currently working as an Associate Professor in Department of Computer Science Engineering, School of Engineering, Presidency University, Bengaluru, India. He is having more than 20 years of academic experience. His current research interests include Cryptography and Network Security, Blockchain, Wireless Networks and Data Mining. He is a life member of Computer Society of India (CSI) and Indian Society for Technical Education (ISTE). He has served as an active reviewer and chair for many reputed international conferences and journals including IEEE and Springer. He has more than 20 publications in reputed international conferences and refereed journals. He can be contacted at email: islabudeen@gmail.com
A comprehensive analysis of consumer decisions on Twitter dataset … (Vigneshwaran Pandi
Mrs. Sindhuja Manickavasagam M.Tech., Ph.D Assistant Professor (Senior Grade) at Department of Information Technology, Rajalakshmi Engineering College from August 2006 onwards. She is having more than 15 years of experience in teaching. Presently she is acting as Assistant Professor (Senior Grade). She is pursuing Doctoral Program under Anna University in the field of Data Analytics. Her research interest includes artificial intelligence, machine learning, deep learning, bigdata analytics, and bioinformatics. She has published 14 international Journals including 3 from SCI and Scopus Indexed Journals. She has published one patent titled “Arm Band for Blood Testing” under the category of Design patent. She has visited Japan and Malaysia for presenting her research work in reputed International Conferences. Presently, she is working in the project of Cancer Analysis. She is a Certified Talend DI developer by Virtusa, IBM certified DB2 and Tivoli developer. She acted as resource person for various workshops held in other Engineering Colleges and University on her research topics. She can be contacted at email: sindhuja.m@rajalakshmi.edu.in
) 1093
Dr. Ragaventhiran Jaganathan received his B.E. and M.E. degree in Computer Science and Engineering and Ph.D. in Information and Communication Engineering from Madurai Kamaraj University and Anna University, Tamil Nadu, India respectively. He is currently working as an Associate Professor in School of Computing and Information Technology in REVA University, Bengaluru India. His research interest includes data mining, big data, data structure and cloud computing. He is a life member in computer society of India (CSI) and Institution of Engineers (IEI) India. He has reviewed and chaired various national and international conferences including IEEE conferences. He is also a reviewer for refereed journals. He can be contacted at email: jragaventhiran@gmail.com
Dr. Muthu Kumar Balasubramania Professor, School of Computing and Information Technology, REVA University, Bengaluru received his B.E., (CSE) degree from Anna University, Chennai in the year 2005, M.Tech., (CSE) (Gold Medalist) received from Dr. MGR. University, Chennai in the year 2007 and Doctoral degree from St. Peter’s University, Chennai in the year 2013. He is having more than 16 years of teaching experience in reputed engineering colleges. He has published more than 40 peer reviewed International Journals, 50 International/National Conference and attended more than 150 Workshops/FDPs/Seminars etc., He organized many events like Conference/FDPs/Workshops/Seminars/Guest Lecture. He has published more than 10 patents in various fields like Wireless Sensor Networking, Image Processing, Optimization Techniques and IoT. He received nearly 5.67 Lakhs funding from various agencies like AICTE, ATAL and IEI. He has written 2 books from reputed publishers. He received Best Researcher Award in the year 2021 and Innovative Research and Dedicated Professor Award in Computer Science and Engineering in the year 2018. He has professional membership on ISTE, CSI, IEI, IACSIT, IAENG, CSTA, and SIAM. He has invited as guest lecture, chairperson, examiner, and reviewer/editorial board member in various institutions, journals, and conferences. He is a recognized supervisor in Anna University, Chennai and currently guiding 4 research scholars. His areas of interest are image processing, wireless networks, IOT and computing techniques. He can be contacted at email: muthu122@gmail.com.
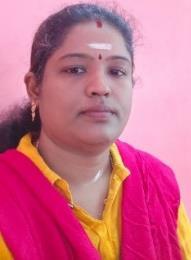



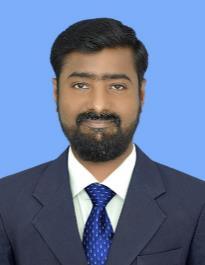



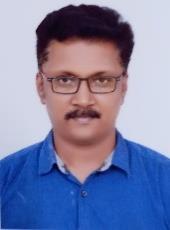



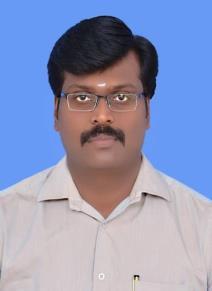



Department of Digital Forensic and Cyber Security, Faculty of Informatics, Telkom University, Bandung, Indonesia
Semi-supervised approach for detecting distributed denial of service in SD-honeypot network environment
Keywords: Cyber Distributedsecuritydenial of service SHoneypotoftwaredefined network Semi supervised This is an open access article under the CC BY SA license. Corresponding Author: Fauzi Dwi Setiawan Sumadi Department of Informatics, Faculty of Engineering, Universitas Muhammadiyah Malang 246 Raya Tlogomas Street, Malang 65144, East Java, Indonesia Email: fauzisumadi@umm.ac.id
Article history: Received Sep 20, 2021 Revised May 22, 2022 Accepted Jun 20, 2022 Distributed Denial of Service (DDoS) attacks is the most common type of cyber attack. Therefore, an appropriate mechanism is needed to overcome those problems. This paper proposed an integration method between the honeypot sensor and software defined network (SDN) (SD honeypot network). In terms of the attack detection process, the honeypot server utilized the Semi supervised learning method in the attack classification process by combining the Pseudo labelling model (support vector machine (SVM) algorithm) and the subsequent classification with the Adaptive Boosting method. The dataset used in this paper is monitoring data taken by the Suricata sensor. The research experiment was conducted by examining several variables, namely the accuracy, precision, and recall pointed at 99%, 66%, and 66%, respectively. The central processing unit (CPU) usage during classification was relatively small, which was around 14%. The average time of flow rule mitigation installation was 40s. In addition, the packet/prediction loss occurred during the attack, which caused several packets in the attack not to be classified was pointed at 43%.
Journal homepage: http://ijai.iaescore.com
1Department of Informatics, Faculty of Engineering, Universitas Muhammadiyah Malang, Malang, Indonesia 2
Fauzi Dwi Setiawan Sumadi1, Christian Sri Kusuma Aditya1 , Ahmad Akbar Maulana1, Syaifuddin1 , Vera Suryani2
1. INTRODUCTION
The development in the networking technology area introduced significant enhancement on the management module. Generally, the traditional network performed both network management and forwarding mechanism into a single layer of abstraction. The main problem that originated from the traditional network implementation is scalability. The network tended to be complex along with the device’s extension. Therefore, several researchers generated programmable architecture called the software defined network (SDN). The primary concept was the separation of networking control and the forwarding function into two independent layers [1]. The communication protocol between the two mentioned layers is maintained by the Southbound Application Programming Interface (API) e.g. and OpenFlow. OpenFlow specifies the rules for managing the forwarding devices to perform particular actions e.g., forward, drop, meter, modify, or even crafting new packet, based on the generation of flow rule from OpenFlow [2] flow table modifications (OFPT_FLOW_MOD) message. However, the deployment of centralized logic control in SDN is vulnerable to a single point of failure affected by various types of cyber attack e.g. and distributed denial of service (DDoS) [3]. In terms of the solution for avoiding the controller’s malfunctioning due to cyber attack, honeypot [4] may have a significant role to monitor the attack. It behaves as a trap for the attackers to perform miscellaneous actions by deliberately opening several ports/services that usually became
IAES International Journal of Artificial Intelligence (IJ AI) Vol. 11, No. 3, September 2022, pp. 1094~1100 ISSN: 2252 8938, DOI: 10.11591/ijai.v11.i3.pp1094 1100 1094
Article Info ABSTRACT
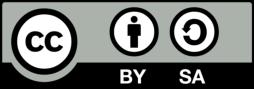
Int J Artif Intell ISSN: 2252 8938 Semi supervised approach for detecting distributed denial of service in … (Fauzi Dwi Setiawan Sumadi) 1095
The experiment was implemented using a real hardware environment depicted in Figure 1. There was one controller (C1) using Ryu [22], three SDN enabled routers (R1, R2, and R3) using Mikrotik [23] which supported OpenFlow version 1.1, and four hosts (H1 H4) using Ubuntu OS. H1 and H3 were pointed as normal hosts for communicating using normal ICMP packets. The attacker resided in H2 where the flooding type was an ICMP flood attack. The transmitted packets consisted of randomly generated medium access control (MAC) and internet protocol (IP) addresses using Scapy [24]. The attack’s flow was at a rate of 100; 200; 500; 1,000; 2,000 packets per second which were transferred using Tcpreplay [25]. H4 was installed by the modern honey network (MHN) server integrated with the Suricata sensor for detecting ICMP flood.
The integration of SDN and honeypot provides a comprehensive attack representation that aims to overwhelm the architecture. SD honeypot network may become a single system for developing both IDS and intrusion prevention system (IPS).
the main target e.g., secure shell (SSH), server message block (SMB), internet control message protocol (ICMP). Several types of honeypot sensors are specialized to attract specific types of attacks [4] e.g., Dionea can log and capture malware activity that uses several types of protocols such as hypertext transfer protocol (HTTP), file transfer protocol (FTP), voice over internet protocol (VOIP), and the other protocols [5], Cowrie focuses to monitor and store malicious activity regarding the brute force attacks performed under the Telnet or SSH [6], Suricata is directed to create an intrusion detection system (IDS) for complex circumstances [7].
Previously, several researchers have already investigated the capability of DDoS for overloading traditional networks. The former concept for detecting DDoS was categorized into two approaches namely the statistic [8], [9] and artificial intelligence (AI) [10] [14]. Several statistics approaches have been deployed e.g., the Entropy [8] which calculated the data randomness and specified the DDoS threshold by its value, Bloom filter [9] which focused the detection phase by comparing the hash value of the incoming packet to assure the packet was not considered as SYN flood attack. The statistical approaches are predominantly constant at measuring the pattern, if the attackers alter the flooding scheme, these methods may not identify the attacks. Therefore, several papers also introduced AI techniques for detecting DDoS. Maslan et al. [10] in implemented the feature selection combined with several classification algorithms to detect DDoS using their dataset. The researchers selected 4 from the whole 25 features extracted using CICFlowMeter V3 and concluded that the most effective algorithm is Random Forest Similarly, Fadlil et al. [11] used their dataset by capturing the attack on simulation using low orbit ion cannon (LOIC). The results stated the Naïve Bayes algorithm could predict the outcomes precisely even though there was no apparent result for the classification metric. Several papers also conducted the classification based on available datasets [12] [14] (NSL KDD, UNB ISCX 12, and UNSW NB15). Idhammad et al. [12] proposed the Semi supervised learning for classifying the DDoS attack gained 98.23% for accuracy. Mohammed et al. [13] and Muhammad et al. [14] utilized deep learning (DL) for detecting DDoS and achieved an accuracy at 97.82% and 99.60% respectively. The programmability feature in SDN may provide manageable structure for implementing AI to detect DDoS. Several papers provided analysis by maintaining the dataset based on the OpenFlow extraction process or existing dataset. Sumadi et al. [15] compared several machine learning (ML) algorithms using datasets generated from the port statistic message combined with the default features for packet extraction information. The results stated that SVM could create the best outcomes in terms of accuracy (100%). Dey and Rahman [16] used network security laboratory KDD (NLS KDD) dataset as the primary dataset for detecting DDoS using both ML and DL gained 88% in accuracy for the result of the gated recurrent unit long short term memory (GRU LSTM) model. The other possible technique for resolving DDoS/Cyber attack is integrating the honeypot in the SDN environment [17] [21]. Wang and Wu [17] proposed a customized topology by combining existed SDN architecture, high level and low level honeypot’s topology. The attacks were redirected to the honeypots topology based on their level. The rest of the mentioned papers [18] [21] presented the deployment of honeypot for migrating the cyber attacks in software defined internet of things (SD IoT) network. Similarly, the authors were directed their research for only monitoring the attack and did not perform further analysis. The former papers concluded that honeypot was appropriate as a tool for attracting, capturing, and monitoring cyber attacks. There was still no paper that aimed to perform in depth processes for analyzing the data captured from the honeypot sensor in SDN. Therefore, this paper is focused to investigate the possibility of Semi supervised learning to detect the ICMP flood attack in the SD honeypot network environment. The main contribution of this paper is constructing an IDS and IPS system which proposes the SD honeypot for resolving DDoS attacks, applying the semi supervised method for classifying the captured data from the Suricata sensor, and mitigating the attack using representational state transfer application programming interface (REST API). The effectiveness of the proposed method is measured using standard classification metrics, resource usage, and the time value for installing the mitigation rule.
2. RESEARCH METHOD
The detailed information of system workflow is described in Figure 2. The received packet was inspected by Mikrotik based on the available Flow Rules. If there was no flow filtering the incoming packet the switch generated Packet In Message (OFPT_PACKET_IN) encapsulating the packet. However, if the packets were intended to attack the vulnerabilities of the Suricata sensor, the switch automatically sent the packet to H4. Subsequently, the H4 stored the packet’s information on the MongoDB database. Based on the proposed scenario, the application installed in H4 collected the data from MongoDB within a range of 10, 20, 30, 40, 50, 60, 70, 80, 90, 100 seconds. The extracted packets were pointed as the data test for the Pseudo labelling and Semi supervised approaches. If there were no packets categorized as DDoS packets, the application notified as normal circumstances. In contrast, the application transmitted the Flow Modification Message (OFPT_FLOW_MOD) encapsulated in JavaScript Object Notation (JSON) format for commanding the controller to generate mitigation flow to all of the available switches through REST API. The flow mitigation had consisted of a flow match structure for filtering the attack based on the protocol’s type and flow action for dropping the packet (no available action needed to be specified based on the OpenFlow protocol).
The classification process included the Pseudo labelling and Supervised Learning method described in Figure 3. The Pseudo labelling was performed by the support vector machine (SVM) algorithm using a Linear Kernel. The dataset used during the experiment contained 27,000 labeled data trains from 70,000 data in total. The installed application extracted the live data test from the MongoDB database which was utilized by the MHN to gather the stored attack data from the honeypot sensors (Suricata). The extraction process was experimented within several ranges of times (10 90 s). The application divided the extracted data into two components of an unlabeled dataset. The first fraction of data was being classified using the labeled data
ISSN: 2252 8938 Int J Artif Intell, Vol. 11, No. 3, September 2022: 1094 1100 1096
Figure 1. Simulation’s topology Figure 2. MHN server’s block diagram
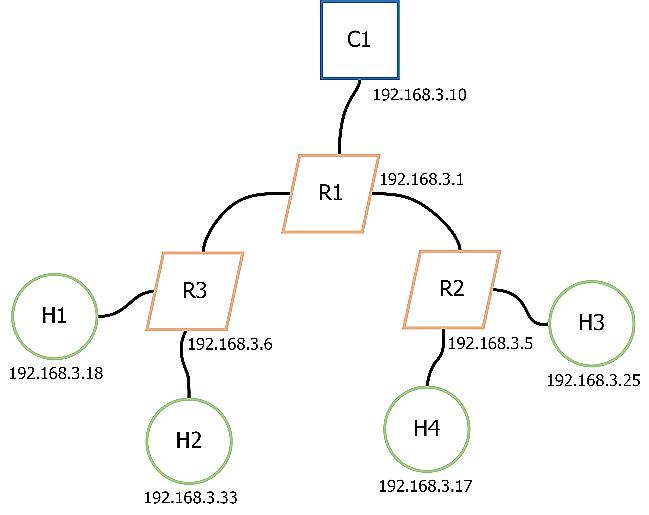
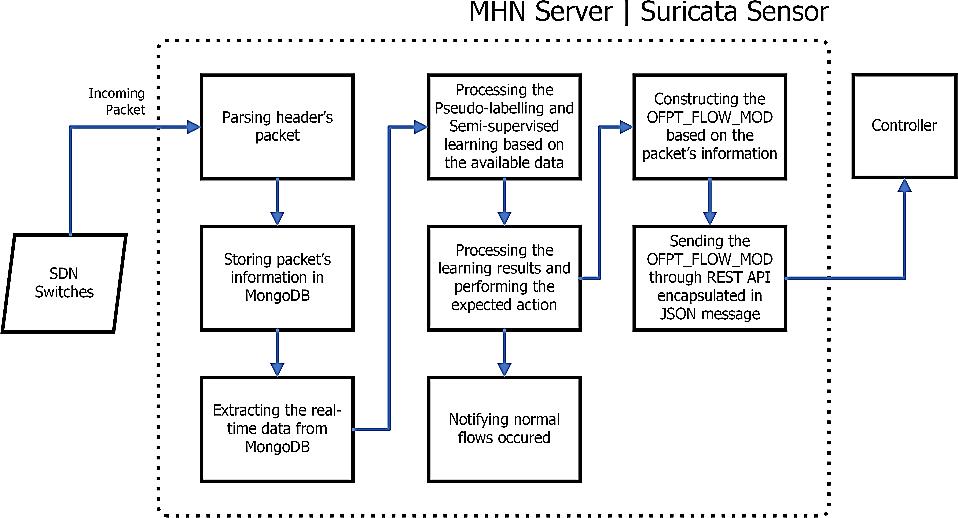
Int J Artif Intell ISSN: 2252 8938 Semi supervised approach for detecting distributed denial of service in … (Fauzi Dwi Setiawan Sumadi) 1097 train. Then the data were integrated which produced the combination of labeled train data and the fraction of classifiedThedata.combined data was pointed as the training set for the Supervised Learning model using Adaptive Boosting algorithm with the number of estimators at ten. The classification process using the Supervised model was performed on the second fraction of the live dataset. The results of the classification were evaluated using standard variables including the accuracy, precision, recall, F1 score, the packet loss during the classification, the central processing unit (CPU) usage of application during the whole process, and the time for the mitigation flow to be installed on the SDN switch/mikrotik.
The sample of the data train and live dataset during the experiment is illustrated in Table 1. It has seven features and one label consisting of two categories, DDoS, and normal packet. The features were the default data provided by the MHN
Figureserver.3.Semi
supervised learning implementation Table 1. The sample of the dataset used during the experiment Protocol Hpfeed_id Timestamp Source_ip Destination_ip Identifier Honeypot Type ICMP ObjectId(5e4fad27f81c700cab511e8d) 2020 21T10:12:55.066Z02 154.25.125.196 192.168.3.25 :: suricata DDOS ICMP ObjectId(5e280d913186f205962bef16) 2020 22T08:53:37.924Z01 192.168.3.33 192.168.3.25 suricata NORMAL ICMP ObjectId(5e4cf03d3186f205953a64c0) 2020 19T08:22:21.679Z02 192.168.3.17 192.168.3.25 suricata NORMAL ICMP ObjectId(5e53f1871d41c80851461452) 2020 24T15:53:43.407Z02 228.156.186.177 192.168.3.25 suricata DDOS
The research results were extracted from several scenarios by comparing the fluctuated rate of the database’s extraction interval and the packet’s sending rate. The MongoDB data acquisition process was delayed after several time intervals within the range of 10, 30, 50, 70, and 90 s. The attacker also maintained the sending rate using Tcpreplay at ranges of 100; 500; 1,000; and 2,000 packets/s. Based on the results provided by Table 2, the average accuracy was pointed at 99% and 66% for the precision, recall, and F1 score. Although the accuracy produced a high value, the precision still pointed at low indicating that the generated model could predict the result and was almost precise. Moreover, the growth of the packet’s
3. RESULTS AND DISCUSSION
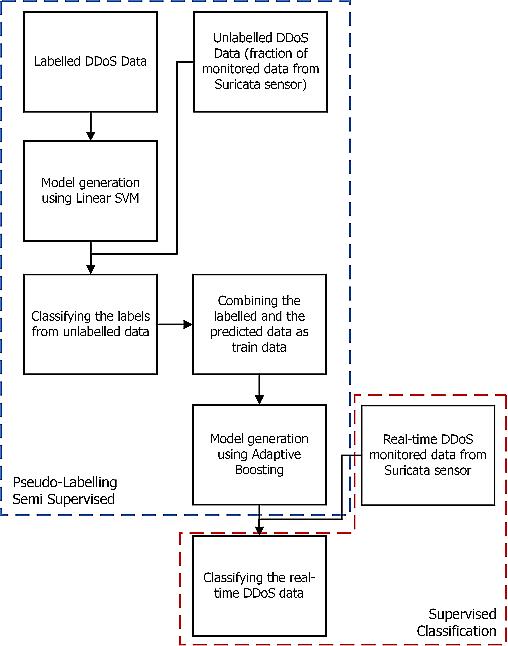
The application installed in the MHN server also performed a mitigation scheme by commanding the controller to send OFPT_FLOW_MOD. The mechanism could be implemented by deploying REST API HTTP POST request provided by Ryu. The time needed to install the mitigation flow was extracted, as shown in Table 4 for measuring the effectiveness of the mitigation approach. The average time for installing the mitigation flow increased along with the growth of packet sending rate. The time growth was affected by the duration for performing the classification since the number of datasets also expanded.
Table 3 describes the percentage of prediction loss during the real time attack scenario for all options. The average prediction loss was 43.5%. This event might be occurred because the Suricata sensor was overwhelmed by the attack and normal flow; therefore, most of the normal packets were dropped and caused the precision value to drop significantly.
In terms of resource usage during the classification process, Table 5 shows the MHN server’s CPU utilization for performing attack detection, classification, and mitigation processes. In average, the CPU usage pointed at 14.5%, indicating that the mentioned processes did not significantly exhaust the MHN server despite the fact that the server was flooded by the DDoS attack.
Table 3. Packet/prediction loss during the experiment Packet’s sending rate Number of packet being sent (normal and DDoS) Number of packet’s receive Packet/prediction loss 100 Packets/s 30,000 12,458 41.53% 500 Packets/s 11,722 49.07% 1,000 Packets/s 12,444 41.48% 2,000 Packets/s 12,577 41.92%
Table 5. MHN’s CPU usage in average Packet’s sending rate CPU usage in percentage 100 Packets/s 14.46% 500 Packets/s 15.24% 1,000 Packets/s 14.04% 2,000 Packets/s 14.51%
Packet’s sending rate Database extraction interval Accuracy Precision Recall F1 score 100 Packets/s 10s 99% 99% 99% 99% 30s 99% 54.12% 54.12% 54.12% 50s 99% 58.17% 58.17% 58.17% 70s 99% 55.41% 55.41% 55.41% 90s 99% 59.29% 59.29% 59.29% 500 Packets/s 10s 99% 99% 99% 99% 30s 99% 57.93% 57.93% 57.93% 50s 99% 56.16% 56.16% 56.16% 70s 99% 60.78% 60.78% 60.78% 90s 99% 55.19% 55.19% 55.19% 1,000 Packets/s 10s 99% 99% 99% 99% 30s 99% 57.29% 57.29% 57.29% 50s 99% 58.06% 58.06% 58.06% 70s 99% 61.74% 61.74% 61.74% 90s 99% 58.66% 58.66% 58.66% 2,000 Packets/s 10s 99% 99% 99% 99% 30s 99% 59.19% 59.19% 59.19% 50s 99% 56.99% 56.99% 56.99% 70s 99% 56.34% 56.34% 56.34% 90s 99% 58.72% 58.72% 58.72%
ISSN: 2252 8938 Int J Artif Intell, Vol. 11, No. 3, September 2022: 1094 1100 1098sending rate not significantly impacted the accuracy and the other variables which indicated that the Pseudo labelling model was consistent to perform the classification process. The low value of precision might be originated from the prediction loss during the attack or normal flow.
Table 4. The duration for installing the flow mitigation Packet’s sending rate Timestamp for flow installation (datetime to epoch ms) Timestamp of the attack (datetime to epoch ms) Time taken for install the mitigation flow 100 Packets/s 1585896414491 1585896400083 14408ms ~ 14s 500 Packets/s 1585897516757 1585897476750 40007ms ~ 40s 1,000 Packets/s 1585897981133 1585897951125 30008ms ~ 30s 2,000 Packets/s 1585898513413 1585898433410 80003ms ~ 80s
Table 2. Classification results
[3] M. A. Naagas, E. L. Mique Jr, T. D. Palaoag, and J. S. Dela Cruz, “Defense through deception network security model: securing university campus network from DOS/DDOS attack,” Bull. Electr. Eng. Informatics, vol. 7, no. 4, pp. 593 600, Dec. 2018, doi: 10.11591/eei.v7i4.1349.
4. CONCLUSION
[4] C. Kelly, N. Pitropakis, A. Mylonas, S. McKeown, and W. J. Buchanan, “A comparative analysis of honeypots on different cloud platforms,” Sensors, vol. 21, no. 7, Apr. 2021, doi: 10.3390/s21072433.
[5] V. Sethia and A. Jeyasekar, “Malware capturing and analysis using dionaea honeypot,” in 2019 International Carnahan Conference on Security Technology (ICCST), Oct. 2019, pp. 1 4., doi: 10.1109/CCST.2019.8888409.
[9] T. M. Thang, C. Q. Nguyen, and K. Van Nguyen, “Synflood spoofed source DDoS attack defense based on packet ID anomaly detection with bloom filter,” in 2018 5th Asian Conference on Defense Technology (ACDT), Oct. 2018, pp. 75 80., doi: 10.1109/ACDT.2018.8593121.
ACKNOWLEDGEMENTS
[15] F. D. S. Sumadi and C. S. K. Aditya, “Comparative Analysis of DDoS Detection Techniques Based on Machine Learning in OpenFlow Network,” in 2020 3rd International Seminar on Research of Information Technology and Intelligent Systems, ISRITI 2020, Dec. 2020, pp. 152 157., doi: 10.1109/ISRITI51436.2020.9315510.
[16] S. K. Dey and M. M. Rahman, “Effects of machine learning approach in flow based anomaly detection on software defined networking,” Symmetry (Basel)., vol. 12, no. 1, Dec. 2019, doi: 10.3390/sym12010007.
[10] A. Maslan, K. M. Bin Mohamad, and F. Binti Mohd Foozy, “Feature selection for DDoS detection using classification machine learning techniques,” IAES Int. J. Artif. Intell., vol. 9, no. 1, pp. 137 145, Mar. 2020, doi: 10.11591/ijai.v9.i1.pp137 145. [11] A. Fadlil, I. Riadi, and S. Aji, “Review of detection DDoS attack detection using naive bayes classifier for network forensics,” Bull. Electr. Eng. Informatics, vol. 6, no. 2,pp. 140 148, Jun. 2017, doi: 10.11591/eei.v6i2.605.
[21] W. Tian, M. Du, X. Ji, G. Liu, Y. Dai, and Z. Han, “Honeypot detection strategy against advanced persistent threats in industrial internet of things: a prospect theoretic game,” IEEE Internet Things J., vol. 8, no. 24, pp. 17372 17381, Dec. 2021, doi: 10.1109/JIOT.2021.3080527. [22] S. Asadollahi, B. Goswami, and M. Sameer, “Ryu controller’s scalability experiment on software defined networks,” in 2018 IEEE International Conference on Current Trends in Advanced Computing (ICCTAC), Feb. 2018, pp. 1 5., doi: 10.1109/ICCTAC.2018.8370397.
[8] X. Qin, T. Xu, and C. Wang, “DDoS attack detection using flow entropy and clustering technique,” in 2015 11th International Conference on Computational Intelligence and Security (CIS), Dec. 2015, pp. 412 415., doi: 10.1109/CIS.2015.105.
Int J Artif Intell ISSN: 2252 8938 Semi supervised approach for detecting distributed denial of service in … (Fauzi Dwi Setiawan Sumadi) 1099
[7] K. Nam and K. Kim, “A study on SDN security enhancement using open source IDS/IPS Suricata,” in 2018 International Conference on Information and Communication Technology Convergence (ICTC), Oct. 2018, pp. 1124 1126., doi: 10.1109/ICTC.2018.8539455.
[17] H. Wang and B. Wu, “SDN based hybrid honeypot for attack capture,” in 2019 IEEE 3rd Information Technology, Networking, Electronic and Automation Control Conference (ITNEC), Mar. 2019, pp. 1602 1606., doi: 10.1109/ITNEC.2019.8729425.
REFERENCES
The integration of SD honeypot network might become one of availability problem occurred on computer network. The implementation of SD honeypot integration produced positive impacts proven by the classification metrics stated in the previous section. The precision, recall, and F1 Score were not pointed at a high value because there was a fraction of the data test that was not being classified because of the packet loss. The time needed to install the mitigation rule increased with the growth of the database’s extraction interval. This might be occurred since the size of the captured data also expanded. In order to increase the classification metrics as a future project’s reference, the utilization of Extract, transform, and load (ETL) technique can be deployed for capturing all of the attacks over several similar honeypot sensors directly without involving MHN.
[2] “OpenFlow switch specification.” Open Networking Foundation.
[14] A. W. Muhammad, C. F. M. Foozy, and K. M. bin Mohammed, “Multischeme feedforward artificial neural network architecture for DDoS attack detection,” Bull. Electr. Eng. Informatics, vol. 10, no. 1, pp. 458 465, Feb. 2021, doi: 10.11591/eei.v10i1.2383.
[1] H. Kim and N. Feamster, “Improving network management with software defined networking,” IEEE Commun. Mag., vol. 51, no. 2, pp. 114 119, Feb. 2013, doi: 10.1109/MCOM.2013.6461195.
[18] M. Du and K. Wang, “An SDN enabled pseudo honeypot strategy for distributed denial of service attacks in industrial internet of things,” IEEE Trans. Ind. Informatics,vol. 16, no. 1, pp. 648 657, Jan. 2020,doi: 10.1109/TII.2019.2917912.
The authors would like to express sincere gratitude to the Informatics laboratory at The University of Muhammadiyah Malang for providing resources during the experiment.
[13] A. J. Mohammed, M. H. Arif, and A. A. Ali, “A multilayer perceptron artificial neural network approach for improving the accuracy of intrusion detection systems,” IAES Int. J. Artif. Intell., vol. 9, no. 4, pp. 609 615, Dec. 2020, doi: 10.11591/ijai.v9.i4.pp609 615.
[19] H. Lin, “SDN based in network honeypot: preemptively disrupt and mislead attacks in IoT networks,” May 2019 [20] X. Luo, Q. Yan, M. Wang, and W. Huang, “Using MTD and SDN based honeypots to defend DDoS attacks in IoT,” in 2019 Computing, Communications and IoT Applications (ComComAp), Oct. 2019, pp. 392 395., doi: 10.1109/ComComAp46287.2019.9018775.
[12] M. Idhammad, K. Afdel, and M. Belouch, “Semi supervised machine learning approach for DDoS detection,” Appl. Intell., vol. 48, no. 10, pp. 3193 3208, Oct. 2018, doi: 10.1007/s10489 018 1141 2.
[23] J. M. Ceron, C. Scholten, A. Pras, and J. Santanna, “MikroTik devices landscape, realistic honeypots, and automated attack classification,” in NOMS 2020 2020 IEEE/IFIP Network Operations and Management Symposium, Apr. 2020, pp. 1 9., doi: 10.1109/NOMS47738.2020.9110336.
[6] W. Cabral, C. Valli, L. Sikos, and S. Wakeling, “Review and analysis of cowrie artefacts and their potential to be used deceptively,” in 2019 International Conference on Computational Science and Computational Intelligence (CSCI), Dec. 2019, pp. 166 171., doi: 10.1109/CSCI49370.2019.00035.
Syaifuddin graduated with Master of Computer from Sepuluh Nopember Technological Institute (ITS), Surabaya. Currently, he is a senior lecturer in the Informatics Department University of Muhammadiyah Malang (UMM) and active as a cyber community builder at the local campus and regional levels. His areas of interest are cyber security, network forensics, malware analysis and security analysis. He can be contacted at email: syaifuddin_skom@umm.ac.id.
[25] Y. Li, R. Miao, M. Alizadeh, and M. Yu, “DetER: deterministic TCP replay for performance diagnosis,” in Proceedings of the 16th USENIX Symposium on Networked Systems Design and Implementation (NSDI), 2019, pp. 437 451.
Christian Sri Kusuma Aditya graduated with Master of Computer from Sepuluh Nopember Technological Institute (ITS), Surabaya. Currently, he is a lecturer in the Informatics Department University of Muhammadiyah Malang (UMM). His areas of interest are Data Science, Machine Learning, and Text Processing. He can be contacted at email: christianskaditya@umm.ac.id.
Ahmad Akbar Maulana graduated with bachelor’s degree of Informatics from the University of Muhammadiyah Malang (UMM), Malang. Currently he is a Fullstack Java Consultant in PT Xsis Mitra Utama, Jakarta. His area of interest is software development, computer network, and DevOps. He can be contacted at email: alanmy.maulana@gmail.com.
ISSN: 2252 8938 Int J Artif Intell, Vol. 11, No. 3, September 2022: 1094 1100 1100 [24] R. R. S, R. R, M. Moharir, and S. G, “SCAPY a powerful interactive packet manipulation program,” in 2018 International Conference on Networking, Embedded and Wireless Systems (ICNEWS), Dec. 2018, pp. 1 5., doi: 10.1109/ICNEWS.2018.8903954.
Fauzi Dwi Setiawan Sumadi achieved his master degree program in computer science at the University of Queensland, Australia which focused to analyze the vulnerability in software defined network. Nowadays, he becomes one of the main lecturers in Informatics Department at the University of Muhammadiyah Malang and maintains his research in the implementation of artificial intelligence in computer network, distributed computing, Cyber security, IoT, and the SDN. He can be contacted at email: fauzisumadi@umm.ac.id.
BIOGRAPHIES OF AUTHORS
Vera Suryani works as a Lecturer at School of Computer and Informatics, Telkom University since 2003. She achieved her Ph.D. from the Department of Electrical and Information Engineering Technology, Gadjah Mada University, Indonesia in 2019. Her research interests include computer networks, cybersecurity, distributed systems, and Internet of Things security. She can be contacted at email: verasuryani@telkomuniversity.ac.id.
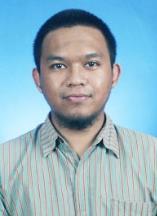




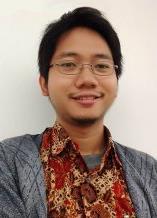




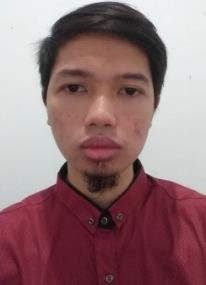




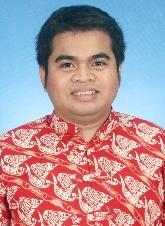




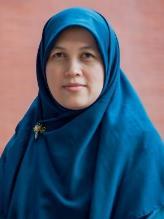




1. INTRODUCTION
Article history: Received Feb 17, 2022 Revised Jun 2, 2022 Accepted Jun 20, 2022 When the roads are monotonous, especially on the highways, the state of vigilance decreases and the state of drowsiness appears. Drowsiness is defined as the transitional phase from the awake to the sleepy state. However, In Morocco, the majority of fatal accidents on the highway are caused by drowsiness at the wheel, reaching 33.33% rate. Therefore, we proposed the conception and realization of an automatic method based on electroencephalogram (EEG) signals that can predict drowsiness in real time. The proposed work is based on time frequency analysis of EEG signals from a single channel (FP1 Ref), and drowsiness is predicted using a personalized and optimized machine learning model (optimized decision tree classification method) under Python. The results are much significant and optimized, improving the accuracy from 95.7% to 96.4% and a time consuming from 0.065 to 0.053 seconds.
The rate of deadly accidents on highway caused by drowsiness and falling asleep while driving based on the latest statistics of the ministry of equipment, transport, logistics and water, directorate of roads in Morocco is 33.3% as provided in [1], [2] These statistics gave us the idea of developing an automatic model that can predict drowsiness when occurring and before the situation becomes worst leading to dangerous accidents. Therefore, the idea of our system is not new, but it came to improve the performance and solve the limitations of the existing ones by using the latest processing software ‘Python’, also by providing the best processing techniques ‘time and frequency’ and machine learning (ML) algorithms to perform a better hybrid and automatic method of detecting drowsiness based on single channel of (EEG) signals [3]. As a result, our model based on an optimized decision tree (DT) classifier shows a higher performance compared to our previous one and to all the previous works, improving the accuracy and the time consuming. Our previous study (conference paper publishing in progress) was to conceive an efficient model based on a heavy analysis, during that period a detailed study was carried on the existing systems and their limitations. Therefore, the existing works like cited in our previous work were based on sensors only, based on physiological signals like EEG, electrocardiogram (ECG), and electro oculogram (EOG) [4] [8], or even a mix of these two techniques [9]. Chang et al. [10] proposed a smart glasses system that detects drowsiness
Keywords: drowsiness detection signals
IAES International Journal of Artificial Intelligence (IJ AI) Vol. 11, No. 3, September 2022, pp. 1101 1107 ISSN: 2252 8938, DOI: 10.11591/ijai.v11.i3.pp1101 1107 1101
Article Info ABSTRACT
RoadRealMachineHybridanalysisalgorithmlearningtimeanalysissafety
Electroencephalogram
A new hybrid and optimized algorithm for drivers’ drowsiness detection Mouad Elmouzoun Elidrissi1, Elmaati Essoukaki2, Lhoucine Ben Taleb1, Azeddine Mouhsen1 , Mohammed Harmouchi1 1Laboratory of Radiation Matter and Instrumentation, Faculty of Science and Technology, University Hassan 1st, Settat, Morocco 2Higher Institute of Health Sciences, University Hassan 1st, Settat, Morocco
electroencephalogram
Journal homepage: http://ijai.iaescore.com
This is an open access article under the CC BY SA license. Corresponding Author: Mouad Elmouzoun Elidrissi Laboratory of Radiation Matter and Instrumentation, Faculty of Science and Technology, University Hassan 1st Settat, Email:MoroccoM.elmouzounelidrissi@uhp.ac.ma
Drivers’
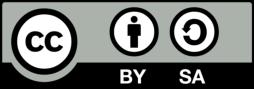
ISSN: 2252 8938 Int J Artif Intell, Vol. 11, No. 3, September 2022: 1101 1107 1102 using signals generated by accelerometers and gyroscopes, capturing the head ’s micro falls in addition to an infra red transceiver for capturing the blinking frequency and the eyes closure degree. Other works used algorithms that can detect drowsiness using a facial recognition or eyes regions detection by [11], [12], or also using a thermal imaging tehniques proposed by [13]. However, We did mention on our last work that using signals issued only from sensors and not physiological signals is not accurate nor evident to confirm the detection’s efficiency, because a driver’s blinking or eyes closure or even his head’s movement are a standard and spontaneous actions. So, the solution was to use a method and technique based on signals recorded from EEG, ECG, EOG and others, EEG signals in our work [14] [18]. To situate our work, the following works used a single channel study in addition to using the same dataset of EEG signals available at the Physionet database to compare our results and show the improvement added by our hybrid method.
Belakhdar et al. [19] proposed a technique that analyses the spectral domain of the EEG signals using MATLAB, applying the Fourier transform and an artificial neural network (ANN) classification. Their work reached an accuracy of 88.8% Bajaj et al. [20] reached an accuracy of 91% using tunable Q factor wavelet transform (TQWT) algorithm appliyed on the EEG signals, and the extreme machine learning classifier (ELM) The highest accuracy of 94.45% is reached by [21] using the wavelet packet transform (WPT) method and fed to the extra trees classifier. The proposed work aims to improve our previous algorithm’s efficiency of detecting drowsiness of drivers in the terms of rapidity and accuracy, using a personalized and optimized DT classifier that we will explain next. Our method proposed in this paper aims to provide an optimized and new hybrid algorithm drivers’ drowsiness detection based on the mixed temporal and frequential domains by processing a single channel of EEG records (FP1). Many researchers have confirmed that the most accurate position for detecting drowsiness is the FP1 position like published by [22]. Our proposed method is shown in Figure 1.
Figure 1. Flowchart of our proposed method
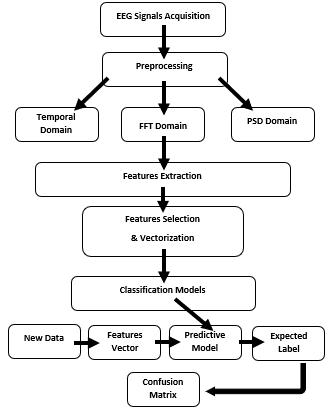
Int J Artif Intell ISSN: 2252 8938 A new hybrid and optimized algorithm for drivers’ drowsiness detection (Mouad Elmouzoun Elidrissi) 1103
2.1. Pre processing Phase
The open Physionet database is the one we used in our works because it’s the best to use for similar works. All the EEG records were artefact free and noise filtered right after the acquisition step using a 30 Hz low pass filter and a 50 Hz notch. The signals were extracted from the subjects under the 10 20 international system, they were males and females with different ages [23], [24].
2.2. Time segmentation phase
For: 0 ⩽ k ⩽ N 1 Xk =∑ ������ 2�������� �� �� 1 ��=0 (6) A comparison of the brain band’s power is calculated using the burg algorithm (spectrum analysis) to allow a good discrimination between the awake and drowsy states.
2. METHOD
In this phase, we proposed a frequency analysis of the recorded EEG signals using the fast Fourier transform. After extracting the same previous feature, the modulus of these features is calculated to eliminate the imaginary part and have only the real significant part.
This step aims to extract the most significant features using a single channel of EEG from three mixed domains (temporal, Fourier and spectral). We designed a function that can extract all the features one by one, and scales all of them in the right shape for the classification step. The use of the mixing features was not chosen randomly but after an analyze where we found that this mixture shows the highest accuracies and results. 2.3.1. Temporal domain analysis Eight parameters are calculated in the time domain in a way to distinguish the awake from the drowsy state. Using conditions to process intervals of 3 seconds, we calculated all the features manually according to the best ones resulting the best accuracies of the models. These features were the minima, the maxima, the amplitude peaks and our proper mean of amplitude peaks parameter, in addition to the following Theones:median: P(y ⩽ x) = P(x ⩽ z) (1) The mean: �� =∑���� �� (2) The variance: ������ = ∑(���� ��)2 �� (3) The standard deviation: ������ = √������ (4) The root mean square: RMS =√∑����2 �� (5) 2.3.2. Fourier and power spectral domain analysis
We applied a segmentation of 3 seconds of EEG signals instead of using the whole 30 seconds recording. The benefice of this time segmentation is to ensure stationarity of spectral analysis (fast Fourier transform (FFT) and power spectral density (PSD) analysis). In other terms also, to ensure the real time condition so that the process of detecting the drowsiness state do not take a higher time consumption.
2.3. Features Extraction Phase
Achieving a higher accuracy of a model depends on two studies, either we use a large segment of data to give the classifier a higher margin for the training and testing, or you try to build the analysis on solid features, therefore the first method is based on only PSD features, the second on only FFT features, the third method used only time features and the last one is our method based on the mixed features. As we can conclude, our hybrid model based on the mixed domains of features and our optimized DT classifier achieved the best accuracy compared to our previous work presented during an international conference (BML21: publishing on progress) and all the other selection of features and classifiers shown in Table 1. We used a personalized SearchGrid algorithm to select the best hyperparameters values of the DT classifier to achieve the best of accuracies as shown in Figure 2. A two axes study was conducted to compare our method to the previous ones using the same dataset and the aspect of single channel based processing in order to situate our method. The results are shown in Table 2. Right after we generated a comparison in terms of the executing time and accuracies In addition to the confusion matrix result of our optimized ML model shown in FigureComparing3. the results in Table 3, we conclude that the execution time is different from one classifier to another. But in terms of both time and accuracy, our optimized DT classifier is the most efficient and effective. The accuracy reached 96.4% and the execution time was within 53 milliseconds.
Table 1. Performance comparison between different classifiers applied on our selected features
A total of eight ML classification methods is tested in our study to compare the efficiency and keep the best model, and secondly, to select the most appropriate features. As a result, our optimized model showed the best of accuracies and time performance. The classifiers we used to compare our model’s efficiency are gaussian process (GP), K nearest neighbors (KNN), multilayer perceptron (MLP), support vector machine (SVM) (with its four kernels), our previous DT classifier, and finally the proposed optimized DT. 3. RESULTS AND DISCUSSION After extracting the features, all the calculated parameters were scaled and processed using ML classifiers. These classifiers depend on four parameters: i) True positive (TP): Prediction is positive (Drowsy state is predicted) and X is Drowsy; ii) True negative (TN): Prediction is negative (Awake state is predicted) and X is Awake; iii) False positive (FP): Prediction is positive (Drowsy state is predicted) and X is Awake; and iv) False negative (FN): Prediction is negative (Awake state is predicted) and X is Drowsy. Based on these parameters we could calculate our different scoring outputs: Precision = ���� ����+���� (8) Accuracy = ����+���� ����+����+����+���� (9) Sensitivity(Recall) = ���� ����+���� (10) F1 score = 2∗ ����������������∗������������ ������������������+������������ (11)
ISSN: 2252 8938 Int J Artif Intell, Vol. 11, No. 3, September 2022: 1101 1107 1104 PSD = 1 ��∑ ��(��)�� 2���� �� �� 1 ��=0 = 1 �� ���� (7)
2.4. Features selection & classification
Classifier First method Second method Third method Hybrid method
Optimized DT 51.2% 94.7% 95.0% 96.4% DT (previous work) 49.3% 93.6% 94.3% 95.7% SVM (Linear kernel) 49.7% 49.9% 49.4% 49.5% SVM (Plynomial kernel) 54.6% 85.5% 93.2% 83.6% SVM (Sigmoid kernel) 35.3% 66.8% 88.7% 66.0% SVM (RBF kernel) 71.9% 86.5% 93.3% 87.8% MLP 49.8% 74.1% 48.9% 75.6% KNN 90.6% 92.9% 94.1% 93.1% GP 49.1% 86.9% 49.0% 56%
Table 3. Time comparison between the different classifiers used in our method
Proposed Python 100 Hz 3s Hybrid Optimized Decision Tree 96.4% Previous work Python 100 Hz 3s Hybrid Decision Tree 95.7% (B and Chinara, 2021) [21] MATLAB 100 Hz 5s WPT ET 94.45% (Bajaj et al., 2020) [20] TQWT ELM 91.8% (Budak et al., 2019) [25] MATLAB 250 Hz 30s STFT, TQWT LSTM 94.31% (Belakhdar et al., 2018) [19] MATLAB 250 Hz 30s FFT ANN 88.8% (Ogino and Mitsukura, 2018) [26] Ipad app 512 Hz 10s PSD SVM, SWLDA 72.7%
Classifier Accuracy Time(s)
Figure 2. Search Grid output
Figure 3. Output of our optimized model (confusion matrix)
Int J Artif Intell ISSN: 2252 8938 A new hybrid and optimized algorithm for drivers’ drowsiness detection (Mouad Elmouzoun Elidrissi) 1105
Table 2. Performance comparison between our proposed model and existing models using same Physionet EEG dataset Works Platformused frequencySampling Size segmentsof Processingmethod Classification method Accuracy
The final phase was to save our model (trained) and using it to predict the state of new subjects in order to approve our work and calculate the prediction time. The state of these subjects used for the approval was known already and tested by our new hybrid model. Effectively, the model could predict all the given data and gave perfect predictions.
Proposed (Optimized DT) 96.4% 0.053 PreviousWork (DT) 95.7% 0.062 SVM (Linear kernel) 87.8% 0.985 Gaussian Process 56% 12.57 Stochastic Gradient Descent 65.5% 0.366 Multi Layer Perceptron 75.6% 5.144 Nearest Centroid 73.4% 0.006
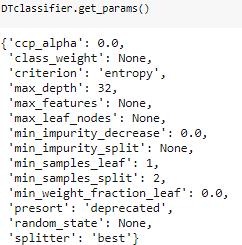
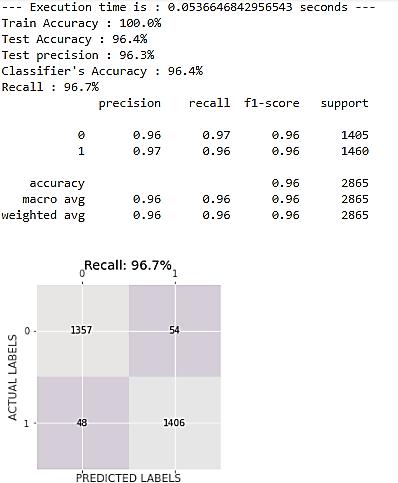
[17] T. L. T. Da Silveira, A. J. Kozakevicius, and C. R. Rodrigues, “Automated drowsiness detection through wavelet packet analysis of a single EEG channel,” Expert Syst. Appl., vol. 55, pp. 559 565, 2016, doi: 10.1016/j.eswa.2016.02.041.
[21] V. P. B and S. Chinara, “Automatic classification methods for detecting drowsiness using wavelet packet transform extracted time domain features from single channel EEG signal,” J. Neurosci. Methods, vol. 347, p. 108927, 2021, doi: 10.1016/j.jneumeth.2020.108927.
[24] A. L. Goldberger et al., “PhysioBank, PhysioToolkit, and PhysioNet: components of a new research resource for complex physiologic signals.,” Circulation, vol. 101, no. 23, 2000, doi: 10.1161/01.cir.101.23.e215.
[5] C. Jacobé de Naurois, C. Bourdin, C. Bougard, and J. L. Vercher, “Adapting artificial neural networks to a specific driver enhances detection and prediction of drowsiness,” Accid. Anal. Prev., vol. 121, no. July, pp. 118 128, 2018, doi: 10.1016/j.aap.2018.08.017.
[6] M. Tasaki, M. Sakai, M. Watanabe, H. Wang, and D. Wei, “Evaluation of Drowsiness during Driving using Electrocardiogram A driving simulation study,” no. Cit, pp. 1480 1485, 2010, doi: 10.1109/CIT.2010.264.
[4] T. Wijayanto, S. R. Marcillia, G. Lufityanto, B. B. Wisnugraha, T. G. Alma, and R. U. Abdianto, “The effect of situation awareness on driving performance in young sleep deprived drivers,” IATSS Res., 2020, doi: 10.1016/j.iatssr.2020.10.002.
[20] V. Bajaj, S. Taran, S. K. Khare, and A. Sengur, “Feature extraction method for classification of alertness and drowsiness states EEG signals,” Appl. Acoust., vol. 163, p. 107224, 2020, doi: 10.1016/j.apacoust.2020.107224.
Mr. Mouad Elmouzoun Elidrissi receives an excellence scholarship from the National Center for Scientific and Technical Research (CNRST Morocco) that we like to thank.
ISSN: 2252 8938 Int J Artif Intell, Vol. 11, No. 3, September 2022: 1101 1107 1106 4. CONCLUSION
[2] R. D. E. S. Statistiques, “Recueil des statistiques des accidents corporels de la circulation routière 2017,” (pp. 1 129), Mar. 11, 2022. [Online]. Available: https://www.narsa.ma/fr/etudes et statistiques
ACKNOWLEDGEMENTS
[7] Z. Mu, J. Hu, and J. Min, “Driver fatigue detection system using electroencephalography signals based on combined entropy features,” Appl. Sci., vol. 7, no. 2, 2017, doi: 10.3390/app7020150.
[10] W. Chang, L. Chen, S. Member, and Y. Chiou, “Design and implementation of a drowsiness fatigue detection system based on wearable smart glasses to increase road safety,” IEEE Trans. Consum. Electron., vol. PP, no. c, p. 1, 2018, doi: 10.1109/TCE.2018.2872162.
REFERENCES [1] R. Des and S. Des, “Recueil des statistiques des accidents corporels de la circulation routière 2016,” Mar. 11, 2022. [Online]. Available: http://narsa.ma/sites/default/files/2021 08/recueil%202020.pdf
[18] N. Gurudath and H. Bryan Riley, “Drowsy driving detection by EEG analysis using Wavelet Transform and K means clustering,” Procedia Comput. Sci., vol. 34, pp. 400 409, 2014, doi: 10.1016/j.procs.2014.07.045.
[22] A. M. Strijkstra, D. G. M. Beersma, B. Drayer, N. Halbesma, and S. Daan, “Subjective sleepiness correlates negatively with global alpha ( 8 12 Hz ) and positively with central frontal theta ( 4 8 Hz ) frequencies in the human resting awake electroencephalogram,” vol. 340, pp. 17 20, 2003, doi: 10.1016/S0304 3940(03)00033 8.
[11] E. Ouabida, A. Essadike, and A. Bouzid, “Optik Optical correlator based algorithm for driver drowsiness detection,” Opt. Int. J. Light Electron Opt., vol. 204, no. December 2019, p. 164102, 2020, doi: 10.1016/j.ijleo.2019.164102. [12] S. Dhanalakshmi, J. J. Rosepet, G. L. Rosy, and M. Philominal, “Drowsy Driver Identification Using MATLAB,” vol. 4, no. Iv, pp. 198 205, 2016. [13] S. E. H. Kiashari, A. Nahvi, H. Bakhoda, A. Homayounfard, and M. Tashakori, “Evaluation of driver drowsiness using respiration analysis by thermal imaging on a driving simulator,” Multimed. Tools Appl., 2020, doi: 10.1007/s11042 020 08696 x. [14] Z. Mardi, S. N. Ashtiani, and M. Mikaili, “EEG based drowsiness detection for safe driving using chaotic features and statistical tests,” J. Med. Signals Sens., vol. 1, no. 2, pp. 130 137, 2011, doi: 10.4103/2228 7477.95297.
[15] R. Kaur and K. Singh, “Drowsiness Detection based on EEG Signal analysis using EMD and trained Neural Network,” vol. 2, no. 10, pp. 157 161, 2013. [16] Ç. İ. Acı, M. Kaya, and Y. Mishchenko, “Distinguishing mental attention states of humans via an EEG based passive BCI using machine learning methods,” Expert Syst. Appl., vol. 134, pp. 153 166, 2019, doi: 10.1016/j.eswa.2019.05.057.
[19] I. Belakhdar, W. Kaaniche, R. Djemal, and B. Ouni, “Single channel based automatic drowsiness detection architecture with a reduced number of EEG features,” Microprocess. Microsyst., vol. 58, pp. 13 23, 2018, doi: 10.1016/j.micpro.2018.02.004.
[9] Y. Jiao, Y. Deng, Y. Luo, and B. L. Lu, “Driver sleepiness detection from EEG and EOG signals using GAN and LSTM networks,” Neurocomputing, 2020, doi: 10.1016/j.neucom.2019.05.108.
[23] A. Gibbings et al., “Clinical Neurophysiology EEG and behavioural correlates of mild sleep deprivation and vigilance,” Clin. Neurophysiol., vol. 132, no. 1, pp. 45 55, 2021, doi: 10.1016/j.clinph.2020.10.010.
[8] M. L. Jackson et al., “The utility of automated measures of ocular metrics for detecting driver drowsiness during extended wakefulness,” Accid. Anal. Prev., vol. 87, pp. 127 133, 2016, doi: 10.1016/j.aap.2015.11.033.
The present work proposed an optimized hybrid method of detecting drivers’ drowsiness based on time frequency analysis of a FP1 of EEG signals. We extracted a total of eight features from the three domains, the time, Fourier and PSD. After that, we trained eight ML models, MLP, GP, KNN, SVM (with its four kernels), DT and finally our optimized DT. We compared our proposed work to our previous one and to the ones based on the same dataset and the use of a single channel of EEG records. The added value of our model is the improvement of the detection’s performance in the term of accuracy, which achieved 96.4% and the processing time 0.053 seconds.
[25] U. Budak, V. Bajaj, Y. Akbulut, O. Atilla, and A. Sengur, “An Effective Hybrid Model for EEG Based,” vol. 19, no. 17, pp. 7624 7631, 2019.
[3] M. Wunderlin et al., “Shaping the slow waves of sleep : A systematic and integrative review of sleep slow wave modulation in humans using non invasive brain stimulation,” vol. 58, 2021, doi: 10.1016/j.smrv.2021.101438.
Mohammed Harmouchi Born in Sefrou, Morocco, in 1959. He is in charge of the master’s degree in biomedical engineering: instrumentation and maintenance. He is the Ex Director of the Laboratory of Radiation Matter and Instrumentation, Hassan First University, Settat, Morocco, where he is currently a Professor of Higher Education and the Ex Head of the Department of Applied Physics, Faculty of Science and Technology. He holds several publications and innovation patents in the fields of biophysics and biomedical engineering. (Based on document published on 9 October 2020). He can be contacted at email: mharmouchi14@gmail.com.
Int J Artif Intell ISSN: 2252 8938 A new hybrid and optimized algorithm for drivers’ drowsiness detection (Mouad Elmouzoun Elidrissi) 1107
Elmaati Essoukaki Born in 1 January 1990. He is currently a Professor of Instrumentation and Biomedical Engineering at Higher Institute of Health Sciences (ISSS), Hassan First University. His research interests include instrumentation and biomedical engineering, biomedical imaging, and signal processing. He holds several publications and innovation patents in the fields of biophysics and biomedical engineering. He can be contacted at email: e.essoukaki@uhp.ac.ma
Lhoucine Ben Taleb Born in February 20, 1991. He is a professor of Electrical and Biomedical Engineering at Hassan First University (UHP), Morocco, since 2021. After a Master degree from UHP (2014), he obtained in 2020 a PhD from the same university speciality electrical and biomedical engineering (in the Laboratory of Radiation Matter and Instrumentation). He has taught courses in instrumentation for the functional exploration and therapeutic applications, medical imaging technologies as well as microcontrollers and programmable logic controllers. He has published 6 scientific papers, and he is an inventor of one patent and a co inventor of another one. He can be contacted at email: l.bentaleb@uhp.ac.ma
Azeddine Mouhsen Born in 10 July 1967, He is Professor of Physics at Hassan First University, Morocco, since 1996. He holds a PhD from Bordeaux I University (France) in 1995 and a thesis from Moulay Isma¨ıl University, Morocco, in 2001. He specializes in instrumentation and measurements, sensors, applied optics, energy transfer, radiation matter interactions. He has taught courses in physical sensors, chemical sensors, instrumentation, systems tech nology, digital electronics and industrial data processing. He has published over 30 papers and he is the co inventor of one patent. Actually, he is the Director of Laboratory of radiation matter and Instrumentation. He can be contacted at email: az.mouhsen@gmail.com
[26] M. Ogino and Y. Mitsukura, “Portable drowsiness detection through use of a prefrontal single channel electroencephalogram,” Sensors (Switzerland), vol. 18, no. 12, pp. 1 19, 2018, doi: 10.3390/s18124477.
BIOGRAPHIES OF AUTHORS
Mouad Elmouzoun Elidrissi Born in 10 September 1996 in Casablanca, Morocco. He is a PhD student, received his master degree in Biomedical Engineering: Instrumentation and Maintenance from Faculty of Science and Technology Settat, in 2019. His research areas include EEG signals analysis for the use in drivers’ drowsiness detection, machine learning models conception, Application of artificial intelligence for drivers and road safety. Laboratory of Radiation Matter and Instrumenta tion (RMI), Faculty of Sciences and Technology, Hassan 1st University, Morocco. BP: 577, route de Casablanca. Settat, Morocco. His researches are in fields of machine learning and artificial intelligence, EEG signal processing, and biomedical instrumentation. He can be contacted at email: m.elmouzounelidrissi@uhp.ac.ma.
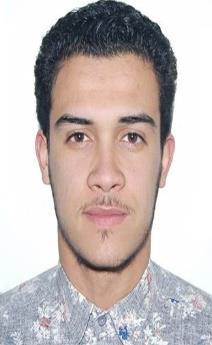



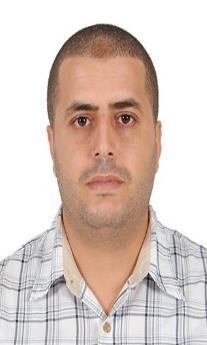



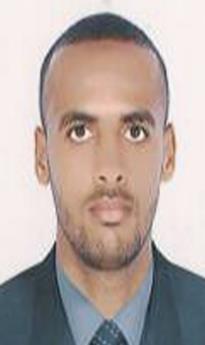



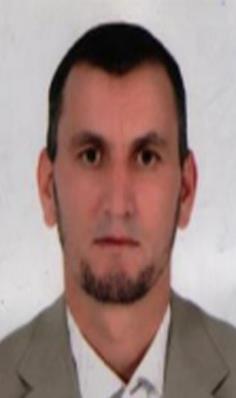



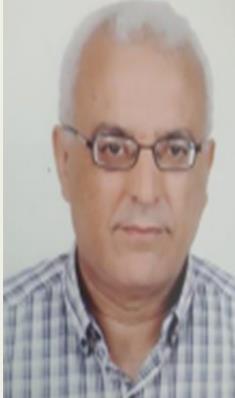



4Academy of Language Studies, Universiti Teknologi MARA, Shah Alam, Malaysia
Hanis Diyana Abdul Rahimapandi1, Ruhaila Maskat2, Ramli Musa3, Norizah Ardi4 1TESS Innovation Sdn Bhd, Petaling Jaya, Malaysia
1. INTRODUCTION As the coronavirus (COVID 19) pandemic spread across the globe, it is causing a significant degree of fear and concern in the public. In terms of public mental health, elevated depression rates are the most significant psychological effect to date. Younger adults had higher mental health rates, while adults enduring serious health issues had more mental health problems [1]. The analysis showed that mental health problems decreased by 5% with every year’s rise in age [2]. Children from lower socio economic classes who were exposed to experiences of mental health problems early in their lives, be it due to both or either parent, were more likely to become mentally ill later in life. Mood disorders and suicide related findings have soared over the past decade [3], [4]. According to the Institute for Public Health, mental health disorders among adults have increasingly become worrying from 10.7% in 1996 to 29.2% in 2015 [5]. Depression, the most common type of mental illness, is a psychological condition that happens to anyone at various ages due to specific reasons such as loss of self esteem and social environment. The symptoms faced by depressed individuals may have a severe effect on their capability to deal with any
2Faculty of Computer and Mathematical Sciences, Universiti Teknologi MARA, Shah Alam, Malaysia
IAES International Journal of Artificial Intelligence (IJ-AI) Vol. 11, No. 3, September 2022, pp. 1108~1118
ISSN: 2252 8938, DOI: 10.11591/ijai.v11.i3.pp1108 1118 1108
Article Info ABSTRACT
3Department of Psychiatry, Kulliyyah of Medicine, International Islamic University Malaysia, Kuantan, Malaysia
Article history: Received Aug 19, 2021 Revised Mar 11, 2022 Accepted Apr 9, 2022 Predicting depression can mitigate tragedies. Numerous works have been proposed so far using machine learning algorithms. This paper reviews publications from online electronic databases from 2016 to 2020 that use machine learning techniques to predict depression. The aim of this study is to identify important variables used in depression prediction, recent depression screening tools adopted, and the latest machine learning algorithms used. This understanding provides researchers with the fundamental components essential to predict depression. Fifteen articles were found relevant. We based our review on the systematic mapping study (SMS) method. Three research questions were answered through this review. We discovered that sixteen variables were deemed important by the literature. Not all of the reviewed literature utilizes depression screening tools in the prediction process. Nevertheless, from the five screening tools discovered, the most frequently used were hospital anxiety and depression scale (HADS) and hamilton depression rating scale (HDRS) for general population, while for literature targeting older population geriatric depression scale (GDS) was often employed. A total of twenty two machine learning algorithms were identified employed to predict depression and random forest was found to be the most reliable algorithm across the publications.
Journal homepage: http://ijai.iaescore.com
Depression prediction using machine learning: a review
Keywords: PredictionMachineLiteratureDepressionreviewlearning
This is an open access article under the CC BY SA license. Corresponding Author: Ruhaila Maskat Faculty of Computer and Mathematical Sciences, Universiti Teknologi MARA Shah Alam, Malaysia Email: ruhaila@fskm.uitm.edu.my
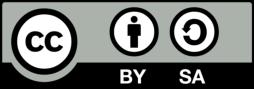
Int J Artif Intell ISSN: 2252 8938
Depression prediction using machine learning: a review (Hanis Diyana Abdul Rahimapandi) 1109 condition in everyday life, which significantly varies from the usual mood variations. Depression affects not only physical but also psychological well being [6]. It is associated with diabetes, hypertension, and back pain [7]. Besides that, a mental disease is often a burden in the form of tension, marriage breakdown, or homelessness for families, friends, caregivers, and other relationships [8]. Therefore, an initiative and commitment to prevention and treatment for depression are necessary.
Depression is one of the leading mental illnesses that is least diagnosed, considering the incidence and seriousness. The diagnosis and evaluation of signs of depression rely almost exclusively on data provided by patients, family members, friends, or caregivers [9]. This type of article, however, is inaccurate because it relies on the reporter’s total integrity. Depression related self perceived shame is widespread in societies worldwide and is associated with unwillingness to seek professional assistance [10]. Patients are also hesitant to express their depressive feelings with physicians, so a discussion of depression often relies heavily on a general practitioner’s willingness to engage with the patient. The prevalence of depression in Malaysia is considerably higher than in the United States and most other Western countries [11]. Depression is a severe mental illness and a significant public health issue that has a massive effect on society. In the worst case, depression can lead to suicide. Even though it is a severe psychological issue, fewer than half of people with this emotional problem have received mental health services [6]. It may be attributed to various reasons, including lack of knowledge of the disease. Additionally, researchers discovered that embarrassment and self stigmatization tend to pose as more significant factors for not obtaining medical attention than others’ actual prejudice and adverse reactions [12] The capability to predict depression using machine learning algorithms before conditions worsen is essential. Therefore, in this paper, we conducted a systematic review of literature from 2016 to 2021 (time of writing) to help researchers better understand this area. This review aims to firstly, identify variables relevant to the prediction of depression using machine learning techniques, secondly, identify the latest and most frequent screening types used in detecting depression and finally, popular state of the art techniques in machine learning to predict depression based on chosen metrics and values of performance.
Using machine learning techniques for the prediction of medical conditions is not new. Recent publications show applications in hepatitis [13], autism [14] and cancer [15]. Nevertheless, it is not without weaknesses. The primary weakness of any prediction pipeline involving machine learning techniques is the substantial dependence on correctly annotated data. If a dataset size is small, manually annotating each data point is feasible, however, in this big data era manual annotation of data has become impractical. Since machine learning techniques are trained on these annotations, a dataset with low quality labels can result in unreliable predictions. Another weakness is the risk of overfitting. In the pursuit of achieving higher prediction performance, these techniques can develop a tendency to induce a model fitted to specific unique data points which do not represent a large portion of the population. Thus, rendering the models useless. Our contribution via this study is a systematic review covering key aspects in predicting depression. Significant variables in previous works are identified, depression screening tools used are investigated and popular machine learning algorithms based on classical as well as new measurements of performance are highlighted.The paper is outlined as follows: in section 2, the systematic literature review methodology is explained. Our proposed methodology and research questions are detailed in section 3. Then, the results of our review are presented in section 4. Finally, in section 5 we conclude this paper.
Systematic mapping study (SMS) method organizes published research and their results into structured categories by systematically perusing its primary contents, methodology and results with the aim of mitigating bias and concluding using statistical meta analysis supported by evidence [16]. Although originally introduced for medical research, SMS method has been adapted for computing. Figure 1 shows the primary three phases of the SMS method used in our study. Each phase produces an outcome which in turn triggers the next phase. The SMS method begins with the formulation of research questions so that the coverage of existing literature can be framed. Once the scope of the review has been determined, a search of the literature is conducted involving the definition of information sources from various academic online databases, digital libraries, and search engines. Exploration of these sources is performed using search terms constructed to encompass the earlier formulated research questions using Boolean operators. From all the papers extracted, screening based on keywords, abstract, introduction and conclusion sections are carried out to identify only relevant papers that can provide answers to the previous questions.
2. SYSTEMATIC MAPPING STUDY (SMS) METHOD
3. RESEARCH METHODOLOGY
RQ2: Which depression screening tools were adopted? The answer to this question identifies the latest and most frequent screening types used in detecting depression.
RQ1: What variables were used by recent proposals in predicting depression? The answer to this question allows researchers to identify variables relevant to the prediction of depression.
3.3. Screening papers
3.2. Literature search A through search was conducted on four prominent electronic databases utilizing the following keywords: “depression prediction”, “mental health prediction”, and “anxiety, depression, and stress prediction”. The keywords were combined using Boolean AND expression and OR expression. The databases searched were: IEEE Xplore (http://ieeexplore.ieee.org), ACM Digital Library (http://www.portal.acm.org/dl.cfm), Elsevier ScienceDirect (http://www.sciencedirect.com), and Google Scholar (http://scholar.google.com)
The papers were examined based on their relevance to our constructed research questions. We analyzed the title, abstracts, and keywords to ascertain they lie within our focus of interest. Then, the papers were classified into two categories based on the following inclusion (I) and exclusion (E) criteria:
I1: Paper should directly relate to depression prediction using machine learning techniques.
At this phase, research questions were formed to seek literature within the scope of predicting depression using machine learning methods. The first question is concerned with what variables were used by recent proposals for the prediction process. This answer allows researchers to identify relevant variables. A good selection of variables helps to produce good prediction performance. The second question is which depression screening tools were adopted. This question provides an understanding of a particular screening tool that has been continuously used by researchers and how many of the proposals are not utilizing any screening tools. From the answer to this question, researchers can decide the necessity of adopting specific screening tools into their work. The final question is what machine learning techniques were proposed by existing research? This question helps direct researchers to state of the art machine learning techniques applied to depression prediction. Table 1 lists the constructed research questions and the motivations behind them.
1. Phases of the SMS method
In this section, we describe the steps on how we applied the SMS method to systematically review existing literature from 2016 till 2020 (time of writing). In each following subsection, we describe in detail the input, activity and output involved in each step. Finally, we illustrate the summarized evolution of paper filtration process to obtain the final relevant papers for review. These steps are defined research questions, literature search and screening papers.Figure
Table 1. Research questions and motivation Research questions Motivation
3.1. Define research questions
RQ3: What machine learning techniques were proposed by existing research? The answer to this question provides researchers with popular state of the art techniques in machine learning to predict depression based on chosen metrics and values of performance.
ISSN: 2252 8938 Int J Artif Intell, Vol. 11, No. 3, September 2022: 1108 1118 1110
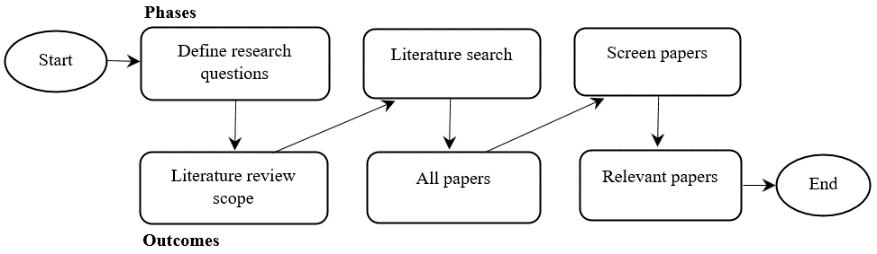
4.1. RQ1: what variables were used by recent proposals in predicting depression?
I2: Papers should provide answers to the research questions.
To predict depression, the researchers use several types of datasets. Some of them predict depression using demographics and clinical attributes, some use social media to collect information by using text analytics, hence, benefits from textual features instead of attributes. The various common variables in depression prediction found in 6 of the relevant papers are presented in this section. Table 3 shows the demographics and clinical variables that had been used in past research. Based on previous studies, it is found that the most used variable is age and marital status, followed by gender, educational status, and socio economic status. For clinical variables, diabetes has been used twice in previous studies, while others have only been used once and most of them in P3.
Int J Artif Intell ISSN: 2252 8938
I3: Papers should contain at least one of the search keywords.
The initial collection of papers from all electronic databases yielded 73 papers. Since there exists an overlap due to the search on Google Scholar, duplicates were removed with a remaining of 50 papers. Next, 32 irrelevant papers were excluded after the title and abstract of each paper were perused. The resulting 18 papers were then fully read through and resulted in 3 found irrelevant whereas the rest of the 15 papers were included in this review. Figure 2 shows the screening process.
E1: Posters, panels, abstracts, presentations, and article summaries. E2: Duplicates E3: Papers without full text
Depression prediction using machine learning: a review (Hanis Diyana Abdul Rahimapandi) 1111
Figure 2. Paper screening process
4. RESULTS AND DISCUSSION
The 15 relevant papers included in this review is listed in Table 2 by year, source, the scope of prediction and number of citations. The list suggests that studies on depression prediction were actively conducted in 2020 (31%) and 2016 (25%). The former is most likely due to the COVID 19 pandemic whereas for the latter no prominent event could be linked. In relation to the number of citations, sources based on computing and technology received a large number of citations since they lead to the introduction of new techniques, whereas medical centered sources are lesser cited, owing to their more general application of these new techniques. IEEE, a widely known online database, recorded the highest number of cited sources (ICHI and KDE).
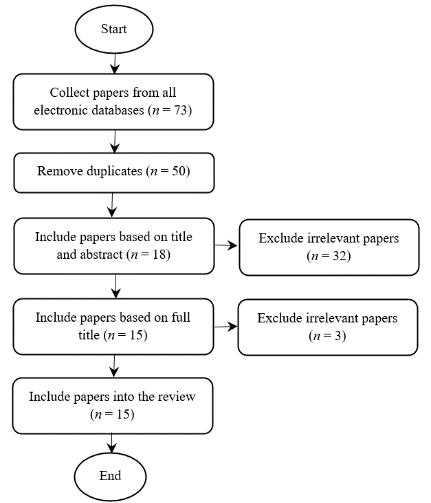
8.
3.
scale
which depression screening tools were adopted? Our review discovered
past studies in depression prediction: geriatric
patient
5.
Table 3. Variables used by recent proposals Variable
7.
6.
and
Table 2. List of relevant literatures
13.
15.
4.
screening tools popularly
PaperID Year Reference Source Scope of prediction Numbercitationsof P1 2016 [17] Biomedical Signal Processing and Control Depression 18 P2 2016 [18] International Journal of Computer Applications Depression 21 P3 2017 [19] Healthcare Technology Letters Anxiety and depression 34 P4 2017 [20] Proceedings 2017 IEEE International Conference on Healthcare Informatics, ICHI 2017 Depression 7 P5 2017 [21] Proceedings of the Twenty Sixth International Joint Conference on Artificial Intelligence Depression 109 P6 2018 [22] CEUR Workshop Proceedings Depressionanorexiaand 16 P7 2019 [23] Informatics in Medicine Unlocked Anxiety and depression 32 P8 2019 [24] Journal of Medical Internet research Depression 41 P9 2019 [25] International Conference on Human Centered Computing Depression NA P10 2019 [26] International Conference on Advances in Engineering Science Management and Technology (ICAESMT) 2019 Anxiety,anddepression,stress 16 P11 2020 [27] IEEE Transactions on Knowledge and Data Engineering Depression 85 P12 2020 [28] Procedia Computer Science Depression 20 P13 2020 [29] Doctoral dissertation, École de technologie supérieure Superior Technology School Depression 1 P14 2020 [30] Healthcare Depression 1 P15 2020 [31] Journal of Affective Disorders Anxiety,anddepression,stress 2 P2 P3 P4 P7 P14 P15 1. Age ✓ ✓ ✓ ✓ ✓ ✓ 2. Gender ✓ ✓ ✓ ✓ ✓ Residence status ✓ ✓ Educational status ✓ ✓ ✓ ✓ Marital status ✓ ✓ ✓ ✓ ✓ ✓ Income ✓ ✓ ✓ Employment status ✓ ✓ ✓ ✓ Socio economic status ✓ ✓ 9. Smoking Status ✓ ✓ ✓ 10. Drinking ✓ ✓ 11. Diabetes ✓ ✓ 12. Hearing problem ✓ Visual impairment ✓ 14. Mobility impairment ✓ Insomnia ✓ 16. Stroke ✓ 4.2. RQ2: 5 used by depression (GDS), depression (HADS), health questionnaire (PHQ), hamilton depression rating scale (HDRS) depression anxiety stress scale 21 (DASS 21). Refer to Table 4. We discovered that proposals predicting depression utilize screening tools when their methodology require the self construction of a dataset. The motivation driving this construction is mainly because of the absence of an available dataset necessary to accomplish a research’s unique objective of filling up a specific gap in the knowledge. For example, the use of GDS is targeted at screening depression in elders. These tools allow patients to assess themselves and ratings are based on this assessment. These self assessment tools are not meant to replace a psychiatrist’s diagnosis but instead function as a signpost to the presence of symptoms or to reinforce an earlier diagnosis that a psychiatrist may be considering. Our result shows that both HADS and HDRS were adopted by more research as compared to PHQ and DASS 21 in relation to the general population. GDS, however, was adopted when the older population is the subject of interest.
scale
hospital anxiety and
ISSN: 2252 8938 Int J Artif Intell, Vol. 11, No. 3, September 2022: 1108 1118 1112
Table 5. GDS severity ratings Severity Depression Normal 0 4 Mild 5 8 Moderate 9 11 Severe 12 15 4.2.2. Hospital anxiety and depression scale (HADS) HADS [34], [35] measures the severity of not only depression but also anxiety. Since its introduction in 1983, HADS has become a popular screening tool for these two mental conditions. Comprising of 7 questions for anxiety and 7 questions for depression, HADS can be easily completed within a few minutes. The validity of HADS has been proven and is now on the recommendation list of the National Institute for Health and Care Excellence (NICE) to diagnose depression and anxiety. Table 6 displays HADS’ severity ratings.
P1
Table 6. HADS severity ratings Severity Depression Mild 8 10 Moderate 11 14 Severe 15 21 4.2.3. PHQ The PHQ [36], [37] is a multipurpose method for screening, tracking, diagnosing, and measuring depression severity. It is a self administered instrument with two distinct types, the PHQ 2 containing two items and the PHQ 9 containing nine items. PHQ 2 assesses the frequency of depressive episodes and anhedonia for the last two weeks, while PHQ 9 presents a clinical diagnosis of depression and measures the severity of symptoms. Table 7 shows the PHQ severity rating.
Table 7. PHQ severity ratings Severity Depression Mild 0 5 Moderate 6 10 Moderately severe 11 15 Severe 16 20
Int J Artif Intell ISSN: 2252 8938 Depression prediction using machine learning: a review (Hanis Diyana Abdul Rahimapandi) 1113
Table 4. Screening tools adopted ID Screening tools GDS P2 GDS P3 HADS P4 None P5 None P6 None P7 HADS P8 None P9 PHQ P10 None P11 None P12 DASS 21 P13 HDRS P14 None P15 HDRS 4.2.1. Geriatric depression scale (GDS) GDS [32], [33] consists of 30 questions targeted at the older population of 65 years and more who are medically ill. Although other depression screening tools are available, GDS has become the popular tool for this category of people. GDS simply requires a yes or no answer of how an elder feels in the past week. Because of its high sensitivity of 92% and specificity of 89%, GDS is viewed to be a valid and reliable tool. Table 5 shows the severity ratings produced by GDS.
Paper
ISSN: 2252 8938 Int J Artif Intell, Vol. 11, No. 3, September 2022: 1108 1118 1114 4.2.4. DASS-21 DASS 21 is a compilation of three scales of self report by a patient that determines the patient’s depression, anxiety, and emotional stress states. The underlying notion is these states tend to be correlated where anxiety and depression were discovered to be comorbid illnesses [38] and depression is a stress related mental disorder [39]. Each state is measured by answering 7 questions relating to how a patient feels over the past week. DASS was designed to calculate the level of negative emotions to assist both researchers and clinicians to observe a patient’s condition over time with the aim of determining the course of treatment. Table 8 shows the DASS 21 severity ratings.Table8. DASS 21 severity ratings Severity Depression Anxiety Stress Normal 0 9 0 7 0 14 Mild 10 13 8 9 15 18 Moderate 14 20 10 14 19 25 Severe 21 27 15 19 26 33 Extremely Severe 28+ 20+ 34+ 4.2.5. HDRS HDRS [40], [41] is specialized in assessing the severity of depression and has also been proven useful before, during, and after therapy to assess a patient’s level of depression. It is widely perceived as an effective treatment for hospitalized patients. 21 items are listed in the HDRS form. The scoring basis is on the first 17 items, with 18 to 21 items used to qualify depression further. Table 9 shows the HDRS severity rating. Table 9. HDRS severity ratings Severity Depression Normal 0 7 Mild 8 13 Moderate 14 18 Severe 19 22 Very severe 23+ 4.3. RQ3: What machine learning techniques were proposed by existing research?
Table 10 shows a list of the proposed machine learning techniques that were used in past research. For papers that compare the performance of the techniques, the highest scored technique is also listed in the table. Figure 3 summarizes in a tree map the number of papers using the proposed technique. Most papers experimented on random forest (RF), support vector machine (SVM), random tree (RT), naïve Bayes (NB), logistic regression (LR) and decision tree (DT). While this indicates the popularity of a specific machine learning technique among researchers, it is more importantly to know which of these techniques consistently scores the best performance when applied over different datasets. Out of the 15 papers reviewed, 12 papers conducted a comparison of performance. Therefore, from Figure 4, the graph shows RF returning the best performance in 4 instances of the comparison. RF prevails across different performance metrics in terms of achieving the best performance against other machine learning techniques. This is not only true for classical performance metrics e.g., accuracy, precision, and recall, but also newer forms of performance metrics such as early risk detection error (ERDE). It is noteworthy of publications proposing newer machine learning techniques i.e., Sons & Spouses algorithm (SS) superseding RF on traditional measurements of performances specifically accuracy, f measure, precision, recall and area under the receiver curve. A particularly new performance metric is ERDE formulated specifically for detecting mental illness early.
CNNBNBAADANomenclature::AdaBoost:Bagging:BayesNet:convolutional neural networks DT: decision Tree GB: gradient Boosting KNN: K nearest neighbor LR: logistic regression MDL: multimodal depressive dictionary learning
Proposed machine learning techniques
Int J Artif Intell ISSN: 2252 8938 Depression prediction using machine learning: a review (Hanis Diyana Abdul Rahimapandi) 1115
NB:
PaperID
NN:
Machine techniqueslearningused Best technique Performance metrics performanceBest P1 RF, RT, MLP, and SVM RF RooRootMeanAccuracyabsoluteerrormeansquarederrorRelativeabsoluteerrortrelativesquarederror 44.7924.3095.450.120.22 P2 BN, LR, MLP, SMO, and decision table BN ROCAccuracyPrecisionarea Root mean squared error 91.670.920.980.25 P3 BN, LR, MLP, NB, RF, RT, DT, subsequential,optimization,randomrandomspace,andKstar RF Precision/positiveFalseTrueAccuracypositiveratepositiverateprediction value F measures Area under the receiver curve 94.389.110.9898989 P4 Stacking of LR DT, NBN, NN, SVM LR (base level learner) with DT, NBN, NN, SVM (meta level learner) Mean area under the receiver curve Mean accuracy 8675 P5 NB, MSNL, WDL and MDL MDL F1PrecisionRecallmeasureAccuracy 84848584 P6 CNN with TF informationIDF Not compared ERDE5 ERDE50 F score 10.819.2237 P7 CatBoost, LR, NB, RF, and SVM CatBoost AccuracyPrecision 8489 P8 DT, RT, and RF RF ERDE5 ERDE50 FPrecisionmeasureRecall 15.2018.5120120 P9 BN, SVM, SMO, RT, and DT BN Accuracy 77.8 P10 NB, RF, GB, and EnsembleClassifierVote Ensemble Vote Classifier AccuracyFscore 76.859 P11 CNN Not compared ERDE20 ERDE20 Flatency 0.457.479.46 P12 NB, RF, DT, SVM, and KNN RF SpecificityErrorAccuracyratePrecisionRecallF1score 76.691.067.888.10.2079.8 P13 SVM, RT, and RF RT AccuracyRecallPrecision 91.391.291.3 P14 SS, TAN, LR, DT, NN, SVM, ADA, BA, RF, RSS SS FAccuracymeasure Area under the receiver curve PrecisionRecall 90.693.176.993.091.8
MLP: multi layer perceptron MSNL: multiple social networking learning naïve Bayes neural network random forest RT: random tree RSS: random subspace SMO: sequential minimal optimization SS: Sons & Spouses SVM: support vector machine WDL: wasserstein dictionary Tablelearning10.
RF:
We are extremely grateful to the reviewers who took the time to provide constructive feedback and useful suggestions for the improvement of this article. The Malaysian Government is funding this research through the Fundamental Research Grant Scheme (FRGS) at Universiti Teknologi MARA (UiTM) Shah Alam, Malaysia (FRGS/1/2019/SS05/UITM/02/5).
REFERENCES
Figure 3. The number of papers using the proposed technique
5. CONCLUSION
ACKNOWLEDGEMENTS
ISSN: 2252 8938 Int J Artif Intell, Vol. 11, No. 3, September 2022: 1108 1118 1116
Figure 4. Techniques with consistently high performance
In this timely paper, we have reviewed depression prediction literature from 2016 to 2020 that used machine learning techniques. We employed the SMS method, and the result is a total of 15 works were found relevant to the research questions constructed. The research questions focus on three important aspects of predicting depression using machine learning; they are the variables used in the literature to predict, the screening tools adopted, the machine learning techniques experimented, the metrics employed to measure each techniques’ performance and the highest values achieved by the top performing techniques. Our review has led us to conclude that information on age, marital status, gender, educational status, and socio economic status are repeatedly used across the proposals. In addition, most of the works which made use of depression screening tools relied on self reporting types. Furthermore, Random Forest was not only the most popular machine learning algorithm among researchers but also returns the best performance in a majority of the time inclusive of newer performance metrics e.g., ERDE. It is expected that this survey will enlighten researchers on the latest machine learning techniques, performance measurements and variables used in predicting depression.
[1] H. Dai, S. X. Zhang, K. H. Looi, R. Su, and J. Li, “Perception of health conditions and test availability as predictors of adults’ mental health during the COVID 19 pandemic: a survey study of adults in Malaysia,” International Journal of Environmental Research and Public Health, vol. 17, no. 15, Jul. 2020, doi: 10.3390/ijerph17155498. [2] N. Sahril, N. A. Ahmad, I. B. Idris, R. Sooryanarayana, and M. A. Abd Razak, “Factors associated with mental health problems among Malaysian children: a large population based study,” Children, vol. 8, no. 2, Feb. 2021, doi: 10.3390/children8020119.
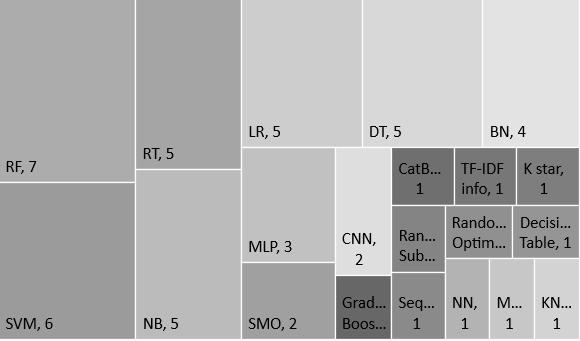
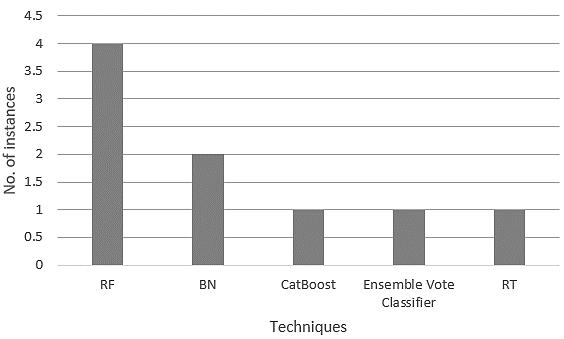
[21] G. Shen et al., “Depression detection via harvesting social media: a multimodal dictionary learning solution,” in Proceedings of the Twenty Sixth International Joint Conference on Artificial Intelligence, Aug. 2017, pp. 3838 3844., doi: 10.24963/ijcai.2017/536.
[23] A. Sau and I. Bhakta, “Screening of anxiety and depression among seafarers using machine learning technology,” Informatics in Medicine Unlocked, vol. 16, 2019, doi: 10.1016/j.imu.2019.100228.
[25] Z. Yang, H. Li, L. Li, K. Zhang, C. Xiong, and Y. Liu, “Speech based automatic recognition technology for major depression disorder,” in Lecture Notes in Computer Science (including subseries Lecture Notes in Artificial Intelligence and Lecture Notes in Bioinformatics), vol. 11956, 2019, pp. 546 553., doi: 10.1007/978 3 030 37429 7_55.
[29] B. Abdallah, “Computer based technique to detect depression in Alzheimer patients,” Université du Québec, 2020.
Int J Artif Intell ISSN: 2252 8938 Depression prediction using machine learning: a review (Hanis Diyana Abdul Rahimapandi) 1117
[32] S. A. Greenberg, “The geriatric depression scale (GDS) validation of a geriatric depression screening scale: a preliminary report,” Best Practices in Nursing Care to Older Adults, no. 4, 2019 [33] J. Wancata, R. Alexandrowicz, B. Marquart, M. Weiss, and F. Friedrich, “The criterion validity of the geriatric depression scale: a systematic review,” Acta Psychiatrica Scandinavica, vol. 114, no. 6, pp. 398 410, Dec. 2006, doi: 10.1111/j.1600 0447.2006.00888.x.
[34] A. S. Zigmond and R. P. Snaith, “The hospital anxiety and depression scale,” Acta Psychiatrica Scandinavica, vol. 67, no. 6, pp. 361 370, Jun. 1983, doi: 10.1111/j.1600 0447.1983.tb09716.x.
[31] N. Xiong et al., “Demographic and psychosocial variables could predict the occurrence of major depressive disorder, but not the severity of depression in patients with first episode major depressive disorder in China,” Journal of Affective Disorders, vol. 274, pp. 103 111, Sep. 2020, doi: 10.1016/j.jad.2020.05.065.
[16] K. Petersen, R. Feldt, S. Mujtaba, and M. Mattsson, “Systematic mapping studies in software engineering,” in International Journal of Software Engineering and Knowledge Engineering, Jun. 2008, vol. 17, no. 1, pp. 33 55., doi: 10.14236/ewic/EASE2008.8.
[15] C. K. Chin, D. A. binti Awang Mat, and A. Y. Saleh, “Hybrid of convolutional neural network algorithm and autoregressive integrated moving average model for skin cancer classification among Malaysian,” IAES International Journal of Artificial Intelligence (IJ AI), vol. 10, no. 3, pp. 707 716, Sep. 2021, doi: 10.11591/ijai.v10.i3.pp707 716.
[4] L. Brådvik, “Suicide risk and mental disorders,” International Journal of Environmental Research and Public Health, vol. 15, no. 9, Sep. 2018, doi: 10.3390/ijerph15092028.
[20] E. S. Lee, “Exploring the performance of stacking classifier to predict depression among the elderly,” in 2017 IEEE International Conference on Healthcare Informatics (ICHI), Aug. 2017, pp. 13 20., doi: 10.1109/ICHI.2017.95.
[5] A. Bakar et al., “National health and morbidity survey 2015 volume ii : non communicable diseases, risk factors & other health problems,” 2015.
[14] N. A. Mashudi, N. Ahmad, and N. M. Noor, “Classification of adult autistic spectrum disorder using machine learning approach,” IAES International Journal of Artificial Intelligence (IJ AI), vol. 10, no. 3, pp. 743 751, Sep. 2021, doi: 10.11591/ijai.v10.i3.pp743 751.
[17] I. M. Spyrou, C. Frantzidis, C. Bratsas, I. Antoniou, and P. D. Bamidis, “Geriatric depression symptoms coexisting with cognitive decline: a comparison of classification methodologies,” Biomedical Signal Processing and Control, vol. 25, pp. 118 129, Mar. 2016, doi: 10.1016/j.bspc.2015.10.006.
[22] Y. T. Wang, H. H. Huang, and H. H. Chen, “A neural network approach to early risk detection of depression and anorexia on social media text,” in CEUR Workshop Proceedings, 2018, vol. 2125.
[30] F. J. Costello, C. Kim, C. M. Kang, and K. C. Lee, “Identifying high risk factors of depression in middle aged persons with a novel sons and spouses bayesian network model,” Healthcare, vol. 8, no. 4, Dec. 2020, doi: 10.3390/healthcare8040562.
[12] G. Schomerus, H. Matschinger, and M. C. Angermeyer, “The stigma of psychiatric treatment and help seeking intentions for depression,” European Archives of Psychiatry and Clinical Neuroscience, vol. 259, no. 5, pp. 298 306, Aug. 2009, doi: 10.1007/s00406 009 0870 y. [13] J. E. Aurelia, Z. Rustam, I. Wirasati, S. Hartini, and G. S. Saragih, “Hepatitis classification using support vector machines and random forest,” IAES International Journal of Artificial Intelligence (IJ AI), vol. 10, no. 2, pp. 446 451, Jun. 2021, doi: 10.11591/ijai.v10.i2.pp446 451.
[6] K. Katchapakirin, K. Wongpatikaseree, P. Yomaboot, and Y. Kaewpitakkun, “Facebook social media for depression detection in the Thai community,” in 2018 15th International Joint Conference on Computer Science and Software Engineering (JCSSE), Jul. 2018, pp. 1 6., doi: 10.1109/JCSSE.2018.8457362.
[10] K. M. Griffiths, B. Carron Arthur, A. Parsons, and R. Reid, “Effectiveness of programs for reducing the stigma associated with mental disorders. a meta analysis of randomized controlled trials,” World Psychiatry, vol. 13, no. 2, pp. 161 175, Jun. 2014, doi: 10.1002/wps.20129. [11] S. H. Yeoh, C. L. Tam, C. P. Wong, and G. Bonn, “Examining depressive symptoms and their predictors in malaysia: stress, locus of control, and occupation,” Frontiers in Psychology, vol. 8, pp. 1 10, Aug. 2017, doi: 10.3389/fpsyg.2017.01411.
[18] I. Bhakta and S. Arkaprabha, “Prediction of depression among senior citizens using machine learning classifiers,” International Journal of Computer Applications, vol. 144, no. 7, pp. 11 16, 2016 [19] A. Sau and I. Bhakta, “Predicting anxiety and depression in elderly patients using machine learning technology,” Healthcare Technology Letters, vol. 4, no. 6, pp. 238 243, Dec. 2017, doi: 10.1049/htl.2016.0096.
[26] A. Kumar, A. Sharma, and A. Arora, “Anxious depression prediction in real time social data,” SSRN Electronic Journal, pp. 1 7, 2019, doi: 10.2139/ssrn.3383359. [27] M. Trotzek, S. Koitka, and C. M. Friedrich, “Utilizing neural networks and linguistic metadata for early detection of depression indications in text sequences,” IEEE Transactions on Knowledge and Data Engineering, vol. 32, no. 3, pp. 588 601, Mar. 2020, doi: 10.1109/TKDE.2018.2885515.
[24] F. Cacheda, D. Fernandez, F. J. Novoa, and V. Carneiro, “Early detection of depression: social network analysis and random forest techniques,” Journal of Medical Internet Research, vol. 21, no. 6, Jun. 2019, doi: 10.2196/12554.
[8] T. Kongsuk, S. Supanya, K. Kenbubpha, S. Phimtra, S. Sukhawaha, and J. Leejongpermpoon, “Services for depression and suicide in Thailand,” WHO South East Asia Journal of Public Health, vol. 6, no. 1, 2017, doi: 10.4103/2224 3151.206162.
[7] J. F. Greden and R. Garcia tosi, Mental health in the workplace: strategies and tools to optimize outcomes. Cham: Springer International Publishing, 2019., doi: 10.1007/978 3 030 04266 0.
[9] Y. Yang, C. Fairbairn, and J. F. Cohn, “Detecting depression severity from vocal prosody,” IEEE Transactions on Affective Computing, vol. 4, no. 2, pp. 142 150, Apr. 2013, doi: 10.1109/T AFFC.2012.38.
[28] A. Priya, S. Garg, and N. P. Tigga, “Predicting anxiety, depression and stress in modern life using machine learning algorithms,” Procedia Computer Science, vol. 167, pp. 1258 1267, 2020, doi: 10.1016/j.procs.2020.03.442.
[3] J. Bilsen, “Suicide and youth: risk factors,” Frontiers in Psychiatry, vol. 9, pp. 1 5, Oct. 2018, doi: 10.3389/fpsyt.2018.00540.
Prof. Ramli Musa is a consultant psychiatrist at Department of Psychiatry, International Islamic University Malaysia (IIUM). He received a few accolades including Outstanding Research Quality Award (Health and Allied Sciences) for 2 consecutive years (2012 and 2013), University Best Researcher Award; Best Fundamental Research Grant Scheme (FRGS) (Social Science) 2015, winner in the 14th International Conference and Exposition on Inventions by Institutions of Higher Learning (PECIPTA 2015), Commercial Potential Award; winner for The Best Project FRGS 2/2011 by Ministry of Higher Education, Malaysia Technology Expo 2018. Being passionate in research, he established a database of all the translated and validated questionnaires in Malaysian language and a website called the Mental Health Information and Research (MaHIR). He can be contacted at: drramli@iium.edu.my.
[39] P. Koutsimani, A. Montgomery, and K. Georganta, “The relationship between burnout, depression, and anxiety: a systematic review and meta analysis,” Frontiers in Psychology, vol. 10, Mar. 2019, doi: 10.3389/fpsyg.2019.00284.
[40] S. Obeid, C. Abi Elias Hallit, C. Haddad, Z. Hany, and S. Hallit, “Validation of the hamilton depression rating scale (HDRS) and sociodemographic factors associated with Lebanese depressed patients,” L’Encéphale, vol. 44, no. 5, pp. 397 402, Nov. 2018, doi: 10.1016/j.encep.2017.10.010.
ISSN: 2252 8938 Int J Artif Intell, Vol. 11, No. 3, September 2022: 1108 1118 1118 [35] I. Bjelland, A. A. Dahl, T. T. Haug, and D. Neckelmann, “The validity of the hospital anxiety and depression scale,” Journal of Psychosomatic Research, vol. 52, no. 2, pp. 69 77, Feb. 2002, doi: 10.1016/S0022 3999(01)00296 3. [36] J. Ford, F. Thomas, R. Byng, and R. McCabe, “Use of the patient health questionnaire (PHQ 9) in practice: interactions between patients and physicians,” Qualitative Health Research, vol. 30, no. 13, pp. 2146 2159, Nov. 2020, doi: 10.1177/1049732320924625. [37] L. P. Richardson et al., “Evaluation of the patient health questionnaire 9 item for detecting major depression among adolescents,” Pediatrics, vol. 126, no. 6, pp. 1117 1123, Dec. 2010, doi: 10.1542/peds.2010 0852. [38] N. H. Kalin, “The critical relationship between anxiety and depression,” American Journal of Psychiatry, vol. 177, no. 5, pp. 365 367, May 2020, doi: 10.1176/appi.ajp.2020.20030305.
[41] M. Zimmerman, J. H. Martinez, D. Young, I. Chelminski, and K. Dalrymple, “Severity classification on the Hamilton depression rating scale,” Journal of Affective Disorders, vol. 150, no. 2, pp. 384 388, Sep. 2013, doi: 10.1016/j.jad.2013.04.028.
BIOGRAPHIES OF AUTHORS Hanis Diyana Abdul Rahimapandi completed her M.Sc. in Data Science from the Faculty of Computer and Mathematical Sciences, Universiti Teknologi MARA, in 2021. In 2018, she received a B.Sc. in Management Mathematics from the Faculty of Computer and Mathematical Sciences, Universiti Teknologi MARA, Arau. She is currently employed with TESS Innovation Sdn Bhd in Selangor, Malaysia, as a Data Analyst. Machine learning and data mining are two of the topics of her research. She can be contacted at email: hd.hanisdiyana@gmail.com. Dr. Ruhaila Maskat is a senior lecturer at the Faculty of Computer and Mathematical Sciences, Universiti Teknologi MARA Shah Alam, Malaysia. In 2016, she was awarded a Ph.D. in Computer Science from the University of Manchester, United Kingdom. Her research interest then was in Pay As You Go dataspaces which later evolved to Data Science where she is now an EMC Dell Data Associate as well as holding four other professional certifications from RapidMiner in the areas of machine learning and data engineering. Recently, she was awarded with the Kaggle BIPOC grant. Her current research grant with the Malaysian government involves conducting analytics on social media text to detect mental illness. She can be contacted at: ruhaila@fskm.uitm.edu.my.
Dr. Norizah Ardi is an Associate Professor at Academy of Language Studies, Universiti Teknologi MARA Shah Alam since July 2017. She has Ph D in Malay Language Studies and an expertise in Malay Linguistics (Sociolinguistics, Applied Linguistics, Translation Studies). She was teaching Malay Language course at Universiti Teknologi MARA since 1994. Currently, she involved with several research project regarding language and computational linguistics. She is member of Malaysian Translation Association and Member of Malaysian Linguistic Association Malaysia. She has published over 40 papers in national and international journals and conferences. She can be contacted at email: norizah@uitm.edu.my.
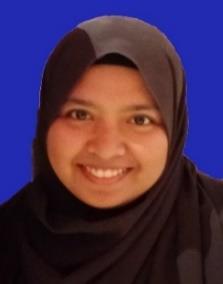




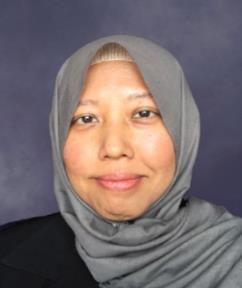




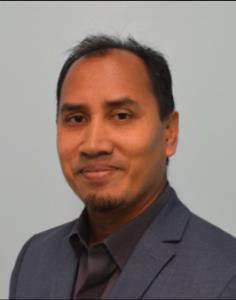




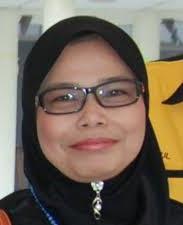




Corresponding Author: Anindita Septiarini Department of Informatics, Engineering Faculty, Mulawarman University Jl. Sambaliung No. 9, Samarinda, Indonesia Email: anindita@unmul.ac.id
Article Info ABSTRACT
Hamdani Hamdani1 , Heliza Rahmania Hatta1 , Novianti Puspitasari1 , Anindita Septiarini1 , Henderi2
1Department of Informatics, Engineering Faculty, Mulawarman University, Samarinda, Indonesia 2Informatics Engineering, Faculty of Science and Technology, University of Raharja, Tangerang, Indonesia
This is an open access article under the CC BY SA license.
SMachineDengueCrossClassificationvalidationlearningupportvectormachine
1. INTRODUCTION
Article history: Received Oct 16, 2021 Revised May 20, 2022 Accepted Jun 18, 2022 Dengue is a dangerous disease that can lead to death if the diagnosis and treatment are inappropriate. The common symptoms that occur, including headache, muscle aches, fever, and rash. Dengue is a disease that causes endemics in several countries in South Asia and Southeast Asia. There are three varieties of dengue, such as dengue fever (DF), dengue hemorrhagic fever (DHF), and dengue shock syndrome (DSS). This disease can currently be classified using a machine learning approach with the input data being the dengue symptoms. This study aims to classify dengue types consisting of three classes: DF, DHF, and DSS using five classification methods including C.45, decision tree (DT), k nearest neighbor (KNN), random forest (RF), and support vector machine (SVM). The dataset used consists of 21 attributes, which are the dengue symptoms. It was collected from 110 patients. The evaluation method was conducted using cross validation with k folds of 3, 5, and 10. The dengue classification method was evaluated using three parameters: precision, recall, and accuracy, which were most optimally achieved. The most optimal evaluation results were obtained using SVM with k fold 3 and 10 with precision, recall, and accuracy values reaching 99.1%, 99.1%, and 99.1%, respectively.
Nowadays, computer technology has been applied in various fields, including the medical field, in expert systems [1]. Over the last few years, expert systems have been developed. The expert system is constantly evolving because it can be integrated into clinical decision making to predict disease and assist physicians in diagnosis. This system is a computer program that contains knowledge from one or more human experts related to a particular disease. Expert systems help patients find out the diagnosis results more efficiently based on the symptoms that occur and are felt. Moreover, it can be used at any time to become more economical. Therefore, this system contributes to disseminating expert knowledge to wider users. Expert systems can provide a more accessible and helpful way for human experts to develop and test new theories, especially in healthcare. The data used in the expert system can vary, such as images [2] [4], signals [5], or medical record data which includes name, age, laboratory test results, and symptoms of the patient [6] [8]. In the medical field, several expert systems have been developed, for example for estimating drug doses [6], [9], monitoring the disease progress [1], [2], [10], and detecting several types of diseases such as diabetes mellitus [11], pancreatic cancer [12], breast cancer [13], glaucoma [14], and dengue fever (DF) [15] [17]
Keywords:
IAES International Journal of Artificial Intelligence (IJ-AI) Vol. 11, No. 3, September 2022, pp. 1119~1129 ISSN: 2252 8938, DOI: 10.11591/ijai.v11.i3.pp1119 1129 1119
Journal homepage: http://ijai.iaescore.com classification method using support vector machines and cross-validation techniques
Dengue
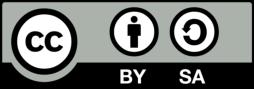
The study based on machine learning was developed using several approaches, including KNN, linear SVM, naive Bayes, J48, adaboost, bagging, and stacking, to classify autism spectrum disorders in adults. The best results were obtained utilizing the bagging, linear SVM, and naive Bayes methods with an accuracy of 100% based on the test results using cross validation with k fold 3, 5, and 10 [6]. Classification of hepatitis disease applied based on SVM including linear SVM, polynomial SVM, gaussian radial basis function (RBF) SVM, and RF with a comparison of training and test data of 90% and 10%. The proposed SVM and RF methods succeeded in predicting the data correctly. They managed to achieve the best results with a value of 0.995 [8]. Comparison of SVM kernel selection implemented on diabetes dataset using linear SVM, polynomial SVM, and RBF kernel. The results obtained using the linear SVM kernel get the best results with an accuracy of 77.34%, while the RBF kernel obtains the lowest results with an accuracy of 65.10% [27]Subsequently, the extraction of the contour cup on the retinal fundus image was carried out to detect the patient of glaucoma by applying the multi layer perceptron (MLP), KNN, naive Bayes, and SVM methods. The SVM method achieved the best accuracy results with a value of 94.44%, while the lowest results were performed by the MLP method with a value of 72.22% [14]. Improving the quality of mammogram images based on the region of interest is needed to obtain optimal breast cancer classification results using the hybrid optimum feature selection (HOFS) and ANN as the classifier feature selection method. The use of feature selection is able to reduce the number of features and improve the classification results with fewer features based on the values of accuracy, sensitivity, and specificity of 99.7%, 99.5%, and 100%, respectively [13]. Antibiotic resistance detection based on machine learning was classified into two classes: resistant and sensitive. With the area under the curve weighted metrics of 0.822 and 0.850, respectively, the stack ensemble technique produced the best results in the original and balanced datasets. Sex, age, sample type, Gram stain, 44 antimicrobial substances, and antibiotic susceptibility values were all included as the dataset attributes [7] This study aims to classify dengue disease varieties divided into DF, DHF, and DSS. The input data used are symptoms caused by the disease. Classification is done by applying several machine learning methods consisting of C.45, DT, KNN, RF, and SVM, where the evaluation is carried out using cross validation. The following sections structure the paper: section 2 describes the dataset and methods used, section 3 presents the result and discussion for each classification method based on the performance evaluation, and section 4 concludes the paper.
ISSN: 2252 8938 Int J Artif Intell, Vol. 11, No. 3, September 2022: 1119 1129 1120 Dengue fever is an arboviral disease caused by infection with one of the four dengue virus serotypes. It is spread through contact with the dengue (DENV) virus. According to the World Health Organization (WHO), this disease is estimated to have a global burden of 50 million illnesses annually, and about 2.5 billion people worldwide live in dengue endemic areas [18]. A person can develop dengue fever with various symptoms, such as headache, muscle aches, fever, and a measles like rash, also known as fracture fever [16]. Regarding statistical data in varied countries, several dengue endemic cases were reported in Saudi Arabia, especially in the western and southern provinces of the Jeddah and Mecca areas, the first in 2011, when 2,569 cases were reported, and the second, in 2013 when 4,411 cases including 8 deaths were reported. Dengue has also occurred in other areas of Saudi Arabia, including Medina (2009) and Aseer and Jizan (2013) [18]. Meanwhile, the Malaysian Ministry of Health reports that dengue fever has grown rapidly since 2012. In 2015, the Malaysian Ministry of Health published a report recording 107,079 cases of dengue fever with 293 deaths, while there were 43,000 cases of dengue fever with 92 deaths in 2013 [19]. The rapid spread of the dengue virus has become more and more dangerous, and addressing this issue should be considered an urgent case. Additionally, the national incidence of dengue hemorrhagic fever (DHF) in Indonesia increased from 50.8 per 100,000 population in 2015 to 78.9 per 100,000 population in 2016 [20]. The clinical diagnosis can range from symptomatic dengue fever (DF) to a more severe form known as DHF, and the most fatal is dengue shock syndrome (DSS) [18] Classification of dengue varieties has been carried out using a computer based system. The input data are symptoms suffered by patients such as fever, headache, pain behind the eyeball, joint pain, muscle pain, and other symptoms. In addition, the thrombocyte and hemoglobin values of the patient are also indicative of dengue disease. The classification system is needed to immediately find out the symptoms suffered by the patient without convening the expert or doctor. It can be applied using several methods, such as rule based [21] [24], or using machine learning [25]. The following methods were used in prior studies to implement the machine learning based classification process: naive Bayes [6], logistic regression [12], random forest (RF) [8], [12], [16], k nearest neighbor (KNN) [26], artificial neural network (ANN) [10], [13], dan support vector machine (SVM) [8], [14]
Int J Artif Intell ISSN: 2252 8938 Dengue classification method using support vector machines and cross validation (Hamdani Hamdani) 1121
Thrombocyte: 43.000, Hemoglobin: 49 DHF KP004 10 Female Fever, headache, pain behind the eyes, muscle aches, petechiae, bleeding manifestasions, shock, anxious, vomitting, diarrhea, abdominal pain, red eyes. Thrombocyte: 4.000, Hemoglobin: 20 DSS KP005 16 Female Fever, headache, pain behind the eyes, joint pain, petechiae, bleeding manifestasions, vomitting, diarrhea, abdominal pain, red eyes, jaw pain. Thrombocyte: 102.000, Hemoglobin: 41 DHF KP006 3 Male Fever, headache, pain behind the eyes, joint pain, skin rash, vomitting, cough, red eyes. Thrombocyte: 27.000, Hemoglobin: 32 DSS ⋮ ⋮ ⋮ ⋮ ⋮ KP106 9
Male Fever, headache, joint pain, muscle aches, skin rash, petechiae, bleeding manifestasions, shock, anxious, vomitting, sore throat, cough. Thrombocyte: 77.000, Hemoglobin: 34 DSS KP110 20
Pcode Age Gender Symptoms Diagnose KP001 7 Male Fever, headache, pain behind the eyes, skin rash, cough, vomitting, sore throat.
2. MATERIALS AND METHODS
Table 1 The examples of several dengue patient data
Female Fever, headache, pain behind the eyes, joint pain, nausea, red eyes. Thrombocyte: 166.000, Hemoglobin: 38 DHF KP107 2 Male Fever, headache, pain behind the eyes, joint pain, skin rash, vomitting, red eyes Thrombocyte: 120.000, Hemoglobin: 32 DF KP108 47
This section describes the dataset details used and the classification method. It also provides information on the process for evaluating the performance of each classification method. In this study, the dataset provided by Dirgahayu Hospital, Samarinda, Indonesia, consisted of 110 cases of dengue patients. The dataset is divided into three classes, including DF, DHF, and DSS, comprising 40 data, 61 data, and 9 data, respectively. The data was collected in the form of patient code (Pcode), age, and symptoms experienced, including thrombocyte and hemoglobin values from each patient and the results of the diagnosis obtained from the expert. The symptoms experienced by each patient may vary, so the results of the diagnosis of dengue type from the expert vary. The example of several data collected from the patients is shown in Table 1. This study consists of two stages: training and testing. Both of them have two main processes are pre processing and classification. Additionally, there is an evaluation method process required to measure each classifier’s performance. The input of the evaluation method is the diagnosis resulting from the expert (actual class) and classification method (predicted class). The overview of the dengue classification method is depicted in Figure 1.
Thrombocyte: 95.000, Hemoglobin: 32 DF KP002 8 Male Fever, pain behind the eyes, joint pain, skin rash, red eyes, vomitting, cough. Thrombocyte: 97.000, Hemoglobin: 39 DF KP003 17 Female Fever, headache, pain behind the eyes, muscle aches, skin rash, petechiae, bleeding manifestasions, vomitting, diarrhea, abdominal pain, red eyes, jaw pain.
Female Fever, headache, muscle aches, skin rash, petechiae, bleeding manifestasions, vomitting, diarrhea, abdominal pain, jaw pain, inflammation. Thrombocyte: 63.000, Hemoglobin: 47 DHF KP109 9
Female Fever, headache, joint pain, muscle aches, skin rash, petechiae, bleeding manifestasions, shock, anxious, vomitting, diarrhea, abdominal pain, cough, sore throat Thrombocyte: 32.000, Hemoglobin: 30 DSS
Figure 1. The overview of the processes on the dengue classification method
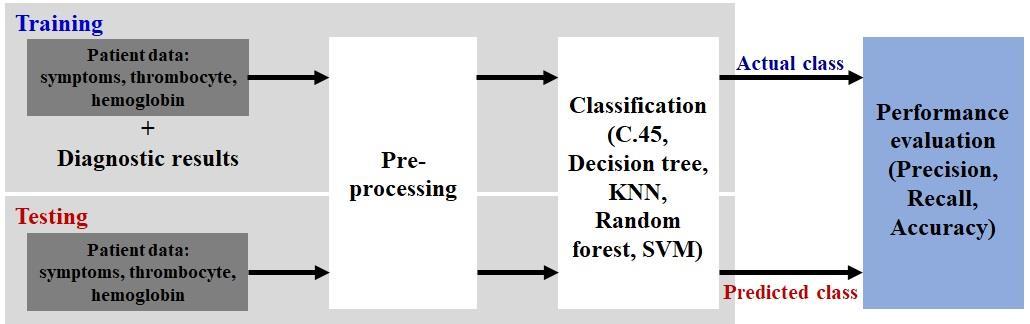
2.2.1. K nearest neighbor (KNN) KNN is a supervised machine learning algorithm that can address classification and regression issues [24]. The vast majority of neighbors are considered input data. The KNN method must be performed numerous times with various K values in order to find the K that minimizes errors while maintaining prediction accuracy. With n number of data, a brute force search technique is implemented using the Euclidean distance function for the nearest neighbor search as in (1), where xi and yi are the testing and training data to i, respectively.
2.2.2. Random forest (RF) RF is a file producing machine learning method that is flexible and simple to use. Even without hyperparameter tweaking, a superb outcome will produce most of the time [8]. The RF is one of the most extensively used algorithms due to its simplicity and diversity. A RF is a group of trees that combines each decision tree (DT) based on a set of random variables. The DT is a vectorized flowchart [12]. For dimension ��, the predictor variables are represented by the random vector ��=(��1, ��2, …, ����) ��, while a random variable y represents the real value response. Figure 2 is an illustration of the structure of a RF
The classification process is carried out using a machine learning approach. Machine learning is an artificial intelligence (AI) area that contains techniques that allow computers to learn from empirical data, such as sensor data databases [7]. There are five classification methods implemented in this study, consisting of C.45, DT, KNN, RF, and SVM. Those classification method has been successfully implemented in several previous studies [6], [8], [16]. Classification is done using a cross validation technique with k fold 3, 5, and 10 to distribute training and testing data [6]. An explanation regarding each classification method used is explained in the following sub section.
The majority of RF parameters are based on two data objects. One to a third of the instances is not counted in the sample used to obtain unbiased data, notably from the batch or OOB, which estimates the classification error and the significance of the variable when substituting the sample for the current tree when producing the training set. The tree then processes all data for each case pair, and the closeness is calculated.
�� = √∑ (���� ����) ��2 ��=1 (1)
ISSN: 2252 8938 Int J Artif Intell, Vol. 11, No. 3, September 2022: 1119 1129 1122 2.1. Pre-processing Discretization was used to accomplish pre processing. The data in Table 1 had to be converted into numerical data to be utilized as input into the classification process. The patient’s data included 18 different kinds of dengue disease symptoms (S), including fever (S1), headache (S2), joint pain (S3), muscle soreness (S4), maculopapular skin rash (S5), and petechiae (S6) (S4). S6), bruising (S7), shock (S8), anxiety (S9), vomiting (S10), constipation (S11), diarrhea (S12), heartburn (S13), red eyes (S14), lower jaw discomfort (S15), cough (S16), sore throat (S17), and nasal cavity inflammation (S18) and thrombocyte (T) and hemoglobin (H) values. Therefore, there are 20 attributes that become input for the following process, namely classification. The symptom data obtained from the patients in Table 1 are not numerical type so that in this process, each symptom experienced by the patient is given a value of 1. In contrast, if the patient does not experience these symptoms, it is given a value of 0. Meanwhile, the data on platelets and hemoglobin do not need to be pre processed. Based on the pre processing, the data obtained are ready to be used in the classification process. The pre processing data in this study are shown in Table 2. Table 2 The resulting of pre processing Patient S1 S2 S3 S4 S5 S6 S7 S13 S14 S15 S16 S117 S18 T H Diagnose P1 1 1 1 0 0 1 0 0 0 0 0 1 1 9500 32 DF P2 1 0 1 1 0 1 0 0 0 1 0 1 0 97000 39 DF P3 1 1 1 0 1 1 1 1 1 1 1 0 0 43000 49 DHF P4 1 1 1 1 1 1 1 0 0 0 0 1 1 4000 20 DHF P5 1 1 1 0 1 1 1 0 0 1 0 0 0 102000 41 DSS P6 1 1 1 1 0 0 0 1 1 1 1 0 0 27000 32 DHF ⋮ ⋮ ⋮ ⋮ ⋮ ⋮ ⋮ ⋮ ⋮ ⋮ ⋮ ⋮ ⋮ ⋮ ⋮ ⋮ ⋮ ⋮ P106 1 0 1 0 0 1 1 0 0 1 0 1 0 166000 38 DHF P107 1 0 0 1 1 1 0 1 1 0 0 1 0 120000 32 DF P108 1 1 1 1 1 1 0 0 0 1 0 0 0 63000 47 DHF P109 1 1 1 1 0 1 1 0 0 1 0 1 0 77000 34 DSS P110 1 0 1 0 1 1 0 1 1 0 0 1 0 32000 30 DSS
2.2. Classification
2.2.4. Decision tree (DT)
SVM is a supervised learning method for classification and regression. The primary purpose of SVM is to classify results by mapping data between input vectors and a large viewpoint space. As a result, linear SVM seeks to fully use the distance between the decision hyperplane and the marginal distance, the closest data point [27]. This study used linear SVM defined in (2), where {���� and ��j} is the dataset. ��,����)=[����]������ (2)
��(��
Dengue classification method using support vector machines and cross validation (Hamdani Hamdani) 1123
ii) Classification procedure: to classify a new instance that only has the values of all of its attributes. It is conducted by starting at the root of the built tree, taking the path that corresponds to the observed attribute value in the inner tree node. This technique is repeated until the leaf is found. Finally, we use a bound label to determine a specific instance's anticipated class value.
Int J Artif Intell ISSN: 2252 8938
A DT is a hierarchical structure that resembles a block diagram and comprises three essential elements: decision nodes that correspond to attributes, edges, or branches that correspond to multiple possible attribute values [28]. The leaf component is the third component, and it contains items that are usually of the same type or are quite similar. This view enables us to define decision rules for classifying new instances. In reality, each path from the root to a leaf corresponds to a conjunction of the test qualities, and the tree is thought of as a substitute for these conjunctions. The building (induction) and classification (inference) processes form the majority of DTs [28]:
i) Build procedure: to set the training data. A DT is typically formed for a given training set by starting with an empty tree and using the attribute selection measure to select a "suitable" test attribute for each decision node. The rule is to pick an attribute that reduces class confusion between each test generated training subset, making it easier to define the object's classes. The process is repeated for each sub decision tree until the desired foliage is attained and the grades are approved.
Figure 2. The illustration of the structure of a RF 2.2.3 C.45 decision tree (DT) Most studies employ the C.45 decision tree, which is a comprehensive method of machine learning. C45 is typically used to generate a classification tree based on a hierarchical tree system, with attributes and leaf nodes illustrating the solution findings. The C45 approach’s visual categorization is successful and efficient. C45, on the other hand, is prone to data noise. Regression tree (CART), automatic chi square interaction detector (CHAID), ID3, and C4.5 are some DT techniques employed. As a result, C.45 was used as one of the ways to improve classification accuracy in this investigation [6]
2.2.5. Support vector machine (SVM)
While the distance between two enclosures grows by one, they occupy the same end node. At the end of the run, the number of split trees is used to conduct proximity normalization. The detection of outliers is based on proximity, data substitution, and highlight. Outlier detection uses proximity as well as missing data replacement and highlighting to obtain low dimensional representations of data [8]
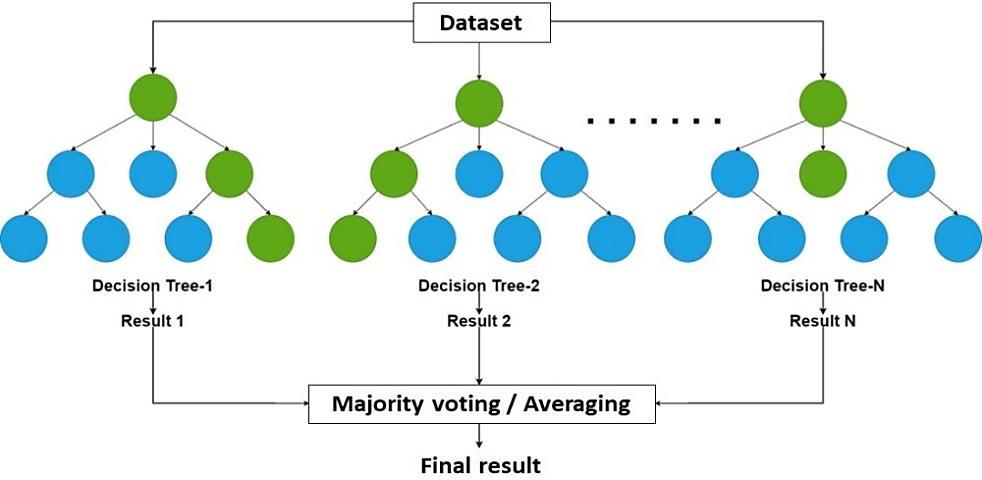
Figure 3. Confusion matrix multi class 3. RESULTS AND DISCUSSION Pre processing was done by discretization, which aims to convert all patient data into a numeric type. Based on the data collected from dengue patients, there were 18 symptoms (S1 S18) and values for thrombocyte (T) and hemoglobin (H). Hence, a total of 20 attributes were used as input data for the following process, namely classification. Symptom data is given a value of 1 if the patient experiences it. Otherwise, it is worth 0 if the patient does not experience it. The dengue diagnosis results are divided into three classes, namely: DF, DHF, and DSS. In the classification process, five methods were applied, consisting of C.45, DT, KNN, RF, and SVM. These were done to obtain the most optimal method performance, which was measured using three parameters, namely precision, recall, and accuracy. This value is obtained based on a multiclass confusion matrix using a cross validation technique with three different k fold values, namely 3, 5, and 10. A comparison of the performance of the five classification methods obtained with the barbed k fold value is summarized in Table 3.
ISSN: 2252 8938 Int J Artif Intell, Vol. 11, No. 3, September 2022: 1119 1129 1124
������������������ = ∑ ������ �� ��=1 ������ ×100, (3) ������������ = ∑ ������ �� ��=1 ������ ×100, (4) ���������������� = ∑ ������ �� ��=1 ∑ ∑ ������ �� ��=1 �� ��=1 ×100 (5)
Table 3 shows KNN with k fold 3 yielded the lowest performance indicated by precision, recall, and accuracy values of 93.9%, 93.6%, and 93.6%, respectively. At the same time, the three parameters achieve a value of 94.8%, 94.5%, and 94.5% for k fold 5 and 10. RF with k fold 10 and SVM with k fold 3 and 10 respectively had the maximum performance of the classification method with precision, recall, and accuracy of 99.1%, 99.1%, and 99.1%, respectively.
Performance evaluation was conducted against the classification method using three measures parameters: precision, recall, and accuracy [12] based on the confusion matrix multiclass. The value of evaluation parameters is in the range of 0 to 100. The method indicates high performance if those parameters are close to 100. Those parameters are defined [29]:
A confusion matrix is a machine learning concept that stores information about a classification system’s actual and expected classifications. A confusion matrix contains two dimensions: indexed by the actual item class and the classifier predicts class. The fundamental structure of a confusion matrix for multi class classification problems is shown in Figure 3, with the classes A1, A2, and An The number of samples belonging to class Ai but identified as class Aj [29] in the confusion matrix is represented by Nij. Figure 3 shows a multi class confusion matrix with n number of classes. The dengue patient dataset is divided into k subsets for the type of diagnosis data. In general, the data (k 1)/k is used for training, and the data 1/k is used for the testing. Then, the process is reiterated k times. The validation result of mean k time is selected as the last rate estimation as a final point. In this study, the performance is measured using cross validation with the k fold value of 3, 5, and 10.
2.3. Performance evaluation
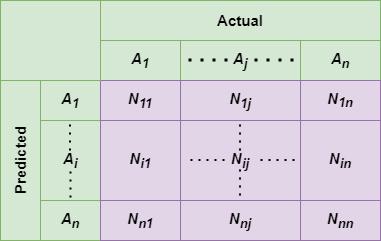
Figure 4. Confusion matrix of C.45 classifier with (a) k fold 3 and (b) k fold 5 and 10
Dengue classification method using support vector machines and cross validation (Hamdani Hamdani) 1125
The comprehensive study results of each classifier present in Figures 4 8. Figure 4(a) depicts the confusion matrix of the C.45 classifier for k folds 3, while Figure 4(b) depicts the confusion matrix for k folds 5 and 10. Figure 4(a) shows the implementation of k fold 3 leads to misclassification of 3 data in the DF class, which is classified as DHF class, and 3 data in the DHF class classified as DF class. The classification results using a DT with k fold 3, 5, and 10 are shown in Figures 5(a) (c). Figures 5(a) and 5(c) show that errors also occur in the DF and DHF classes. While using k fold 5 as shown in Figure 5(b), errors only occur in the DHF class that is classified as DF class as much as 3 data. Moreover, the results of the application of KNN with k fold 3, 5, and 10 as shown in Figure 6. It indicates misclassification occurs in DF and DHF classes using k fold 3 as presented in Figure 6(a), but errors occur in all classes using k fold 5 and 10 as shown in Figures 6(b) and 6(c). Furthermore, the application of RF with k fold 3 shows that misclassification also occurs in all classes, as depicted in Figure 7(a). Meanwhile, misclassification occurs in DF and DHF classes with k fold 5 as shown in Figure 7(b). In comparison, misclassification occurs only in 1 data in DHF class, classified as DF class with k fold 10 as shown in Figure 7(c). Furthermore, Figure 8 presents the classification results using SVM with k fold 3, and 10 as shown in Figure 8(a) shows only 1 data in the DHF class misclassified as DF class. Meanwhile, in k fold 5 as shown in Figure 8(b) there are 2 data, namely 1 data on classes DF and DHF are misclassified. Overall, Figures 4 8 show that misclassification often occurs in the DHF class, which is classified as the DF class. (a) (b)
As seen in Table 3, the k fold value has an effect on the precision, recall, and accuracy values. K fold 5 and 10 implemented in the C.45 classification method and the optimal performance KNN were created, respectively, with 97.3% and 94.5% accuracy values. Meanwhile, the DT with the best accuracy value, 97.3%, was generated with k fold 5, while RF with k fold 10 and SVM with k fold 3 and 10 achieved the highest accuracy of 99.1%. Overall, k fold 10 yields the best results for each classifier, with the exception of the DT, which yields the best results at k fold 5. Table 3 represents the number of successfully classified and incorrectly classified data for each method.
Table 3. Dengue classification results using various classifiers and k fold Classifier K fold Evaluation parameter (%) Precision Recall Accuracy C.45 3 94 5 94 5 95 5 5 97 5 97 3 97.3 10 97 5 97 3 97.3 DT 3 94 5 94 5 95 5 5 97 5 97 3 97.3 10 95 5 95 5 95 5 KNN 3 93 9 93 6 93 6 5 94 8 94 5 94 5 10 94 7 94 5 94 5 RF 3 96 4 96 4 96.4 5 98 2 98 2 98.2 10 99 1 99 1 99.1 SVM 3 99 1 99 1 99.1 5 98 2 98 2 98.2 10 99 1 99 1 99.1
Int J Artif Intell ISSN: 2252 8938
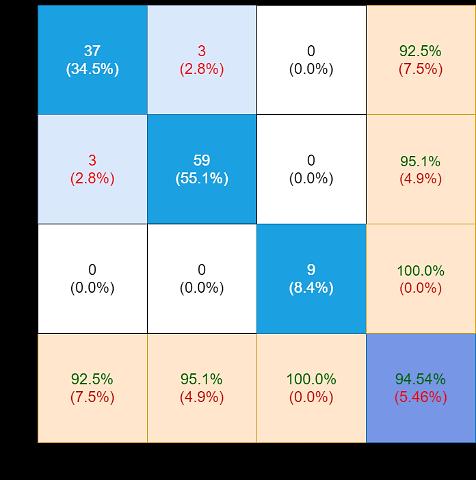
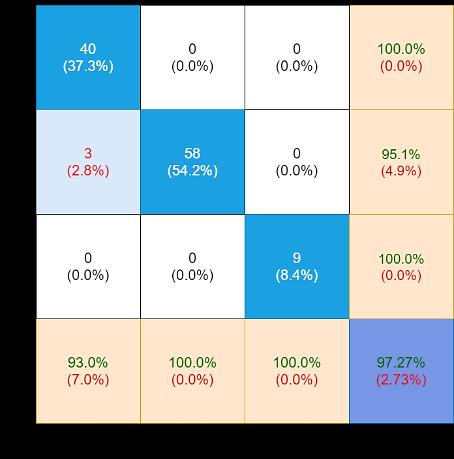
(c)
ISSN: 2252 8938 Int J Artif Intell, Vol. 11, No. 3, September 2022: 1119 1129 1126 (a) (b) (c)
Figure 5 Confusion matrix of DT classifier with (a) k fold 3, (b) k fold 5, and (c) k fold 10 (a) (b)
Figure 6 Confusion matrix of KNN classifier with (a) k fold 3, (b) k fold 5, and (c) k fold 10
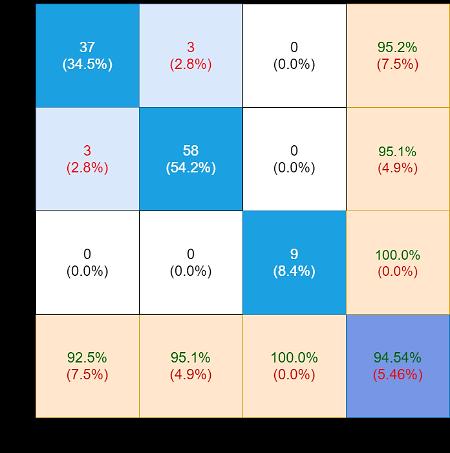
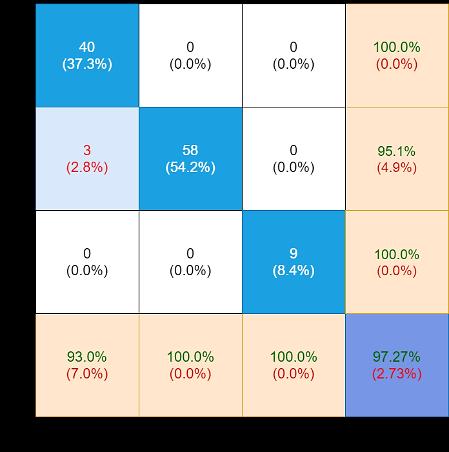
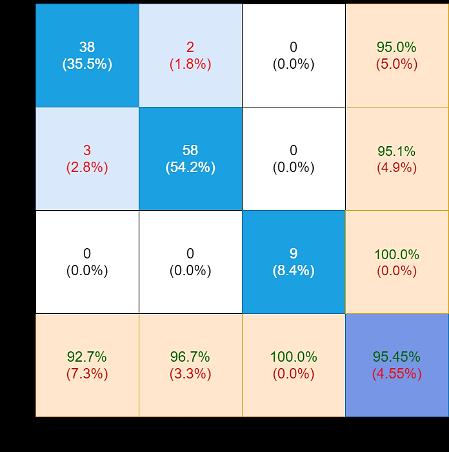
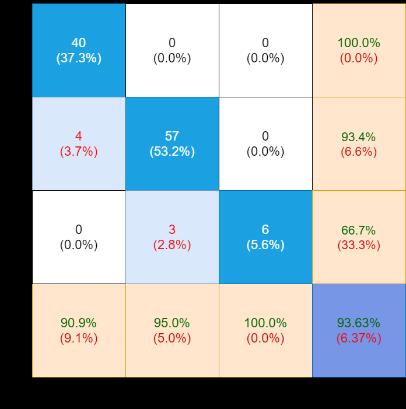
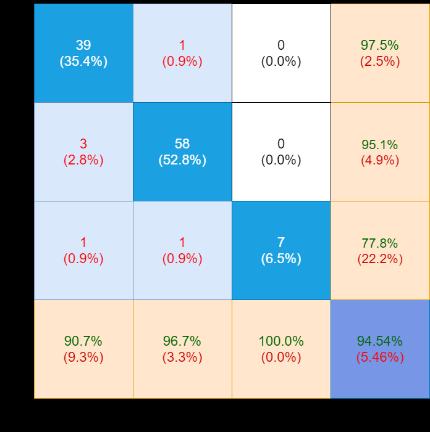
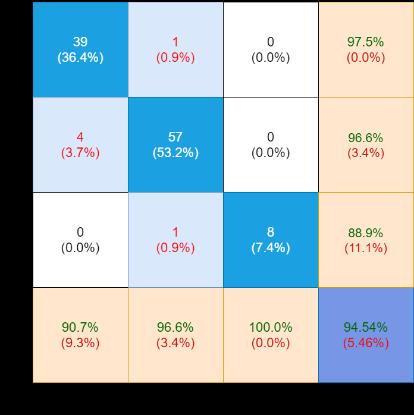
Int J Artif Intell ISSN: 2252 8938
(c)
Dengue is a dangerous disease that can cause death with different symptoms experienced by patients. There are three varieties of dengue: DF, DHF, and DSS. This disease needs to be detected early to get the appropriate treatment. Classification of dengue types can be done using a machine learning approach.
The evaluation was conducted using cross validation with barbed k fold values of 3, 5, and 10 for each classifier. The evaluation results show that the performance of SVM with k fold 3 and 10 managed to achieve the highest accuracy value of 99.1%. Meanwhile, the RF also achieved an accuracy of 99.1% but only at k fold 10, with errors only occurring in 1 data in the DHF class classified as DF class. It shows the k fold value can affect the classification results. Although the accuracy obtained is lower, other classifiers, namely C.45, KNN, and DT, have achieved more than 90% accuracy. This method is expected to be used for datasets
4. CONCLUSION
Five classifiers were used, consisting of C.45, DT, KNN, RF, and SVM. The input data for each classifier is 20 attributes consisting of 18 symptoms experienced by patients and the values of platelets and hemoglobin.
Dengue classification method using support vector machines and cross validation (Hamdani Hamdani) (b)
Figure 7 Confusion matrix of RF classifier with (a) k fold 3, (b) k fold 5, and (c) k fold 10 (a) (b)
Figure 8. Confusion matrix of SVM classifier with (a) k fold 3 and 10 and (b) k fold 5
1127 (a)
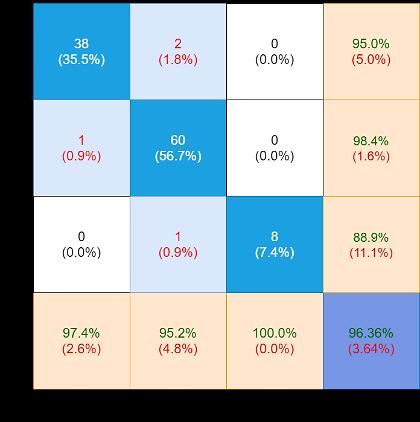
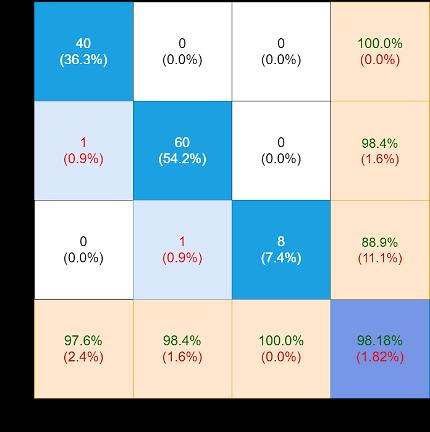
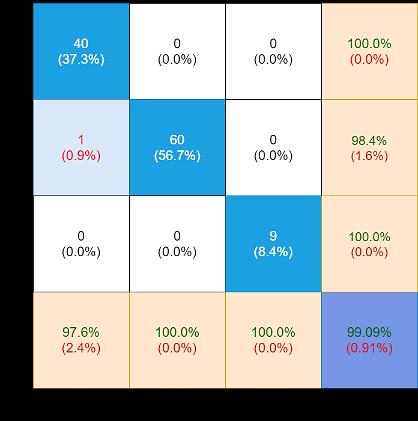
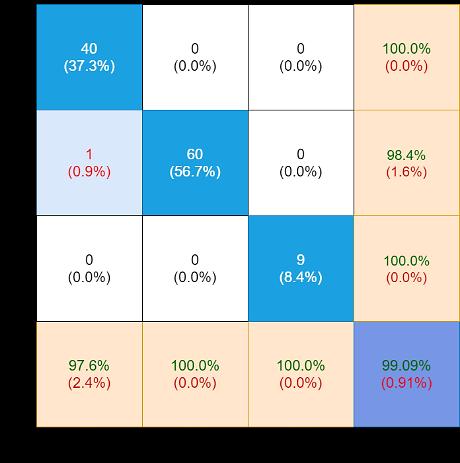
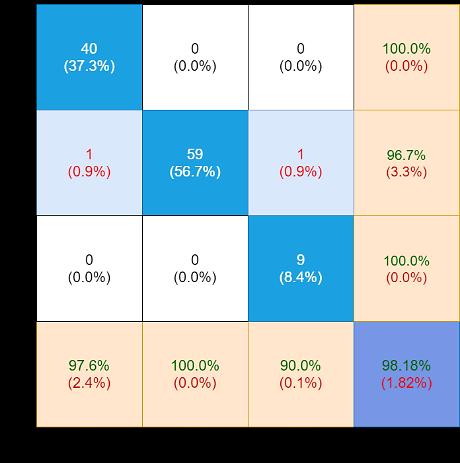
[22] R. Sicilia, M. Merone, R. Valenti, and P. Soda, “Rule based space characterization for rumour detection in health,” Engineering Applications of Artificial Intelligence,vol. 105, Art. no. 104389, Oct. 2021, doi: 10.1016/j.engappai.2021.104389.
[15] S. Adak and S. Jana, “A model to assess dengue using type 2 fuzzy inference system,” Biomedical Signal Processing and Control, vol. 63, Art. no. 102121, Jan. 2021, doi: 10.1016/j.bspc.2020.102121.
[4] Z. Rustam, A. Purwanto, S. Hartini, and G. S. Saragih, “Lung cancer classification using fuzzy c means and fuzzy kernel C Means based on CT scan image,” IAES International Journal of Artificial Intelligence (IJ AI), vol. 10, no. 2, pp. 291 297, Jun. 2021, doi: 10.11591/ijai.v10.i2.pp291 297. [5] S. R. Ashwini and H. C. Nagaraj, “Classification of EEG signal using EACA based approach at SSVEP BCI,” IAES International Journal of Artificial Intelligence (IJ AI), vol. 10, no. 3, pp. 717 726, Sep. 2021, doi: 10.11591/ijai.v10.i3.pp717 726.
[20] R. T. Sasmono et al., “Molecular epidemiology of dengue in North Kalimantan, a province with the highest incidence rates in Indonesia in 2019,” Infection, Genetics and Evolution, vol. 95, Art. no. 105036, Nov. 2021, doi: 10.1016/j.meegid.2021.105036.
[18] B. A. Ajlan, M. M. Alafif, M. M. Alawi, N. A. Akbar, E. K. Aldigs, and T. A. Madani, “Assessment of the new World Health Organization’s dengue classification for predicting severity of illness and level of healthcare required,” PLOS Neglected Tropical Diseases, vol. 13, no. 8, Art. no. e0007144, Aug. 2019, doi: 10.1371/journal.pntd.0007144.
[23] S. Shishehchi and S. Y. Banihashem, “A rule based expert system based on ontology for diagnosis of ITP disease,” Smart Health, vol. 21, Art. no. 100192, Jul. 2021, doi: 10.1016/j.smhl.2021.100192.
[17] W. Hoyos, J. Aguilar, and M. Toro, “Dengue models based on machine learning techniques: A systematic literature review,” Artificial Intelligence in Medicine, vol. 119, Art. no. 102157, Sep. 2021, doi: 10.1016/j.artmed.2021.102157.
[1] A. Saibene, M. Assale, and M. Giltri, “Expert systems: Definitions, advantages and issues in medical field applications,” Expert Systems with Applications, vol. 177, Art. no. 114900, Sep. 2021, doi: 10.1016/j.eswa.2021.114900.
[24] A. Septiarini, R. Pulungan, A. Harjoko, and R. Ekantini, “Peripapillary atrophy detection in fundus images based on sectors with scan lines approach,” in 2018 Third International Conference on Informatics and Computing (ICIC), Oct. 2018, pp. 1 6, doi: 10.1109/IAC.2018.8780490.
[25] I. Tougui, A. Jilbab, and J. El Mhamdi, “Impact of the choice of cross validation techniques on the results of machine learning based
[2] A. Septiarini, A. Harjoko, R. Pulungan, and R. Ekantini, “Automated detection of retinal nerve fiber layer by texture based analysis for glaucoma evaluation,” Healthcare Informatics Research, vol. 24, no. 4, pp. 335 345, 2018, doi: 10.4258/hir.2018.24.4.335.
[10] A. Septiarini, A. Harjoko, R. Pulungan, and R. Ekantini, “Automatic detection of peripapillary atrophy in retinal fundus images using statistical features,” Biomedical Signal Processing and Control, vol. 45, pp. 151 159, Aug. 2018, doi: 10.1016/j.bspc.2018.05.028.
[12] Z. Rustam, F. Zhafarina, G. S. Saragih, and S. Hartini, “Pancreatic cancer classification using logistic regression and random forest,” IAES International Journal of Artificial Intelligence (IJ AI), vol. 10, no. 2, pp. 476 481, Jun. 2021, doi: 10.11591/ijai.v10.i2.pp476 481.
ISSN: 2252 8938 Int J Artif Intell, Vol. 11, No. 3, September 2022: 1119 1129 1128witha larger amount of data for further study. Therefore, it can increase the value of accuracy and is better for predicting or classifying other diseases.
REFERENCES
ACKNOWLEDGEMENTS
[11] S. Abhari, S. R. Niakan Kalhori, M. Ebrahimi, H. Hasannejadasl, and A. Garavand, “Artificial intelligence applications in type 2 diabetes mellitus care: focus on machine learning methods,” Healthcare Informatics Research, vol. 25, no. 4, pp. 248 261, 2019, doi: 10.4258/hir.2019.25.4.248.
[16] R. Gangula, L. Thirupathi, R. Parupati, K. Sreeveda, and S. Gattoju, “Ensemble machine learning based prediction of dengue disease with performance and accuracy elevation patterns,” Materials Today: Proceedings, Jul. 2021, doi: 10.1016/j.matpr.2021.07.270.
[19] H. Abid, M. Malik, F. Abid, M. R. Wahiddin, N. Mahmood, and I. Memon, “Global health action nature of complex network of dengue epidemic as scale free network,” Healthcare Informatics Research, vol. 25, no. 3, pp. 182 192, 2018.
[21] M. N. Kamel Boulos, “Expert system shells for rapid clinical decision support module development: An ESTA demonstration of a simple rule based system for the diagnosis of vaginal discharge,” Healthcare Informatics Research, vol. 18, no. 4, Art. no. 252, 2012, doi: 10.4258/hir.2012.18.4.252.
[6] N. A. Mashudi, N. Ahmad, and N. M. Noor, “Classification of adult autistic spectrum disorder using machine learning approach,” IAES International Journal of Artificial Intelligence (IJ AI), vol. 10, no. 3, pp. 743 751, Sep. 2021, doi: 10.11591/ijai.v10.i3.pp743 751. [7] G. Feretzakis et al., “Machine learning for antibiotic resistance prediction: a prototype using off the shelf techniques and entry level data to guide empiric antimicrobial therapy,” Healthcare Informatics Research, vol. 27, no. 3, pp. 214 221, Jul. 2021, doi: 10.4258/hir.2021.27.3.214. [8] J. E. Aurelia, Z. Rustam, I. Wirasati, S. Hartini, and G. S. Saragih, “Hepatitis classification using support vector machines and random forest,” IAES International Journal of Artificial Intelligence (IJ AI), vol. 10, no. 2, pp. 446 451, Jun. 2021, doi: 10.11591/ijai.v10.i2.pp446 451. [9] U. L. Mohite and H. G. Patel, “Optimization assisted Kalman filter for cancer chemotherapy dosage estimation,” Artificial Intelligence in Medicine, vol. 119, Art. no. 102152, Sep. 2021, doi: 10.1016/j.artmed.2021.102152.
The author would like to thank the Faculty of Engineering, Mulawarman University, Samarinda, Indonesia, for funding the research in 2021.
[3] A. K. Al Khowarizmi and S. Suherman, “Classification of skin cancer images by applying simple evolving connectionist system,” IAES International Journal of Artificial Intelligence (IJ AI), vol. 10, no. 2, pp. 421 429, Jun. 2021, doi: 10.11591/ijai.v10.i2.pp421 429.
[13] J. J. Patel and S. K. Hadia, “An enhancement of mammogram images for breast cancer classification using artificial neural networks,” IAES International Journal of Artificial Intelligence (IJ AI), vol. 10, no. 2, pp. 332 345, Jun. 2021, doi: 10.11591/ijai.v10.i2.pp332 345. [14] A. Septiarini, H. Hamdani, and D. M. Khairina, “The contour extraction of cup in fundus images for glaucoma detection,” International Journal of Electrical and Computer Engineering (IJECE), vol. 6, no. 6, pp. 2797 2804, Dec. 2016, doi: 10.11591/ijece.v6i6.pp2797 2804.
BIOGRAPHIES OF AUTHORS
Novianti Puspitasari is a lecturer at the Faculty of Engineering, Universitas Mulawarman, Indonesia. She completed her Master in Computer Science from Universitas Gajah Mada, Yogyakarta, Indonesia. She is a member of the Institute of Electrical and Electronics Engineers (IEEE), Indonesian Computer, Electronics, Instrumentation Support Society (IndoCEISS), and Association of Computing and Informatics Institutions Indonesia (APTIKOM) societies. Her research interest is in data science and analytics, artificial intelligence, machine learning areas. Email: novipuspitasari@unmul.ac.id
Anindita Septiarini is a lecturer and researcher since 2009 at Mulawarman University, Indonesia. Her research interests lie in the field of artificial intelligence, especially pattern recognition, image processing and computer vision. She received her bachelor’s degree in 2005 from Surabaya University, Indonesia, her master’s degree in 2009 from Gadjah Mada University, Indonesia, and her doctoral degree in computer science in 2017 from Gadjah Mada University Indonesia. She can be contacted at email: anindita@unmul.ac.id. Henderi is an Associate Professor in Computer Science focusing on research about data warehousing and data mining. Decision support system and information system. He has received a Ph.D. (Computer Science) from Universitas Gadjah Mada, Yogyakarta, Indonesia, and M. Comp. (Computer engineering) from STTI Benarif Indonesia, Jakarta. His area of expertise is Business Intelligence, Data Warehousing, and Data Mining, Decision Support Systems, and Information System Development. He can be contacted at email: Sinta:henderi@raharja.infohttps://sinta.kemdikbud.go.id/authors/detail?id=5974125&view=overview.
Int J Artif Intell ISSN: 2252 8938 Dengue classification method using support vector machines and cross validation (Hamdani Hamdani) 1129 diagnostic applications,” Healthcare Informatics Research, vol. 27, no. 3, pp. 189 199, Jul. 2021, doi: 10.4258/hir.2021.27.3.189.
[26] A. Septiarini, D. M. Khairina, A. H. Kridalaksana, and H. Hamdani, “Automatic glaucoma detection method applying a statistical approach to fundus images,” Healthcare Informatics Research, vol. 24, no. 1, pp. 53 60, 2018, doi: 10.4258/hir.2018.24.1.53.
[27] T. R. Baitharu, K. P. Subhendu, and S. K. Dhal, “Comparison of kernel selection for support vector machines using diabetes dataset,” Journal of Computer Sciences and Applications, vol. 3, no. 6, pp. 181 184, 2016.
[28] I. Jenhani, N. Ben Amor, and Z. Elouedi, “Decision trees as possibilistic classifiers,” International Journal of Approximate Reasoning, vol. 48, no. 3, pp. 784 807, Aug. 2008, doi: 10.1016/j.ijar.2007.12.002.
Hamdani Hamdani is a lecturer and researcher since 2005 at Mulawarman University, Indonesia. Her research interests lie in the field of artificial intelligence, especially decision support system and expert system. He received her bachelor’s degree in 2002 from Ahmad Dahlan University, Indonesia, his master’s degree in 2009 from Gadjah Mada University, Indonesia, and her doctoral degree in computer science in 2018 from Gadjah Mada University Indonesia. He can be contacted at email: hamdani@unmul.ac.id. Heliza Rahmania Hatta received a Bachelor of Computer Science from Mulawarman University, Indonesia. Master of Informatics Engineering from Institut Teknologi Sepuluh Nopember, Surabaya, Indonesia. Currently, she is a lecturer at Department of Informatics in Mulawarman University, Samarinda, East Kalimantan, Indonesia. Her research areas of interest are artificial intelligence, decision support systems, expert system, information system, and information technology. She can be contacted at Email: heliza_rahmania@yahoo.com
[29] X. Deng, Q. Liu, Y. Deng, and S. Mahadevan, “An improved method to construct basic probability assignment based on the confusion matrix for classification problem,” Information Sciences, vol. 340 341, pp. 250 261, May 2016, doi: 10.1016/j.ins.2016.01.033
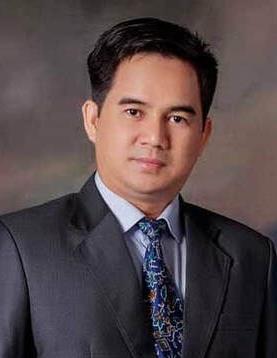




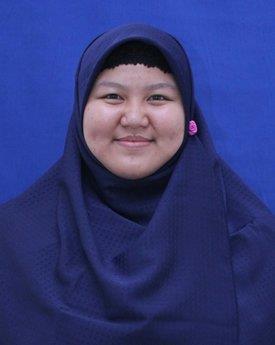




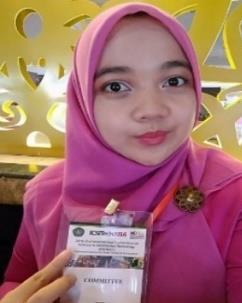




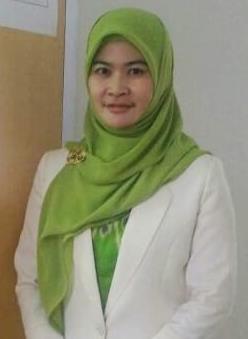




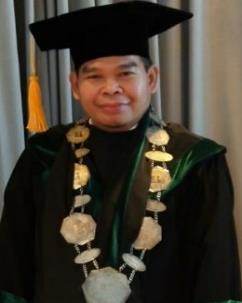




Keywords: Data SaLinearJobstreetvisualizationMalaysiaregressionlaryprediction
2Faculty of Computer and Mathematical Sciences, Universiti Teknologi MARA Shah Alam, Selangor, Malaysia
3Department of Computer Science Education, Universitas Pendidikan Indonesia, Bandung, Indonesia
This study explicitly discusses helping job seekers predict salaries and visualize job vacancies related to their future careers. Jobstreet Malaysia is an ideal platform for discovering jobs across the country. However, it is challenging to identify these jobs, which are organized according to their respective and specific courses. Therefore, the linear regression approach and visualization techniques were applied to overcome the problem. This approach can provide predicted salaries, which is useful as this enables job seekers to choose jobs more easily based on their salary expectations. The extracted Jobstreet data runs the pre processing, develops the model, and runs on real world data. A web based dashboard presents the visualization of the extracted data. This helps job seekers to gain a thorough overview of their desired employment field and compare the salaries offered. The system’s reliability as tested using mean absolute error, the functionality test was performed according to the use case description, and the usability test was performed using the system usability scale. The reliability results indicate a positive correlation with the actual values. The functionality test produced a successful result, and a score of 96.58% was achieved for the system usability scale result, proving the system grade is ‘A’ and usable
Article history: Received Nov 9, 2021 Revised Jun 14, 2022 Accepted Jun 21, 2022
Article Info ABSTRACT
Khyrina Airin Fariza Abu Samah1 , Nurqueen Sayang Dinnie Wirakarnain1 , Raseeda Hamzah2 , Nor Aiza Moketar1 , Lala Septem Riza3, Zainab Othman1
This is an open access article under the CC BY SA license. Corresponding Author: Khyrina Airin Fariza Abu Samah Faculty of Computer and Mathematical Sciences, Universiti Teknologi MARA Cawangan Melaka Kampus Merlimau,Jasin 77300, Melaka, Malaysia Email: khyrina783@uitm.edu.my 1. INTRODUCTION
Journal homepage: http://ijai.iaescore.com
Internet job searching means using the Internet to find employment and job candidates; as such, it is performed by employers and job seekers. Nowadays, many Internet users, especially teenagers, search for their desired job [1]. Job seekers find this easier than traditional job searching methods, which involve newspapers, flyers, and advertisements. Therefore, searching for work on the Internet is faster and offers more options. Moreover, Internet job hunting provides an empirical scenario for understanding the correlation between the quality and amount of information. This study aims to visualize a vast range of jobs from all over the country, according to Jobstreet Malaysia. Jobs are abundantly available for all graduates from any university or faculty in Malaysia to browse. Jobstreet’s job information consists of multiple attributes such as the salary, position, job type and
Faculty of Computer and Mathematical Sciences, Universiti Teknologi MARA Cawangan Melaka Kampus Jasin, Melaka, Malaysia
1
IAES International Journal of Artificial Intelligence (IJ-AI) Vol. 11, No. 3, September 2022, pp. 1130~1142 ISSN: 2252 8938, DOI: 10.11591/ijai.v11.i3.pp1130 1142 1130
A linear regression approach to predicting salaries with visualizations of job vacancies: a case study of Jobstreet Malaysia
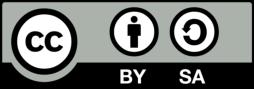
One of the leading employment information providers in Asia is Jobstreet. It was founded in Malaysia in 1997 and is widely acknowledged to be one of Asia’s leading online employment marketplaces. Its main vision is to connect businesses with talent and improve lives by advancing careers Jobstreet Malaysia entered the market and achieved profitability in early 1999 [10] As a company, Jobstreet noticed that over 10 million job seekers and over 90,000 employers utilized their services. Despite its many competitors, such as LinkedIn, Monster Inc, and others, job seekers in Malaysia are still utilizing the Jobstreet website because of its ease of use [11]. Most graduates use this website to search for a job. Jobstreet has been in a robust position in Malaysia for almost 23 years. Hence, the Jobstreet website was chosen for this studyThscopeisstudy proposes the development of a web based application written in Python. Obtained from an online platform, the data was extracted from this website [12] with Python using web scraping. The data scraping from the Jobstreet website was extracted for only six months from November 2020 to May 2021 because Jobstreet only allows clients to advertise their job vacancies Only information in English was used However, the jobs offered on the Jobstreet website were not as expected because of the ongoing COVID 19 pandemic. The study scope covers all 13 jobs on Jobstreet, as listed in Table 1.
and Communication Job 4 Building and Construction Job 5
and
Job 12 Sciences Job 13 Services Job
Int J Artif Intell ISSN: 2252 8938 A linear regression approach to predicting salaries with (Khyrina Airin Fariza Abu Samah) 1131 description, and location. However, far less information is provided in relation to the salary offered, the job scope, and the requirements needed to apply for the job, which graduate students need to find and explore [2]. The Society for Human Resource Management (SHRM) has received many requests from job seekers to identify salaries [3]. Salary information has been a significant problem for job seekers because they tend to compare salaries during their job search. Shlee and Karns [4] supported the evidence that graduate students frequently search for a job with a higher starting salary. They tend to notice that a salary for a vacant position is based on the current salary trends [5], but Jobstreet seems to offer limited information on the salaries offered.
and Training Job 6 Engineering Job 7 Healthcare Job 8 Restaurant and Hotel Job 9 Computer and Information Technology Job 10 Manufacturing Job 11
Table 1. Types of Media Education Sales Marketing
This study implements a web based application using Python based on the problems discussed above. It helps users to quickly view the jobs offered on Jobstreet Malaysia in all domains. Therefore, visualizing the data provides a visual representation that allows the user to see more quickly an overview of all the posted jobs that met their criteria. Data extraction and visualization are the two key modules in the study. A vast amount of data with multiple properties can be analyzed more easily with this tool. Hence, this system allows job seekers to analyze jobs on Jobstreet more easily. This paper is organized as follows: it begins with a brief introduction in section 1. Section 2 explains the related work, followed by the research methodology in section 3. Section 4 elaborates on the results and discusses their reliability, functionality, and usability. Finally, section 5 concludes the study and briefly mentions potential future improvements.
13 jobs in Jobstreet No Types of Jobs 1 Account and Finance Job 2 Admin and Human Resources Job 3 Arts,
Besides, job seekers may face difficulties comparing and visualizing the demand and trends of the jobs offered on Jobstreet. They are unaware of the higher positions offered on Jobstreet, so they cannot choose a suitable company. Takle [6] claimed that most job seekers do not read the job description details, and [7] proved that some vacancies do not provide the complete requirements, descriptions, expectations, and provisions. According to [8], an effective job search strategy begins with the knowledge of yesterday’s, today’s, and tomorrow’s technologies. Job searchers should pay greater attention to the current employment trends when seeking work. As a result, it is critical to analyze and track the top ten job title categories to monitor the frequency of the most in demand titles and visualize this information [9]
2. RELATED WORK 2.1. Jobstreet Malaysia
ISSN: 2252 8938 Int J Artif Intell, Vol. 11, No. 3, September 2022: 1130 1142 1132 2.2. Linear regression A supervised machine learning method known as linear regression (LR) is often used in mathematical research approaches It measures the expected effects and models these against numerous input variables [13] In biological or clinical research, the researcher frequently attempts to comprehend or link two or more independent (predictor) factors to predict an outcome or a dependent variable [14]. A prediction is a forecasted future event [15], and LR is the most fundamental and widely used predictive analysis [16] [18] The internet is growing continuously; for example, in recent years, Twitter has been generating 12 terabytes (TB) of data daily, while Facebook has been generating 4 petabytes (PB) of data daily. As a result, collecting, examining, and modeling this massive amount of data is critical to predicting future events in various fields [19] Simple LR approaches are used in this study to predict each job’s salary based on years of experience. The data trend is taken between November 2020 and May 2021 and helps the jobseeker predict the trend, such as salary, using the data from Jobstreet. Analysis of regression estimates shows the connection between one or more independent variables and a dependent variable. The task of fitting a single line through a scatter plot is at the regression analysis’s core. The LR formula is in (1). �� =��+���� (1)
The explanatory and dependent variables are both given as X. The slope of the line is b and the intercept is a. The best fit regression line has the smallest sum of the squares. The equation line of regression is obtained by minimizing the sum of the squares created; this is presented as (2). Using the Scikit learn function, the LR was imported. Table 2 shows the three parameters involved in the LR ensemble process Using the Scikit learn, we imported the LR, and Table 2 shows three parameters involved in the ensemble process of LR. �� = ∑(�� ��)(�� ��) ∑(�� ��)2 (2) Table 2. Parameters in the ensemble process of linear regression Parameter’s Name Explanation Fit (x, y) Fit linear model Predict (x) Predict using the linear model Score (x,y) Return the coefficient of determination of R2 of the prediction
There is no standard conceptual framework for visualization users across different application areas can explain and share techniques [20]. Computer to perceptual representation mapping is called visualization, and it uses encoding techniques to improve human comprehension and communication [21] [22]. Enhancing trust in modelling machine learning has driven demand increment in better and more effective tools for visualization [23] [25] The four visualization techniques used in the study were the line chart, bar chart, treemap chart, and word cloud. This made visualizing the Jobstreet website data more effective [26]. Hence, it became easier to identify the patterns, trends, and outliers in massive datasets. This study intended to transform the data into an effective visualization technique to provide information for job seekers We use plotly, an open source interactive graphics library for Python for data visualization. The data was first imported into the pandas’ data frames in interactive Python charts and displayed. The data was visualized on the charts to enable rapid comparisons using several visualization techniques
2 3 Visualization techniques
3.1. System design
3. RESEARCH METHOD
Figure 1 shows the overview of the research design for overall system development. The study methodology was divided into three sections for detailed development. It involved the system design, back end and front end development.
The application of a system’s product development principles might be considered system design. The design process is facilitated by developing design diagrams. It involved the use case diagrams, flowcharts, and user interfaces, as described in the following subsections.
3.1.2. Flowchart diagram Figure 3 describes the flowchart of the overall design. Firstly, the user signs in to the system. In this flowchart, users can register a new account or sign in. The user can register a new account if they do not yet have an account. Following the “Visualization of Each Jobs” page, the user must choose a job and visualization type. Next, if the user clicks the “Map Visualization” page, they must choose a job in order to view the map visualization. For the “Tables” page, the user can download each job’s comma separated value (CSV) files. The user can also click on the “Jobstreet Website” page to be redirected to the Jobstreet site. If the user clicks the “Prediction Salary” page, they are redirected to the prediction page, which predicts salaries based on years of experience. Lastly, the user can click on the “Logout” button to log out.
Figure 2 displays the use case diagram for this system as the interactions needed to complete the task. There are eight cases involved directly from the user and four indirect cases for the system.
Internal and external factors are included in the use case diagram to gather the system requirements.
Int J Artif Intell ISSN: 2252 8938 A linear regression approach to predicting salaries with (Khyrina Airin Fariza Abu Samah) 1133
Figure 1. Flow diagram of research design
3.1.1. Use case diagram
Figure 2. Use case diagram of the system
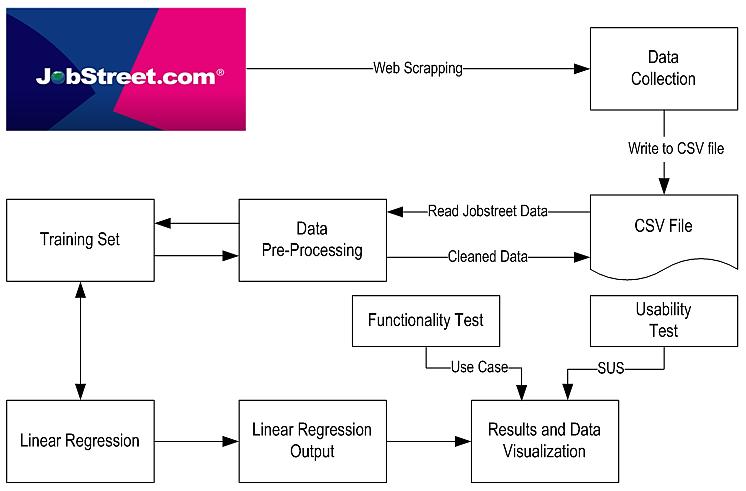
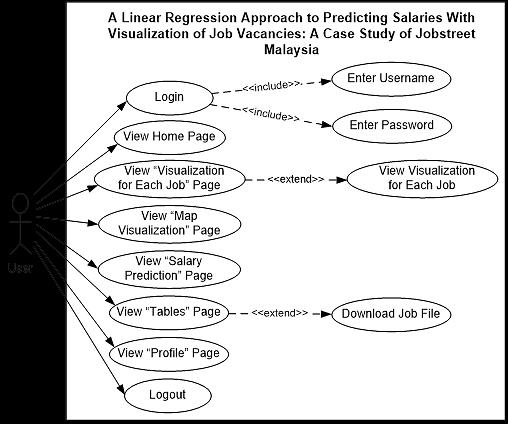
3.1.3.
Figure
ISSN: 2252 8938 Int J Artif Intell, Vol. 11, No. 3, September 2022: 1130 1142 1134 User interface
The system’s user interface depicts the system’s visual component layout. The user interface was developed according to the use case diagram and the description of the system’s features was added. This stage is described in the back end development subsection. 3. Flowchart diagram for overall system
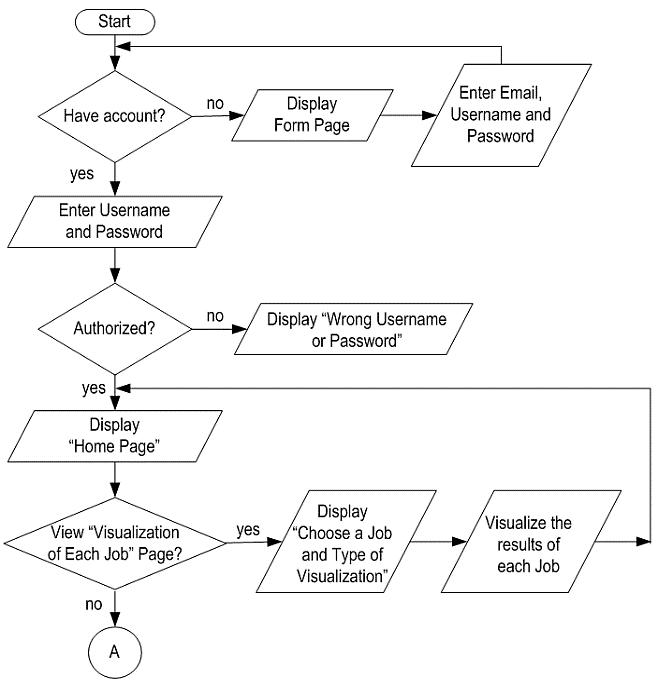
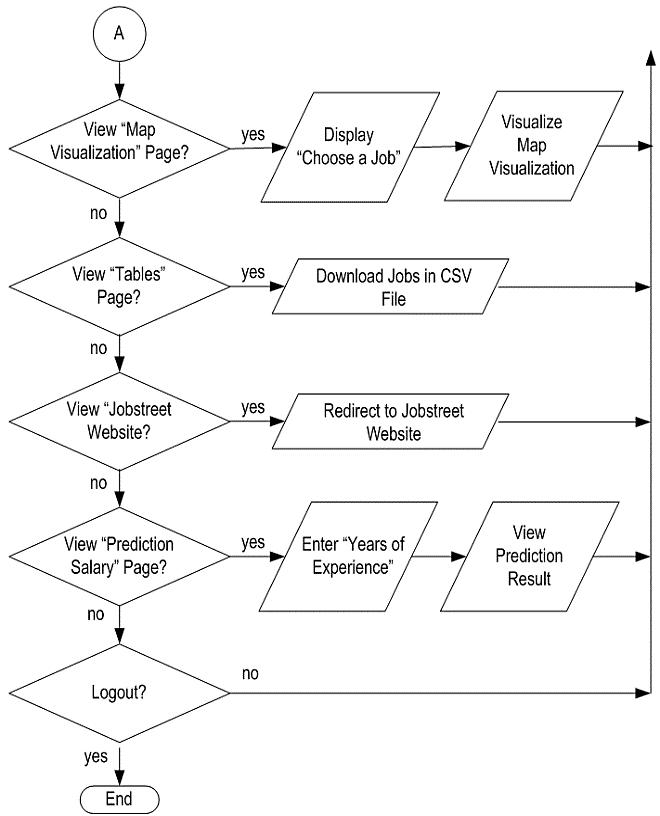
The MAE is a regression model evaluation statistic. LR was unsuitable for accuracy tests because this project
c. Visualize the training and testing sets the LR model was built after the training and testing sets are ready. The code “L.fit(xtrain, ytrain)” was used to pass the xtrain, which contains the years of experience value, and ytrain, which includes the salary values. Thus, the model was formed.
3.2.2. Prediction model
A regression model’s dependability is assessed by how well its predictions match actual values. Nevertheless, error metrics created by statisticians allow a model’s dependability to be evaluated and comparisons of regressions with different parameters [29]. The metrics summarize the data’s quality concisely and practically. The mean absolute error (MAE) was used to determine the quality of the model.
Int J Artif Intell ISSN: 2252 8938 A linear regression approach to predicting salaries with (Khyrina Airin Fariza Abu Samah) 1135
e. Predict the data based on the years of experience that a specific user wants to predict.
4.1. Reliability testing
a. Import the dataset the dataset was in a CSV format file, where x represents the years of experience column and y represents the salary column.
3.2. Back end development
4. RESULTS AND DISCUSSION
d. Initialize and fit the regression model to ensure that the training and testing sets are visualized in the same direction. If so, the model can be considered good to use for the dataset.
3.3. Front end development
The development on the server side is called back end development the back end code aids in transmitting the underlying data to the front end site. Python was the back end programming language used for data development and implementation. Data preparation and the prediction model using the LR algorithm are the two of the back end task that are important for training and testing data:
3.2.1. Data preparation
We collected the dataset from www.jobstreet.com using web scraping, which scrapes all the job categories on the Jobstreet website except the “others” category. The total data scraped produced 22,250 jobs with ten attributes: job title, salary, company, location, description, requirements, qualification, job type, career level, and years of experience. These jobs were scraped from November 2020 to May 2021 because Jobstreet only allows clients to advertise a job vacancy for six months. Besides, the jobs offered on the Jobstreet website were not as expected because of the COVID 19 pandemic. Despite the many ways to scrap data, such as using Rapidminer and Octopurse software, Python is more efficient and could customize the data required for this project. The scraped data for one job took more than 30 minutes to acquire, and limited time was available to discard the data since the Jobstreet website does not allow data scraping during its maintenance periods
In this project, data cleaning was performed to remove all duplicated data and enter the dataset’s null values using Microsoft Excel. The “remove duplicates” function in an Excel file achieved this much faster. The dataset was saved to a working directory after data cleaning. It was then ready to be fed directly into the machine learning algorithm to extract meaningful information in relation to salary predictions
The data collection was split 80:20, whereby 80% was used for training data and the remainder was used for testing data [27]. LR predicts the salary based on the years of experience specified for each job. This model used four libraries: NumPy, pandas for the dataset, sklearn to implement machine learning functions, and matplotlib to visualize plots for viewing. Five steps were involved in this LR:
Front end development creates the client side, focusing on what consumers see graphically in their browser or on their application [28] Front end languages such as hypertext markup language (HTML), cascading style sheets (CSS), Javascript, and Jquery were used in this project to integrate Flask’s back end framework. After the data processing phases, the Flask web framework and the Python data visualization tools were used to build custom plots and charts in a Python web application context, leveraging the power of both the front end and back end development. For each interface, the workflow was depicted in the application with which the user would interact. Also discussed are the correct subsections for each feature of the completed system’s interface design, including the home page, visualization for all the job pages, as well as the visualization for each job page, map page, predicted salary page, tables page, Jobstreet website, and profile page
b. Split the data into two sets: the training and testing sets. The ratio of the testing set for this project was 0.2. A testing set must not be bigger than a training set because it may lack data to train. The random state is the seed for the random number generator, which can be left blank or as 0.
It is critical to test the features of an application to ensure they all work correctly and that any detected errors are corrected. Functionality testing aims to test each function of the visualization application rigorously to determine how closely the specifications match by providing the appropriate input and comparing the output to the functional requirements outlined in previous chapters. The test was conducted using test case scenarios derived from the program specifications with the functionalities tested. The results show that the system ran successfully as planned, without any prompted errors.
Computer/Information Technology Job 2306.2030678463498 Manufacturing Job 1577.8285877577500 Sales/Marketing Job 1925.2649997237856 Sciences Job 1707.7019373539054
Table 3. Mean absolute error value of each job Jobs Mean Absolute Error (MAE)
Figure 4. Scatter diagram MAE with the actual value
Account Job 2167.3575862456923 Admin/Human Resouces Job 1856.2874681381668 Arts Job 1477.1881267666222
ISSN: 2252 8938 Int J Artif Intell, Vol. 11, No. 3, September 2022: 1130 1142 1136predicts the salary based on the years of experience required. Hence, it was appropriate to ascertain the quality of the data and the difference between the actual and predicted data [30]. As shown in (3), MAE is the most straightforwardly understood regression error metric; where y is the prediction, �� ̂ is the true value and n stand for total number of data points. ������ = 1 �� ∑|�� ��̂| (3)
Moreover, the MAE was the easiest metric to understand because this project evaluated the absolute variation using the actual results and those predicted by a computer model [31]. Using the absolute residual values, the MAE does not indicate whether a model is performing well or poorly (no matter whether the model undershoots or overshoots the actual values). The overall error is proportional to each residual, so larger errors are added linearly. A small MAE suggests a successful prediction, but a large MAE indicates that the model may encounter difficulties in some areas. Table 3 shows the MAE value for each job tested using the testing and prediction data. Figure 4 shows the scatter graph plotted to compare the MAE values with the actual values for two jobs. It can be observed that the predicted values positively correlate with the actual values
4.2. Functionality testing
Building/Construction Job 1952.8038000710462 Education/Training Job 1922.3948270772370 Engineering Job 2082.1180991543570 Healthcare Job 2042.8448050388540 Hotel/Restaurant Job 424.79798113363614
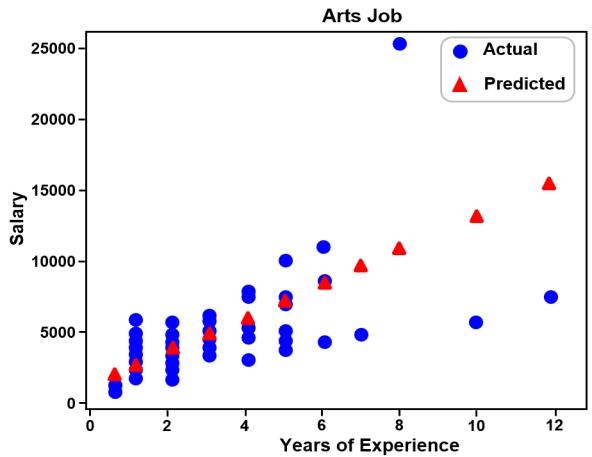
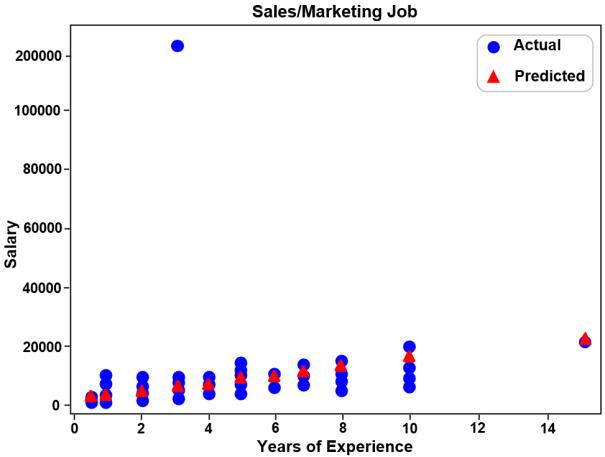
A linear regression approach to predicting salaries with (Khyrina Airin Fariza Abu Samah) 1137
Int J Artif Intell ISSN: 2252 8938
The data visualization was also tested to ensure users could view the selected visualization graph. The visualization provides an easily accessible reporting feature so that the user can instantly see and understand the trends and patterns. Figure 5 shows the visualization page menu for all 13 jobs on Jobstreet that are covered in this study. Figure 6 shows the visualization page that visualized four visualizations for the technology jobs: i) Figure 7 shows the top five locations with the highest number of jobs; ii) Figure 8 shows a line chart of the salaries from each job; iii) Figure 9 shows the qualifications word cloud; and iv) Figure 10 shows job titles word cloud. The user can choose what visualization they want to view. The bar and line chart visualizations are presented interactively. This allows the user to zoom in or out of the chart, download it onto their computer as a png file, pan, box select, lasso select, autoscale, reset axes, toggle the spike lines, show the closest data on hover, and compare data on hover. Figure 11 shows the interface of the “Map Visualization” page, where the user can zoom in and out on the map to view the number of jobs at a specific location. The cluster in red means that the location has many jobs being offered, the green cluster has few jobs, and the yellow cluster means that the location has an average number of jobs being offered. Figure 12 shows the interface that allows users to predict their salary based on experience by entering whichever years of experience they choose.
Figure 5. View the “Visualization of Each Job” page Figure 6 Four visualizations menu for computer/information technology jobs
Figure 7. Top 5 locations that have a higher number of computer/information technology jobs
computer/information
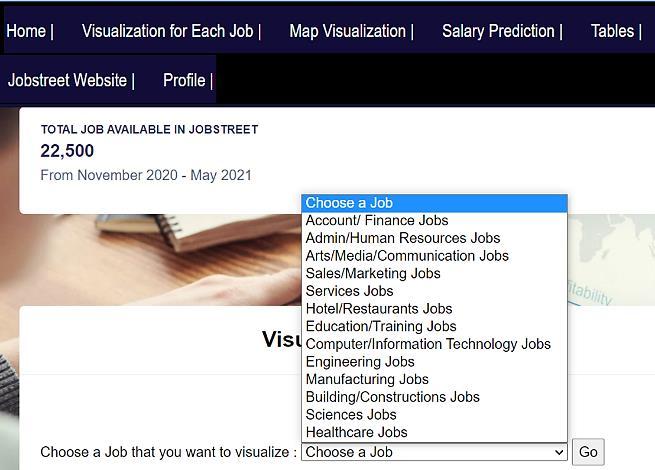
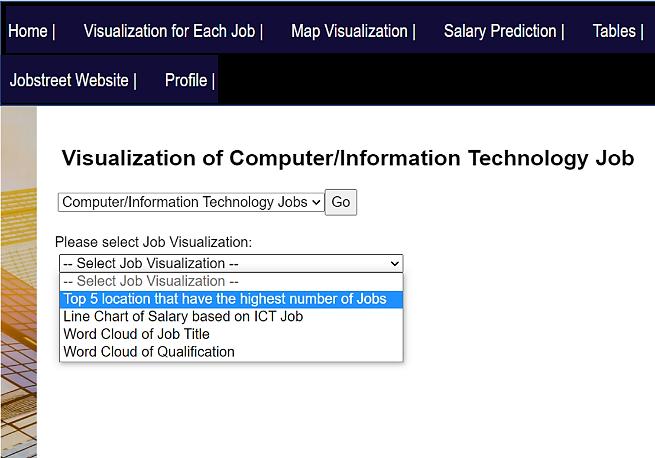
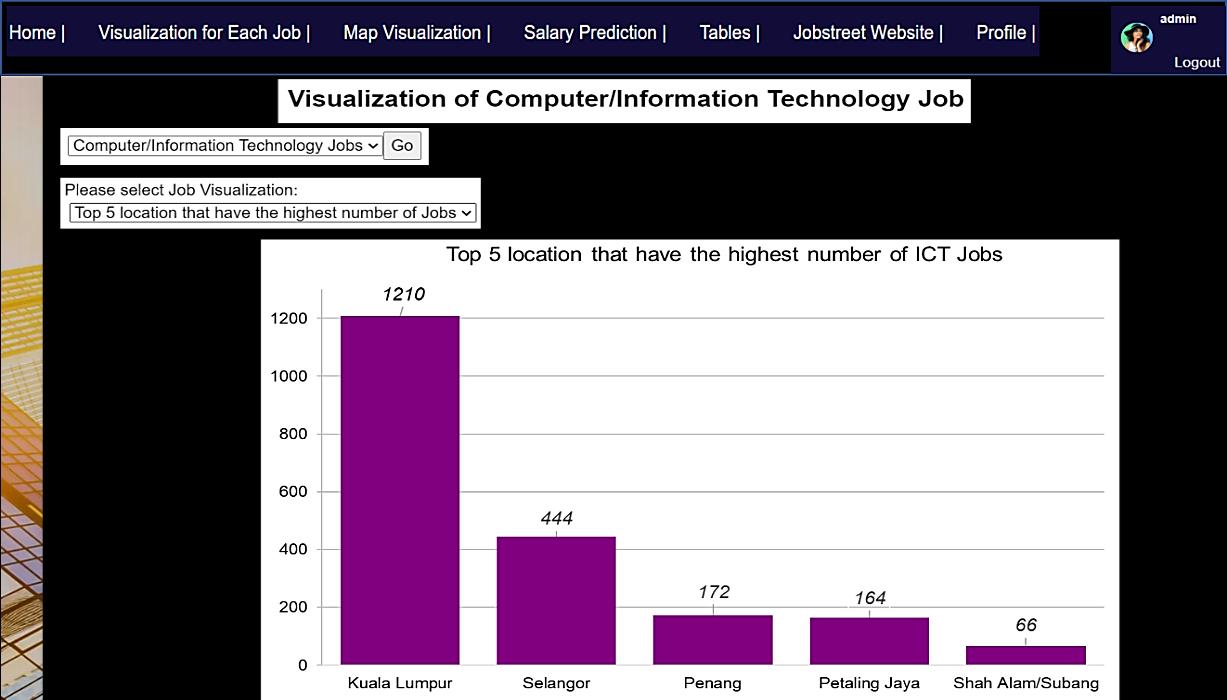
ISSN: 2252 8938 Int J Artif Intell, Vol. 11, No. 3, September 2022: 1130 1142 1138 Figure 8. Line chart of salary based on computer/information technology job Figure 9. A word cloud for qualification required for computer/information technology jobs Figure 10. A word cloud for job title offered for Computer/Information Technology jobs Figure 11. Top 5 locations that have a higher number of computer/information technology jobs
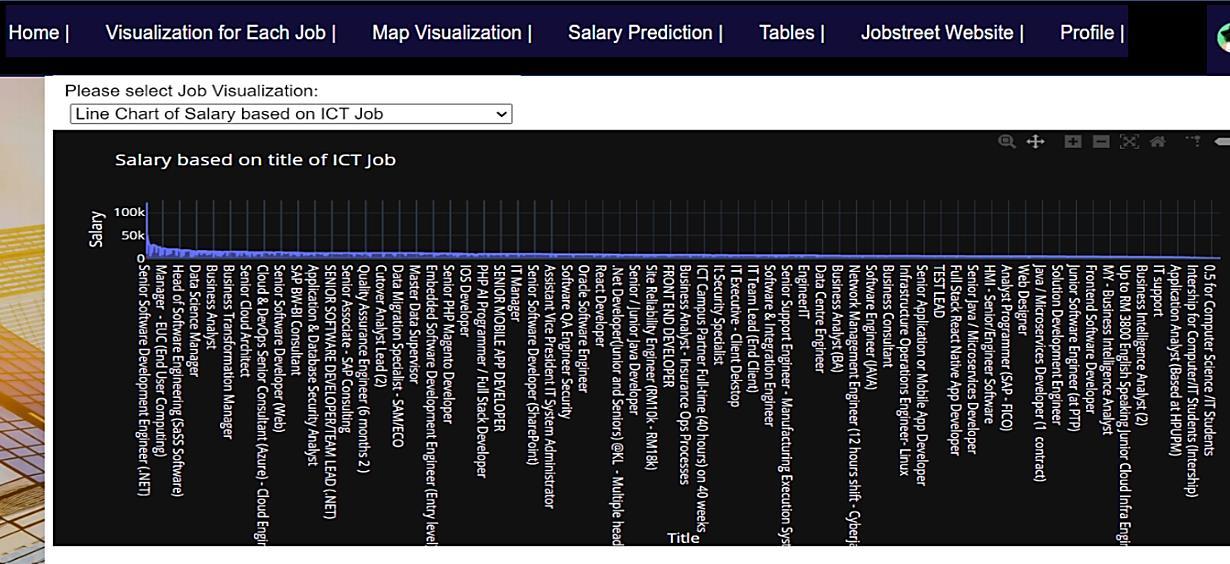
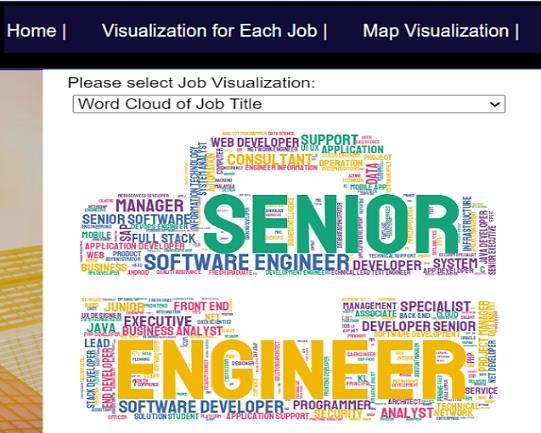
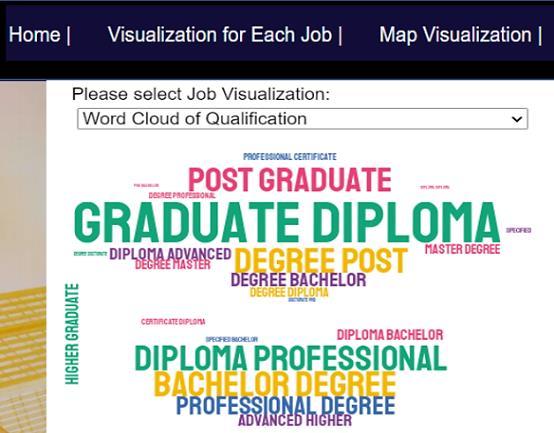
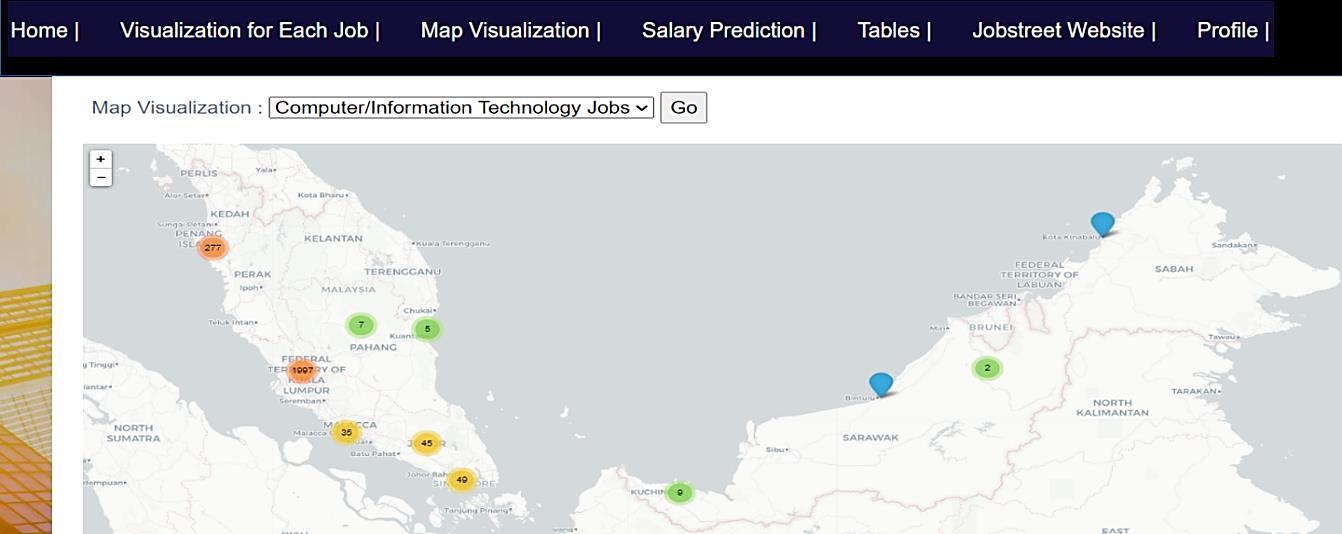
Int J Artif Intell ISSN: 2252 8938 A linear regression approach to predicting salaries with (Khyrina Airin Fariza Abu Samah) 1139
Figure 12. Selection of the job visualization from computer/information technology jobs 4.3. Usability testing
extreme circumstances of COVID 19, in person usability testing was not possible. The usability tests were conducted individually with the respondents via TeamViewer, with respondents receiving remote access to the program. Thirty respondents seeking jobs were chosen randomly for the usability test and asked to evaluate each system’s functionality. Basic information was requested, such as their name, status, and feedback, using the SUS questionnaires on the google form
Figure 14 shows the histogram of the SUS scores. The y axis (the plotted histogram’s vertical axis) illustrates the frequency of users that answered the SUS, while the x axis (the horizontal axis) shows the percentage range of the SUS scores. The data spread between 90% to 100%, based on the histogram. The plotted graph has a normal distribution with a range starting at 88%, followed by a new range for every increase of 2%. The highest frequency was 96% to 98%, a range that seventeen respondents fell into. The histogram centered on a frequency of 94% to 96%. Ten respondents fell into the category below the central value, and eleven respondents lay in above the central value In total, the 30 users who participated in the SUS questionnaire had a SUS score of 96.58%. The baseline for the SUS average score was 68%, indicating the average usability of the system. A score of less than 68% would indicate that the application’s usability was likely to cause problems, which would need to be addressed. When the SUS is greater than 80%, the system is entirely usable. Wang [33] claimed that if SUS scores of 80.3% or higher can be obtained, this equates to an ‘A’ result. Since a high score of more than 80% was obtained, this web based application was proven usable. It is also worth noting that most respondents gave positive feedback and said they would suggest it to their friends.
The bar chart diagram of the ten SUS statement scores is plotted, as shown in Figure 13. This displays the scales of the SUS statements, as answered by the users in the questionnaire. The graph demonstrates that most respondents agreed with the odd numbered questions, reflecting affirmative statements, which meant that the users deemed the application to be useful. The respondents also thought the system interface was pleasant and included all the expected features and functionality. This indicates that consumers would not require technical support to use the application’s features and navigate other sites. The majority of respondents were pleased with the application.
The system usability scale (SUS) was adopted to evaluate the system usability. This was a Likert scale consisting of ten short questions, which were answered by the application users. Based on [32], usability testing is a commonly used method for evaluating user efficiency and their acceptance of products and systems. Usability testing is a technique used in user centric interface design to assess a product by testing it on Dueusers.tothe
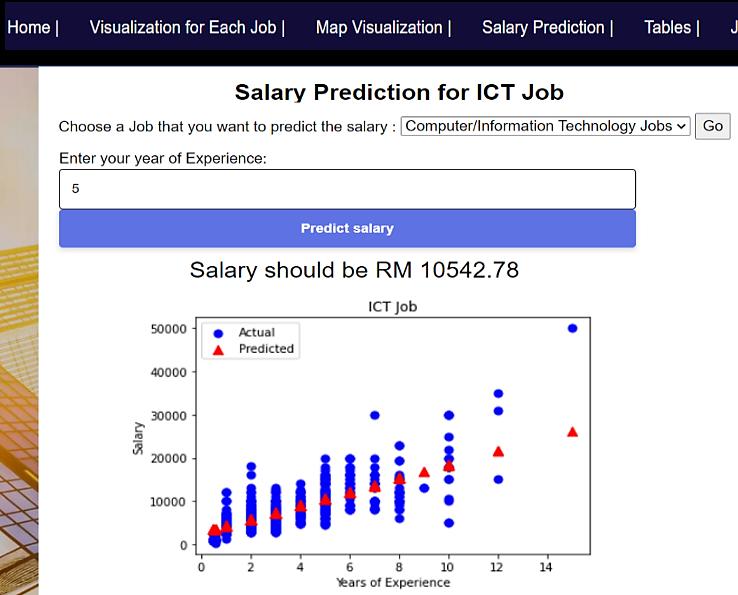
[5] J. Säve Söderbergh, “Gender gaps in salary negotiations: Salary requests and starting salaries in the field,” J. Econ. Behav. Organ., vol. 161, pp. 35 51, May 2019, doi: 10.1016/j.jebo.2019.01.019.
ISSN: 2252 8938 Int J Artif Intell, Vol. 11, No. 3, September 2022: 1130 1142 1140 Figure 13. Bar chart of SUS result Figure 14. Histogram of SUS result
[2] Y. L. Liu, “Providing more or less detailed information in job advertisements Does it matter?,” Int. J. Sel. Assess., vol. 28, no. 2, pp. 186 199, Jan. 2020, doi: 10.1111/ijsa.12281.
[7] S. Chala and M. Fathi, “Job seeker to vacancy matching using social network analysis,” in 2017 IEEE International Conference on Industrial Technology (ICIT), Mar. 2017, pp. 1250 125, doi: 10.1109/icit.2017.7915542.
The research was sponsored by Universiti Teknologi MARA Cawangan Melaka under the TEJA Grant 2021 (GDT 2021/1 28).
ACKNOWLEDGEMENTS
[4] R. P. Schlee and G. L. Karns, “Job requirements for marketing graduates: Are there differences in the knowledge, skills, and personal attributes needed for different salary levels?,” J. Mark. Educ., vol. 39, no. 2, pp. 69 81, Jun. 2017, doi: 10.1177/0273475317712765.
The visualization application developed using the dashboard can assist job seekers to easily gain an overview of the jobs offered by identifying the patterns and trends on the Jobstreet website. This visualization changes large and complex data from Jobstreet into understandable and usable data. The researchers have highlighted the drawbacks of using the limited information on the Jobstreet website as single source data. The application’s interactive visualization has changed how users interact with data by focusing on graphic representations. The LR used to predict salaries was incorporated into the application, permitting users to employ the model to predict salaries based on years of experience or similar paths. This would allow the users to gain an overview of the visualization of jobs on the Jobstreet website and predict their salaries according to the years of experience required. Future recommendations concern potential upgrades to the application that could allow the real time data from the Jobstreet website to be visualized, thus offering job seekers a more rewarding experience. The authors recommended purchasing Jobstreet premium APIs as this allows more data to be extracted, such as the job seeker’s details; thus, this application would be suitable for both companies and job seekers.
[6] J. Takle, “Jobviz A visual tool to explore the employment data,” University of New Jersey, 2015.
REFERENCES [1] E. A. J. van Hooft, J. D. Kammeyer Mueller, C. R. Wanberg, R. Kanfer, and G. Basbug, “Job search and employment success: A quantitative review and future research agenda.,” J. Appl. Psychol., vol. 106, no. 5, pp. 674 713, May 2021, doi: 10.1037/apl0000675.
[3] B. Sareen, “Relationship between strategic human resource management and job satisfaction,” Int. J. Curr. Res. Life Sci., vol. 7, no. 3, pp. 1229 1233, 2018.
[8] R. B. Mbah, M. Rege, and B. Misra, “Discovering job market trends with text analytics,” in 2017 International Conference on Information Technology (ICIT), Dec. 2017, pp. 137 142, doi: 10.1109/icit.2017.29.
[9] L. Samek, M. Squicciarini, and E. Cammeraat, “The human capital behind AI: Jobs and skills demand from online job postings,” OECD Sci. Technol. Ind. Policy Pap., vol. 120, Sep. 2021, doi: 10.1787/2e278150 en. [10] “Jobstreet Corporation Bhd,” Jobstreet.com, 2011. . [11] N. Kamaruddin, A. W. A. Rahman, and R. A. M. Lawi, “Jobseeker industry matching system using automated keyword selection and visualization approach,” Indones. J. Electr. Eng. Comput. Sci., vol. 13, no. 3, pp. 1124 1129, Mar. 2019, doi: 10.11591/ijeecs.v13.i3.pp1124 1129. [12] “Jobstreet,” 2021. https://www.jobstreet.com.my/ (accessed Mar. 11, 2021). [13] H. I. Lim, “A linear regression approach to modeling software characteristics for classifying similar software,” in 2019 IEEE 43rd
5. CONCLUSION
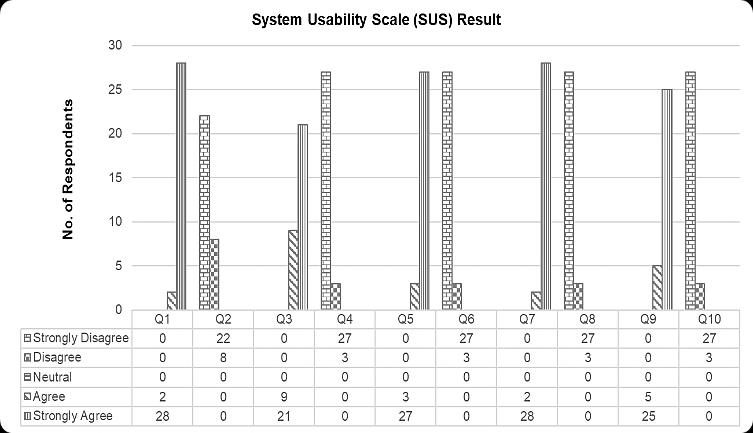
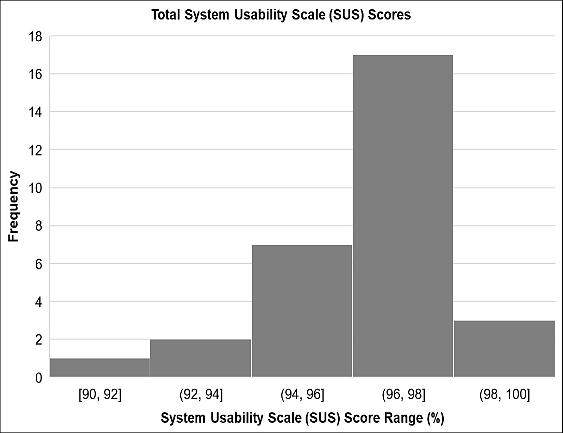
[27] N. M. Khan, N. Abraham, and M. Hon, “Transfer learning with intelligent training data selection for prediction of Alzheimer’s disease,” IEEE Access, vol. 7, pp. 72726 72735, 2019, doi: 10.1109/access.2019.2920448.
[16] N. Tiraiyari, K. Idris, U. Jegak, and H. Azimi, “Competencies influencing extension workers’ job performance in relation to the good agricultural practices in Malaysia,” Am. J. Appl. Sci., vol. 7, no. 10, pp. 1379 1386, Oct. 2010, doi: 10.3844/ajassp.2010.1379.1386.
[33] Y. Wang, “System usability scale : A quick and efficient user study methodology,” IXD@Pratt, 2018. .
[32] A. M. Wichansky, “Usability testing in 2000 and beyond,” Ergonomics, vol. 43, no. 7, pp. 998 1006, Jul. 2000, doi: 10.1080/001401300409170.
BIOGRAPHIES OF AUTHORS Khyrina Airin Fariza Abu Samah is a senior lecturer at the Faculty of Computer and Mathematical Sciences in Universiti Teknologi MARA (UiTM), Melaka Jasin Campus. Before joining UiTM, she has 13 years of working experience in the semiconductor industry. She has a Diploma, Bachelor’s Degree and Master’s Degree in Computer Science and PhD in Information Technology. Her research interest is in Artificial Intelligent, Operational Research, Algorithm Analysis, Clustering and Optimization, Evacuation Algorithm, Internet of Things (IoT) and Sentiment Analysis. She can be contacted at email: khyrina783@uitm.edu.my
Int J Artif Intell ISSN: 2252 8938 A linear regression approach to predicting salaries with (Khyrina Airin Fariza Abu Samah) 1141 Annual Computer Software and Applications Conference (COMPSAC), Jul. 2019, pp. 942 943, doi: 10.1109/compsac.2019.00152.
[14] K. Kumari and S. Yadav, “Linear regression analysis study,” J. Pract. Cardiovasc. Sci., vol. 4, no. 1, pp. 33 36, 2018, doi: 10.4103/jpcs.jpcs_8_18.
[18] S. Liu, M. Lu, H. Li, and Y. Zuo, “Prediction of gene expression patterns with generalized linear regression model,” Front. Genet., vol. 10, pp. 1 11, Mar. 2019, doi: 10.3389/fgene.2019.00120.
[28] M. Kaluža and B. Vukelić, “Comparison of front end frameworks for web applications development,” Zb. Veleučilišta u Rijeci, vol. 6, no. 1, pp. 261 282, 2018, doi: 10.31784/zvr.6.1.19.
[19] S. Rong and Z. Bao wen, “The research of regression model in machine learning field,” MATEC Web Conf., vol. 176, p. 1033, 2018, doi: 10.1051/matecconf/201817601033.
[15] K. E. Ehimwenma and S. Krishnamoorthy, “Design and analysis of a multi agent e learning system using prometheus design tool,” IAES Int. J. Artif. Intell., vol. 10, no. 1, pp. 9 23, Mar. 2021, doi: 10.11591/ijai.v10.i1.pp9 23.
[23] C. Xiong, L. Padilla, K. Grayson, and S. Franconeri, “Examining the components of trust in map based visualizations,” in Proceedings of the EuroVis Workshop on Trustworthy Visualization (TrustVis ’19), 2019, pp. 19 23. [24] A. Chatzimparmpas, R. M. Martins, I. Jusufi, K. Kucher, F. Rossi, and A. Kerren, “The state of the art in enhancing trust in machine learning models with the use of visualizations,” Comput. Graph. Forum, vol. 39, no. 3, pp. 713 756, Jun. 2020, doi: 10.1111/cgf.14034. [25] M. Z. A. Razak, S. S. M. Fauzi, R. A. J. M. Gining, and M. N. F. Jamaluddin, “Data visualisation of vehicle crash using interactive map and data dashboard,” Indones. J. Electr. Eng. Comput. Sci., vol. 14, no. 3, pp. 1405 1411, Jun. 2019, doi: 10.11591/ijeecs.v14.i3.pp1405 1411. [26] Z. Yang and S. Cao, “Job information crawling, visualization and clustering of job search websites,” in 2019 IEEE 4th Advanced Information Technology, Electronic and Automation Control Conference (IAEAC), Dec. 2019, pp. 637 641, doi: 10.1109/iaeac47372.2019.8997713.
[17] A. F. Schmidt and C. Finan, “Linear regression and the normality assumption,” J. Clin. Epidemiol., vol. 98, pp. 146 151, Jun. 2018, doi: 10.1016/j.jclinepi.2017.12.006.
[29] A. Botchkarev, “A new typology design of performance metrics to measure errors in machine learning regression algorithms,” Interdiscip. J. Information, Knowledge, Manag., vol. 14, pp. 45 76, 2019, doi: 10.28945/4184.
[31] C. Chen, J. Twycross, and J. M. Garibaldi, “A new accuracy measure based on bounded relative error for time series forecasting,” PLoS One, vol. 12, no. 3, p. e0174202, Mar. 2017, doi: 10.1371/journal.pone.0174202.
[22] A. Srinivasan, S. M. Drucker, A. Endert, and J. Stasko, “Augmenting visualizations with interactive data facts to facilitate interpretation and communication,” IEEE Trans. Vis. Comput. Graph., vol. 25, no. 1, pp. 672 681, Jan. 2018, doi: 10.1109/tvcg.2018.2865145.
Nurqueen Sayang Dinnie Wirakarnain is a Bachelor’s student in Computer Science currently undergoing her internship programme. Her research interests are machine learning algorithms, data analysis and visualization. She is actively involved in the research group at UiTM. Currently, she is under an internship at Petronas Digital Sdn Bhd. She can be contacted at email: 2018276258@isiswa.uitm.edu.my
[20] M. Diamond and A. Mattia, “Data visualization: An exploratory study into the software tools used by businesses,” J. Instr. Pedagog., vol. 18, no. 1, 2017. [21] H. C. Purchase, N. Andrienko, T. J. Jankun Kelly, and M. Ward, “Theoretical foundations of information visualization,” in Lecture Notes in Computer Science (including subseries Lecture Notes in Artificial Intelligence and Lecture Notes in Bioinformatics), vol. 4950 LNCS, Springer Berlin Heidelberg, 2008, pp. 46 64.
[30] L. Frías Paredes, F. Mallor, M. Gastón Romeo, and T. León, “Dynamic mean absolute error as new measure for assessing forecasting errors,” Energy Convers. Manag., vol. 162, pp. 176 188, Apr. 2018, doi: 10.1016/j.enconman.2018.02.030.
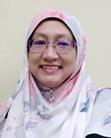




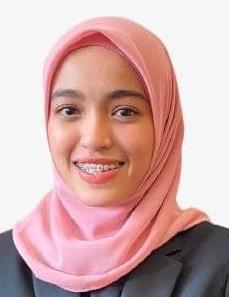




ISSN: 2252 8938 Int J Artif Intell, Vol. 11, No. 3, September 2022: 1130 1142 1142
Raseeda Hamzah is currently working as a Senior Lecturer in the Faculty of Computer and Mathematical Sciences, Universiti Teknologi MARA (UiTM), Shah Alam, Selangor, Malaysia. She received her bachelor and master degrees from Universiti Teknikal Malaysia Melaka and the University of Malaya. Her PhD in (Computational Linguistics) from UiTM. She is actively researching signal processing, pattern recognition and feature analysis for various areas and different types of data. She can be contacted at email: raseeda@uitm.edu.my
Nor Aiza Moketar is a Senior Lecturer in the Faculty of Computer and Mathematical Sciences, Universiti Teknologi MARA (UiTM), Melaka Jasin Campus. She holds a PhD and MSc in Software Engineering and Intelligence from UTeM and BSc in Information Technology from University Malaya. Before commencing as an academician, she worked as a software developer in a multi national company. Her research interests are Software Engineering, requirements, testing and Intelligence. She can be contacted at email: noraiza1@uitm.edu.my
Lala Septem Riza received PhD in Computer Science from Universidad de Granada, Spain in 2015. He works in the Department of Computer Science Education, Universitas Pendidikan Indonesia, Indonesia. He teaches machine learning, big data platform, and statistical data science. His research interests are in machine learning, data science, and education. He can be contacted at email: lala.s.riza@upi.edu.
Zainab Othman is a senior lecturer of Computer Science at the Faculty of Computer and Mathematical Sciences (UiTM), Melaka Jasin Campus. She holds MSc in Information Technology from UUM, Diploma and Bachelor’s Degree in Computer Science from UiTM and UKM, respectively. She has 24 years of teaching experience in various courses such as Software Engineering, Programming, Data Structures, Algorithm Analysis. Her research interest in Software Engineering, Algorithm, Programming, Game Based Learning, Augmented Reality. She can be contacted at email: zainab_othman@uitm.edu.my.
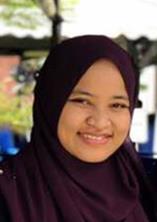




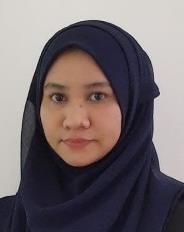




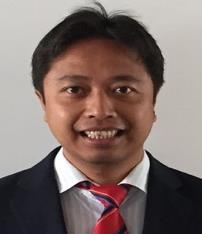




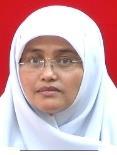




Email:talhasarwar40@gmail.com
INTRODUCTION
IAESInternationalJournalofArtificialIntelligence(IJ-AI) Vol.11,No.3,September2022,pp.1143∼1152 ISSN:2252-8938,DOI:10.11591/ijai.v11i3.pp1143-1152 ❒ 1143 AmachinelearningapproachforBengalihandwritten vowelcharacterrecognition ShahrukhAhsan1,ShahTarikNawaz1,TalhaBinSarwar2,M.SaefUllahMiah2,AbhijitBhowmik1 1DepartmentofComputerScience,AmericanInternationalUniversity-Bangladesh(AIUB),Dhaka,Bangladesh 2FacultyofComputing,CollegeofComputingandAppliedSciences,UniversitiMalaysiaPahang,Pekan,Malaysia ArticleInfo Articlehistory: ReceivedSep7,2021 RevisedJun17,2022 AcceptedJun24,2022 Keywords: BanglaLekha-isolated Bengalihandwrittenvowel recognition Handwrittencharacter recognition Machinelearning Supportvectormachine
Inaddition,severalworkshavebeendoneonBengalicharacterrecognition,whereithasbeenchallengingtoachievebetterexecutionandpredictionresultsduetothenaturalcomplexityofmostBengalialphabets. Thelanguagehasalongandrichscientificheritageofoverathousandyearsandahistoryoflanguageevolution.Researchershavepresenteddifferenttypesoffeatureextractiontechniquesandproposedsomenew featureextractiontechniquesforrecognizinghandwrittenBengalicharacters.SinceBengaliconsistsofdifferJournalhomepage: http://ijai.iaescore.com
1.
CCBY-SA license.
Thisisanopenaccessarticleunderthe
Handwritingrecognitionhasproventobequitechallenginginrecentyears.Handwrittencharacters bydifferentpeopleshowmanycomplexitiesasitisnotidenticalandvariesinshapesandwritingstyles[1], [2].TherehavebeenseveralmethodsthatareintroducedforEnglishcharacterrecognition.Oneofthemost applicabletechniquesisbytrainingneuralnetworksfortheacknowledgmentofcharacters[3].Atpresent,Bengaliisoneoftheutmostspokenlanguages,placedaroundfifthintheworldandsecondamongtheSouthAsian AssociationforRegionalCooperation(SAARC)countries[4].InalmostallphasesoflifeinBangladeshandin somepartsofIndia,languageisusedtocommunicate.Around220millionindividualsworldwidepresentlyutilizeBengalitotalkandcomposereason.Apropermachinelearningsystemthatworksefficientlytorecognize itscharactersislongoverdueforsuchawidelyusedlanguage.
CorrespondingAuthor: TalhaBinSarwar 26600,Pekan,Malaysia
ABSTRACT
Recognitionofhandwrittencharactersiscomplexbecauseofthedifferentshapesand numbersofcharacters.Manyhandwrittencharacterrecognitionstrategieshavebeen proposedforbothEnglishandothermajordialects.Bengaliisgenerallyconsideredthe fifthmostspokenlocallanguageintheworld.ItistheofficialandmostwidelyspokenlanguageofBangladeshandthesecondmostwidelyspokenamongthe22posted dialectsofIndia.ToimprovetherecognitionofhandwrittenBengalicharacters,we developedadifferentapproachinthisstudyusingfacemapping.Itisquiteeffectivein distinguishingdifferentcharacters.Therealhighlightisthattherecognitionresultsare moreefficientthanexpectedwithasimplemachinelearningtechnique.Theproposed methodusesthePythonlibraryScikit-Learn,includingNumPy,Pandas,Matplotlib, andsupportvectormachine(SVM)classifier.TheproposedmodelusesadatasetderivedfromtheBanglaLekhaisolateddatasetforthetrainingandtestingpart.Thenew approachshowspositiveresultsandlookspromising.Itshowedaccuracyupto94% foraparticularcharacterand91%onaverageforallcharacters.
FacultyofComputing,CollegeofComputingandAppliedSciences,UniversitiMalaysiaPahang
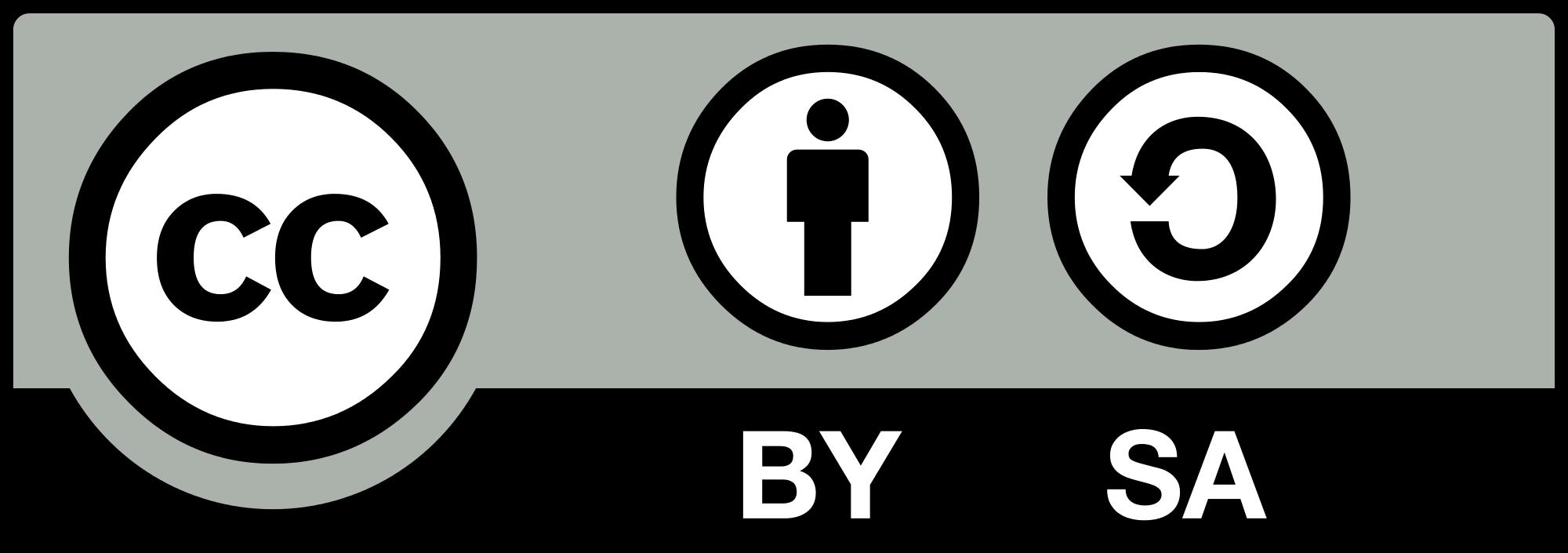
1144 ❒ ISSN:2252-8938 entpartssuchastheupperpart,middlepart,lowerpart,anddisjunctivepart,manyresearchershavedeveloped anatomicalfeatureextractiontechniques[5],[6].Sincethecharacterscanbedividedintodifferentzones,researchershaveusedazone-basedfeatureextractionmethod[7].Islam etal. usedamodifiedsyntacticmethod forrecognizingBengalihandwrittencharacters[8].Thetwomostpopularclassifiersusedtoclassifyhandwrittencharactersarethesupportvectormachine(SVM)andthehiddenMarkovmodel(HMM)[9, 10].Inthe SVM,thekernelthatworkswellistheradialbasisfunction(RBF)kernel.Itcanclassifywelldespitethedifferentwaysofwritingthecharacters.TheBengalilanguagehasfiftybasiccharacterswithnumerouscomparable signs.Toensurebetterexecutioninrecognizingthehandwrittencharacters,amethodfocusingonelevenvowel charactershasbeenproposedinthispaper.Figure
2.
1 representsasampleofelevenhandwrittenBengalivowel characters.
অ আ ই ঈ উ ঊ ঋ এ ঐ ও ঔ
Figure1.SampleofelevenhandwrittenvowelcharactersofBengalialphabet RELATEDSTUDY
ThereareseveralpreviousresearchworksontherecognitionofBengalihandwrittencharacters.Most ofthemusethetraditionalmachinelearningapproachorneuralnetworksforthistask.Convolutionalneuralnetworks(ConvNetsorCNNs)aredeepartificialneuralnetworksusedtoclassifyimages,groupthemby proximity,andrecognizeobjectswithinscenes[13],[14].Numerouscomputationscanrecognizefaces,street signs,andnumerouspiecesofvisualinformation.Hardlylessnoteworthyworkexistsfortherecognitionof Bengalicharacters.Bhowmik etal.proposedacombinedclassifierutilizingtheRBFsystemandSVM,multilayerperceptron(MLP)[15].Theyconsideredsomecomparablecharactersasasingleexampleandprepared theclassifierfor45classes[15].Anotherworkstatesthatthreedifferentcomponentextractionstrategieswere usedinthesegmentationphase,butthecharactersamplesweredividedinto36classes,combiningcomparative charactersintoasingleclass[16].Ontheotherhand,fewerworksarenotbasedonConvNets.Oneofthese overlookedthingsisanalyzingthepatternoftheimageasamatrixanduseoftheScikit-learnlibrary,whichalso specializesinclassificationandregressionalgorithms,includingSVM.SomestudiesrelysolelyontheCNN architecture.Das etal.[17]proposedaCNN-basedarchitectureforrecognizinghandwrittenBanglacharac-
IntJArtifIntell,Vol.11,No.3,September2022:1143–1152
TheproposedmethodmainlyusesthePythonlibraryScikit-learn[11]andanadditionalSVMclassifier onaderivedmatrixdataset.Ithasbeenusedtosolvepatternrecognitionproblemsmostlyappliedtovisual images.RecognitionofBengalihandwrittencharactersischallengingcomparedtothepublishedformsof characters.Thisisbecausethecharactersputonpaperbydifferentindividualsarenotidenticalanddifferin severalfeaturessuchassize,shape,andorientationofthewriting.Scikit-learnisoneofthenewmetricsfor imagecharacterizationframeworksthatlegitimatelyextractvisualexamplesfrompixelimageswithminimal pre-processing.OurproposedmethodclassifiesindividualcharactersusingadaptedScikit-learnfunctions,such asclassificationandregressionalgorithms,e.g.,SVM.SVMisusedtoclassifydifferentshapes,andvariantsof handwrittencharactersfromtheBanglaLekha-Isolated[12]dataset.TheproposedmethodusingSVMexhibits highorderingaccuracyandoutperformsseveralotherapproaches.
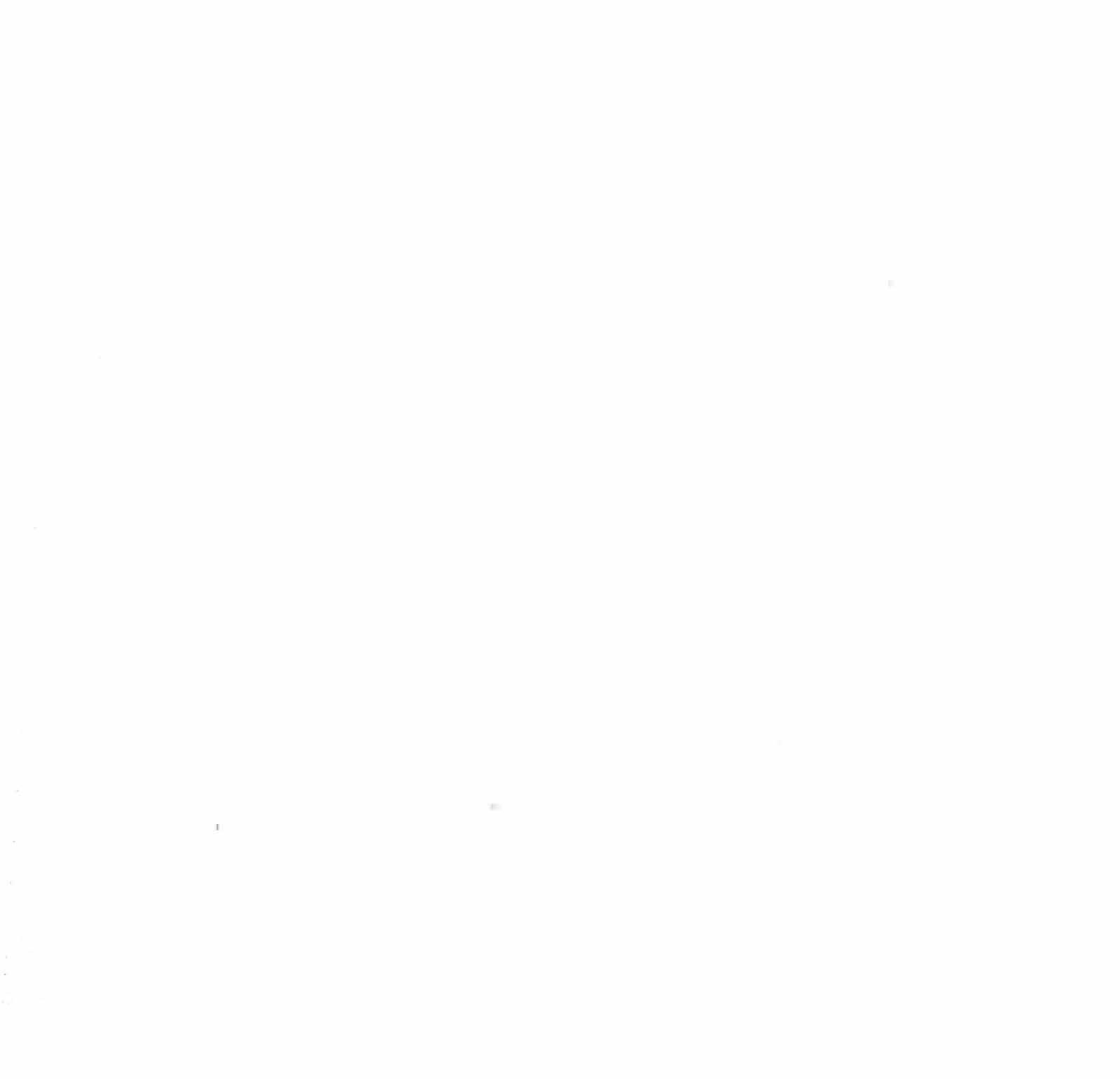
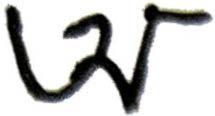
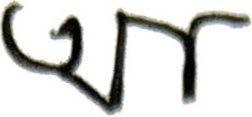
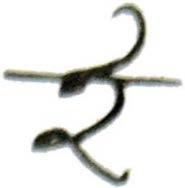
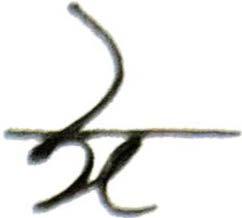
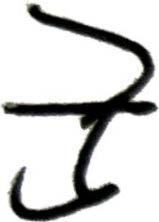
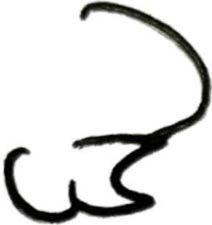
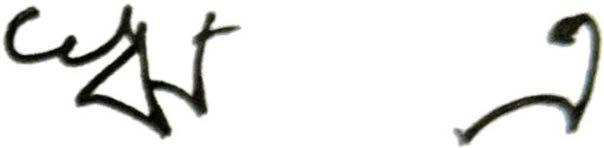
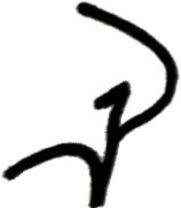
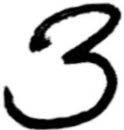
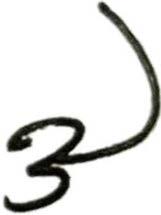
InSVM,allpointsinasupportvectorproblemareconsideredasonevectorwithonemagnitudeand onedirection.Thevectorofthepoint x isprojectedontoanothervector ⃗w thatisperpendiculartothemedian linetoclassifyitaspositiveornegative.Ifthisprojectedvalueisgreaterthanconstant c,thenitisapositive sample.Otherwise,itisanegativeone.TheSVMisdefinedasthedotproductoftwovectors,where x isthe inputand w istheperpendicularvectorofthemedianline,expressedby(1).
IntJArtifIntell ISSN:2252-8938 1145 ters.Theauthorsachieved93.18%accuracyforhandwrittenBanglavowelcharacters,99.5%fordigits,and 92.25%forconsonantcharacters.Reza etal.[18]proposedatransferlearning-basedmodelincombinationwith CNNtorecognizecompositecharactersfrombasiccharacters.Theauthorsusedatransferlearningapproach torecognizecompoundcharactersbytransferringknowledgefrompre-trainedbasiccharacterstoCNN.
Mostofthestudiesmentionedaboveuseneuralnetworkarchitectures,deepneuralnetworkarchitectures,andacombinationofSVMandMLP.VeryfewpapersuseSVMalone.Moreover,thestudiesthathave usedSVMhavealsousedMLPinconjunctionwithit.Theworksthatusedneuralnetworksproposedavery complexarchitectureandaresource-intensivesystem.So,itishightimetoinvestigateasimpleapproachand comparetheresultswiththeexistingapproaches.Withthisbackground,thisstudyproposesaverysimple architectureforrecognizingthevowelsignsoftheBanglaalphabet.
⃗w ⃗x>= c (1)
Thissectiondescribesthestepsrequiredtopreparethedatafortrainingthemachinelearningmodel. Severalstepsarerequiredtopreparethedataset,namelycollectingrawimages,derivingpixeloutput,and creatingamachine-readablefilewithcomma-separatedvalues(CSV).Figure 2 givesanoverviewofthedata preparationsteps. AmachinelearningapproachforBengalihandwrittenvowelcharacterrecognition(ShahrukhAhsan)
Scikit-learnisanundeniablyprominentAIlibrary.ItiswritteninPythonandisintendedtobeproductiveandstraightforward,accessibletonon-specialists,andreusableinvariouscontexts[19].Ithighlightsvariousclustering,classification,andregressioncomputations,includingSVM,k-means,randomforests,andDBSCAN,andalsoworkswiththescientificandnumericalPythonlibrariescalledSciPyandNumPy.FewPython librarieshavestrongexecutioninmostareasofAIcomputation,andScikit-Learnistrulyoutstanding[20].Itis apackagethatprovideseffectivevariantsofanenormousnumberofcommonalgorithms.Scikit-Learnfeatures anew,unified,andstreamlinedAPI,aswellasequallyvaluableandcompleteonlinedocumentation[11].One advantageofthisconsistencyisthatonceweunderstandtheessentialuseandlanguagestructureofScikit-Learn foronetypeofmodel,switchingtoanothermodeloralgorithmisexceptionallyeasy[21].TheScikit-Learn librarymustbeinstalledpriortouseandisessentiallybasedonscientificPython(SciPy).Thisstackincludes NumPy,SciPy,Matpotlib,IPython,Sympy,andPandas.Thelibraryisfocusedonmodelingdata.Itdoesnot focusonloading,controlling,andsketchingdata[22].
3. PROPOSEDMETHOD
Nowthatmanysystemshavealreadybeenestablishedforthetaskofcharacterrecognition,many resultsarecomparedbasedonmaximumprecision[23],[24].TherolethatScikit-learnplaysinclassifying languagesisoutstanding.Scikit-learnestimatorsfollowcertainprinciplestomaketheirbehaviourincreasingly predictive.TheprecisionlevelofScikit-learn,whichworksevenbetterwhencombinedwithothertechniques suchasSVM,candistinguishthecomplexcharactersofanylanguagewithmoreeaseandgivesbetterresults. SomefamouscompilationsofmodelsofferedbyScikit-learnincludeclustering,cross-validation,dimensionalityreduction,ensemblemethods,featureextraction/selection,parametertuning,andmanifoldlearning[25], [26].
3.1. Datapreparation
❒
Theproposedmethodisdividedintotwosegmentsforeasyandunderstandableimplementation.The firstsegmentisaboutthepreparationofthetrainingdataset,whichisuniqueforBengalicharacterrecognition andcomesfromthe”BanglaLekha-Isolated”dataset.Thesecondsegmentisthepredictionpartwhichcanbethe coreofthesystem.Inthissegment,thederiveddatasetistrainedwithaSVMmachinelearningalgorithm,and apredictionmodelisbuilt.Thederiveddatasetusedinthisstudyisdividedintotwoparts,oneofwhichisused fortrainingthesystemandtheotherfortesting.TheproposedmethodusesaverysimplearchitectureforBangla vowelcharacterclassification.Commonimageprocessingtaskssuchasedgedetectionandmatrixgeneration areused,andasimplemachinelearningmodellikealinearsupportvectormachineisused.Althoughthe architectureissimple,theperformanceinclassifyingvowelcharactersisremarkablecomparedtootherworks.
Figure2.Datapreparationstepsfortheproposedmethod
ThereareveryfewBengalidatasetsavailableonline.”BanglaLekha-Isolated”ischosentotakethe firsthandwrittensamplessothatwecantrainourdataset.Theentiresetcontainedasignificantnumberofsamplesrangingfromsimpletomodernhandwritingandcursive.Twohundredsamplesaretakenforindividual charactersforimplementation.Theseselectedsamplesarelaterconvertedintotheindividualmatrixofcharacters.Forthepre-processingoftheexamples,amedianfilterisusedtoremovethenoiseandkeepthevisual edgescomparativelysharp.
3.1.2. Derivingpixeloutput
3.1.3. CreatingtrainingCSVfile
3.2.
ISSN:2252-8938
1146 ❒
TheproposedmethodrequiresaCSVfilecontainingallpixelmatricesofhandwrittensamplecharactersasinputtothemachinelearningalgorithm.ThishasnotyetbeenimplementedforBengalidigitsand characterstothebestoftheauthors’knowledge.Aftergettingthematrixasoutputfromthepixelgeneration ofeachrawimage,itisstoredinasinglelineforeachoutputintheCSVfile.Thislinecontainsalabelthatis thefirstindexorcharacterinthatline.Weseparatethelabelfollowedbythematrixwithacomma.ThisCSV fileisneededforthenextphasetodistinguishthetwo.
Machinelearningmodeltrainingandprediction
3.1.1. Collectinghandwrittensamples
Inthisstep,amachinelearningmodel,namelySVM,istrainedwiththepreparedtrainingdatasetand laterthepredictionisexecutedinthetrainedmodel.AcustomPythonmoduleisdevelopedtoreadthedataset fromtheCSVfileasindividualmatrices.Allimagepixelmatricesfromseparaterowsandtheircorresponding labelsaretrainedseriallyandthetraineddataisstoredinadecisiontreeclassifierasarrays.Theimplemented algorithmtakestherowofinputimagepixelvaluesandmatchesthemwiththetrainedimagesfromthedecision treeclassifier.Thevalueoftheinputimagepixelsandthetrainedimagepixelsarematched,andthelabelto whichthedatamostcloselymatchesisreturnedfromthetrainedimagesandprintedasapredictionofthe inputimage.Ablackandwhiteoutputimageofthe28x28matrixisdisplayedinanewanddifferentwindow. IntJArtifIntell,Vol.11,No.3,September2022:1143–1152
ThePythonimaginglibrary(PIL)isusedforimageprocessing-relatedtasksinthisthesis.Forexample, formanipulationandI/Ooperationofvariousimagefileformats.Someofthefiletypesprocessedinthis systemarejointphotographicexpertsgroup(JPEG),portablenetworkgraphics(PNG),taggedimageformat (TIF),graphicsinterchangeformat(GIF),andportabledocumentformat(PDF).Theincludedmodulescontain definitionsforapredefinedsetoffiltersthatallowtheuseofcolorstrings,imagesharpeners,andahigh-quality downsamplingfilter.SamplefilesinputviaourcustomPythonmoduleareprocessed,andoutputisapixel matrixforindividualalphabets.
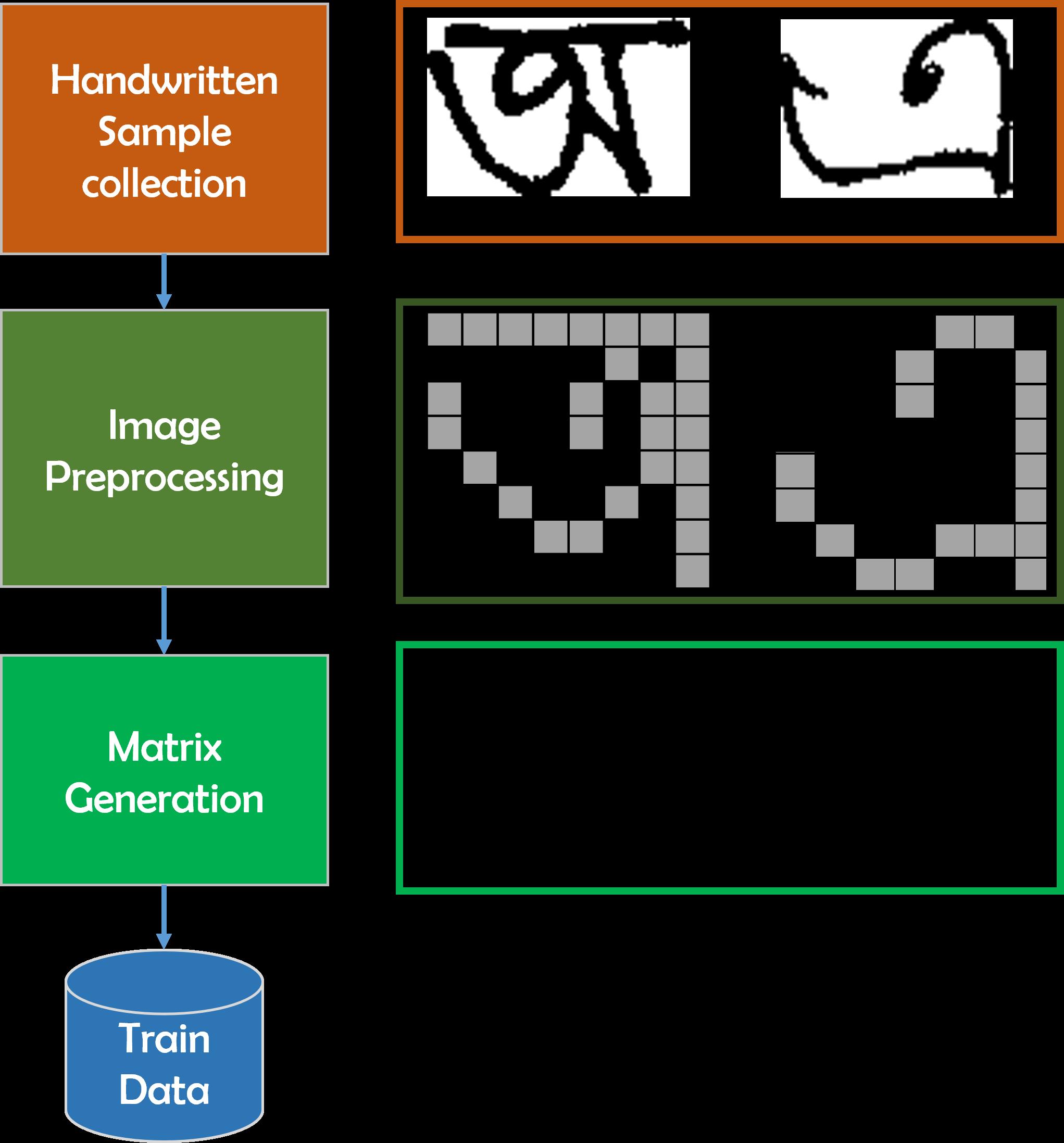
Figure3.Systemtrainingandpredictionstepsoftheproposedmethod
Evaluationmetrics
3.2.1. ImplementationofSVMmodel
Precision,recall,andF1areevaluationmetricsformachinelearningclassificationmodels[33].However,theyaredifferentmethodstomeasuretheaccuracyofamodelfromdifferentangles.Truepositives(TP) andtruenegatives(TN),andfalsepositives(FP)andfalsenegatives(FN)arevaluesthatindicatehowoftena modelcorrectlyorincorrectlypredictsaparticularclass.Forinstance,aclassificationmodelpredictswordsA andB.IfthemodelavoidsmosterrorsinpredictingbothwordsAandB,thenthemodelhashighprecision. IfthemodelmakesnoerrorsinpredictingAasB,thenithashighrecall.However,whatifthemodelexcels atpredictingoneclassbutfailsattheother?Hereitwouldbemisleadingtoconsiderprecisionorrecallin isolation.ThisiswhereF1comesin,whichbalancesandconsidersbothprecisionandrecall.Themetricsare calculatedusing(2)to(4).
IntJArtifIntell ISSN:2252-8938
Pythonisanincrediblyusefulprogramminglanguageonitsown,butwiththehelpofsomemainstream librariesitbecomesanamazingdomain.RunningSVMwithScikit-Learninthisprojectrequiresimporting librarieslikeNumPy[29],Pandas[30],andMatplotlib[31]alongsidethedataset.NumPystoresestimatesof similarinformationtypesinamultidimensionalarray.Scikit-LearnhastheSVMlibrary,whichhasworkedin classesforvariousSVMalgorithms.Thesupportvectorclassifier(SVC)class,whichisincludedintheSVM libraryasSVC,performstheclassificationtask[32].Thisclassrequiresoneimportantparameter,whichisthe kerneltype.GivenasimpleSVM,weessentiallysetthisparameterto”linear”sinceasimpleSVMcanonly characterizelinearlydistinguishableinformation.ThefittechniquefortheclassSVCisinvokedtoprepare thealgorithmforthetrainingdatathatispassedasaparametertothefitstrategy.Tomakepredictions,the predictiontechniqueforclassSVCisused[19].
Precision = TP TP + FP (2) Recall = TP TP + FN (3) F 1=2 ∗ Precision ∗ Recall Precision + Recall (4) AmachinelearningapproachforBengalihandwrittenvowelcharacterrecognition(ShahrukhAhsan)
Figure 3 representstheoverviewofthetrainingandpredictionprocessofthesystem.Inthisstudy,SVMwas usedfortheclassificationtaskbecausetheSVMalgorithmissimpleandfast.Comparedtootheralgorithms suchasartificialneuralnetwork(ANN),CNN,randomforest(RF),thestructureofSVMisverysimpleto implementandfasterthanthementionedapproachesconsideringtheperformanceofthemodel[27, 28].
3.3.
❒ 1147
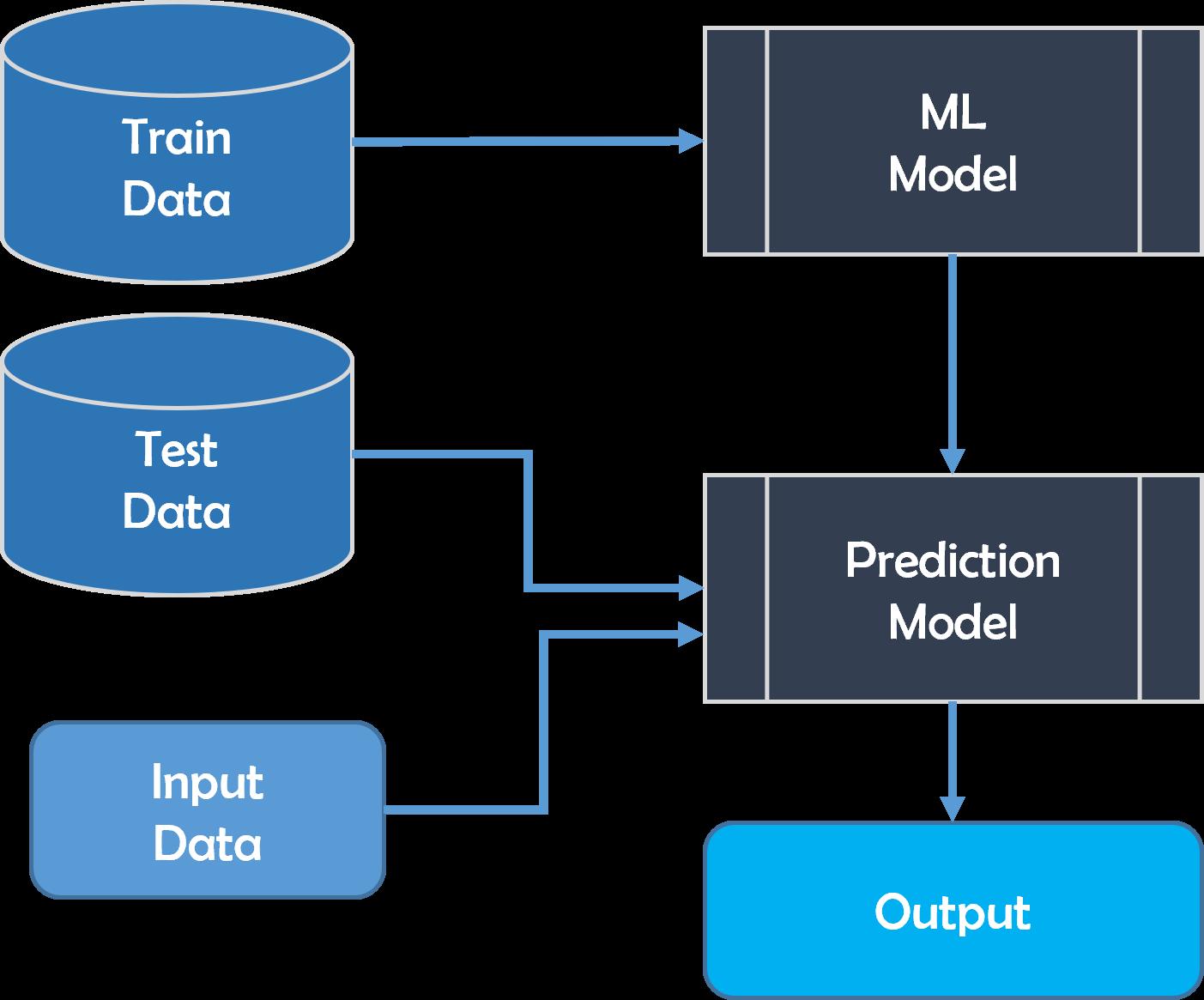
Table1.Obtainedresultofallvowelcharacterswithaccuracy,precision,recallandF1scoresfromthe experiment CharacterAccuracyPrecisionRecallF1 অ 94 61.97 8872.73 আ 92.36 55.56 8065.57 ই 92.36 55.13 8667.19 ঈ
Experimentalsetup
4.
TheSVMisawidelyusedmachinelearningtechniquewithsignificantresultsonhigh-dimensional datasets.SVMshavebeenparticularlystudiedandevaluatedaspixel-basedimageclassifiers[34].Sample imagesareusedtotraintheSVM,andthefinalimageclassificationresultsaresatisfactoryintheexperiment conducted.Theresultsofthisexperimentarebasedonthederivedoutputs.Thepredictionisachievedbythe numberofcorrectpredictionsofthetestimages.AfterprocessingandtrainingourdatasetswithSVMinPython, theresultisextracted.Acorrectpredictionisconsideredonlyifthepredictedvaluematchestheactuallabelof theimage.Tomeasureaccuracy,wedeterminehowmanyofthetestimagesareactuallypredictedcorrectly. Ifthepredictedvaluematchestheactuallabeloftheimage,theimageispredictedcorrectly.Therefore,a variablecountisincrementedforeachcorrectlypredictedimagebasedontheactuallabel.Oncethefinal countvalueisdetermined,itisdividedbythenumberofinputimagesenteredandthenmultipliedby100 toobtainthepercentageaccuracyofthesystem.Theresultisapredictionaccuracybetween87%and94% andanaverageaccuracyof91%forallvowelsigns.However,theworkmentionedin[17]achieved93.18% accuracyinclassifyingvowelcharacters,butitusedaverycomplexdeepneuralnetworkmodel,whereasthe modelweproposedusesaverysimplestructureandthedifferenceinaccuracyisverysmall.Table 1 showsthe experimentalresultsobtainedforallvowelcharacters. 92.73 58.33 7063.64 উ 92.27 60 7867.83 ঊ 90.73 49.23 6455.65 ঋ 87.82 38.03 5444.63 এ 92 53.57 9067.16 ঐ 88.54 43.3 8457.14 ও 87.64 40.63 7853.42 ঔ 90.18 47.5 7658.46 ItcanbeobservedfromTable 1 thattheletterswhichpossesssimilarpatternstoothers(আ,ঊ,ঐ,ঔ ) hasacomparativelylowerrecognitionaccuracy.Eveninreal-worldscenarios,differenthandwritingtypeslead toconfusion,eventothehumaneyeattimes.Withtheexclusionofsomemisclassifications,machinelearning doesreducethis,giventhatsignificantlymoretrainingisdone.Avisualrepresentationoftheoutcomeofthe experimentispresentedinFigure 4,whichshowsthecomparativescoresofaccuracy,precision,recall,and F1scoresobtainedbytheexperimentforallelevenBengalihandwrittenvowelcharacters.Furthermore,the trainingandtestingaccuraciesofthemodelfor50epochsaredepictedinFigure 5. Therefore,fromtheapproach,itcanalsobededucedthatScikit-learnisagoodlibraryforimage classificationandclustering.Itfullytransformstheinputdataforthemachinelearningalgorithmandcompares theparameters.Itisusedinourfeatureextractionandnormalizationmethodforbetterpredictionoftheresult. Overall,theSVMclassificationapproachhasshowngreatpromiseforrecognizingvowelcharactersinBengali handwriting.TheefficiencyofSVMinreadingadatasetlikeoursshowsexcellentresultswithonlyasmall amountoftrainingrequired.Itpromisesevenbetterresultswithmoresupervisedtraining.SVMimplementation inPythoniscomparativelyfastandmakestherecognitiontaskefficient.Althoughaverylargedatasetwasnot trainedforthisstudy,thefirststepwithSVMonacharactermatrixdatasetshowedimpressiveresults.Table 2 showsthecomparativeresultswithsomemodernclassificationtechniques. IntJArtifIntell,Vol.11,No.3,September2022:1143–1152
AllexperimentcodesarewritteninPythonprogramminglanguage,version3.6.5.TheScikit-learn libraryisusedforsystemtraining,dataprocessing,andimageprocessing-relatedtasks.AlaptopwithanAMD Ryzen4800Hprocessor,aGTX1650GPU,and24GBRAMisusedastrainingandtestinghardwarewiththe Windows10ProfessionalEditionoperatingsystem.
1148 ❒ ISSN:2252-8938 3.4.
RESULTSANALYSISANDDISCUSSION
IntJArtifIntell ISSN:2252-8938 ❒ 1149 0 10 20 30 40 50 60 70 80 90 100 অ আ ই ঈ উ ঊ ঋ এ ঐ ও ঔ Precision Recall F1 Accuracy Figure4.Comparativescoresofaccuracy,precision,recallandF1metricsforelevenhandwrittenbengali vowelcharacters,fromlefttoright,eachbarrepresentsprecision,recallandF1scores,thebluelinerepresents theaccuracyscore 0 0.1 0.2 0.3 0.4 0.5 0.6 0.7 0.8 0.9 1 0 5 10 15 20 25 30 35 40 45 50 Accuracy Epoch Train-Acc Test-Acc Figure5.Trainingandtestingaccuracyoftheproposedmodel Table2.Comparisonoftheproposedapproachwithsomeexistingstudies Study Classificationmethod Accuracy Rahman etal. [35] CNN 85.96% Das etal. [36] MLP 85.40% Bhowmik etal. [15] SVM 89.22% Das etal. [2] MLP 79.25% Roy etal. [37] DCNN 90.33% Rahman etal. [38]BWS+FWS+TMS+MLP+MPC88.38% Proposedapproach SVMwithmatrixmapping 91% 5. CONCLUSION ThispaperdescribesanapproachtoimplementSVMinPythonthroughminimaltrainingforhandwrittencharacterrecognitiontasks.ThissystemprovidesamodelforrecognizingBengalivowelsthatcanbe usedefficientlyforanyotherlanguage.Thenewstrategyhasprovidedexcellentresultsandseemspromising. Ithasanaccuracyofupto94%forasinglecharacterandanaverageof91%forallcharacters.Itisexpected thattheresearchwillprovidepositiveinsightintothefewconceptsinvolvedandleadtoadvancesinthefield. Thisisanareaofgreatinterestatpresent,andalargenumberofresearchersarealreadyworkingonit.This methodprovidesgoodresultscomparedtoexistingmethodsofBengalihandwritingrecognitionandismore efficient.Inthefuture,weaimtoimprovefurtheranddevelopnewperspectives.Ourcurrentresearchislimitedtovowelcharacters,butthismethodcanbeimprovedtorecognizeoverwrittenorcompoundcharacters, words,sentences,andevenentiredocuments.Ithasbeenshownthatselectingappropriatefeatureextraction andclassificationmethodsplaysacrucialroleintheperformanceofsimilarsystems.Weplantobecomemore efficientingeneratingresultsandshowingourmodel’scompatibilityinhandwritingrecognition.Inthefuture, wewilltrytomakethissystemmoreprecisetoachievehigheraccuracy. AmachinelearningapproachforBengalihandwrittenvowelcharacterrecognition(ShahrukhAhsan)
[2] N.Das,B.Das,R.Sarkar,S.Basu,M.Kundu,andM.Nasipuri,“Handwrittenbanglabasicandcompoundcharacter recognitionusingMLPandSVMclassifier,” CoRR,2010.[Online].Available:http://arxiv.org/abs/1002.4040.
[5] T.I.Aziz,A.S.Rubel,M.S.Salekin,andR.Kushol,“Banglahandwrittennumeralcharacterrecognitionusing directionalpattern,”in 201720thInternationalConferenceofComputerandInformationTechnology(ICCIT),2017, pp.1–5,doi:10.1109/ICCITECHN.2017.8281820
[3] Y.PerwejandA.Chaturvedi,“Neuralnetworksforhandwrittenenglishalphabetrecognition,” CoRR,2012.[Online]. Available:http://arxiv.org/abs/1205.3966.
[8] M.B.Islam,M.M.B.Azadi,M.A.Rahman,andM.M.A.Hashem,“Bengalihandwrittencharacterrecognition usingmodifiedsyntacticmethod,”in 2ndNationalConferenceonComputerProcessingofBangla(NCCPB-2005), 2005. [9] V.L.SahuandB.Kubde,“Offlinehandwrittencharacterrecognitiontechniquesusingneuralnetwork:Areview,” InternationaljournalofscienceandResearch(IJSR),vol.2,no.1,pp.87–94,2013. [10] A.Pal,“Bengalihandwrittennumericcharacterrecognitionusingdenoisingautoencoders,”in 2015 IEEEInternationalConferenceonEngineeringandTechnology(ICETECH),2015,pp.1–6,doi: 10.1109/ICETECH.2015.7275002.
[7] R.GhoshandP.P.Roy,“Studyoftwozone-basedfeaturesforonlinebengalianddevanagaricharacterrecognition,” in 201513thInternationalConferenceonDocumentAnalysisandRecognition(ICDAR),2015,pp.401–405,doi: 10.1109/ICDAR.2015.7333792.
[4] A.MajumdarandB.Chaudhuri,“Curvelet-basedmultisvmrecognizerforofflinehandwrittenbangla:Amajorindian script,”in NinthInternationalConferenceonDocumentAnalysisandRecognition(ICDAR2007),2007,pp.491–495, doi:10.1109/ICDAR.2007.4378758.
Datainbrief,vol.12,pp.103–107, 2017,doi:10.1016/j.dib.2017.03.035.
JournalofComputerandCommunications,vol.9,no.3,pp.158–171, 2021,doi:10.4236/jcc.2021.93012.
[18] S.Reza,O.B.Amin,andM.Hashem,“Basictocompound:Anoveltransferlearningapproachforbengalihandwritten characterrecognition,”in 2019InternationalConferenceonBanglaSpeechandLanguageProcessing(ICBSLP), 2019,pp.1–5,doi:10.1109/ICBSLP47725.2019.201522. [19] L.Buitinck, etal.,“APIdesignformachinelearningsoftware:experiencesfromthescikit-learnproject,” CoRR, 2013.[Online].Available:http://arxiv.org/abs/1309.0238. [20] J.Briggs,“HowtosetupPythonformachinelearning,”Sep.2021.[Online].Available: https://towardsdatascience.com/how-to-setup-python-for-machine-learning-173cb25f0206. [21] J.VanderPlas,“IntroducingScikit-Learn,” PythonDataScienceHandbook,2016. [22] M.A.Karim, Technicalchallengesanddesignissuesinbanglalanguageprocessing,2013. [23] R.Ghosh,C.Vamshi,andP.Kumar,“RNNbasedonlinehandwrittenwordrecognitionindevanagariandbengali IntJArtifIntell,Vol.11,No.3,September2022:1143–1152
[1] B.Sarma,K.Mehrotra,R.KrishnaNaik,S.R.M.Prasanna,S.Belhe,andC.Mahanta,“Handwrittenassamese numeralrecognizerusinghmmamp;svmclassifiers,”in 2013NationalConferenceonCommunications(NCC),2013, pp.1–5,doi:10.1109/NCC.2013.6488009.
REFERENCES
JournalofMachineLearningResearch,vol.12,no.85,pp.2825–2830,2011.
[15] T.K.Bhowmik,P.Ghanty,A.Roy,andS.K.Parui,“Svm-basedhierarchicalarchitecturesforhandwrittenbangla characterrecognition,” InternationalJournalonDocumentAnalysisandRecognition(IJDAR),vol.12,no.2,pp. 97–108,2009,doi:10.1007/s10032-009-0084-x.
1150 ❒ ISSN:2252-8938
ProgressinIntelligentComputingTechniques:Theory,Practice,and
[12] M.Biswas,R.Islam,G.K.Shom,M.Shopon,N.Mohammed,S.Momen,andA.Abedin,“Banglalekha-isolated:A multi-purposecomprehensivedatasetofhandwrittenbanglaisolatedcharacters,”
[16] S.Basu,N.Das,R.Sarkar,M.Kundu,M.Nasipuri,andD.K.Basu,“AhierarchicalapproachtorecognitionofhandwrittenBanglacharacters,”
[13] K.Maladkar,“6typesofartificialneuralnetworkscurrentlybeingusedinmachinelearning,”Jan.2018. [Online].Available:https://analyticsindiamag.com/6-types-of-artificial-neural-networks-currently-being-used-intodays-technology/. [14] D.S.Maitra,U.Bhattacharya,andS.K.Parui,“CNNbasedcommonapproachtohandwrittencharacterrecognition ofmultiplescripts,”in 201513thInternationalConferenceonDocumentAnalysisandRecognition(ICDAR),2015, pp.1021–1025,doi:10.1109/ICDAR.2015.7333916.
[6] P.Das,T.Dasgupta,andS.Bhattacharya,“Abengalihandwrittenvowelsrecognitionschemebasedonthedetection ofstructuralanatomyofthecharacters,”in Applications 2018,pp.245–252,doi:10.1007/978-981-10-3373-5_24.
PatternRecognition,vol.42,no.7,pp.1467–1484,2009,doi: 10.1016/j.patcog.2009.01.008. [17] T.R.Das,S.Hasan,M.R.Jani,F.Tabassum,andM.I.Islam,“Banglahandwrittencharacterrecognitionusing extendedconvolutionalneuralnetwork,”
[11] F.Pedregosa,G.Varoquaux,A.Gramfort,V.Michel,B.Thirion,O.Grisel,M.Blondel,P.Prettenhofer,R.Weiss, V.Dubourg,J.Vanderplas,A.Passos,D.Cournapeau,M.Brucher,M.Perrot,andÉdouardDuchesnay,“Scikit-learn: machinelearninginpython,”
AmachinelearningapproachforBengalihandwrittenvowelcharacterrecognition(ShahrukhAhsan)
AmericanInternationalUniversityBangladesh(AIUB),Dhaka,Bangladesh.Heiscurrentlyworking asSoftwareQualityAssuranceEngineeratEchoLogyxLtd,Dhaka,Bangladesh.Hisresearchinterest includesComputerVision,ImageProcessing,DataMining,NaturalLanguageProcessing,Machine Learning,andHumanComputerInteraction.Hecanbecontactedatemail:tariknz17@gmail.com.
InternationalJournalofImage,GraphicsandSignalProcessing,vol.7,no.8, pp.42–49,2015,doi:10.5815/ijigsp.2015.08.05.
[34] R.Azim,W.Rahman,andM.F.Karim,“Banglahand-writtencharacterrecognitionusingsupportvectormachine,” InternationalJournalofEngineeringWorks,vol.3,no.6,pp.36–46,2016,doi:10.5281/zenodo.60329.
[26] A.BhowmikandA.E.Chowdhury,“Genreofbanglamusic:amachineclassificationlearningapproach,” AIUB JournalofScienceandEngineering(AJSE)
[27] G.D.Luca,“AdvantagesanddisadvantagesofneuralnetworksagainstSVMs,”2021.[Online].Available: https://www.baeldung.com/cs/ml-ann-vs-svm.
J.Cardoso-Fernandes,A.Teodoro,A.Lima,andE.Roda-Robles,“Evaluatingtheperformanceofsupportvectormachines(svms)andrandomforest(rf)inli-pegmatitemapping:Preliminaryresults,”in ProceedingsVolume2889,High-PowerLasers:SolidState,Gas,Excimer,andOtherAdvancedLasers,2019,p.111560Q,doi: 10.1117/12.2532577. [29] C.R.Harris, etal.,“ArrayprogrammingwithNumPy,” Nature,vol.585,no.7825,pp.357–362,Sep.2020,doi: 10.1038/s41586-020-2649-2. [30] W.McKinney etal.,“Datastructuresforstatisticalcomputinginpython,”in Proceedingsofthe9thPythoninScience Conference,vol.445,2010,pp.51–56,doi:10.25080/Majora-92bf1922-00a. [31] J.D.Hunter,“Matplotlib:A2dgraphicsenvironment,”
,vol.18,no.2,pp.66–72,2019,doi:10.53799/ajse.v18i2.42.
[25]
PatternRecognitionLetters,vol.90,pp.15–21,2017,doi: 10.1016/j.patrec.2017.03.004.
ShahrukhAhsan hascompletedhisBachelorofScienceinComputerScienceandEngineeringat
[37] S.Roy,N.Das,M.Kundu,andM.Nasipuri,“Handwrittenisolatedbanglacompoundcharacterrecognition:A newbenchmarkusinganoveldeeplearningapproach,”
[35] M.M.Rahman,M.Akhand,S.Islam,P.C.Shill,andM.H.Rahman,“Banglahandwrittencharacterrecognition usingconvolutionalneuralnetwork,”
[36] N.Das,S.Basu,R.Sarkar,M.Kundu,M.Nasipuri,andD.K.Basu,“Animprovedfeaturedescriptorforrecognition ofhandwrittenbanglaalphabet,” CoRR,2015.[Online].Available:http://arxiv.org/abs/1501.05497.
AmericanInternationalUniversityBangladesh(AIUB),Dhaka,Bangladesh.Heiscurrentlyworking asOperationsExecutiveatBatteryLowInteractive,Dhaka,Bangladesh.HisresearchinterestincludesComputerVision,ImageProcessing,DataMining,NaturalLanguageProcessingandMachine Learning.Hecanbecontactedatemail:shahrukh.ahsan2009@gmail.com.
BIOGRAPHIESOFAUTHORS
[28]
ComputinginScience&Engineering,vol.9,no.3,pp.90–95, 2007,doi:10.1109/MCSE.2007.55. [32] X.Zhou,J.Li,C.Yang,andJ.Hao,“Studyonhandwrittendigitrecognitionusingsupportvectormachine,”in IOPConferenceSeries:MaterialsScienceandEngineering,2018,vol.452,no.4,p.042194,doi:10.1088/1757899X/452/4/042194. [33] K.P.Shung,“Accuracy,precision,recallorf1,”2018.[Online].Available:https://towardsdatascience.com/accuracyprecision-recall-or-f1-331fb37c5cb9.
[38] A.F.R.Rahman,R.Rahman,andM.C.Fairhurst,“Recognitionofhandwrittenbengalicharacters:anovelmultistage approach,” PatternRecognition,vol.35,no.5,pp.997–1006,2002,doi:10.1016/S0031-3203(01)00089-9.
J.Brownlee,“Agentleintroductiontoscikit-learn:Apythonmachinelearninglibrary,”Apr.2014.[Online].Available:https://machinelearningmastery.com/a-gentle-introduction-to-scikit-learn-a-python-machine-learning-library/.
ShahTarikNawaz hascompletedhisBachelorofScienceinComputerScienceandEngineeringat
[24]
IntJArtifIntell ISSN:2252-8938 ❒ 1151 scriptsusinghorizontalzoning,” PatternRecognition,vol.92,pp.203–218,2019,doi:10.1016/j.patcog.2019.03.030.
B.Purkaystha,T.Datta,andM.S.Islam,“Bengalihandwrittencharacterrecognitionusingdeepconvolutionalneural network,”in 201720thInternationalConferenceofComputerandInformationTechnology(ICCIT),2017,pp.1–5, doi:10.1109/ICCITECHN.2017.8281853.
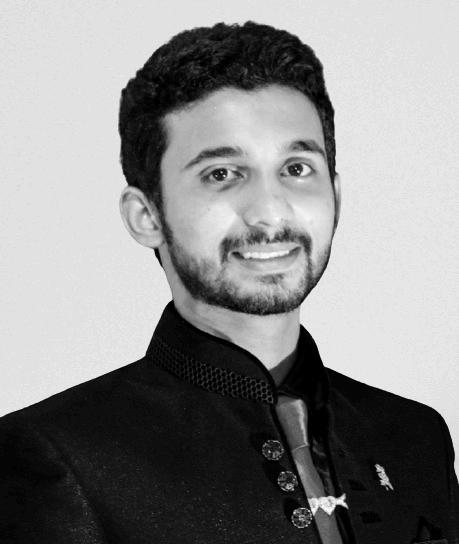

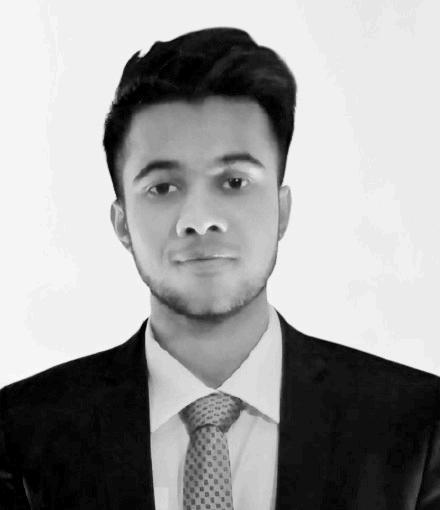

TalhaBinSarwar receivedhisMasterofScienceinComputerScience(MScCS)fromAmericanInternationalUniversity-Bangladesh(AIUB),Dhaka,Bangladesh.Helikewisereceivedhis BachelorofScienceinComputerScienceandEngineering(BScinCSE)fromAmericanInternational University-Bangladesh(AIUB),Dhaka,BangladeshwithacademicdistinguishedhonorMagnaCum Laude.HewasmentionedintheDean’sListhonoraswell.AftertheconsummationofMScCS,he joinedasalecturerintheDepartmentofComputerScience,AmericanInternationalUniversity–Bangladesh(AIUB).HisresearchinterestincludesNaturalLanguageProcessing,DataMining,and MachineLearning.Hecanbecontactedatemail:talhasarwar40@gmail.com.
1152 ❒ ISSN:2252-8938
M.SaefUllahMiah isaPhDcandidateatUniversityMalaysiaPahang(UMP)andis currentlyworkingasaGraduateResearchAssistantattheFacultyofComputing,UMP.Hewas anassistantprofessorintheDepartmentofComputerScience,AmericanInternationalUniversityBangladesh(AIUB).Heiscurrentlyengagedinresearchandteachingactivitiesandhaspractical experienceinsoftwaredevelopmentandprojectmanagement.HeearnedhisMasterofScienceand BachelorofSciencedegreesfromAIUB.Inadditiontohisprofessionalactivities,heispassionate aboutworkingonvariousopensourceprojects.Hismainresearchinterestsaredataandtextmining, naturallanguageprocessing,machinelearning,materialinformaticsandblockchainapplications.He canbecontactedatemail:md.saefullah@gmail.com.
IntJArtifIntell,Vol.11,No.3,September2022:1143–1152
AbhijitBhowmik CompletedhisB.Sc.inComputerScience&Engineeringin2009andM.Sc. inComputerSciencein2011fromtheAmericanInternationalUniversity–Bangladesh(AIUB).CurrentlyheispursuinghisPHDdegreefromUniversityMalaysiaPahanginNLPandMachineLearning.HeisworkingasAssociateProfessorandSpecialAssistant,OfficeofStudentAffairs(OSA)in theDepartmentofComputerScience,AIUB.HisresearchinterestsincludeNLP,MachineLearning, wirelesssensornetworks,videoondemand,softwareengineering,mobile&multimediacommunication,anddatamining.Mr.Bhowmikcanbecontactedatabhijit@aiub.edu.
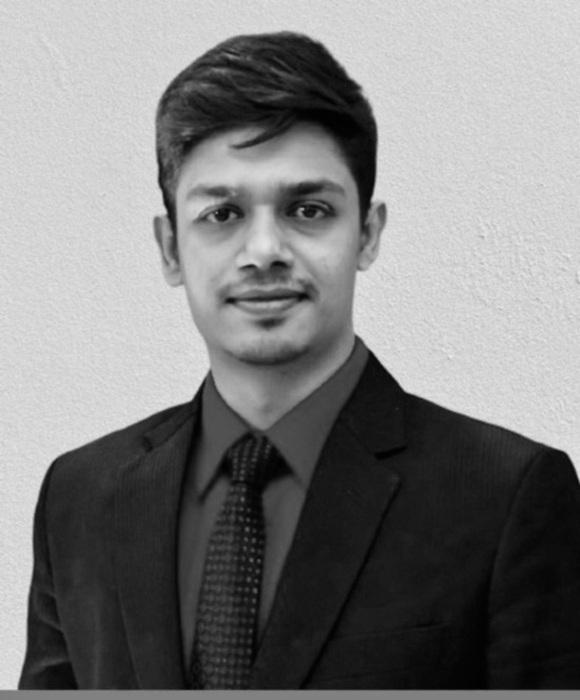


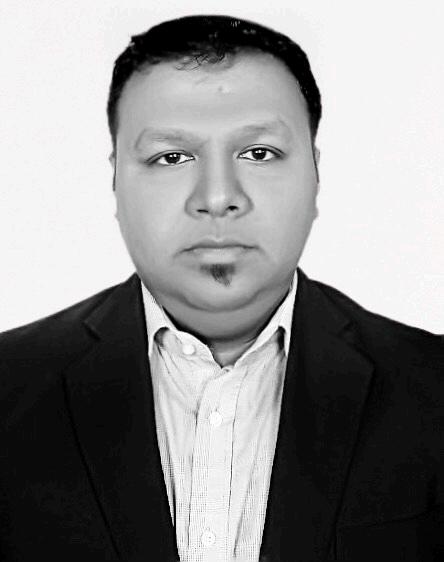



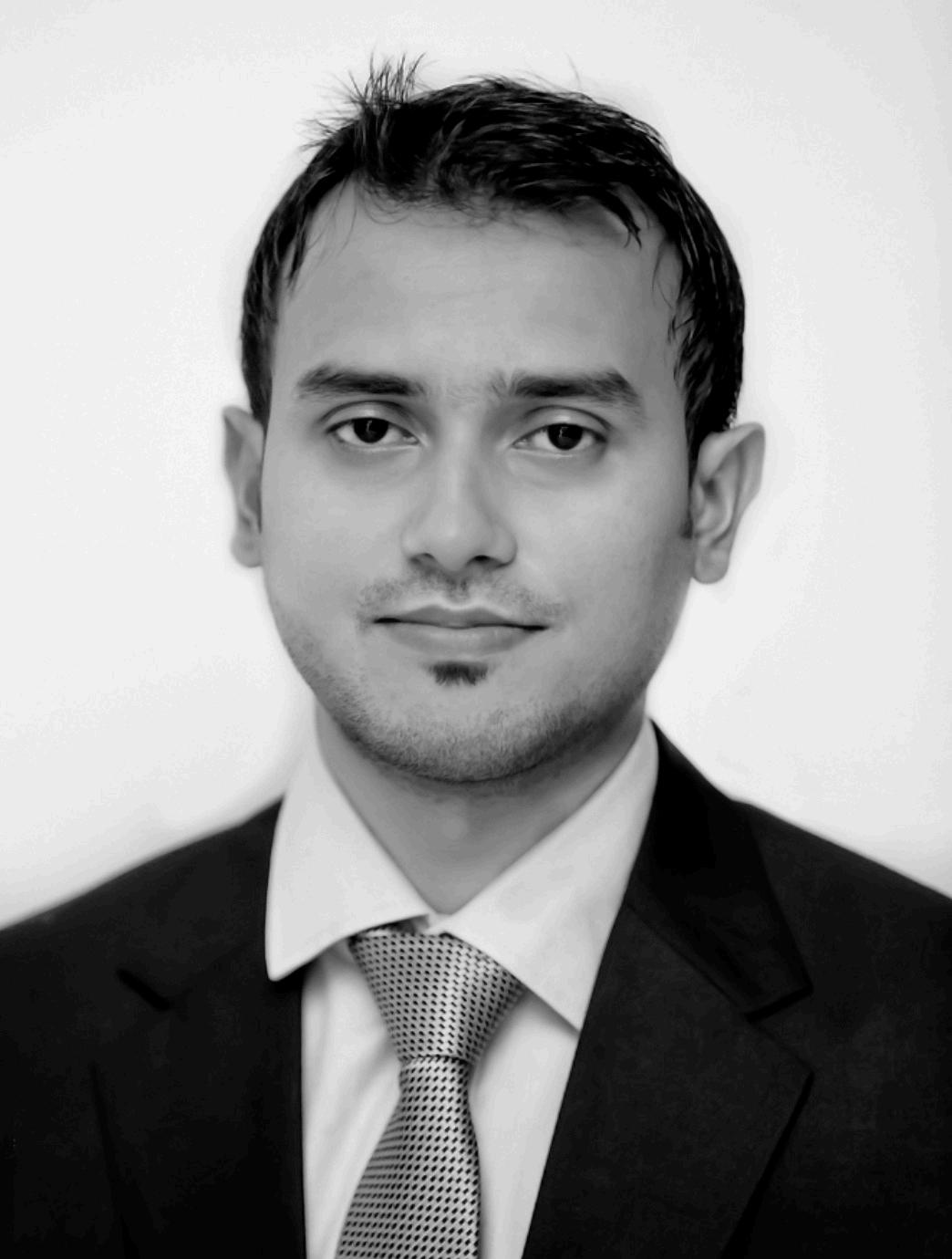


Machine learning of tax avoidance detection based on hybrid metaheuristics algorithms
1. INTRODUCTION Particle swarm optimization (PSO) [1], [2] and genetic algorithm (GA) [3], [4] are two common metaheuristics algorithms that have been widely used to solve different applications of optimization problems. To date, the hybridization between PSO and GA has been very useful to enable high performance searching of the optimization space. Recently, with the broad utilization of machine learning [5] [7], PSO and GA have given their important roles to help the models in achieving highly accurate results for classification, regression, clustering, and forecasting models [8]. PSO and GA can be used in many ways for the machine learning models, either individually [9] or in combination [10] to automate the important tasks in the machine learning pipelines. This paper presents the roles of PSO GA hybridization as an optimization tool for machine learning features selection. It is anticipated in this research that the PSO GA hybridization [11], [12] can be very useful to be deployed for the application of machine learning, mainly for the problem that involved real cases dataset. The results of machine learning models from our previous studies in [13], [14] on the tax avoidance detection
Article history: Received Aug 9, 2021 Revised May 31, 2022 Accepted Jun 18, 2022 This paper addresses the performances of machine learning classification models for the detection of tax avoidance problems. The machine learning models employed automated features selection with hybrid two metaheuristics algorithms namely particle swarm optimization (PSO) and genetic algorithm (GA). Dealing with a real dataset on the tax avoidance cases among companies in Malaysia, has created a stumbling block for the conventional machine learning models to achieve higher accuracy in the detection process as the associations among all of the features in the datasets are extremely low. This paper presents a hybrid meta heuristic between PSO and adaptive GA operators for the optimization of features selection in the machine learning models. The hybrid PSO GA has been designed to employ three adaptive GA operators hence three groups of features selection will be generated. The three groups of features selection were used in random forest (RF), k nearest neighbor (k NN), and support vector machine (SVM). The results showed that most models that used PSO GA hybrids have achieved better accuracy than the conventional approach (using all features from the dataset). The most accurate machine learning model was SVM, which used a PSO GA hybrid with adaptive GA mutation.
Keywords: Automated features selection Genetic
Article Info ABSTRACT
This is an open access article under the CC BY SA license. Corresponding Author: Rahayu Abdul Rahman Faculty of Accountancy, Universiti Teknologi MARA Perak Branch, Malaysia Email: rahay916@uitm.edu.my
Suraya Masrom1 , Rahayu Abdul Rahman2 , Masurah Mohamad1, Abdullah Sani Abd Rahman3 , Norhayati Baharun1 1Faculty of Computer and Mathematical Sciences, Universiti Teknologi MARA, Perak Branch, Malaysia 2Faculty of Accountancy, Universiti Teknologi MARA, Perak Branch, Malaysia 3Faculty of Sciences and Information Technology, Universiti Teknologi PETRONAS, Perak, Malaysia
IAES International Journal of Artificial Intelligence (IJ AI) Vol. 11, No. 3, September 2022, pp. 1153 1163 ISSN: 2252 8938, DOI: 10.11591/ijai.v11.i3.pp1153 1163 1153
Journal homepage: http://ijai.iaescore.com
TaxParticleMachinealgorithmlearningswarmoptimizationavoidance
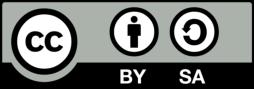
In PSO, the particles consists of a D dimensional position vector and a D dimensional velocity vector as shown in Figure 1. Each position of an ith particle can be represented as x while the ith particle velocity can be denoted as v. In each iteration t, every velocity of each particle in the population has to move towards the best fitness based on the previous experience. The calculation to update the next velocity of each particle i is determined by (1).
2. RESEARCH METHOD 2.1. PSO, GA, and PSO GA hybrids
ISSN: 2252 8938 Int J Artif Intell, Vol. 11, No. 3, September 2022: 1153 1163 1154were undesirable due to the problem of very weak correlation among the dataset features. Although data engineering [15] exercises can be conducted to improve the knowledge extrapolations from the dataset it is expected that automating the features selection without original data manipulation can be more helpful. The contributions of this paper are multifaceted. Firstly, the machine learning models can be used to resolve the problem of tax avoidance issue that occurs in Malaysia. Although corporate tax is the highest contributor to government revenues, it can signify the biggest burden of the cost incurred by the firms. Thus, managers attempt to minimize the tax liability by using various legal and illegal strategies including tax avoidance plans. Therefore, the development of the corporate tax avoidance model has long been seen as significant to the tax authorities and business community. Research in machine learning classification models for detecting tax avoidance is infrequently in the current literature. This work was initially inspired by the machine learning tax avoidance prediction research conducted by [16] that used logistic regression, decision tree, and random forest machine learning algorithms. Based on our previous studies, using automated machine learning has given more advantages compared to manual machine learning configurations [14] but this approach only used genetic programming (GP) algorithm to optimize the features selections of the tax avoidance dataset. To get a research report on PSO with adaptive GA operators is difficult from the literature neither in tax avoidance nor in other machine learning applications.
������ =����(�� 1) +(��1 ∗��1 ∗(���������� ����(�� 1) ))+(��2 ∗��2 ∗(���������� ����(�� 1)) (1) where ��1 and ��2 are the acceleration coefficient with positive constants and the ��1 and ��2 are two different numbers generated randomly between 0 and 1.
Thirdly, this paper provides a research report that extends the study on a problem related to PSO premature convergence. The results reported in this paper present the benefits of hybridizing adaptive GA operators in PSO to resolve the premature convergence in achieving the most optimal results. The single PSO faced an imbalanced problem between the exploration and exploitation searching direction [17]. The GA has some operators when used appropriately in the PSO, and can be useful to enable exploration and exploitation steadiness.
������ =������ +������ (2) where ������ is the updated position at the current iteration and ������ is the new velocity that was calculated from (1). The proses of updating each particle’s velocity and position will be repeated until the optimization objective has been met or until the maximum iteration has been reached. Early or premature convergence in PSO is a common problem that occurred when the PSO ends this process before reaching the optimal
PSO and GA are among the popular nature inspired metaheuristics algorithms that use the current state of the search performances to determine the next search direction. Both algorithms are from the population based meta heuristics [18], [19]. The PSO mimics the cognitive and social behaviors of birds flocking [20] while GA simulates the evolution of creatures [21]
The (1) affects accelerating particles toward the weighted sum of its personal best position pbest and global best position of the swarm (gbest) from the previous particle position ����(�� 1) . After updating each particle velocity, (2) will be implemented for updating the position of each particle i at each iteration t
The second contribution of this paper is on the deployment of hybridization approach in machine learning. As features selection is critical in machine learning, different approaches of PSO GA features selection have been intensively tested in different machine learning models. Additionally, the problem that exists in the collected dataset related to the tax avoidance cases among government link companies (GLCs) in Malaysia is very low correlations among the independent variables (IVs). Removal of some features from the IVs without adequate research might lower the classification accuracy of the machine learning models. Therefore, the optimization of features selection based on the proposed PSO GA hybrids was expected to be useful in the tax avoidance application.
Int J Artif Intell ISSN: 2252 8938 Machine learning of tax avoidance detection based on … (Suraya Masrom) 1155 objective. To resolve the problem of premature convergence, hybridization with GA operators (selection, crossover, and mutation) is expected to be helpful in PSO.
Figure 1. The flowchart of the PSO algorithm
Figure
Different from PSO which implements individual repositioning, GA uses individual reproduction. As depicted in Figure 2, a proportion of the existing population in GA will be chosen to produce a new generation during each successive reproduction. The proportion of individual of chromosome from the current population is implemented with a fitness based selection. 2 The flowchart of the GA algorithm
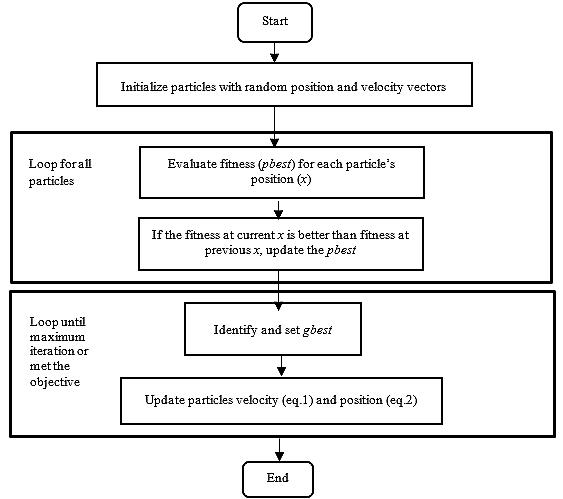
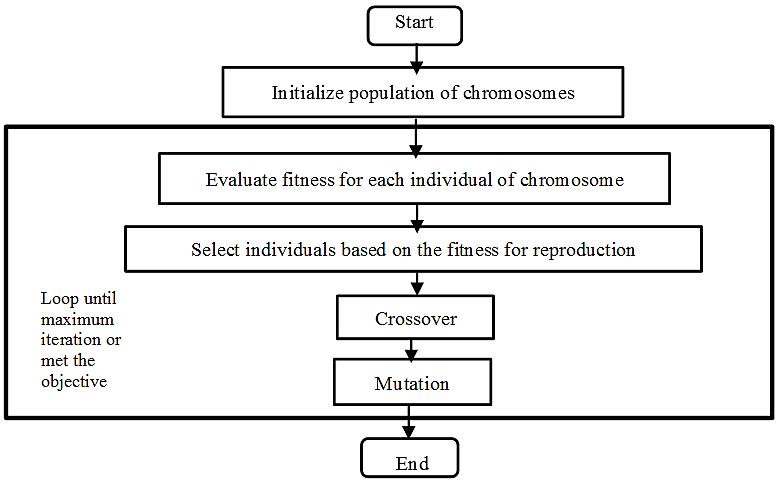
The dataset was collected from the GLCs in Malaysia from the period between 2010 to 2016. This study used effective tax rates (ETR) to denote the tax avoidance, which was formulated based on the ratio of the total tax expenses with the total income before tax. The GLCs companies can be classified as tax avoidance firms if the ETR is smaller than the corporate statutory tax rates [13]. A detailed description of the features can be referred in [13]. As depicted in Figure 3, all the features have a very weak correlation to the ETR mainly from the finance indicator (IND Finance). Particularly, the Muslim Chief Executive Officer (MusCEO) and Audit Firm variables have no significance at all to the ETR. However, in machine learning models, all the features even with low or zero correlation to the ETR, when combined may contribute some degree of knowledge to the classification models. But the question raised is related to which feature combination has optimal contribution to help the models produce the best accuracy results. Manual features selection is quite impractical to involve all the possible sets of combinations. Therefore, automated features selection was implemented for the machine learning models.
After the general best fitness of the PSO has been identified in a particular loop, the selection operator from GA will be executed. Then, the adaptive crossover, adaptive mutation, or both adaptive crossover mutation will be implemented. Figure 4 presents the flowchart of the proposed PSO GA hybrids that used adaptive GA operators. To examine the effect of each adaptive operator, three types of PSO GA hybrids for the features selection have been developed. The first approach used both adaptive operators (PSO ACM), the second used adaptive crossover (PSO AC), and the third used adaptive mutation (PSO AM). Based on Figure 5, after randomly selecting two particles, the adaptive crossover will be implemented followed by adaptive mutation for the PSO ACM.
ISSN: 2252 8938 Int J Artif Intell, Vol. 11, No. 3, September 2022: 1153 1163 1156
The crossover is an operation that combines two chromosomes/parents to produce a new chromosome/offspring. The new offspring should be better than the parents. Moreover, the mutation is the GA operator that alters one or more gene values in a chromosome from its current state. Hybridization of PSO with GA is not a new technique in meta heuristics algorithms. They can be combined in a variety of approaches either with low level or high level hybridizations [22]. High level hybridization allows them to be linked without internal algorithm modification such as executing the algorithm separately in parallel or sequences. Otherwise, the low level hybridization involves internal flow amendment of the algorithms individually or in combinations. The proposed PSO GA in this paper is a low level hybridization. 2.2. The tax avoidance dataset
2.3. The proposed PSO GA hybrids
Figure 3 The pearson correlation of each feature to the ETR
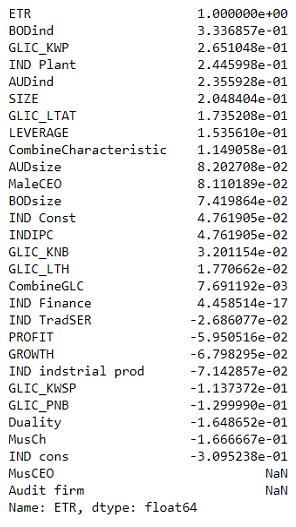
In the PSO AC, two particles will be selected to generate new off string particles while in the PSO AM, only one particle is selected for adaptive mutation. Figure 5 presents the pseudocodes of PSO AC. The adaptive crossover in PSO AC executes the crossover of two particles that are randomly selected according to the adaptive crossover probability Cp. The Cp of all particles in line 2 used the adaptive technique introduced by in Qin et al. [23], which introduced the formulation of individual search ability (ISA). As given in (3), the ISA formula calculates the ratio of distance for particle i in the d dimension.
Update particles velocity (eq.1) position (eq.2)
If the fitness at current x is better than fitness at previous x, update the pbest
and
��������(��) = |����(��) ������������(��)| |������������(��) ������������|+�� (3) where ������(��) denotes the recent position of the ith particle. The personal best position of the ith particle in the current iteration is denoted as ������������(��). Then, ������������ is the latest global best position of the entire swarm, a nd �� is a positive constant that is nearest to zero. The numerator is a distance from the personal best to the current position while denominator is the result of distance from the global best to the personal best.
Identify and set gbest AdaptiveSelectioncrossoverAdaptivemutation
Start Initialize
Figure 5 The algorithm for adaptive crossover particles with random position and velocity vectors fitness (pbest) for each particle’s position (x)
Evaluate
Figure 4 The proposed PSO GA hybrids
maximumLoopsparticlesforLoopalluntiliterationormettheobjective
Stop
Int J Artif Intell ISSN: 2252 8938 Machine learning of tax avoidance detection based on … (Suraya Masrom) 1157
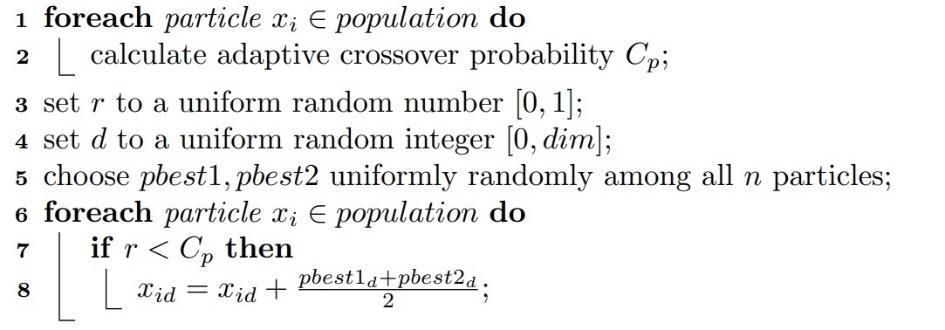
Table 2. The machine learning algorithms and their parameters configuration Machine learning call and parameters parsing in Python svc = SVC(C=2,kernel='poly') knn = KNeighborsClassifier (n_neighbors = 8) rf= RandomForestClassifier(estimator’s=100)
Table 1. General experiment setting for the PSO hybrid with adaptive GA Attribute Value Particles number, n 10, 20, 30 Particle dimension, dim Number of features (28) Personal learning rate, c1 0.9 Social learning rate, c2 0.9 Iterations number, i 100 1000
If the fitness is better than the global best fitness, then the position vector x is also saved for the global best gbest. Finally, the particle’s velocity and position are updated with (1) and (2) until the termination condition is satisfied. The general experiment setting is given in Table 1.
Regardless of each algorithm, the number of function evaluations is the number of particles multiply the number of iterations. Therefore, for the 30 particles with 1,000 iterations, the total number of evaluations is 30,000. It is important to identify the suitable number of particles and iterations based on the input dataset.
The dimension size of each particle is the total number of features (28). Each particle stores the features’ column id (a1..an) of the dataset (converted to a data frame in Python). The objective fitness function is to get the maximum accuracy value from the machine learning that will use the randomized features selection. When the fitness is better than the previous pbest, the latest position vector is saved for the particle.
ISSN: 2252 8938 Int J Artif Intell, Vol. 11, No. 3, September 2022: 1153 1163 1158
The machine learning algorithms used in this research were support vector machine (SVM), random forest (RF), and K nearest neighbor (k NN). Table 2 lists the classification machine learning algorithms with the parameters setting implemented in Python codes. The hardware for running the machine learning models is a Lenovo notebook Intel i7 7th generation processor with 16 GB RAM.
The threshold r value has been set with a random number within an interval of zero and one, which the value has to be compared with the particle’s probability Cp of crossover in deciding whether this particle at its random position d should be modified using the crossover operator or not. The crossover operator in line 8 was adopted from [24]. Furthermore, the adaptive mutation also used the ISA scheme for the mutation probability Mp to decide which particles should be mutated. As shown in Figure 4 at line 5, the mutation only chooses one pbest particle from the uniformly random n particles. Furthermore, the operation in line 8 in Figure 5 was replaced with (4) ����(��) =����(��) +����������������(��) (4) where the Gaussian function [25] returns a random value from the range of the particle dimension and the �� value is in between 0.1 times of the particle dimension. Referring back to Figure 4, the population of the PSO is a set of particles that represent the Malaysian GLCs records listed in the year 2010 2016. Figure 6 is the particle representation for the features.Figure 6. The PSO solution representation
The preliminary experiments have been conducted with particles 10, 20 and 30. The iterations number to be observed is from 100 to 1000. Figure 7 presents the implementation of features selection optimization with the proposed PSO GA in the machine learning classification model for tax avoidance detection.

Figure 7. The PSO GA features selection in the machine learning models
The results are divided into two. The first results are in plotted graphs to present the accuracy of each features selection approach (PSO AM, PSO AC, PSO ACM) in the machine learning models according to the number of particles (population size at n=10,n=20, n=30). The y axis is the accuracy percentage of models and the x axis presents several iterations (100 1000). From the graphs, the suitable number of particles n that can generate the most accurate result and the number of iterations i of when the algorithm was stagnated can be depicted. Furthermore, the second results compare the accuracy results of machine learning models that used the three features selection approaches at the selected n and i, the single PSO without hybridization, and the conventional manual features selection (all features). Figure 8 presents the graphs of accuracy results from the PSO AM features selection approach in the SVM, k NN, and RF at a different number of iterations.
Figure 8. The PSO AM features selection in the k NN, SVM, and RF
Int J Artif Intell ISSN: 2252 8938 Machine learning of tax avoidance detection based on … (Suraya Masrom) 1159
3. RESULTS AND DISCUSSION
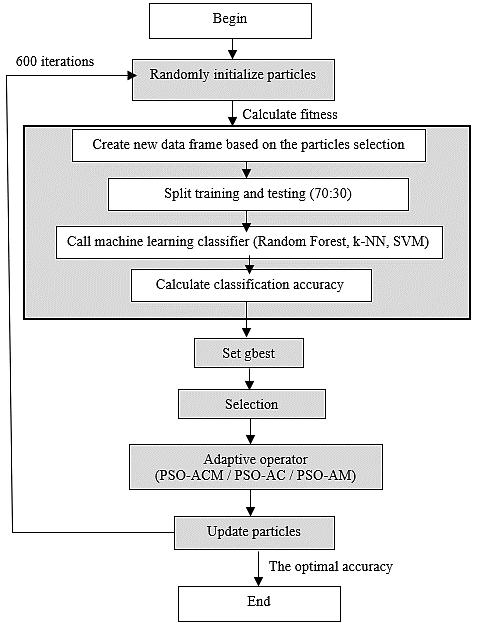
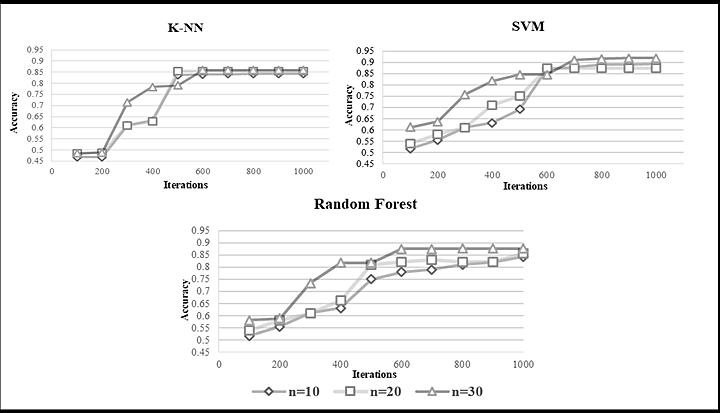
Figure 9. The PSO AC features selection in the k NN, SVM, and Random Forest
The results in Figure 8 show that most of the machine learning models converged for optimal accuracy at 600 iterations. It can be seen in all the machine learning models that the convergences have occurred earlier with small populations (n=10, n=20) compared to n=30 mainly in SVM and k NN. The 30 number of particles in k NN and RF, have shown prior convergence at 600 iterations compared to 700 iterations in SVM. In RF, the algorithm tends to converge at 500 iterations with small populations but slight improvements have been shown at 1,000 iterations. Even with n=10, a good accuracy level (more than 75%) can be achieved by using the PSO AM features selection mainly in k NN and SVM. When the n=30, all the models have produced above 85% of accuracy level after 600 number iterations. Furthermore, Figure 9 presents the convergence rates of PSO AC. By using adaptive crossover in PSO AC features selection, most of the machine learning models even with n=30 have shown faster convergences (less than 600) than the adaptive mutation (PSO AM) but it decreased the accuracy result of all machine learning models. In RF, populations n=10 and n=20 have started to achieve the stagnation level at 300 iterations with an accuracy of less than 60%. Overall, all models with PSO AC generated accuracy results of less than 80% when reached stagnation levels at iterations less than 600. In k NN, even at the late convergence (iterations 600) has occurred for n=30, the optimal accuracy remained lower than the PSO AM (less than 80%). Therefore, hybridizing the PSO with adaptive crossover does not appear to have much benefit in the accuracy of the machine learning models. However, as presented in Figure 10, the inclusion of adaptive mutation together with the adaptive crossover can be useful to improve the results of PSO AC. The convergence rates presented in Figure 10 are between 500 to 700 iterations (SVM and RF took 500 while k NN with 700), and most of them were longer than PSO AM but shorter than PSO AC. Most of the models have generated better accuracy than PSO AC (more than 85%). In SVM, n=20 and n=30 present the same number of iterations for the convergence at 500 and slightly late (600) with n=10. In k NN, the models converged at 700 for all numbers of particles and the accuracy results are above 80%. In RF, the models have taken 500 iterations to converge with slight differences in accuracy levels (minimum above 75% and maximum below 90%). In general, most of the machine learning models with the PSO GA hybrids can achieve optimal accuracy results with 30 particles at iteration less or equal to 600. The results of each model with this configuration can be achieved within a reasonable time (less than 60 minutes). Therefore, it is important to compare the results of all models with the different PSO GA hybrid approaches at this setting (n=30, i=600) with the manual approach that used all features. Additionally, it is interesting to compare all results with the model that used a single PSO without hybridization as listed in Table 3.
Generally, most models that used PSO GA hybrids have achieved better accuracy than the conventional ones that used all features as well as with the use of a single PSO. SVM model can reach up to
ISSN: 2252 8938 Int J Artif Intell, Vol. 11, No. 3, September 2022: 1153 1163 1160
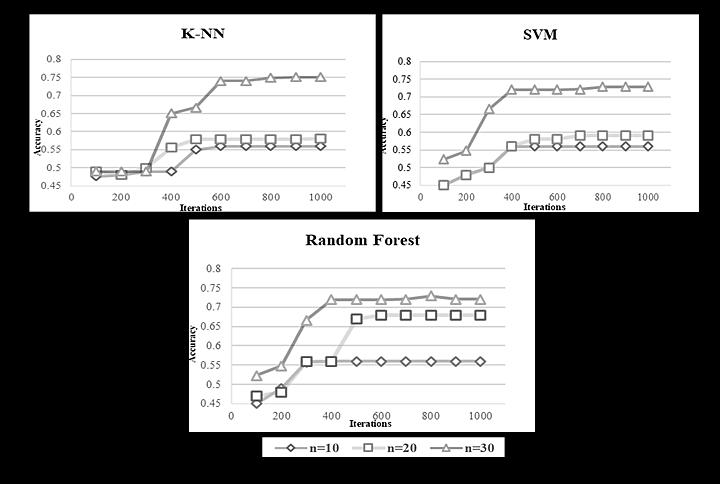
ACKNOWLEDGEMENTS
To the best of our knowledge on the state of the art of features selection based on PSO GA, the adaption of adaptive parameterization has not yet been introduced in the machine learning model. The literature revealed that adaptive GA operators can make significant improvements regarding the PSO algorithm’s performance when applied to many kinds of real life problems. Therefore, this study attempts to introduce several PSO hybrids combined with adaptive GA operators. The results from the proposed approaches present additional advantages to the machine learning models when tested with a real dataset of tax avoidance problems. This research has opened up many research opportunities related to automated features selection of machine learning models as well as to the tax avoidance problem. For example, the proposed PSO GA can be furtherly improved with different approaches to parameterizations within the PSO and the GA. Besides adaptive parameterizations, time vary formulations can be other options for calculating the mutation and crossover rates. Furthermore, these varieties of PSO GA hybrid approaches can be used not just for the features selections but also for the automated parameters tuning of the machine learning models. On the tax avoidance problem, different factors of ETR and different types of firms or businesses are also important to be explored. Last but not least, the variety of approaches from the PSO GA hybrid can be applied or tested in any kind of application or problem domain.
4. CONCLUSION
Int J Artif Intell ISSN: 2252 8938 Machine learning of tax avoidance detection based on … (Suraya Masrom) 1161
We acknowledge the Ministry of Higher Education Malaysia and the Universiti Teknologi MARA that have given full support to this project under the FRGS grant number 600 IRMI/FRGS 5/3 (208/2019).
Figure 10. PSO ACM features selection in the k NN, SVM, and RF Table 3. The accuracy results of the machine learning with different features selection approaches PSO AM PSO AC PSO ACM Single PSO All features SVM 0.91 0.72 0.85 0.75 0.83 k NN 0.86 0.75 0.75 0.70 0.72 Random Forest 0.88 0.72 0.86 0.78 0.83
91% of accuracy with the use of PSO AM, which is the most outperformed machine learning model for the tax avoidance problem. Including both mutation and crossover PSO ACM improved the performance of the machine learning models that used crossover (PSO AC) in all cases. Moreover, the use of single PSO, as well as PSO AC, appeared to be no benefit to the machine learning models as the accuracy results are lesser than the conventional approach that used all features.
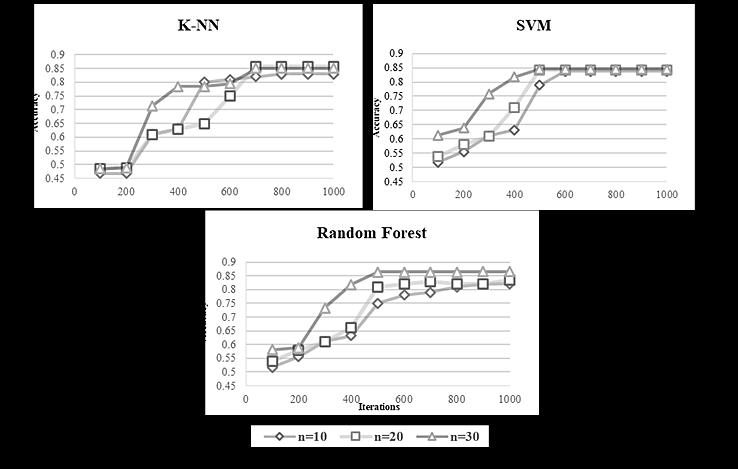
[13] R. A. Rahman, S. Masrom, and N. Omar, “Tax avoidance detection based on machine learning of malaysian government linked companies,” Int. J. Recent Technol. Eng., vol. 8, no. 2S11, pp. 535 541, Nov. 2019, doi: 10.35940/ijrte.B1083.0982S1119.
ISSN: 2252 8938 Int J Artif Intell, Vol. 11, No. 3, September 2022: 1153 1163 1162
[9] T. Khadhraoui, S. Ktata, F. Benzarti, and H. Amiri, “Features selection based on modified PSO algorithm for 2D face recognition,” in 2016 13th International Conference on Computer Graphics, Imaging and Visualization (CGiV), Mar. 2016, pp. 99 104, doi: 10.1109/cgiv.2016.28.
Associate Professor Ts. Dr Suraya Masrom is the head of the Machine Learning and Interactive Visualization (MaLIV) Research Group at Universiti Teknologi MARA (UiTM) Perak Branch. She received her Ph.D. in Information Technology and Quantitative Science from UiTM in 2015. She started her career in the information technology industry as an Associate Network Engineer at Ramgate Systems Sdn. Bhd (a subsidiary of DRB HICOM) in June 1996 after receiving her bachelor’s degree in computer science from Universiti Teknologi Malaysia (UTM) in Mac 1996. She started her career as a lecturer at UTM after receiving her master’s degree in computer science from Universiti Putra Malaysia in 2001. She transferred to the Universiti Teknologi MARA (UiTM), Seri Iskandar, Perak, Malaysia, in 2004. She is an active researcher in the meta heuristics search approach, machine learning, and educational technology. She can be contacted at email: suray078@uitm.edu.my.
[15] A. Zheng and A. Casari, Feature engineering for machine learning: principles and techniques for data scientists. O’Reilly Media, Inc., 2018. [16] J. Lismont et al., “Predicting tax avoidance by means of social network analytics,” Decis. Support Syst., vol. 108, pp. 13 24, Apr. 2018, doi: 10.1016/j.dss.2018.02.001. [17] A. Hussain and Y. S. Muhammad, “Trade off between exploration and exploitation with genetic algorithm using a novel selection operator,” Complex Intell. Syst., vol. 6, no. 1, pp. 1 14, Apr. 2019, doi: 10.1007/s40747 019 0102 7.
[2] P. Matrenin et al., “Generalized swarm intelligence algorithms with domain specific heuristics,” IAES Int. J. Artif. Intell., vol. 10, no. 1, p. 157, Mar. 2021, doi: 10.11591/ijai.v10.i1.pp157 165. [3] K. Kamil, K. H. Chong, H. Hashim, and S. A. Shaaya, “A multiple mitosis genetic algorithm,” IAES Int. J. Artif. Intell., vol. 8, no. 3, pp. 252 258, Dec. 2019, doi: 10.11591/ijai.v8.i3.pp252 258.
[22] C. Blum and A. Roli, “Hybrid metaheuristics: an introduction,” in Hybrid Metaheuristics, Springer Berlin Heidelberg, 2008, pp. 1 30. [23] Z. Qin, F. Yu, Z. Shi, and Y. Wang, “Adaptive inertia weight particle swarm optimization,” in International conference on Artificial Intelligence and Soft Computing, Springer Berlin Heidelberg, 2006, pp. 450 459. [24] D. Chen and C. Zhao, “Particle swarm optimization with adaptive population size and its application,” Appl. Soft Comput., vol. 9, no. 1, pp. 39 48, Jan. 2009, doi: 10.1016/j.asoc.2008.03.001. [25] A. Sarangi, S. Samal, and S. K. Sarangi, “Analysis of gaussian & cauchy mutations in modified particle swarm optimization algorithm,” 019 5th Int. Conf. Adv. Comput. Commun. Syst., pp. 463 467, 2019.
[18] J. Sato, T. Yamada, K. Ito, and T. Akashi, “Performance comparison of population based meta heuristic algorithms in affine template matching,” IEEJ Trans. Electr. Electron. Eng., vol. 16, no. 1, pp. 117 126, 2021, doi: https://doi.org/10.1002/tee.23274.
[19] Z. Beheshti and S. M. H. Shamsuddin, “A review of population based meta heuristic algorithms,” Int. J. Adv. Soft Comput. Appl., vol. 5, no. 1, 2013. [20] S. S. Aote, M. M. Raghuwanshi, and L. G. Malik, “Improved particle swarm optimization based on natural flocking behavior,” Arab. J. Sci. Eng., vol. 41, no. 3, pp. 1067 1076, Mar. 2016, doi: 10.1007/s13369 015 1990 5. [21] S. Katoch, S. S. Chauhan, and V. Kumar, “A review on genetic algorithm: past, present, and future,” Multimed. Tools Appl., vol. 80, no. 5, pp. 8091 8126, Feb. 2021, doi: 10.1007/s11042 020 10139 6.
[7] A. M. Abdu, M. M. M. Mokji, and U. U. U. Sheikh, “Machine learning for plant disease detection: an investigative comparison between support vector machine and deep learning,” IAES Int. J. Artif. Intell., vol. 9, no. 4, pp. 670 683, Dec. 2020, doi: 10.11591/ijai.v9.i4.pp670 683.
[8] O. Almomani, “A feature selection model for network intrusion detection system based on PSO, GWO, FFA and GA algorithms,” Symmetry (Basel)., vol. 12, no. 6, p. 1046, Jun. 2020, doi: 10.3390/sym12061046.
[10] A. Benvidi, S. Abbasi, S. Gharaghani, M. D. Tezerjani, and S. Masoum, “Spectrophotometric determination of synthetic colorants using PSO GA ANN,” Food Chem., vol. 220, pp. 377 384, Apr. 2017, doi: 10.1016/j.foodchem.2016.10.010.
[6] V. S. Padala, K. Gandhi, and P. Dasari, “Machine learning: the new language for applications,” IAES Int. J. Artif. Intell., vol. 8, no. 4, pp. 411 412, Dec. 2019, doi: 10.11591/ijai.v8.i4.pp411 421.
REFERENCES
[5] N. Razali, S. Ismail, and A. Mustapha, “Machine learning approach for flood risks prediction,” IAES Int. J. Artif. Intell., vol. 9, no. 1, pp. 73 80, Mar. 2020, doi: 10.11591/ijai.v9.i1.pp73 80.
[12] R. P. Noronha, “Diversity control in the hybridization GA PSO with fuzzy adaptive inertial weight,” in 2021 5th International Conference on Intelligent Computing and Control Systems (ICICCS), May 2021, pp. 1055 1062, doi: 10.1109/iciccs51141.2021.9432269.
[11] A. M. Manasrah and H. B. Ali, “Workflow scheduling using hybrid GA PSO algorithm in cloud computing,” Wirel. Commun. Mob. Comput., vol. 2018, pp. 1 16, 2018, doi: 10.1155/2018/1934784.
[4] S. Mirjalili, J. Song Dong, A. S. Sadiq, and H. Faris, “Genetic algorithm: Theory, literature review, and application in image reconstruction,” Nature inspired Optim., pp. 69 85, 2020.
[1] S. Sengupta, S. Basak, and R. A. Peters, “Particle Swarm Optimization: A survey of historical and recent developments with hybridization perspectives,” Mach. Learn. Knowl. Extr., vol. 1, no. 1, pp. 157 191, 2018.
[14] S. Masrom, R. A. Rahman, N. Baharun, and A. S. A. Rahman, “Automated machine learning with genetic programming on real dataset of tax avoidance classification problem,” in Proceedings of the 2020 9th International Conference on Educational and Information Technology, Feb. 2020, pp. 139 143, doi: 10.1145/3383923.3383942.
BIOGRAPHIES OF AUTHORS
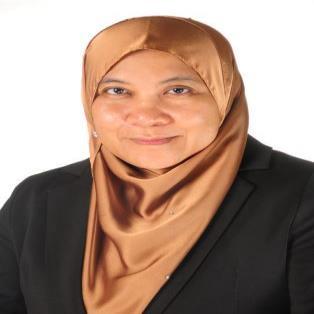



Ts. Abdullah Sani Abd Rahman obtained his first degree in Informatique majoring in Industrial Systems from the University of La Rochelle, France in 1995. He received a master’s degree from Universiti Putra Malaysia in Computer Science, with a specialization in Distributed Computing. Currently, he is a lecturer at the Universiti Teknologi PETRONAS, Malaysia and a member of the Institute of Autonomous System at the same university. His research interests are cybersecurity, data analytics and machine learning. He is also a registered Professional Technologist. He can be contacted at email: sani.arahman@utp.edu.my. Dr. Norhayati Baharun is an Associate Professor of Statistics, Universiti Teknologi MARA Perak Branch, Tapah Campus. She received her PhD in Statistics Education from the University of Wollongong Australia in 2012. Her career started as an academic from January 2000 to date at the Universiti Teknologi MARA that specialized in statistics. Other academic qualifications include both Master Degree and Bachelor Degree in Statistics from Universiti Sains Malaysia and Diploma in Statistics from Institute Teknologi MARA. Among her recent academic achievements include twelve on going and completed research grants (local and international), four completed supervision of postgraduate studies, fifteen indexed journal publications, two academic and policy books, twenty six refereed conference proceedings and book chapter publications, a recipient of 2013 UiTM Academic Award on Teaching, and fourteen innovation projects with two registered Intellectual Property Rights by RIBU, UiTM. She is also a certified Professional Technologist (Ts.) (Information & Computing Technology) of the Malaysia Board of Technologist (MBOT), a Fellow Member of the Royal Statistical Society (RSS), London, United Kingdom, a Professional Member of the Association for Computing Machinery (ACM), New York, USA, and a Certified Neuro Linguistic Program (NLP) Coach of the Malaysia Neuro Linguistic Program Academy. Her research interests continue with current postgraduate students under her supervision in the area of decision science now expanding to a machine learning application. She can be contacted at email: norha603@uitm.edu.my.
Dr. Rahayu Abdul Rahman is an Associate Professor at the Faculty of Accountancy, UiTM. She received her PhD in Accounting from Massey University, Auckland, New Zealand in 2012. Her research interest surrounds areas, like financial reporting quality such as earnings management and accounting conservatism as well as financial leakages including financial reporting frauds and tax aggressiveness She has published many research papers on machine learning and its application to corporate tax avoidance. She is currently one of the research members of the Machine Learning and Interactive Visualization Research Group at the UiTM Perak Branch. She can be contacted at email: rahay916@uitm.edu.my
Dr. Masurah Mohamad is currently a Senior Lecturer at Universiti Teknologi MARA Perak Branch, Tapah Campus (UiTM Perak) which is an educational institute established by the Ministry of Higher Education Malaysia for 15 years in the Department of Computer Science. She received her Ph.D. in Computer Science from UTM in 2021. Before joining Universiti Teknologi MARA, she served with Management and Science University (MSU) for 1 year. She has received several research grants such as the Fundamental Research Grant (FRGS) funded by the Ministry of Higher Education in 2012 and 2021, and the Lestari Research Grant funded by Universiti Teknologi MARA (UiTM) in 2019 and other internal and external research grants (2009 2020). Currently, she is serving on the Editorial Boards of Mathematical Sciences and Informatics Journal (MIJ) under UiTM Press Publications, Malaysia. She is a Publication chair for the 1st International Conference on Artificial Intelligence and Data Sciences (AiDAS2019) and the 2nd International Conference on Artificial Intelligence and Data Sciences (AiDAS2021). She also has contributed to several conferences organized by UiTM Perak Branch as secretariat committee (2010, 2013, and 2015) and reviewed several articles for several journals and conferences. Her research interests include data sciences and analytics, data mining and information retrievals, Artificial Intelligence and machine learning, recommender systems, soft computing, and data visualization. She can be contacted at email: masur480@uitm.edu.my.
Int J Artif Intell ISSN: 2252 8938 Machine learning of tax avoidance detection based on … (Suraya Masrom) 1163
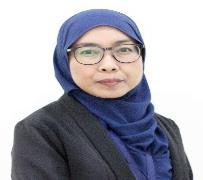








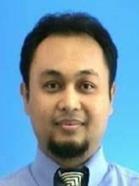



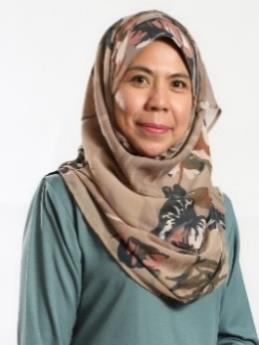



IAES International Journal of Artificial Intelligence (IJ AI) Vol. 11, No. 3, September 2022, pp. 1164~1174 ISSN: 2252 8938, DOI: 10.11591/ijai.v11.i3.pp1164 1174 1164
Journal homepage: http://ijai.iaescore.com
This is an open access article under the CC BY SA license. Corresponding Author: Baydaa Sulaiman Bahnam Department of Software, College of Computer Science and Mathematics, Mosul University Mosul, Iraq Email: baydaa_sulaiman@uomosul.edu.iq
Baydaa Sulaiman Bahnam1, Suhair Abd Dawwod2
Diabetes mellitus (DM) is one of the most diffuse diseases in the world. At present, about 425 million people have been infected worldwide, and it is expected that up to 700 million people will be infected by 2045 [1] It is a chronic metabolic disease caused by the pancreas not producing enough insulin or the body's cells not responding to the insulin that is produced. Thus, high blood sugar occurs, which leads to many health disorders. Depending on World Health Organization (WHO) and American Diabetes Association (ADA), DM is classified into four types [2] [4]: i) type I DM: or insulin dependent diabetes mellitus (IDDM). The failure of the body to produce insulin due to the destruction of the pancreas generates this type. It is usually diagnosed in children and young age, approximately 5% 10% of all diabetes mellitus are of this type; ii) type II DM: also called non insulin dependent diabetes mellitus (NIDDM) or "adult onset diabetes." It is the most common, about 90% of diabetics. It results from the failure of the body's cells from consuming the secreted insulin and thus leads to an increase in blood sugar levels; iii) gestational diabetes (GDM): 4% of pregnant women develop this type due to pregnancy changes in the body, and it usually turns into the second type after pregnancy; iv) rare specific diabetes. it is caused by genetic and metabolic disorders.
A proposed model for diabetes mellitus classification using coyote optimization algorithm and least squares support vector machine
Article Info ABSTRACT
Article history: Received Oct 27, 2021 Revised May 19, 2022 Accepted Jun 17, 2022
1Department of Software, College of Computer Science and Mathematics, Mosul University, Mosul, Iraq 2Department Management Information Systems, College of Administration and Economics, Mosul University, Mosul, Iraq
One of the most dangerous health diseases affecting the world's population is diabetes mellitus (DM), and its diagnosis is the key to its treatment. Several methods have been implemented to diagnose diabetes patients. In this work, a hybrid model which combines of coyote optimization algorithm (COA) and least squares support vector machine (LS-SVM) is proposed to classify of Type II DM patients. LS-SVM classifier is applied for classification process but it's very sensitive when its parameter values are changed. To overcome this problem, COA algorithm is implemented to optimize parameters of the LS-SVM classifier. This is the goal of the proposed model called the COA-LS-SVM. The proposed model is implemented and evaluated using Pima Indians Diabetes Dataset (PIDD). Also, it's compared with several classification algorithms that were implemented on the same PIDD. The experimental results demonstrated the effectiveness of the proposed model and its superiority over other algorithms, as it could accomplish an average classification accuracy of 98.811%.
1. INTRODUCTION
Keywords: CoyoteClassificationoptimization algorithm Diabetes mellitus Least squares support vector Swarmmachineintelligence algorithms
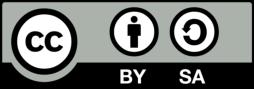
Int J Artif Intell ISSN: 2252 8938 A proposed model for diabetes mellitus classification … (Baydaa Sulaiman Bahnam) 1165
The present work intends to propose a model a model COA LS SVM based on COA algorithm and LS SVM classifier. The proposed model was used to classify DM patient accurately. The next two sections provide an overview of these algorithms used.
The main objective of this paper is to propose a hybrid model for diagnosing diabetes to increase health awareness in the community with the help of health practitioners in diagnosing the disease to control it and avoid its danger. This proposed model coyote optimization algorithm and least squares support vector machine (COA LS SVM) is based on the COA algorithm and the LS SVM classifier. Where the COA algorithm uses to find the optimal values for the LS SVM parameters to overcome its sensitivity to changes in its parameter values and the LS SVM classifier uses to classify Type II DM. Achieving a balance between the exploration and exploitation distinguishing the algorithm of COA from others during the optimization process.
2.1. Coyote optimization algorithm (COA) The swarm intelligence algorithms (SIAs) are inspired from the social action of creatures to solve several problems [24] [26]. One of these recent algorithm is COA for global optimization problems. It was lately proposed meta heuristic algorithm by Pierezan et al. in 2018 [27]. The major scheme of COA
Diabetes is one of the main reasons for the increase in the number of deaths in the world, especially the Type II DM, which is the most common [4]. Many serious health disorders occur when neglected and not treated, such as heart attack, myocardial infarction, stroke, renal failure, blindness, neuropathy, gangrene, micro vascular damage and increased susceptibility to infection [5], [6] With its spread, places a great strain on the public health system [7], [8]. So, the important step is to detect and diagnose it early. In the modern times, a lot of research works concentrated on using machine learning (ML) algorithms to detect and diagnose of DM using pima Indian diabetes dataset (PIDD) [9] [11] A study of Patel and Tamani [11] showed that the accuracy of the logistic regression (LR) and gradient boost (GB) algorithms were higher than the other algorithms at 79%. Patil et al. [12] proposed approach depending on Mayfly algorithm for feature selection and support vector machine (SVM) classifier to diagnose Type II DM. The outcome showed that the accuracy of this approach is 94.5% comparing with other studies. Panda et al. [13] used four algorithms of ML: SVM, k nearest neighbor (KNN), LR, and gradient boost (GB) to predict DM. The results showed that the GB algorithm outperforms the other algorithms with the highest accuracy of 81.25%. Alalwan [14] proposed two conceptual models of data mining: self organizing map (SOM) and random forest algorithm (RFA). The experimental showed that the accuracy of SOM is outperformed RFA, which reached 85%. Rajni and Amandeep [15] used the RB Bayes algorithm, which reached 72.9% the highest prediction accuracy compared to other algorithms. Bozkurt et al. [16] used six various neural networks to classify DM patients. The experiments showed that distributed time delay net works (DTDN) is the best comparing with others with accuracy of 76.00%. Rahman and Afroz [17] used data mining tools to comparative study of different classification techniques. These techniques are multilayer perceptron (MLP), bayesnet, naïve bayes, J48graft, fuzzy lattice reasoning (FLR), JRip (JRipper), fuzzy inference system (FIS) and adaptive neuro fuzzy inference system (ANFIS). The results showed that J48graft classifier is best with an accuracy of 81.33%. Khashei et al [18] constructed a hybrid model of MLP which depended on the idea of soft computing and artificial intelligence techniques. The experiments showed that the hybird model MLP is outperform over the other methods with accuracy 81.2%. Marcano Cedeño et al. [19] proposed a prediction model AM MLP that based on artificial metaplasticity (AM) with MLP to predicate diabetes. The accuracy obtained from this model was 89.93%. Karegowda et al. [20] presented a hybrid approach GA BPN that combines genetic algorithm (GA) and back propatation network (BPN). The GA was used to optimize the weight of BPN. The accuracy of the GA BPN model was 84.713% which was better than without GA. Fiuzy et al. [21] proposed a model based on three techniques which are: fuzzy system to instant and precise decision making, ant colony algorithm (ACO) to select best rules in fuzzy systems while ANN for modeling, structure identification and parameter identification. The accuracy reached from this model was 95.852%. Haritha et al [22] used firefly and cuckoo search algorithms to reduce dimension and then classify UCI dataset type I and type II using traditional KNN classifier and fuzzy KNN. The accuracy obtained of UCI type II is 71.3% for firefly fuzzy KNN and 74.8% for cuckoo fuzzy KNN. Zhang et al [23] used a multi layer feed forward neural network to predict of DM. This network provided results with 82% accuracy.
2. OVERVIEW OF METHODOLOGIES
For this reason, the authors in this paper motivated to use this algorithm for the first time to find the optimal values of LS SVM parameters to overcome the problem of its sensitivity to changes in its parameter values.
Also, this study compares the performance of this proposed approach with others ones. The implementation results demonstration the powerful of this proposed model COA LS SVM, which has the average accuracy of 98.811% outperforms the other algorithms. The rest of the paper is structured; next section presents COA and LS SVM algorithms. Section 3 described the proposed model and the data set. The experimental results are covered in section 4. Finally, the conclusion and future work of this paper are mentioned in section 5
ISSN: 2252 8938 Int J Artif Intell, Vol. 11, No. 3, September 2022: 1164 1174 1166optimizer is stimulated on Canis latrans species that stay principally in North America [27]. This algorithm is concerned to represent the coyotes’ social society and acclimatize it with a various algorithmic structure. An important advantage of this method is that it maintains a balance between the exploitation and exploration phase through this optimization approached [28]. COA is not attentive with the hierarchy and dominance rules pursued in grey wolf optimization (GWO) animals, and also it does not rely only on the hunting prey pursued in the GWO, but on the social structure and exchange of methodical experiences among wolves. By moving towards the prey as a group, it made it characterized by a cooperative trait while devouring the prey individually [29]. And coyotes can locate prey through their strong sense of smell. The hunt takes place as the coyotes attack the prey in groups, and this requires the agents to update their positions to the best. When Coyotes’ infecting their rivals, they are fully ready with a threat chance and flit to new position as excessive random distance away from its current position. Consider the following given to start with COA algorithm [30] [33]:The COA technique has been prepared based on the social conditions �������� ��,�� of ����ℎ coyote in ����ℎ pack at ����ℎ instant of time for the decision variables �� ⃗ which can be written: �������� ��,�� =�� ⃗ =(��1,��2, ,����) (1) where, �� is the dimension of the search space. The COA starts with setting coyotes’ global population, the social condition, is ��������,�� ��,�� for the ����ℎ dimension which can be written: ��������,�� ��,�� =������ +����(������ ������) (2) where, ���� ∈ [0,1] is the real random number, ������ ������������ are the lower and upper bounds of the ����ℎ decision variable. The fitness function of each coyote in their current social conditions is calculated in (3): �������� ��,�� =���� ��,��(�������� ��,��) (3)
Randomly, the algorithm updates the packs location. As well as the candidates update their position by departing their packs to other one. This behavior can be represented by the following Probability ���� which based on ���� : ���� =0.005.���� 2 (4) where the number of ���� that makes ���� >1 is restricted to 14 coyotesinner the pack. The alpha coyote as in (5) which is the best solution of each iteration. It means that the alpha coyote is only one for the global population to optimize the problem in ����ℎ pack at ����ℎ instant of time: ������ℎ����,�� ={�������� ��,��|��������={1,2, ����} ��������(�������� ��,��)} (5) All the coyotes’ information in COA are linked and calculated as culture transformation as the following: ���������� ��,�� ={��(����+1)⁄2,�� ��,�� , ���� ���������� ������/2,�� ��,�� +��(���� 2 +1),�� ��,�� 2 , ����ℎ������������ (6) where ����,�� , is the ordered social conditions of coyotes ����ℎ pack at ����ℎ instant of time. The birth and death of a coyote in COA are two important happenings, as this coyote' age is the �������� ��,�� ∈��. The birth of a new coyote is affected by the social conditions surrounding the randomly chosen parents, as well as the influence of the environment, such as (7): �������� ��,�� = { ��������1,��, ��,�� �������� <���� ������ = ��1 ��������2,��, ��,�� �������� ≥���� +���� ������ = ��2 ���� , ����ℎ������������ (7)
Int J Artif Intell ISSN: 2252 8938 A proposed model for diabetes mellitus classification … (Baydaa Sulaiman Bahnam) 1167 where ��1��������2 are random coyotes from ����ℎ pack, ��1 ��������2 are random dimensions of the problem, ���� ���������� are scatter and association probabilities respectively that declare the coyote’s cultural diversity from the pack, ���� is random number within the bounds of the ����ℎ decision variable and �������� is random number in [0,1] generated by uniform probability. The cultural diversity of the coyotes in the pack described by ���� ����������, which can be calculated: ���� =1⁄�� (8) ���� = (1 ����)⁄�� (9) There are three rules for life cycle of COA as shown in the pseudo code 1 [31] [36]: The pseudo code 1. Life cycle rules of COA Calculate �� and �� (�� is the worst fitness function of the coyotes; �� is the coyotes’ number in pack)
If �� =1 Parent survive while the only coyote in �� dies Else if �� >1 Parent survive while the oldest coyote in �� dies ParentElse die End if The cultural adaptation among the packs is determined by two factors alpha influence ��1 and pack influence ��2 as: ��1 =����,�� ����������1 ��,�� (10) ��2 =��������,�� ����������2 ��,�� (11) where, ����1 ����������2 are the random coyotes. To update the social condition of the coyote is wrriten as: ������ �������� ��,�� = �������� ��,�� +����1.��1 +����2.��2 (12) where, ����1����������2 are random numbers in the range [0,1]. Finally, the new fitness function and the updating process of the social condition are founded by (13) and (14) respectively: ������ �������� ��,�� =��(������ �������� ��,��) (13) �������� ��,��+1 = {������ �������� ��,�� , ������ �������� ��,�� <�������� ��,�� �������� ��,�� ����ℎ������������ (14) The following is the pseudo code 2 that illustrates COA [34] [36]: The pseudo code 2. Coyote optimization algorithm COA Determine population Np and coyote Nc by (2) Find the Fitness function of the coyote by (3) While stop criteria is not meet do For each population P do Determine the alpha coyote by (5) Calculate the culture transformation by (6) For coyote C of each population P do Find the new social condition by (12) Find the new fitness function by (13) Update the social condition by (14) End Perffororm the birth and death process by (7) and pseudo code 1 End Performfor pack's transitions by (4) Update the age of coyotes End Outputwhilethe global best coyote 2.2. Least squares support vector machine (LS SVM)
One of the versions for SVM classifiers is LS SVM classifier, which suggested by Suykens Vandewalle in 1999 [37]. The goal of LS SVM classifier is to detect optimal separating hyper plane in higher dimensional space by using euclidean distance [37], [38]. The advantage of LS SVM is that it can handle a set of linear equations instead of the quadratic programming problem that suffers from high arithmetic operations [39]. It is famous for its extreme sensitivity to a change in the values of its parameters.
Consider the following given to start with LS SVM [39] [41]: In the primal weight space, the optimization problem is formulated in (15), if we consider {����,����}��=1 �� is a training set of N points, in which ���� ∈���� for input data and ���� ∈ �� for output data: ��������(��,��)��,��,�� = 1 2 ������+ 1 2��∑ ���� ��2 ��=1 (15) yield to: ���� (���������� +��)=����,�� =1,2, ,�� (16) where �� is regularizationfactor, ���� is the difference between the desiredoutput ���� and the actualoutput, ��( ) is nonlinearfunction, �� is weightvector and �� is biasterm,where�� ∈��. Also, a linear classifier in the new area takes as in (17): ��(��) =��������(��.��(��)+��) (17) Calculating the duple area instead of the initial area by finding the following Lagrangian function: ��(��,��,��)=��(��,��) ∑ ∝�� (������(����)+���� ����)�� ��=1 (18) where ∝�� is Lagrangian multipliers called support vectors. An objective function in (18) is optimal when it satisfies the following conditions of karush kuhn tucker (KKT) in (19): ���� ���� =0→�� =∑ ����������(����)�� ��=1 (19) ���� ���� =0→∝��=������ ,�� =1, ,�� ���� ���� =0→������(����)+���� ���� =0,�� =1,….,�� The following linear system could obtain after removal of �� and ��: (��+1��)∝=�� �������� ��,��+1 = {������ �������� ��,�� , ������ �������� ��,�� <�������� ��,�� �������� ��,�� ����ℎ������������ (20) where the Kernel Matrix is �� =[��1,��2,…,����]�� , �� =[��,��2,…,����]��where �� ∈�������� , �� is Gaussian Kernel function. The function estimation has been obtained as the result of LS SVM model in (21): ��(��) =∑ ������(��,����)�� ��=1 (21) and, to perform LS SVM, the radial basis function (RBF) has been utilized: ��(��,����)=exp( |�� ����|2 ��2 ) (22) The following is the pseudo code 3 that illustrates LS SVM [42] [45]: The pseudo code 3. LS-SVM Algorithm Enter the data set of n data point {xk,yk}k=1 N , where xi is the ith input vector and yi ∈R is the corresponding ith target with values { 1,+1} For each enter data point, randomly generate weights.
ISSN: 2252 8938 Int J Artif Intell, Vol. 11, No. 3, September 2022: 1164 1174 1168
Data set No. of cases Feature Input Output classes Pima 768 8 2 Healthy cases ���� cases 500 268
3. METHODOLOGY
A
For each enter data point, randomly set the initial bias b and error e. Randomly set the initial values of γ and σ Calculate and look for the values of (w, b, e) that minimize the objective function using (15) and Calculate(16).the function of Lagrangian using (18) with the solution, which must meet the conditions of KKT in a group of (19).
Classify the training data of LS SVM using (21) with Kernel function RBF using (22).
Calculate the number of support vectors ∝ using (20).
Figure 1. A block diagram of the proposed algorithm COA LS SVM
Classify any new data point using (17) and Kernel function RBF using (22). Repeat till stopping criteria is met, usually till reach the maximum number of iterations.
The proposed algorithm is a combination of two algorithms COA and LS SVM as shown in the Figure 1. Where COA algorithm was used in the first stage in order to obtain the optimal parameters for LS SVM, while in the second stage LS SVM classifier was used to classify patients as: i) first stage: optimizing parameters. The goal of this stage is to obtain the optimal parameter values of LS SVM. The COA algorithm was used to optimize the LS SVM parameter values to overcome its sensitivity to changes in its parameter values. These parameters are the regularization factor γ and Gaussian Kernel function σ; and ii) second stage: classification. This stage consisting of two important stages: training stage then followed by testing stage. The goal of this stage is classifying the Type II DM patients into one of two classes Healthy and DM.
3.1. The proposed algorithm COA LS SVM
Table 1 Information of data set
For each enter data point, randomly set the initial bias b and error e Calculate the optimal values of γ and σ using the pseudo code 2. Calculate the optimal values of (w, b, e) for the objective function using (15) and (16). Calculate the number of support vectors ∝ using (20). Classify any new data point using (17) and Kernel function RBF using (22). Repeat till stopping criteria is met, usually until the maximum number of iterations is reached 3.2. Data set PIDD used in this research was collected from the machine learning database at UCI repository and all the details about it are available in [46]. The data set consists of 768 cases whom were at least 21 years old. Table 1 and Figure 2 are summarized the information and features about this data set.
The following is the pseudo code 4 that explain the proposed algorithm COA LS SVM in details:
For each enter data point, randomly generate weights.
Int J Artif Intell ISSN: 2252 8938 proposed model for diabetes mellitus classification … (Baydaa Sulaiman Bahnam) 1169
The pseudo code 4. Proposed Algorithm COA LS SVM Enter the data set of n data points, {xk,yk}k=1 N , where xi is the ith input vector and yi ∈R is the conforming ith target with values { 1,+1}
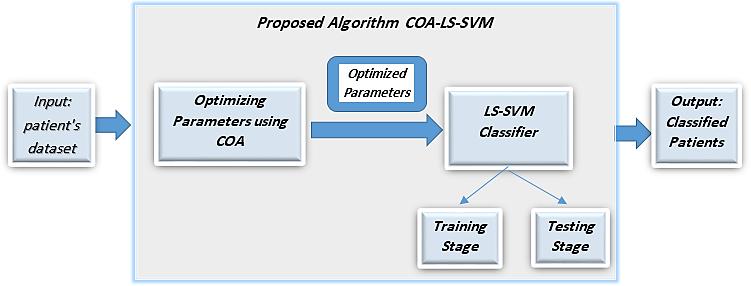
TN = True Negative FN= False Negative denote the numbers of cases incorrectly diagnosed
ISSN: 2252 8938 Int J Artif Intell, Vol. 11, No. 3, September 2022: 1164 1174 1170 Figure 2 Features data set
The input of the COA is 768 cases of PIDD. In the search domain, these cases are randomly created for 100 iterations. The output from the first stage COA of the proposed algorithm is the optimal values of LS SVM parameters which are γ=100 and σ=0.5. These optimal parameters used with the second stage of the proposed algorithm LS SVM classifier and RBF kernel function (22), in order to find the optimal hyperplane that detaches the search area into two classes (Healthy, DM) by calculating the optimal values of (w, b, e) in the objective function (15) and (16).
4. EXPERIMENTAL RESULTS
Accuracy metric was used to evaluate the performance of the proposed method [47] [50]: ���������������� = ����+���� ����+����+����+���� (23) where: TP= True Positive denote the numbers of cases correctly diagnosed
FP = False Positive where the records with healthy label denotes positive cases while DM label denotes negative ones. The proposed COA LS SVM algorithm is validated using the k fold cross validation (K Fold CV) method for getting the best average accuracy value. K Fold CV divides data into �� folds. At each iteration, one fold (��) is used as test data set while training data set is resided folds (K1) in �� experiments [51], [52] In this work, the value of �� =10 folds, nine data sets for training and one for testing, then repeat this process ten times until all data has been evaluated. Figure 3 illustrates the 10 Fold CV. The testing average accuracy value of LS SVM is 98.811% using the kernel function RBF for 10 times iteration. Table 2 shows the testing accuracy value for each 10 Fold CV. The performance of the proposed model COA LS SVM has been compared with the models of other works using the PIDD database. The main objective is to diagnose whether the patient is diabetic or not using this data. It is appropriate to analyze and evaluate the result of the proposed model with other works since the past 10 years, using the accuracy scale of classification. Table 3 shows the comparison and analysis of the proposed model with the previous works selected based on the classification of accuracy; in addition to the number of cases used in each study.
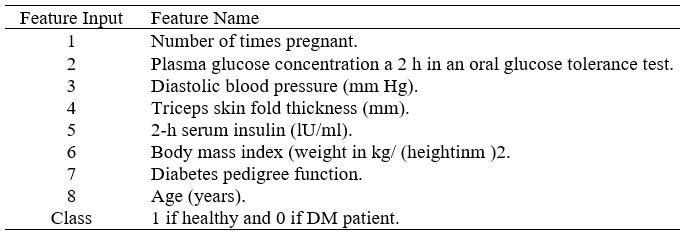
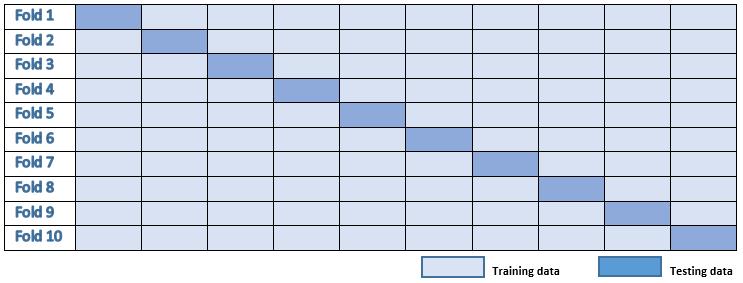
Neural network with genetic algorithm [56] 87.46% 768 cases LDA MWSVM [57] 89.74% 768 cases AMMLP [19] 89.93% 768 cases K means and DT [58] 90.03% 768 cases A modified mayfly SVM [12] 94.5% 768 cases Fuzzy, DT, ACO and ANN model [21] 95.852% 247 cases The proposed algorithm 98.811% 768 cases
3. A comparative study of related research works for average classification accuracy of PIDD Algorithm Accuracy No. of cases PCA, Kmeans algorithm [53] 72% 768 cases RB bayes algorithm [15] 72.9% 768 cases Cuckoo fuzzy KNN [22] 74.8% 768 cases DTDN [16] 76% 768 cases SVM [54] 78% 460 cases LR, GB [11] 79% 768 cases Naïve Bayes [55] 79.56% 768 cases GB [13] 81.25% 768 cases J48graft [17] 81.33% 768 cases Multi layer feed forward neural network [23] 82% 768 cases Hyper MLP [18] 82.4% 768 cases GA BPN [20] 84.713% 392 cases SOM [14] 85% 768 cases
Figure 3. 10 fold cross validation Table 2. Accuracy value for each 10 fold CV Fold No. Accuracy value Fold 1 95.953% Fold 2 96.963% Fold 3 98.837% Fold 4 99.98% Fold 5 98.981% Fold 6 99.678% Fold 7 98.9359% Fold 8 99.99% Fold 9 99.99% Fold 10 98.81% Average 98.811%
Figure 4. The classification accuracies of proposed model COA LS SVM and other models
Figure 4 depictes the comparison of the proposed model with the previous approches that used PIDD. This graph denotes that the this work has outdone previous approches. The highest average classification accuracy using proposed model COA LS SVM is 98.811% which has outperformed the other models.Table
Int J Artif Intell ISSN: 2252 8938 A proposed model for diabetes mellitus classification … (Baydaa Sulaiman Bahnam) 1171
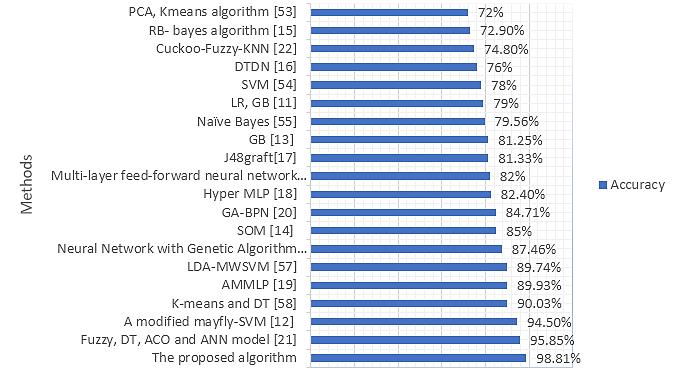
ISSN: 2252 8938 Int J Artif Intell, Vol. 11, No. 3, September 2022: 1164 1174 1172 5. CONCLUSION
[18] M. Khashei, S. Eftekhari, and J. Parvizian, “Diagnosing diabetes type II using a soft intelligent binary classification model,” Rev. Bioinforma. Biometrics, vol. 1, no. 1, pp. 9 23, 2012 [19] A. Marcano Cedeño, J. Torres, and D. Andina, “A prediction model to diabetes using artificial metaplasticity,” in Lecture Notes in Computer Science (including subseries Lecture Notes in Artificial Intelligence and Lecture Notes in Bioinformatics), vol. 6687, no. 2, Springer Berlin Heidelberg, 2011, pp. 418 425, doi: 10.1007/978 3 642 21326 7_45.
[13] M. Panda, D. P. Mishra, S. M. Patro, and S. R. Salkuti, “Prediction of diabetes disease using machine learning algorithms,” IAES Int. J. Artif. Intell., vol. 11, no. 1, pp. 284 290, Mar. 2022, doi: 10.11591/ijai.v11.i1.pp284 290.
[8] M. M. Rosli, N. S. M. Yusop, and A. S. Fazuly, “Design of meal intake prediction for gestational diabetes mellitus using genetic algorithm,” IAES Int. J. Artif. Intell., vol. 9, no. 4, pp. 591 599, 2020, doi: 10.11591/ijai. v9.i4.
[6] R. Sofiana and S. Sutikno, “Optimization of backpropagation for early detection of diabetes mellitus,” Int. J. Electr. Comput. Eng., vol. 8, no. 5, pp. 3232 3237, Oct. 2018, doi: 10.11591/ijece.v8i5.pp3232 3237.
[20] A. G. Karegowda, A. S. Manjunath, and M. A. Jayaram, “Application of genetic algorithm optimized neural network connection weights for medical diagnosis of PIMA Indians diabetes,” Int. J. Soft Comput., vol. 2, no. 2, pp. 15 23, May 2011, doi: 10.5121/ijsc.2011.2202. [21] M. Fiuzy, A. Qarehkhani, J. Haddadnia, J. Vahidi, and H. Varharam, “Introduction of a method to diabetes diagnosis according to optimum rules in fuzzy systems based on combination of data mining algorithm (DT), evolutionary algorithms (ACO) and artificial neural networks (NN),” J. Math. Comput. Sci., vol. 06, no. 04, pp. 272 285, May 2013, doi: 10.22436/jmcs.06.04.03.
[10] L. Syafaah, S. Basuki, F. Dwi Setiawan Sumadi, A. Faruq, and M. H. Purnomo, “Diabetes prediction based on discrete and continuous mean amplitude of glycemic excursions using machine learning,” Bull. Electr. Eng. Informatics, vol. 9, no. 6, pp. 2619 2629, Aug. 2020, doi: 10.11591/eei.v9i6.2387.
[23] Y. Zhang, Z. Lin, Y. Kang, R. Ning, and Y. Meng, “A feed forward neural network model for the accurate prediction of diabetes mellitus,” Int. J. Sci. Technol. Res., vol. 7, no. 8, pp. 151 155, 2018
REFERENCES [1] “IDF diabetes atlas,” International Diabetes Federation 10th Ed. 2021. (Accessed: Sep. 2, 2021). [Online]. Available: https://www.diabetesatlas.org/ [2] “Diabetes,” World Health Organization (Accessed Sep. 2, 2021). [Online]. Available: https://www.who.int/news room/ fact sheets/detail/diabetes [3] “2. Classification and diagnosis of diabetes: standards of medical care in diabetes 2021,” Diabetes Care, vol. 44, pp. 15 33, Jan. 2021, doi: 10.2337/dc21 S002. [4] O. AlShorman, M. S. Masadeh, and B. AlShorman, “Mobile health monitoring based studies for diabetes mellitus: a review,” Bull. Electr. Eng. Informatics, vol. 10, no. 3, pp. 1405 1414, Jun. 2021, doi: 10.11591/eei.v10i3.3019. [5] A. L., H. Singhal, I. Dwivedi, and P. Ghuli, “Diabetic retinopathy classification using deep convolutional neural network,” Indones. J. Electr. Eng. Comput. Sci., vol. 24, no. 1, pp. 208 216, Oct. 2021, doi: 10.11591/ijeecs.v24.i1.pp208 216.
[7] J. Singh, “Centers for disease control and prevention,” Indian Journal of Pharmacology, vol. 36, no. 4. Qeios, pp. 268 269, Feb. 2004, doi: 10.1097/jom.0000000000001045.
[17] R. M. Rahman and F. Afroz, “Comparison of various classification techniques using different data mining tools for diabetes diagnosis,” J. Softw. Eng. Appl., vol. 06, no. 03, pp. 85 97, 2013, doi: 10.4236/jsea.2013.63013.
[22] R. Haritha, D. S. Babu, and P. Sammulal, “A hybrid approach for prediction of type 1 and type 2 diabetes using firefly and cuckoo search algorithms,” Int. J. Appl. Eng. Res., vol. 13, no. 2, pp. 896 907, 2018
[11] R. Patil and S. Tamane, “A comparative analysis on the evaluation of classification algorithms in the prediction of diabetes,” Int. J. Electr. Comput. Eng., vol. 8, no. 5, p. 3966, Oct. 2018, doi: 10.11591/ijece.v8i5.pp3966 3975.
[15] R. Rajni and A. Amandeep, “RB bayes algorithm for the prediction of diabetic in Pima Indian dataset,” Int. J. Electr. Comput. Eng., vol. 9, no. 6, pp. 4866 4872, Dec. 2019, doi: 10.11591/ijece.v9i6.pp4866 4872.
[16] M. R. Bozkurt, N. Yurtay, Z. Yilmaz, and C. Sertkaya, “Comparison of different methods for determining diabetes,” Turkish J. Electr. Eng. Comput. Sci., vol. 22, no. 4, pp. 1044 1055, 2014, doi: 10.3906/elk 1209 82.
The diagnosis of Type II DM has a significant impact on raising health awareness in the community. Therefore, the proposed models for diagnosing this disease can help the practitioners and the patient to avoid its danger, reduce its complications and prevent it. To improve the diagnostic performance of Type II DM disease more efficiently, an effective model based on COA LS SVM approach has been proposed. The COA algorithm was used in the first stage to optimize the parameters of LS SVM to overcome its problem which is very sensitive when its parameter values are changed. Then LS SVM classifier was employed to classify Type II DM. Optimizing LS SVM parameters using COA algorithm can ensure the robustness and effectiveness of the proposed model by finding for optimal values instead of trial and error, as well as making the classification more accurate and in less time. For verifying the efficiency of the proposed model, experiments were performed on the PIDD dataset by detecting Type II DM and comparing the accuracy of the model with the others models. The average accuracy of the proposed model was 98.811% which significantly outperformed the others previous models implemented on PIDD. And as a work for the near future, COA can be as an optimization technique and hybridized with other classification algorithms. Also, other evaluation parameters can be applied as well as other kernel functions.
[14] S. A. Diwan Alalwan, “Diabetic analytics: proposed conceptual data mining approaches in type 2 diabetes dataset,” Indones. J. Electr. Eng. Comput. Sci., vol. 14, no. 1, p. 88, Apr. 2019, doi: 10.11591/ijeecs.v14.i1.pp88 95.
[12] R. Patil, S. Tamane, S. A. Rawandale, and K. Patil, “A modified mayfly SVM approach for early detection of type 2 diabetes mellitus,” Int. J. Electr. Comput. Eng., vol. 12, no. 1, pp. 524 533, Feb. 2022, doi: 10.11591/ijece.v12i1.pp524 533.
[9] S. M. Rosli, M. M. Rosli, and R. Nordin, “A mapping study on blood glucose recommender system for patients with gestational diabetes mellitus,” Bull. Electr. Eng. Informatics, vol. 8, no. 4, pp. 1489 1495, Dec. 2019, doi: 10.11591/eei.v8i4.1633.
[33] E. M. Abdallah, M. I. El Sayed, M. M. Elgazzar, and A. A. Hassan, “Coyote multi objective optimization algorithm for optimal location and sizing of renewable distributed generators,” Int. J. Electr. Comput. Eng., vol. 11, no. 2, pp. 975 983, Apr. 2021, doi: 10.11591/ijece.v11i2.pp975 983. [34] D. Xiao, H. Li, and X. Sun, “Coal classification method based on improved local receptive field based extreme learning machine algorithm and visible infrared spectroscopy,” ACS Omega, vol. 5, no. 40, pp. 25772 25783, Oct. 2020, doi: 10.1021/acsomega.0c03069.
[44] Z. Tian, “Backtracking search optimization algorithm based least square support vector machine and its applications,” Eng. Appl. Artif. Intell., vol. 94, Sep. 2020, doi: 10.1016/j.engappai.2020.103801.
[25] S. Selvaraj and E. Choi, “Survey of swarm intelligence algorithms,” in Proceedings of the 3rd International Conference on Software Engineering and Information Management, Jan. 2020, pp. 69 73, doi: 10.1145/3378936.3378977.
[37] J. A. K. Suykens and J. Vandewalle, “Least squares support vector machine classifiers,” Neural Process. Lett., vol. 9, no. 3, pp. 293 300, 1999, doi: 10.1023/A:1018628609742.
[24] I. Obagbuwa, “Swarm intelligence algorithms and applications to real world optimization problems: a survey,” Int. J. Simul. Syst. Sci. Technol., May 2018, doi: 10.5013/ijssst.a.19.02.05.
[35] J. Arfaoui, H. Rezk, M. Al Dhaifallah, M. N. Ibrahim, and M. Abdelkader, “Simulation based coyote optimization algorithm to determine gains of PI controller for enhancing the performance of solar PV water pumping system,” Energies, vol. 13, no. 17, Aug. 2020, doi: 10.3390/en13174473.
[28] A. Fathy, M. Al Dhaifallah, and H. Rezk, “Recent coyote algorithm based energy management strategy for enhancing fuel economy of hybrid FC/battery/SC system,” IEEE Access, vol. 7, pp. 179409 179419, 2019, doi: 10.1109/ACCESS.2019.2959547
[29] S. Mirjalili, S. M. Mirjalili, and A. Lewis, “Grey wolf optimizer,” Adv. Eng. Softw., vol. 69, pp. 46 61, Mar. 2014, doi: 10.1016/j.advengsoft.2013.12.007.
[31] T. T. Nguyen, T. T. Nguyen, N. A. Nguyen, and T. L. Duong, “A novel method based on coyote algorithm for simultaneous network reconfiguration and distribution generation placement,” Ain Shams Eng. J., vol. 12, no. 1, pp. 665 676, Mar. 2021, doi: 10.1016/j.asej.2020.06.005.
[41] Z. Mat Yasin, N. Ashida Salim, N. F. Ab Aziz, H. Mohamad, and N. Ab Wahab, “Prediction of solar irradiance using grey wolf optimizer least square support vector machine,” Indones. J. Electr. Eng. Comput. Sci., vol. 17, no. 1, pp. 10 17, Jan. 2020, doi: 10.11591/ijeecs.v17.i1.pp10 17. [42] A. Youssef, A. Youssef Ali Amer, N. Caballero, and J. M. Aerts, “Towards online personalized monitoring of human thermal sensation using machine learning approach,” Appl. Sci., vol. 9, no. 16, p. 3303, Aug. 2019, doi: 10.3390/app9163303.
Int J Artif Intell ISSN: 2252 8938 A proposed model for diabetes mellitus classification … (Baydaa Sulaiman Bahnam) 1173
[39] Z. M. Yasin, N. A. Salim, N. F. A. Aziz, Y. M. Ali, and H. Mohamad, “Long term load forecasting using grey wolf optimizer least squares support vector machine,” IAES Int. J. Artif. Intell., vol. 9, no. 3, pp. 417 423, Sep. 2020, doi: 10.11591/ijai.v9.i3.pp417 423. [40] L. Chen, L. Duan, Y. Shi, and C. Du, “PSO_LSSVM prediction model and its MATLAB implementation,” IOP Conf. Ser. Earth Environ. Sci., vol. 428, no. 1, Jan. 2020, doi: 10.1088/1755 1315/428/1/012089.
[43] W. Ma and H. Liu, “Classification method based on the deep structure and least squares support vector machine,” Electron. Lett., vol. 56, no. 11, pp. 538 541, May 2020, doi: 10.1049/el.2019.3776.
[49] C. Do, N. Q. Dam, and N. T. Lam, “Optimization of network traffic anomaly detection using machine learning,” Int. J. Electr. Comput. Eng., vol. 11, no. 3, pp. 2360 2370, Jun. 2021, doi: 10.11591/ijece.v11i3.pp2360 2370.
[50] C. Y. Lim, “Methods for evaluating the accuracy of diagnostic tests,” Cardiovasc. Prev. Pharmacother., vol. 3, no. 1, 2021, doi: 10.36011/cpp.2021.3.e2. [51] P. Ghosh, A. Karim, S. T. Atik, S. Afrin, and M. Saifuzzaman, “Expert cancer model using supervised algorithms with a LASSO selection approach,” Int. J. Electr. Comput. Eng., vol. 11, no. 3, pp. 2631 2639, Jun. 2021, doi: 10.11591/ijece.v11i3.pp2631 2639. [52] F. R. Lumbanraja, E. Fitri, Ardiansyah, A. Junaidi, and R. Prabowo, “Abstract classification using support vector machine algorithm (case study: abstract in a computer science journal),” J. Phys. Conf. Ser., vol. 1751, no. 1, p. 12042, Jan. 2021, doi: 10.1088/1742 6596/1751/1/012042. [53] R. N. Patil and S. Tamane, “A novel scheme for predicting type 2 diabetes in women: using kmeans with PCA as dimensionality reduction,” Int. J. Comput. Eng. Appl., pp. 76 87, 2017 [54] V. A. Kumari and R. Chitra, “Classification of diabetes disease using support vector machine,” Int. J. Eng. Res. Appl., vol. 3, no. 2, pp. 1797 1801, 2013
[36] A. Abaza, R. A. El Sehiemy, K. Mahmoud, M. Lehtonen, and M. M. F. Darwish, “Optimal estimation of proton exchange membrane fuel cells parameter based on coyote optimization algorithm,” Appl. Sci., vol. 11, no. 5, p. 2052, Feb. 2021, doi: 10.3390/app11052052.
[32] G. W. Chang and N. Cong Chinh, “Coyote optimization algorithm based approach for strategic planning of photovoltaic distributed generation,” IEEE Access, vol. 8, pp. 36180 36190, 2020, doi: 10.1109/ACCESS.2020.2975107.
[38] M. Farhadi and N. Mollayi, “Application of the least square support vector machine for point to point forecasting of the PV power,” Int. J. Electr. Comput. Eng., vol. 9, no. 4, pp. 2205 2211, Aug. 2019, doi: 10.11591/ijece.v9i4.pp2205 2211.
[26] B. S. B. Alyas, “A particle swarm optimization algorithm based classifier for medical data set,” Int. J. Enhanc. Res. Sci. Technol. Eng., vol. 4, no. 4, pp. 1 6, 2015 [27] J. Pierezan and L. Dos Santos Coelho, “Coyote optimization algorithm: a new metaheuristic for global optimization problems,” in 2018 IEEE Congress on Evolutionary Computation (CEC), Jul. 2018, pp. 1 8, doi: 10.1109/CEC.2018.8477769.
[45] M. Sivaram et al., “An optimal least square support vector machine based earnings prediction of blockchain financial products,” IEEE Access, vol. 8, pp. 120321 120330, 2020, doi: 10.1109/ACCESS.2020.3005808.
[46] “UCI Repository,” kaggle.com. (Accessed: Sept. 2, 2018). [Online]. Available: https://www.kaggle.com/uciml/pima indians diabetes database [47] K. S. Y. Pande, D. G. H. Divayana, and G. Indrawan, “Comparative analysis of naïve bayes and KNN on prediction of forex price movements for GBP/USD currency at time frame daily,” J. Phys. Conf. Ser., vol. 1810, no. 1, Mar. 2021, doi: 10.1088/1742 6596/1810/1/012012. [48] M. O. Adebiyi, M. O. Arowolo, and O. Olugbara, “A genetic algorithm for prediction of RNA seq malaria vector gene expression data classification using SVM kernels,” Bull. Electr. Eng. Informatics, vol. 10, no. 2, pp. 1071 1079, Apr. 2021, doi: 10.11591/eei.v10i2.2769.
[30] Z. Yuan, W. Wang, H. Wang, and A. Yildizbasi, “Developed coyote optimization algorithm and its application to optimal parameters estimation of PEMFC model,” Energy Reports, vol. 6, pp. 1106 1117, Nov. 2020, doi: 10.1016/j.egyr.2020.04.032.
ISSN: 2252 8938 Int J Artif Intell, Vol. 11, No. 3, September 2022: 1164 1174 1174 [55] A. Iyer, J. S, and R. Sumbaly, “Diagnosis of diabetes using classification mining techniques,” Int. J. Data Min. Knowl. Manag. Process, vol. 5, no. 1, pp. 1 14, Jan. 2015, doi: 10.5121/ijdkp.2015.5101.
[58] W. Chen, S. Chen, H. Zhang, and T. Wu, “A hybrid prediction model for type 2 diabetes using K means and decision tree,” in 2017 8th IEEE International Conference on Software Engineering and Service Science (ICSESS), Nov. 2017, pp. 386 390, doi: 10.1109/ICSESS.2017.8342938.
[56] S. Mohammad, H. Dadgar, and M. Kaardaan, “A hybrid method of feature selection and neural network with genetic algorithm to predict diabetes,” Int. J. Mechatronics, Electr. Comput. Technol., vol. 7, no. 24, pp. 3397 3404, 2017 [57] D. Çalişir and E. Doğantekin, “An automatic diabetes diagnosis system based on LDA wavelet support vector machine classifier,” Expert Syst. Appl., vol. 38, no. 7, pp. 8311 8315, Jul. 2011, doi: 10.1016/j.eswa.2011.01.017.
BIOGRAPHIES OF AUTHORS Baydaa Sulaiman Bahnam is an Assistant Professor in Department of Software, College of Computer Sciences and Mathematics, University of Mosul, Iraq since 2015. She received her M.Sc. Degrees in Computer Sciences in the same College from University of Mosul, Iraq, in 2006. Her research interests include the field of, artificial intelligence, swarm intelligence, machine learning methods, classification, image processing, evolutionary algorithms and data mining. She has a research gate account under the name Baydaa Sulaiman Bahnam. She can be contacted at email: baydaa_sulaiman@uomosul.edu.iq
Suhair Abd Dawwod is currently lecturer in Department of Management Information Systems College of Administration and Economics, University of Mosul, since 2001. I've got my B.Sc. and M.Sc. in Computer Science from the University of Mosul, Iraq in 1992 and 2001, respectively. My research interests include neural networks and artificial intelligence, database, data mining, machine learning, computer networks and security She can be contacted at email: suhair_abd_dawwod@uomosul.edu.iq
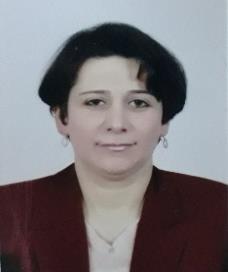



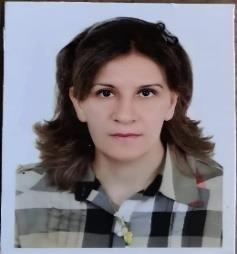



IAES International Journal of Artificial Intelligence (IJ AI) Vol. 11, No. 3, September 2022, pp. 1175~1183 ISSN: 2252 8938, DOI: 10.11591/ijai.v11.i3.pp1175 1183 1175
Journal homepage: http://ijai.iaescore.com
Article history: Received Jul 16, 2021 Revised Mar 14, 2022 Accepted Apr 12, 2022 Internet traffic classification is a fundamental task for network services and management. There are good machine learning models to identify the class of traffic. However, finding the most discriminating features to have efficient models remains essential. In this paper, we use interpretable machine learning algorithms such as decision tree, random forest and eXtreme gradient boosting (XGBoost) to find the most discriminating features for internet traffic classification. The dataset used contains 377,526 traffics. Each traffic is described by 248 features. From these features, we propose a 12 feature model with an accuracy of up to 99.76%. We tested it on another dataset with 19626 flows and obtained 98.40% of accuracy. This shows the efficiency and stability of our model. Also, we identify a set of 14 important features for internet traffic classification, including two that are crucial: port number (server) and minimum segment size (client to server).
This is an open access article under the CC BY SA license. Corresponding Author: Vinasetan Ratheil Houndji Institut de Formation et de Recherche en Informatique, Université d’Abomey Calavi 01 BP 526 Abomey Calavi Bénin Email: ratheil.houndji@uac.bj
1. INTRODUCTION
Article Info ABSTRACT
Erick A. Adje1, Vinasetan Ratheil Houndji2, Michel Dossou3 1Ecole Doctorale des Sciences de l’Ingénieur, Université d’Abomey Calavi, Abomey Calavi, Bénin 2Institut de Formation et de Recherche en Informatique, Université d’Abomey Calavi, Abomey Calavi, Bénin 3Ecole Polytechnique d’Abomey Calavi, Université d’Abomey Calavi, Abomey Calavi, Bénin
Features analysis of internet traffic classification using interpretable machine learning models
Keywords: Classification algorithm Internet TrafficTrafficMachinetrafficlearningclassificationinternetdiscriminators
Internet traffic has increased significantly over the last decade due to new technologies, industries, and applications. It becomes an interesting challenge for network management. Accurate classification of internet traffic is fundamental for better management of network traffic, from monitoring to security, from the quality of service (QoS) to the provision of the right resource. Automatic traffic classification is an automated process that classifies network traffic according to various parameters (e.g., port number, protocol, and the number of packets exchanged) into various traffic classes (e.g., web, multimedia, database, e mail, games, and file transfer). It consists of examining internet protocol (IP) packets to extract some specific characteristics to answer some questions related to their origins such as the content or the user’s intentions. Typically, it deals with packet flows defined as sequences of packets uniquely identified by the source IP address, source port, destination IP address, destination port and protocol used at the transport layer, and many others. While research on traffic classification is quite specific, the author’s motivations are not always the same [1]. Some approaches classify traffic according to its category i.e., whether the traffic represents file transfer, peer to peer (P2P), games, multimedia, web, or attacks [2] [8]. Others try to identify the protocol involved at the application level such as file transfer protocol (FTP), hypertext transfer protocol (HTTP), secure shell (SSH), Telnet [9] [14]. One particular study reviewed current traffic classification methods by classifying them into five categories: statistics based, correlation based, behaviour based, payload based,
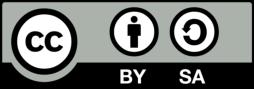
2. MATERIALS AND METHODS In this paper, we considered the following machine learning algorithms: decision tree, random forest, and eXtreme gradient boosting (XGBoost) because of their respective capacities to highlight the most discriminative features. We used the python programming language through libraries such as scikit learn and XGBoost to implement these different machine learning models. This section describes the dataset used, the performance metrics, and the methodology.
ISSN: 2252 8938 Int J Artif Intell, Vol. 11, No. 3, September 2022: 1175 1183 1176andport based [15]. Some studies [16], [17] have provided classification methods for encrypted traffic, which was challenging to perform in the past. Today port based analysis is ineffective, being unable to identify 30 70% of today’s internet traffic [5], [11]. This leads to the exploration of new features for traffic classification.Since the first studies on the statistical classification of internet traffic, the classification of network traffic using supervised and unsupervised machine learning techniques based on flow features such as average packet size, packet arrival times, and flow transmission times, have generated a lot of interest. These features are calculated over several packets grouped into a flow, and these sets of features are associated to the relevant flow class. Khandait et al. [14] inspected the few initial bytes of payload to determine the potential application. This study achieved an accuracy of 98%. Moore and Zuev [6] proposed a statistical approach to classify traffic into different classes of internet applications based on a combination of flow features such as the length of the flow, the time between consecutive flows, and the time between arrivals. The classification process uses a Bayesian classifier combined with a kernel density estimation which gives accuracy up to 95%. The models obtained are generally not very effective for certain types of traffic, such as attacks and P2P. However, they are particularly effective for web and mail traffic, which alone represent more than 94% of the data used for the study. Auld et al. [18] used a classification approach based on Bayesian neural networks to classify traffic into eight classes and present a traffic classifier that can achieve a high accuracy across various application types without any source or destination host address or port information. They achieved up to 99% accuracy for data trained and tested on the same day and 95% accuracy for data trained and tested eight months apart. Fan and Liu [19] used support vector machine (SVM) for internet traffic classification. Several SVM cores have been tested, and the most interesting one was the radial core. Several feature combinations were made from 30 features to create models. The most interesting one was a combination of 13 features which resulted in an overall accuracy of 98%. To ensure the stability of their model, an evaluation phase was carried out on a new dataset obtained later. Later, we compare our results against this study. Erman et al. [20] proposed a semisupervised traffic classification approach that combines unsupervised and supervised methods. This method achieved an accuracy of 94%. Li et al. [21] used the SVM in the classification of multi class network traffic. Thus, from nine features, they built a model capable of predicting six classes of traffic with an accuracy of 99.4%. Este et al. [22] proposed a two step approach to multi class traffic classification based on the SVM: a single class classification step followed by a multi class classification step that achieved an accuracy of around 90% for each class category (http, smtp, pop3, ftp, bittor, However,msn).itis well known that the characterization of the phenomenon to understand or the object to learn in a learning system is a critical step toward having a good classifier. Unfortunately, most existing works in the state of the art miss a formal study to ensure that the features used are informative and discriminating. Some are just based only on a limited number of features without explanation. It is sometimes difficult to identify the features and the properties that influence the results obtained. Moreover, it is difficult to know which features to combine to obtain a simple but efficient model. Note that some state of the art works, despite achieving good performance, fail to perform well on specific classes especially classes traffic related to bulk and attack.
In this paper, our contribution is twofold. Firstly, we studied the internet traffic features to select only relevant and informative enough by most machine learning algorithms. These selected features could be described as essential in the classification of internet traffic. Secondly, we made sure that we can detect any class of traffic, i.e., to be very efficient on all types of traffic thanks to our models. Our models will also be adaptable to data deficits. This means exploiting the available features for a given flow to predict its class without having all the features. The remainder of this paper is organized: section 2 presents the datasets used, the performance metrics used, and the methodology. Then section 3 shows results obtained, some comparisons with the existing works and conclusions to be drawn.
2.1. The dataset In this paper, the dataset used to develop and evaluate our models was collected by high performance monitors [23] at Queen Mary in University of London. The experimental site for collecting
Int J Artif Intell ISSN: 2252 8938
������������������ = ���� ����+���� (1) ������������ = ���� ����+���� (2) ���������������� = ����+���� ����+����+����+���� (3) ��1 ���������� =2∗ ������������������∗������������ ������������������+������������ (4) 2.3.
2.2.
Let true positive (TP) be the number of correct positive classifications, true negative (TN) the number of correct negative classifications, false positive (FP) be the number of incorrect positive classifications, and false negative (FN) be the incorrect negative classification. We consider four main classical machine learning performance metrics: precision, recall, accuracy, and F1 score. Precision (1) is the percentage of correct positive classifications (TP) from samples that are predicted as positive. Recall (2) is the percentage of correct positive classifications (TP) from samples that are actually positive. Accuracy (3) is the percentage of correct classifications from the overall number of samples. F1 score (4) is a combined metric that evaluates the trade off between precision and recall. Methodology We followed a traditional methodology for the machine learning task. The main steps are: Pre processing: cleaning, homogenization, and adaptation of the dataset to the learning algorithm. We replaced each missing quantitative value by the mean of the corresponding column and each qualitative missing value by the most frequent value of the corresponding column for the decision tree and random forest algorithms. It should be noted that, relying on the adaptability of the XGBoost algorithm to missing data, we did not perform any imputation for XGBoost.
data is a large research facility host to approximately 1,200 administrators, technical staff, and researchers. Full duplex gigabit ethernet is used on this site to connect to the internet. The traffic dataset is obtained based on full duplex traffic traces of the research facility over 24 hours. To build the sets of flows, the trace of each day was split into ten blocks of approximately 1,680 seconds (28 minutes). To provide a wider sample of mixing across the day, the beginning of each sample was selected randomly (uniformly distributed over the whole trace). The dataset consists of 377,526 flows, and each flow is characterized by 248 features described in [24]. Thus, we should have 377,526*248=93,626,448 values, but there are 1,105,574 missing values. These features include traffic statistics about inter packet time or packet size and information obtained from the transmission control protocol (TCP) headers, such as acknowledgment counts. Note that the features are provided in both directions. Flows in datasets are manually classified into ten broad traffic categories by applying a content based mechanism. The available traffic classes in this dataset are provided in Table 1. Each flow is mapped to one traffic class. Table 1 shows the number of flows by class/application in the dataset. Note that since game and interactive flows are not sufficient, our work was done on the eight other classes. To evaluate models efficiently, an eleventh block of data was obtained in the same way as the first ten blocks one year later at the same place. This 11th block of 19,626 flows is used for the evaluation phase in our study, as shown in Table 1. All these datasets are public, free to use for academic purposes, and available through a web link [25]
Features analysis of internet traffic classification using interpretable machine learning … (Erick A. Adje) 1177
Table 1. Dataset statistics with traffic classes application Traffic class Applications Nb of flows (10 blocks) Nb of flows (11th block) WWW Web 328,092 15,597 Mail SMTP, POP3, IMAP 28,567 1,799 Bulk FTP 11,539 1,513 Service DNS, X11, NTP 2,099 121 P2P BitTorrent, eDonkey 2,094 297 Database Mysql, Oracle 2,648 295 Multimedia Windows Media Player 576 0 Attack Virus, Worm 1,793 0 Interactive TELNET, SSH 110 4 Games World of Warcraft 8 0 Performance metrics
3.1. Decision tree Table 2 presents the results using the decision tree. We can see that, even without too many parameters, the decision tree is an efficient technique to solve traffic classification problems. Despite the considerable amount of missing data, which were filled, the decision tree can adapt and gives a good performance. The first model built by the decision tree with 248 features has revealed that: i) more than half (129) of the features provided zero information to the model; ii) 236 features had total importance of 1.38%, while the other 12 features provided a total of 98.62% information needed. Then, only the 12 most important features were considered. Note that the 12 feature model is better than the 248 feature model in terms of overall accuracy and specifically on traffic classes such as web, mail, bulk, P2P. In addition, this model consumes less memory space and is faster during the training than the 248 feature model. Table 2. Summary of performance obtained with decision tree Traffic class Test phase Evaluation phase 248 features 12 features 6 features 248 features 12 features 6 features WWW 99.85% 99.86% 99.85% 98.72% 99.76% 99.90% Mail 99.93% 99.97% 99.94% 99.77% 99.94% 95.83% Bulk 99.47% 99.83% 99.38% 83.21% 83.28% 83.61% Service 99.41% 99.17% 99.12% 99.17% 99.17% 99.17% Database 99.88% 99.88% 99.76% 98.98% 98.98% 98.98% P2P 98.53% 97.39% 96.08% 90.90% 93.93% 52.52% Attack 82.92% 82.54% 81.02% Multimedia 93.64% 94.21% 95.95% Overall accuracy 99.74% 99.76% 99.72% 97.52% 98.40% 97.53%
As explained in section 2 about materials and methods, we performed the experiments with three algorithms: decision tree, random forest, and XGBoost. For each algorithm, we used the classical grid search approach to find the best hyperparameters. In this section, we present the experimental results obtained during the test and evaluation phase. These results consist of the accuracy of each traffic class and the overall accuracy.
Repeating: we repeated steps 2 and 3 by considering the new reduced features until we got the fewest possible features with performance better or close to the performance of the model selected in the previous iteration.
1178Learning: for each algorithm, we varied several hyper parameters and trained the different models obtained on the same training set. 70% of total traffic is used for training and 30% for testing. Then we selected the best model based on the metrics used. Moreover, to ensure the efficiency of the model each time (evaluation phase), we test it again on a new dataset of 19,626 flows which is the eleventh block described before.
Features selection: for each model, we identified the importance of each of the 248 features and removed less important ones.
Considering the six most essential features, we have noticed a lot of loss performance in some traffic classes, in particular on P2P, which decreases from 93.93% to 52.52%. We have then considered this model less interesting for the next step. With the 12 feature model, we have noticed that two features have total importance of 92.77% against 7.23% for the other ten features. These two features are the port used at the server for the traffic with importance of 39.96% and the total number of bytes sent in the initial window, i.e., the number of bytes seen in the initial flight of data before receiving the first ack packet from the other endpoint (client to server) with importance of 52.81%. Table 3 shows the importance of the features in the 12 feature model building process.
3.2. Random forest From a random forest model of 248 features with an overall accuracy of 99.71%, the model of 23 features has an accuracy of 99.77%, which is the highest accuracy obtained during the test phase. However, the performance on the attack class is only about 71%. Let us note that the model with 23 features performs very well on the traffic class database during the evaluation phase (98.30% accuracy), knowing that at the beginning with 248 features, we have an accuracy of 38.30%. Subsequently, we have reduced the number of features to 17. The performance is very close to the 23 feature model and particularly a tiny improvement
ISSN: 2252 8938 Int J Artif Intell, Vol. 11, No. 3, September 2022: 1175 1183
3. EXPERIMENTAL RESULTS
Table 3. 12 feature decision tree model features with their importance on the model set up
As we can see, for a better adaptation of the XGBoost algorithm on traffic classification by exploiting a database with missing data, the more the algorithm has features of the flow to exploit, and the more the model obtained is better. Thus, we can hypothesize that if our models are used for real time classification, their efficiencies will depend on the number of the features captured at a specific time of the traffic processing and will improve as the traffic progresses. Future works could look at these aspects to confirm. However, it is worth remembering that even with few features, the models based on XGBoost are still very efficient. The experiments with 23 features highlight the importance of two features which are: the port number (server) with importance of 24.79% and the total number of bytes sent in the initial window (client to server) with importance of 47%. These two features contribute the most to the model construction, and
Table 4. Summary of performance obtained with random forest Traffic class Test phase Evaluation phase 248 ftrs 78 ftrs 23 ftrs 17 ftrs 248 ftrs 78 ftrs 23 ftrs 17 ftrs WWW 99.89% 99.91% 99.93% 99.91% 99.93% 99.97% 99.97% 99.97% Mail 99.94% 99.93% 99.93% 99.94% 90.88% 92.77% 99.94% 99.94% Bulk 99.66% 99.83% 99.72% 99.86% 99.34% 99.67% 99.73% 98.67% Service 99.27% 99.41% 99.41% 99.41% 99.17% 99.17% 99.73% 99.73% Database 99.16% 99.64% 99.76% 99.76% 38.30% 55.93% 98.30% 98.64% P2P 95.92% 96.90% 98.04% 97.23% 95.62% 98.65% 94.61% 96.30% Attack 71.53% 72.48% 72.86% 71.92% Multimedia 88.44% 94.21% 95.37% 94.22% Overall accuracy 99.71% 99.75% 99.77% 99.75% 97.52% 98.61% 99.85% 99.80%
Discriminators: importance (%) Discriminators: importance (%) Discriminators: importance (%) Port number (server): 18.78% The number of unique bytes sent (Server to Client): 3.47% The count of all the packets with at least a byte of TCP data payload (Server to Client): 1.56%
The minimum segment size (Client to Server): 13.61% The average segment size (Server to Client): 7.16% Initial window bytes (Client to Server): 20.32%
Variance of total bytes in IP packet (Server to Client): 4.90% The maximum segment size (Server to Client): 2.65% 3.3. XGBoost
The results obtained with the XGBoost models are presented in Table 6. Note that XGBoost can build models with missing data and still give good models. However, it is an algorithm that requires many features to perform well when dealing with missing data. The best model with XGBoost was obtained by considering 67 features. With 23 features, we have observed some more or less significant drops with an overall accuracy that went from 99.87% to 99.82% during the test phase and from 99.90% to 99.60% for the evaluation phase. The most remarkable drop is in the attack class, decreasing from 80.45% accuracy to 73.43% during the test phase. However, the 23 feature model still performs well on the other traffic classes.
Maximum of Ethernet data bytes (Server to Client): 4.07% Variance of Ethernet data bytes (Server to Client): 5.53%
The total number of Round Trip Time (RTT) samples found (Client to Server): 1.66% Maximum of Ethernet data bytes (Client to Server): 2.40% Maximum of total bytes in IP packet (Client to server): 2.13%
once again on the database traffic, which has increased to 98.64% accuracy during the evaluation phase.
The variance of total bytes in IP packet: 0.43% The theoretical stream length (Client to Server): 1.10% The theoretical stream length (Server to Client): 1.30%
Int J Artif Intell ISSN: 2252 8938
Maximum of total bytes in IP packet (Server to Client): 3.07%
Discriminators: importance (%) Discriminators: importance (%) Discriminators: importance (%) Port number (server): 39.96% Port number (client): 1.32% Maximum of bytes in Ethernet packet: 0.5% Variance of control bytes packet: 0.44% Number of pushed data packets (Client to Server): 1.51% Minimum segment size (Client to Server): 0.20% Average segment size (Client to Server): 0.20% Average segment size (Server to Client): 0.23% Initial window bytes (Client to Server): 52.81%
Table 5. 17 feature random forest model features with their importance on the model set up
Features analysis of internet traffic classification using interpretable machine learning … (Erick A. Adje) 1179
Table 4 presents the results using random forest, and Table 5 shows the importance of the features in the 17 feature model building process.
Initial window bytes (Server to Client): 5.32% The theoretical stream length (Server to Client): 2.13% Total data transmit time (Client to Server): 1.23%
The total number of Round Trip Time (RTT) samples found (Server to Client): 0.48% Maximum of Ethernet data bytes (Client to Server): 0.65% Mean of total bytes in IP packet (Client to Server): 1.19% Maximum of total bytes in IP packet (Client to server): 0.40% Variance of total bytes in IP packet (Client to server): 0.44% Median of Ethernet data bytes (Server to Client): 0.47% Maximum of Ethernet data bytes (Server to Client): 1.13% FFT of packet IAT, Frequency #2 (Client to Server): 0.38% 3.4. Overall discussions
Table 7. 23 feature XGBoost model features with their importance on the model set up
The results show that each algorithm used has its particularities, weaknesses, and strengths. For example, the decision tree was more efficient on attack traffic than the other algorithms. But it gave less interesting results for bulk traffic during the test phase and generally performed worse than other algorithms. The random forest is the algorithm that achieved good results in both the test and evaluation phases with the least number of features but remained less efficient on attack traffic. XGBoost gives a lower performance as we reduce the features. This can be reflected in the data gaps. It then looks at other features to improve performance. In general, XGBoost performed better in our study when considering 67 features.
3.5. Comparison of our results with the state-of-the-art We compare our results to the [19] ones as the context of the studie is the same and they used the same datasets as in our study with good performance. We use our minimal models for each algorithm: the 12 feature decision tree model, the 23 feature XGBoost model, and the 17 feature random forest model. In [19] use SVM for this task. Several SVM cores have been tested and the most interesting one was the radial core. The most interesting model obtained was a combination of 13 features which resulted in an overall accuracy of 98%. However, with our 12 feature decision tree model, we obtained an overall accuracy of 99.76%, which represents fewer features for more performance. We also obtained higher overall accuracies from the other models despite requiring more features (99.75% for the 17 feature random forest
Discriminators: importance (%) Discriminators: importance (%) Discriminators: importance (%) Port number (server): 18.05% Minimum of bytes in Ethernet packet: 1.10% Maximum of bytes in Ethernet packet: 0.47% Mean of total bytes in IP packet: 0.64% The number of unique bytes sent (Client to Server): 2.51% The count of all the packets with at least a byte of TCP data payload (Client to Server): 1.96%
ISSN: 2252 8938 Int J Artif Intell, Vol. 11, No. 3, September 2022: 1175 1183 1180further confirming again their importance in internet traffic classification. Table 7 shows the importance of the features in the 23 feature model building process. Table 6. Summary of performance obtained with decision tree Traffic class Test phase Evaluation phase 248 features 67 features 23 features 248 features 67 features 23 features WWW 99.97% 99.96% 99.97% 99.99% 99.99% 99.99% Mail 99.97% 99.98% 99.98% 99.94% 99.77% 99.97% Bulk 99.97% 99.97% 99.86% 99.93% 99.87% 96.16% Service 99.56% 99.56% 99.41% 95.04% 97.52% 96.69% Database 99.88% 99.88% 99.88% 98.64% 98.64% 98.64% P2P 98.86% 98.86% 98.20% 96.96% 97.31% 96.30% Attack 80.45% 80.45% 73.43% Multimedia 97.69% 98.26% 96.53% Overall accuracy 99.86% 99.87% 99.82% 99.89% 99.90% 99.60%
We obtained an overall accuracy of 99.87% for the test phase and 99.90% for the evaluation phase, representing our best results during the study. Each algorithm works based on the features it considers most discriminating. Nevertheless, several features are very often found in the top 20 features of either two or all three algorithms considered. These features summarized in Table 8 can be considered as essential features for the classification of internet traffic.
If the endpoint requested Window Scaling/Timestamp options as specified (Server to Client): 1.32% Minimum segment size (Client to Server): 2.57% Average segment size (Client to Server): 2.27% Initial window bytes (Client to Server): 55.81% Initial window bytes (Server to Client): 1.31% The theoretical stream length (Client to Server): 2.27% The theoretical stream length (Server to Client): 1.85% The missed data, calculated as the difference between the ttl stream length and unique bytes sent (Server to Client): 2.09% Total data transmit time (Server to Client): 0.60%
Port number (server) Mean of total bytes in IP packet
Maximum of total bytes in IP packet (Client to Server) Mean of total bytes in IP packet (Client to Server)
Int J Artif Intell ISSN: 2252 8938
Initial window bytes (Client to Server) Initial window bytes (Server to Client)
Table 9. Comparison of precision with the work of [19] during the evaluation phase
Minimum segment size (Client to Server) The average segment size observed during the lifetime of the connection (Client to Server)
Traffic class Fan and Liu [19] Decision tree Random forest XGBoost WWW 98.72% 99.76% 99.97% 99.99% Mail 97.10% 100% 99.94% 100% Bulk 71.91% 82.94% 99.73% 96.03% Services 55.37% 99.97% 99.97% 96.69% P2P 54.55% 93.34% 94.61% 95.62% Database 51.52% 98.98% 98.30% 98.30%
In this paper, we have considered three interpretable machine learning algorithms: decision tree, random forest, and XGBoost. These algorithms, thanks to their ability to quantify the importance of a feature in the model, have allowed identifying some essential features for a traffic classification problem. This allowed us to identify a set of 14 features that are considered the most important by most of our algorithms. Two of these 14 features were revealed to be crucial because of their high importance rates each time a model was built. These are the port number (server) and the minimum segment size (client to server). This step of reducing the features to the important ones allowed us to achieve high performance with very few features. Our 12 feature model built using the decision tree is a good example because, with only 12 of the 248 features, we obtained an overall accuracy of 99.76% in the test phase and 98.40% on a new dataset in the evaluation phase. With this model, we get better results than some existing works that use more features for less performance. This confirms once again the relevance of the study performed on the features. Let us note that XGBoost can be efficient in the classification of real time traffic thanks to its ability to give good
The average segment size observed during the lifetime of the connection (Server to Client) Maximum of Ethernet data bytes (Client to Server)
Table 11. Comparison of f1 score with the work of [19] during the evaluation phase
Table 10. Comparison of recall with the work of Fan et al. during the evaluation phase
Traffic class Fan and Liu [19] Decision tree Random forest XGBoost WWW 97.36% 99.76% 99.97% 99.82% Mail 85.22% 99.97% 99.86% 99.92% Bulk 69.53% 90.68% 99.57% 97.75% Services 69.43% 99.97% 99.97% 98.32% P2P 53.67% 93.00% 96.23% 94.98% Database 63.86% 98.15% 97.97% 99.14%
Features analysis of internet traffic classification using interpretable machine learning … (Erick A. Adje) 1181 model and 99.82% for the 23 feature XGBoost model). Tables 9 11 summarise [19]. Results and ours when using the eleventh block. In general, we notice that whatever the considered model our results are better. Moreover, for traffic such as P2P, we have better precision with our models. It is important to mention that 5 of the decision tree model features, as shown in Table 3 are commons to some most discriminating features in the [19]. Study, which once again confirms the importance of some specific features in the classification of internet traffic. However, the use of the other 7 features made the difference and allowed us to achieve better results compared to the study of [19]. Therefore, our analysis of the features was very interesting.
The variance of total bytes in IP packet (Client to Server) Maximum of Ethernet data bytes (Server to Client)
Traffic class Fan and Liu [19] Decision tree Random forest XGBoost WWW 96.03% 99.75% 99.97% 99.65% Mail 75.93% 99.94% 99.78% 99.83% Bulk 69.58% 100% 99.40% 99.52% Services 93.05% 99.97% 99.97% 100% P2P 52.82% 92.08% 97.91% 94.35% Database 83.97% 97.33% 100% 100%
4. CONCLUSION
Table 8. Top 14 of most important features common to the considered algorithms Discriminators Discriminators
Number of unique data bytes (Client to Server) If the endpoint requested Window Scaling/Timestamp options as specified (Server to Client)
[25] A. W. Moore and D. Zuev, “Internet traffic classification using bayesian analysis techniques,” [Dataset], University of Cambridge, 2005. (Accessed: July 17, 2020). [Online]. Available: https://www.cl.cam.ac.uk/research/srg/netos/projects/archive/nprobe/data/papers/sigmetrics/.
REFERENCES
[2] B. C. Park, Y. J. Won, M. S. Kim, and J. W. Hong, “Towards automated application signature generation for traffic identification,” in NOMS 2008 2008 IEEE Network Operations and Management Symposium, 2008, pp. 160 167, doi: 10.1109/NOMS.2008.4575130.
[8] A. McGregor, M. Hall, P. Lorier, and J. Brunskill, “Flow clustering using machine learning techniques,” in Proceedings of the 5th International Conference on Passive and Active Network Measurement, 2004, pp. 205 214.
[11] J. Ma, K. Levchenko, C. Kreibich, S. Savage, and G. M. Voelker, “Unexpected means of protocol inference,” in Proceedings of the 6th ACM SIGCOMM on Internet measurement MC ’06, 2006, p. 313, doi: 10.1145/1177080.1177123.
[19] Z. Fan and R. Liu, “Investigation of machine learning based network traffic classification,” in 2017 International Symposium on Wireless Communication Systems (ISWCS), Aug. 2017, pp. 1 6, doi: 10.1109/ISWCS.2017.8108090.
[16] E. Mahdavi, A. Fanian, and H. Hassannejad, “Encrypted traffic classification using statistical features,” isecure, vol. 10, no. 1, pp. 29 43, 2018.
[13] C. Kohnen, C. Uberall, F. Adamsky, V. Rakocevic, M. Rajarajan, and R. Jager, “Enhancements to statistical protocol identification (SPID) for self organised QoS in LANs,” in 2010 Proceedings of 19th International Conference on Computer Communications and Networks, Aug. 2010, pp. 1 6, doi: 10.1109/ICCCN.2010.5560139.
[5] A. Madhukar and C. Williamson, “A longitudinal study of P2P traffic classification,” in 14th IEEE International Symposium on Modeling, Analysis, and Simulation, pp. 179 188, doi: 10.1109/MASCOTS.2006.6.
[3] A. Callado et al., “A survey on internet traffic identification,” IEEE Communications Surveys and Tutorials, vol. 11, no. 3, pp. 37 52, 2009, doi: 10.1109/SURV.2009.090304.
[15] J. Zhao, X. Jing, Z. Yan, and W. Pedrycz, “Network traffic classification for data fusion: A survey,” Information Fusion, vol. 72, pp. 22 47, Aug. 2021, doi: 10.1016/j.inffus.2021.02.009.
[1] M. Zhang, W. John, K. Claffy, N. Brownlee, and U. S. Diego, “State of the art in traffic classification: A research review,” 10th International Conference on Passive and Active Network Measurement Student Workshop, pp. 1 2, 2009.
ISSN: 2252 8938 Int J Artif Intell, Vol. 11, No. 3, September 2022: 1175 1183 1182results even with missing data. Future studies will focus on real time traffic classification and the effectiveness of XGBoost on this type of problem.
[10] L. Bernaille, R. Teixeira, I. Akodkenou, A. Soule, and K. Salamatian, “Traffic classification on the fly,” ACM SIGCOMM Computer Communication Review, vol. 36, no. 2, pp. 23 26, Apr. 2006, doi: 10.1145/1129582.1129589.
[17] V. A. Muliukha, L. U. Laboshin, A. A. Lukashin, and N. V. Nashivochnikov, “Analysis and classification of encrypted network traffic using machine learning,” in 2020 XXIII International Conference on Soft Computing and Measurements (SCM), May 2020, pp. 194 197, doi: 10.1109/SCM50615.2020.9198811.
[23] A. W. Moore, J. Hall, C. Kreibich, E. Harris, and I. Pratt, “Architecture of a network monitor,” Passive and Active Measurement Workshop, 2003, [Online]. Available: http://citeseerx.ist.psu.edu/viewdoc/summary?doi=10.1.1.12.8563.
[22] A. Este, F. Gringoli, and L. Salgarelli, “Support vector machines for TCP traffic classification,” Computer Networks, vol. 53, no. 14, pp. 2476 2490, Sep. 2009, doi: 10.1016/j.comnet.2009.05.003.
[12] P. Haffner, S. Sen, O. Spatscheck, and D. Wang, “ACAS,” in Proceeding of the 2005 ACM SIGCOMM workshop on Mining network data MineNet ’05, 2005, p. 197, doi: 10.1145/1080173.1080183.
[6] A. W. Moore and D. Zuev, “Internet traffic classification using bayesian analysis techniques,” in Proceedings of the 2005 ACM SIGMETRICS international conference on Measurement and modeling of computer systems SIGMETRICS ’05, 2005, p. 50, doi: 10.1145/1064212.1064220.
[7] A. W. Moore and K. Papagiannaki, “Toward the accurate identification of network applications,” in Proceedings of the Passive and Active Measurement Workshop, 2005, pp. 41 54.
[14] P. Khandait, N. Hubballi, and B. Mazumdar, “Efficient keyword matching for deep packet inspection based network traffic classification,” in 2020 International Conference on COMmunication Systems and NETworkS (COMSNETS), Jan. 2020, pp. 567 570, doi: 10.1109/COMSNETS48256.2020.9027353.
[20] J. Erman, A. Mahanti, M. Arlitt, I. Cohen, and C. Williamson, “Semi supervised network traffic classification,” in Proceedings of the 2007 ACM SIGMETRICS international conference on Measurement and modeling of computer systems SIGMETRICS ’07, 2007, p. 369, doi: 10.1145/1254882.1254934.
[4] N. B. Azzouna and F. Guillemin, “Analysis of ADSL traffic on an IP backbone link,” in GLOBECOM ’03. IEEE Global Telecommunications Conference (IEEE Cat. No.03CH37489), pp. 3742 3746, doi: 10.1109/GLOCOM.2003.1258932.
[18] T. Auld, A. W. Moore, and S. F. Gull, “Bayesian neural networks for internet traffic classification,” IEEE Transactions on Neural Networks, vol. 18, no. 1, pp. 223 239, Jan. 2007, doi: 10.1109/TNN.2006.883010.
[21] Z. Li, R. Yuan, and X. Guan, “Accurate classification of the internet traffic based on the SVM method,” in 2007 IEEE International Conference on Communications, Jun. 2007, pp. 1373 1378, doi: 10.1109/ICC.2007.231.
[9] J. Erman, M. Arlitt, and A. Mahanti, “Traffic classification using clustering algorithms,” in Proceedings of the 2006 SIGCOMM workshop on Mining network data MineNet ’06, 2006, pp. 281 286, doi: 10.1145/1162678.1162679.
[24] A. Moore, D. Zuev, and M. Crogan, “Discriminators for use in flow based classification,” Queen Mary and Westfield College, Department of Computer Science, no. August, pp. 1 14, 2005, doi: 10.1.1.101.7450.
Erick A. Adje was born in Cotonou, Benin on January 13, 1996. He received Engineer degree in Computer Science and Telecommunications (from Ecole Polytechnique d'Abomey Calavi, UAC) in 2019. In December 2021, he obtained a Research Master degree in Computer Science (from Ecole Doctorale des Sciences de l’Ingénieur, UAC). Currently, he is a freelance software developer and member of the Association for the Advancement of Artificial Intelligence (AAAI) Benin chapter. His research interests include IA, machine learning and computer vision. He can be contacted at email: erickadje96@gmail.com.
BIOGRAPHIES OF AUTHORS
Int J Artif Intell ISSN: 2252 8938 Features analysis of internet traffic classification using interpretable machine learning … (Erick A. Adje) 1183
Michel Dossou was born in Cotonou, Benin on June 12, 1982. He received the B.S. and M.S. degrees in electrical engineering from the Polytechnic faculty of Royal Military Academy (RMA), Brussels, BELGIUM in 2003 and 2006, and the Ph.D. degree in non linear optics from the University of Lille, FRANCE in 2011 with a scholarship from the Centre National de la Recherche Scientifique (CNRS). From 2011 to 2012, he was a Research Assistant with the PhLAM (Physique des Lasers, Atomes et Molécules) laboratory of the University of Lille, FRANCE. In 2012, he joined the Polytechnic School of Abomey Calavi, becoming Assistant Professor in 2015. Since 2019, he has been appointed Associate Professor with the Electrical Engineering Department from the University of Abomey Calavi, Benin. He is the author of more than 30 articles. His research interests include optical fibre technology, wireless communications and broadcasting. Dr Dossou was awarded the 2006 Best M.S. dissertation Award of the Association of Engineers from RMA. He can be contacted at email: michel.dossou@epac.uac.bj.
Vinasetan Ratheil Houndji received a Ph.D. in Computer Science (from Université catholique de Louvain UCL, Belgium and Université d’Abomey Calavi UAC, Benin) in 2017 after obtaining a Master of Science degree in Computer Science (from Ecole Polytechnique de Louvain, UCL, Belgium) in 2013 and an Engineer degree in Computer Science and Telecommunications (from Ecole Polytechnique d’Abomey Calavi, UAC) in 2011. He co founded the company Machine Intelligence For You (MIFY) in 2017 and spent one year as Chief Executive Officer of this company. Currently, he is senior lecturer at UAC, mainly in Artificial Intelligence and Combinatorial Optimization. He is also chair of the Association for the Advancement of Artificial Intelligence (AAAI) Benin chapter. He can be contacted at email: ratheil.houndji@uac.bj.
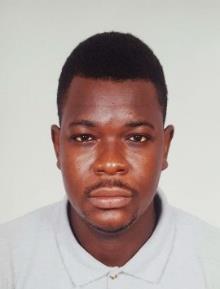



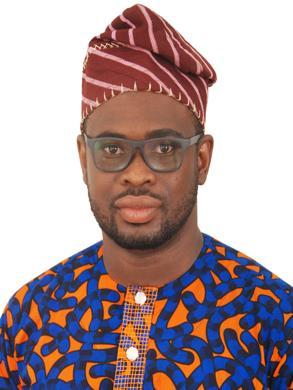



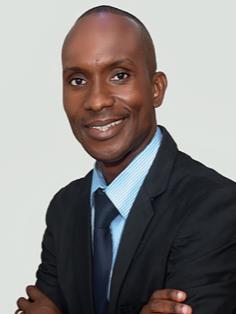



Article Info ABSTRACT
This is an open access article under the CC BY SA license. Corresponding Author: Baraa M. DepartmentAbedofComputer Science, College of Computer Science and Information Technology, University of Email:Ramadi,AnbarIraqburasoft@gmail.com
1. INTRODUCTION
Previously, using robot implemented only in the manufacturing industry. However, nowadays, mobile robots are commonly used in diverse fields such as entertainment, medicine, mining, rescue, education, military, aerospace and agriculture. Smart equipment enables mobile robots to model the environment, determine its location, control movement and discover obstacles while carrying out its tasks. These functions can be performed through navigation technology, of which the most important function is to plan a safe path by detecting and avoiding obstacles when moving from one point to another. Therefore, in planning the robot path for the customer in an environment, whether simple or complex, the most important step is the correct choice of navigation technology [1], [2]. Path planning can be divided into two types based on the robot's knowledge of its environment: global (or offline) and local (or online). Mobile robots have complete knowledge of their environment when it comes to global path planning. Before the robot starts moving, the algorithm generates a complete path for it to follow. Local path planning is performed by mobile robots that have no prior knowledge of their environment and rely on a local sensor to collect data and then construct a new path in response [3]. Depending on the target type: static or dynamic, the above classification can be subdivided. In static targets, the mobile robot looks for a static point in its workspace, but for dynamic targets, it searches for a moving point while avoiding obstacles [4] The path planning algorithms for those
Article history: Received Oct 30, 2021 Revised May 15, 2022 Accepted Jun 13, 2022 Path planning or finding a collision free path for mobile robots between starting position and its destination is a critical problem in robotics. This study is concerned with the multi objective optimization path planning problem of autonomous mobile robots with moving targets in dynamic environment, with three objectives considered: path security, length and smoothness. Three modules are presented in the study. The first module is to combine particle swarm optimization algorithm (PSO) with bat algorithm (BA). The purpose of PSO is to optimize two important parameters of BA algorithm to minimize distance and smooth the path. The second module is to convert the generated infeasible points into feasible ones using a new local search algorithm (LS). The third module obstacle detection and avoidance (ODA) algorithm is proposed to complete the path, which is triggered when the mobile robot detects obstacles in its field of vision. ODA algorithm based on simulating human walking in a dark room. Several simulations with varying scenarios are run to test the validity of the proposed solution. The results show that the mobile robots are able to travel clearly and completely safe with short path, and smoothly proving the effectiveness of this method.
Journal homepage: http://ijai.iaescore.com
Keywords: Bat PathParticleMultiMovingDynamicalgorithmenvironmenttargetobjectiveoptimizationswarmoptimizationplanning
Multi-objective optimization path planning with moving target
IAES International Journal of Artificial Intelligence (IJ AI) Vol. 11, No. 3, September 2022, pp. 1184~1196 ISSN: 2252 8938, DOI: 10.11591/ijai.v11.i3 pp1184 1196 1184
Baraa M. Abed, Wesam M. Jasim Department of Computer Science, College of Computer Science and Information Technology, University of Anbar, Ramadi, Iraq
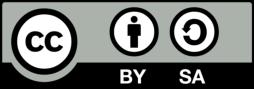
Multi objective optimization path planning with moving target (Baraa M. Abed) 1185 scenarios are different. Path planning problems in a variety of fields have been solved using various methods such as cell decomposition and roadmap approaches., the main disadvantages of this method include inefficiencies due to high processing fees and unreliability due to significant risks of being caught in local minima. These limitations can be overcome by using different heuristic techniques, such as neuronal pathways, genetics, or nature inspired algorithms [5] Several techniques have been used to solve the path planning problem, for a variety of reasons, including safety or single objective optimisation for the shortest path, these methods such as bacterial foraging optimization (BFO) [6], bat algorithm (BA) [7], cuckoo search (CS) [8], whale optimization algorithm (WOA), particle swarm optimization (PSO) [9], and artificial immune systems (AIS) [10]. Additionally, fuzzy logic and neural networks have been used. Another objective of these solutions is multi objective optimization, which tries to satisfy requirements for the shortest and smoothest path in static or dynamic environments. In this study, the previous research that's tried to solve the problem of multiobjective optimization were reviewed For example, Li and Chou [11] employed self adaptive learning PSOs to attain three objectives: path length, collision risk degree, and smoothness. In order to improve the search capability of a PSO, this mechanism selects the most suitable search strategies dynamically at various stages of the optimization process. An alternative algorithm for multi objective mobile robot path planning uses an improved genetic algorithm [12] to find three types of objects for planned paths: length, smoothness, and security. By considering a Mars Rover scenario, Guimari in [13] used A* search algorithm to minimise the path difficulty, danger, elevation and length from a starting point to a destination. A novel multi objective optimization method that uses the WOA is also presented for planning mobile robot paths [14]. The two distance and smooth path criteria of robot path planning are transformed into a minimisation process. In WOA, the solution fitness is determined by the target and obstacle positions in the environment. A multi objective approach based on the shuffled frog leaping algorithm (MOSFLA) was proposed in [15], that is, the natural behaviour of frogs. Three distinct path objectives are considered: safety, length and smoothness. The results are compared with the well known and most commonly used Non dominated Sorting Genetic Algorithm II. In [16], they were also explored the natural flashing behaviour of fireflies and proposed the multi objective firefly algorithm (MO FA). This method addresses three distinct goals: path safety, length and smoothness (related to energy consumption). Furthermore, eight realistic scenarios are used to calculate the path to test the proposed MO FA. An elitist multi objective approach based on coefficients of variation was proposed in [17] The Intelligent water drops (IWD) algorithm was used in the calculation of the Pareto front. Known as CV based MO IWD, this method aims to optimize two goals: path length and safety To optimise the cost performance index with the multi objective fuzzy optimisation strategy and Voronoi diagram method, A new approach was developed in the [18] to improve the performance index, which can coordinate the weight conflict of the sub objective functions. A global path planning algorithm for wheeled robots that utilizes multiobjective memetic algorithms (MOMA) that optimizes several objectives simultaneously, including path length, smoothness, and safety was discussed in [19]. Two MOMA are utilized based on conventional multi objective genetic algorithms combined with elitist, non dominant sorting and decomposition strategies for optimizing the path length and reducing smoothness simultaneously.
To improve the algorithms search capabilities and to ensure the safety of the obtained candidate paths, new path encoding schemes, path refinements, and specific evolutionary operators are designed and introduced in the MOMA. A new method based on Region of Sight is also presented in [20] with the primary goal to easily and rapidly address path planning,the method attempts to meet various robot movement requirements, such as shortest path length, safety and smoothness, while using the least amount of time.An optimization algorithm known as using grey wolf optimization algorithm (GWO) [21] is introduced to solve the problems with multiple objectives. GWO integrates a fixed sized external archive to save and retrieve the pareto optimal solutions, then define the social hierarchy and simulate the hunting behavior of grey wolves in a multi objective search Hybridisationspace.isalso used to improve the performance of path planning meta heuristic algorithms. With hybridisation, the benefits of two or more meta heuristic algorithms are combined to create a better technique. A hybrid multi objective based on the bare bones PSO with differential evolution was illustrated in [22]. To achieve different types of optimisation, Geetha et al. in [23] proposed a path planning algorithm based on Ant Colony Optimisation and Genetic algorithm. The objectives for planned paths include length, smoothness and security. Ajeil et al. [24] optimised paths by conducting a hybridised PSO modified frequency bat (PSO MFB) algorithm. A path was optimized using the hybridisation of Grey Wolf Optimiser PSO algorithm in [25]. Oleiwi et al. in [26] proposed new hybrid approach based on enhanced genetic algorithm based on the modified search A* algorithm and fuzzy logic. However, these studies are mainly focus to solve single /multi objective but they did not take into account the change of location of the target. In reality, the target location commonly changes in time. For example, unmanned aerial vehicles flow target.
Int J Artif Intell ISSN: 2252 8938
The environment represents as room that contains varying shapes of dynamic and static obstacles with different shapes and sizes The two mobile robots are considered as points given their physical bodies, as shown in Figure 1. The motion of mobile robots is not affected by kinematic constraints. Both robot are free to move in any direction at any time.
The primary goal of path planning is to generate a set of reference points that will carry a robot from a starting point to a destination point. In addition, this path is collision free while trying to meet predefined optimization criteria. Some of these criteria are; maximum safety, lowest energy consumption, shortest path, shortest time, and smoothest path. The optimization criteria can be details as following: 2.1.1. Shortest path
Consider a workspace in which a mobile robot is in a start position , and must reach a goal position. This robot referred to as the target for another robot referred to as the second robot. The second robot in a different start position must reach the target robot. Mobile robots' workspaces are assumed to contain a variety of static and dynamic obstacles. The purpose of path planning is to determine the optimal or near optimal paths (which are the safest, shortest, and smoothest) for the second mobile robot to follow in order to reach the target robot. Several assumptions in this study require clarification to avoid colliding with environmental obstacles.
This paper presents a novel path planning algorithm to solve multi objective optimisation path planning problems with moving targets in unknown dynamic environments. The new path planning algorithm achieves safety, shortness, and smoothness It consists of three modules as follows; firstly, a combination of BA and PSO algorithms is used to select points that are short and smooth enough to satisfy the proposed multi objective measures. The PSO is used to optimise the BA parameters, which generates the path points. Secondly, to make the infeasible solutions feasible, new LS is used. Thirdly, to achieve the objective, the novel algorithm is applied to detect and avoid the obstacles.
ISSN: 2252 8938 Int J Artif Intell, Vol. 11, No. 3, September 2022: 1184 1196 1186
The first robot must be avoiding obstacle but the second robot not only avoid obstacle it must flow the best path according to the criteria (safety, smoothness, shortest).
Figure 1. Physical mobile robots
Basically, this is the shortest distance between the starting point and the destination, calculated by summarizing the midpoints (p (1), p (2),……,p(N)) produced by a path planning algorithm between a Start Point that is given by p(1) and the Goal Point that is denoted by p(N). Figure 2 illustrates this measurement in detail. The (1): ��1(��,��) =��((����(��),��(��)) (1) d (.,.) corresponds to Euclidean distance between two points. Taking the sum of all distances from the Start Point p (1) to the Goal Point p(N), the shortest path length (SPL) for an exercise is calculated.
������ =∑ ��(����(��)�� 1 ��=1 ,����(��+1)) (2)
To the best of our knowledge, this is the first use of the hybrid BA and PSO algorithm in multi objective robot path planning with moving target. The remaining of the paper is organized as follows; the problem statement is presented in section 2. Section 3 shows the detailes of swarm intelligence and the proposed approaches are presented in section 4. In section 5, the work of obstacle detection and obstacles avoiding is presented. The simulation results are discussed in section 6 and the main conclusions are presented in section 7.
2. PROBLEM STATEMENT AND ASSIMPTIONS
2.1. Performance objectives of mobile robots
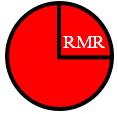
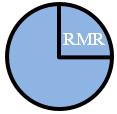
Int J Artif Intell ISSN: 2252 8938
The values of ��1 and ��2 represent the relative importance of the two objectives, respectively. The following requirements must be met by their respective values: ��1 + ��2 = 1 (5) The overall fitness function is defined as shown in (6): �������������� = 1 ��(��,��)+�� (6) The e factor prevents division by zero (e.g., e = 0.001). In each iteration, an optimal solution among competing options can be selected by determining the balance of the two performance objectives stated in (1) and (3). Figure 4 shows that the best point among four competing points is p2 for the t and p3 for the (t + 1) iterations, on the other hand, in the second iteration (t + 2) the distances are shorter for p1 and p4, but the
Multi objective optimization path planning with moving target (Baraa M. Abed) 1187
A detailed description of this measurement details in Figure 2 and Figure 3.
���� = √(������+1(��+1) ������(��))2 +(������+1(��+1) ������+1(��))22 2.1.2. Path smoothness Its consider one of the important optimization criteria. The smoothness of the path is normally measured by reducing as much as possible the angle difference between the current goal and the current position produced. Two lines intersect at this angle, which is then used to determine the robot's location at the conclusion of the process. ��2(��,��) =∑ |��(����(��),����(��+1)) ��(����(��),��(��))|�� 1 ��=1 (3) where, �� (����(��),����(��+1)=tan 1������(t+1) ������(��) ������(t+1) ������(��) �� (����(��),����)=tan 1����(��) ������(��) ����(��) ������(��)
Figure 2. Measurement description Figure 3 Candidate solutions angles at iteration t Figure 3 shows that: θ1 represents the angles between line segments (��(0),��(��)) and ��(0),��(1)); Θ2 represents the angles between line segments (��(0),��(��)) and ��(0),��(2)); θ3 represents the angles between line segments (��(0),��(��)) and ��(0),��(3)).
The index i represent the best solution found by the path planning algorithm.
Clearly, among the competing points (��1, ��2, ��3), ��2 has the minimum angle θ2. Overall, multi objective optimization is a balance between distance and angle of path objectives discussed above. However, finding the candidate with both advantages is difficult, and thus the two objectives are combined using the weight to calculate the fitness value, as �� =(��,��)=��1��1(��,��)+��2��2(��,��) (4)
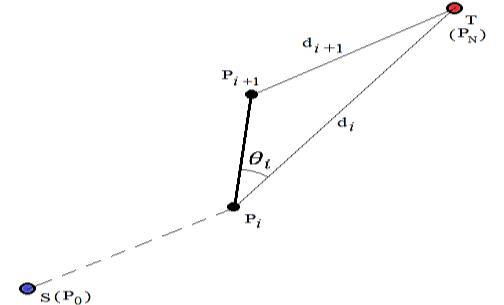
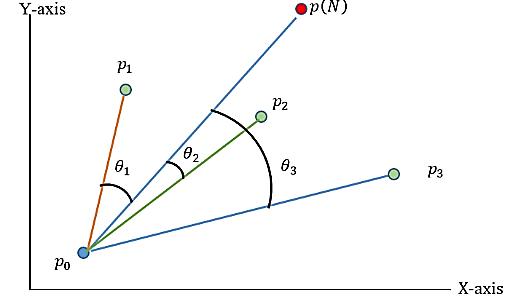
Swarm intelligence models are referred to as mathematical representations of natural swarm systems. Swarm intelligence models have been proposed in literature and been successfully applied in various real world scenarios. In the present study, two such algorithms are used to solve the path planning problem which are PSO and BA algorithms.
ISSN: 2252 8938 Int J Artif Intell, Vol. 11, No. 3, September 2022: 1184 1196 1188angles are larger, therefore p2 is chosen because it strikes a balance between the two criteria. This procedure is repeated until the GP is
3. SWARM INTELLIGENCE MODELS
objective path planning using mid point selection
Figurelocated.4.Multi
In our proposed system, the distance and angle are normalised below to improve the method performance, new_distance = distance/ST, new_angle = angle/90, Where ST is the distance from the starting point to the destination. The distance varies in the range [0, ST], and is thus normalised by ST. The angle varies in the range [0, +90], and is thus normalised by 90º. Near the target, the normalised distance is nearly 0 while the normalised angle is in the range [0, 1]. In this case, the effect of the angle is greater than that of the distance, preventing the robot from arriving at the target and merely vibrates around it. Therefore, distance normalisation is slightly modified to avoid this phenomenon. In other words, the normalised distance is in the range [0.3, 1.0]. Subsequently, although the robot is near the target, the normalised distance is greater than 0 and the above phenomenon can be avoided.
2.1.3. Movement of obstacles In dynamic environment there are moving obstacle. During each time step, the position of the obstacle changes. According to this study, dynamic obstacles move linearly, that is, along a straight line, with a velocity. �������� and direction (��������) defined by (7) and (8). �������������� =�������������� + �������� ×�������������� (7) �������������� =�������������� + �������� × �������������� (8) where �������� is the slope of the linear motion
3.1. PSO algorithm Inspired by swarm intelligence, Eberhart and Kennedy [27] developed PSO as an optimisation algorithm in 1995 to mimic the behaviour of social animals. A flock of birds that flies to find food does not need leaders, but rather follows the member closest to the food. Therefore, the flock of birds receives the solution they require through effective communication with members of the population. PSO algorithms also use particles to represent candidate solutions, each with a position ���� and velocity ���� corresponding to the candidate. Position refers to the solution offered by a particle, and velocity refers to how rapidly it moves relative to its current position. Here, both values (position and velocity), are randomized and the PSO constructs the solution in two phases [28]. The velocity of each particle is updated by (9). ����(�� + 1)= ����(��)+ ��1��1 (�������������� ����)+��2��2(���������� ����) (9)
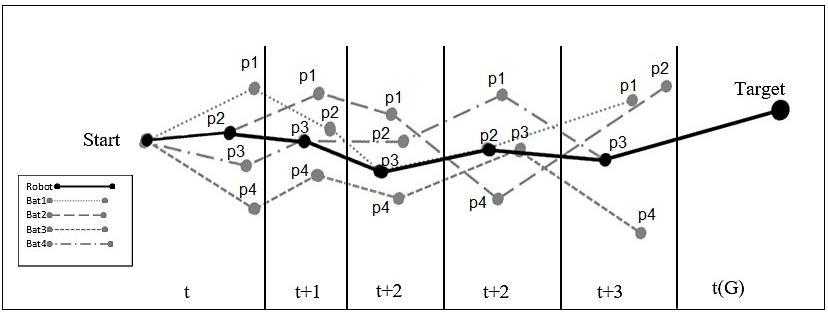
Int J Artif Intell ISSN: 2252 8938 Multi objective optimization path planning with moving target (Baraa M. Abed) 1189 where ����(t) represents the particle velocity, ����(��) represents the particle position, pbest represents the best position of the individual, gbest represents the best position in the group, c1 and c2 represent the constant and r represents the random number [0, 1]. The particle position is updated by (10).
����(��+1)=������(��) (15) ����(��+1)=����(0)[1 exp( ����)] (16) where 0<�� <1 and �� >0 4. PROPOSED METHOD
In order to provide an effective mechanism to control the exploration and exploitation and switch to exploitation stage, when necessary, we have to vary the loudness ���� and pulse rate ����.After each iteration, the level of loudness and pulse rate needs an update. When a bat has located its prey loudness usually decreases, whereas the rate of pulses is increased in accordance with these (15) and (16).
����(�� + 1)= ����(��)+(����(��) ��∗)���� (12)
This section describes the the path planning algorithm as an optimization technique that can help the robot to find its path from the initial position to goal position in static and dynamic environments with moving target. This section discusses the algorithm for planning the path of a mobile robot. It relies on hybrid swarm optimization which consist of PSO and BA algorithms combined with local search, and obstacles detection and avoidance techniques. 4.1. Hybrid PSO BA algorithm proposed A hybridised optimisation algorithm is formed by combining the best characteristics of two or more optimisation algorithms to improve the overall performance. Given the limited exploration capability of BA,
Each bat is associated with a velocity ��(��) and a location ����(��) at iteration t, in a D dimensional search or solution space. Among all the bats, there exists a current best solution. Three rules are used to update the positions and velocities. The bat's position is updated in the following manner:
3.2.1. Artificial bat movement
Here ϵ is a random number ∈ [−1, 1] and represents the intensity and direction of the random walk. (t), describes the average level of loudness for all bats at step t in an iteration. It is possible to control the step size more effectively by providing a scaling parameter σ. 3.2.2. The loudness and emission of pulses
����(��+1)=����(t)+����(��+1) (10)
���� =�������� +(�������� ��������)∗���� (11)
����(�� + 1)= ����(��)+����(��+1) (13)
3.2. Bat algorithm (BA) Yang and Gandomi developed a bio inspired algorithm in 2010 called BA [29].The micro bat uses echolocation or bio sonar to locate prey. Echolocation is a major aspect of bat behaviour: bats use sound pulses to detect obstacles as they fly. By using the difference in time between their ears, they detect obstacles. Loudness of the response, and delay time, a bat can determine the speed, size, and shape of prey and obstacles. A bat can also change its sonar in this way by sending high frequency sound pulses. This allows the bat to gather detailed information about its surroundings in a shorter period of time [30]
where β is a uniformly distributed random vector ∈ [0, 1] and �� ∗ represents the current global best location (solution), which is determined by comparing all solutions among all the number of bats. In each bat, after a solution for LS stage is chosen, a new solution is generated using the random walk principle, �������� =�������� +σϵA(t) (14)
ISSN: 2252 8938 Int J Artif Intell, Vol. 11, No. 3, September 2022: 1184 1196
1190convergence of the obtained solutions to the global optimum point is quite impossibl [30]. BA contains the bat specific factors A and r that have an effect on all dimensions of solutions. In this study, a hybridisation of PSO and BA is proposed to optimise the two parameters of the latter and achieve a balance between exploration and exploitation. The proper control of these parameters is based on two variables: alpha (α)and gamma ( γ ), and thus its optimal value enhances the variation of loudness ���� and pulse rate ����. Thus, Exploration and exploitation can be balanced with BA. The PSO algorithm adapts the appropriate range (α, γ )., Algorithm 1 illustrates the pseudo code for Hybrid PSO BA while Figure 5 illustrates the overall procedure. The particle size of PSO repressed in two dimensional vector denoted as x (α, γ).
Algorithm 1: A pseudo code for the hybrid PSO BA algorithm. 1 Initialise PSO and BA parameters: PSO_ parameters: population size of particles NP, r1, r2, c1, c2 BA _parameters: population size of bats NB, frequency (����), pulse rate(����) and loudness (����) 2 Generate random solutions of PSO s1= [α1, γ1], s1 = [α2, γ2 ],…., sn = [αn, γn ] 3 For i =1 to NP 4 Use BA algorithm from Equations (11) (16) 5 Pick the bat with the best fitness according to Equation (6) ������ ��ℎ������ ��=1:���� 6 Store index (k) of the best bat 7 Gbest= ����,= [ αk, γk]. 8 If the stopping criteria is not satisfied, then 9 Update position and velocity using Equations (9) (10) and go to step 3 10 Otherwise Calculate the result output 11 End if Figure 5. Algorithm proposed for hybrid PSO BA optimisation 4.2. The proposed LS technique In every iteration, new positions for the robot are obtained using PSO BA. However, the position can be inside obstacle (case 1) or the path from the previous to the current position can intersect the obstacle (case 2). Figure 6 shows this scenario, where the local search (LS) algorithm is used to convert the infeasible generated solution in to feasible solution. If the algorithm produces the next point in one of two ways, the solution is considered infeasible: In the first case, the points are inside an obstacle A segment of a line passes through an obstacle. 4.2.1. Candidate feasibility checking Two cases consider the candidate solution is infeasible. The first case is when the candidate is inside obstacle and the second is when the line segment between the current and candidate positions intersects the obstacle. However, the two cases have a common feature: grid points are shown near the line segment between current and the candidate positions. Figure 6 shows the two situations of infeasible solution, where p is the current position, and p1 and p2 are the candidate positions, the neighbouring area is shown by the red outlines, and can thus be considered as one situation that can be explained by: ������ ≤���� ≤��������
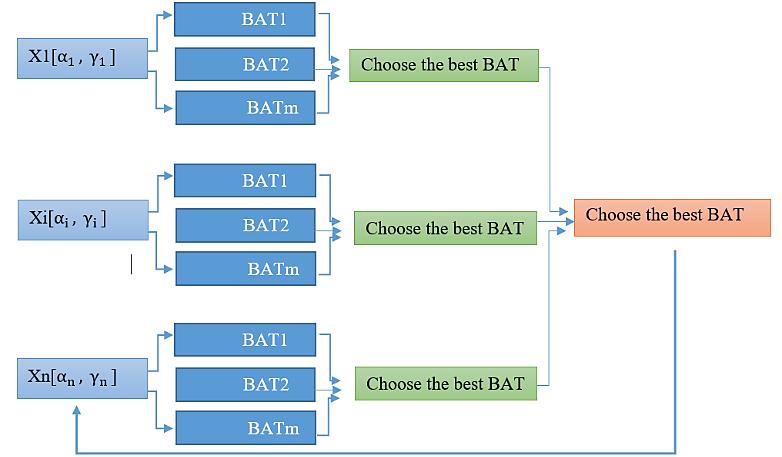
Int J Artif Intell ISSN: 2252 8938 Multi objective optimization path planning with moving target (Baraa M. Abed) 1191 ������ ≤���� ≤�������� ���� ≤��
Figure 6. Infeasible candidate solution Figure 7. Modifying infeasible positions
Thus, the above condition is used for checking the candidate feasibility. Where ���� and ���� represent the grid point of the obstacle, ������and������ are the x, y coordinates of the Wp, ���������������������� is for ��������,s is the scale of grid, dy computed in (17) ���� =|���� (�� ����+��)| (17) where a and b are the slope and offset of the line from Wp and Wpi. In other words, the line that passes both Wp and Wpi can be defined as (18).
The LS technique is used to convert these infeasible solutions to feasible ones. This section illustrates the proposed solutions of the two previous types of situations using graphical and mathematical representations. Figure 7 shows that Wp1 is inside the obstacle and Wp2 is outside the obstacle but the line segment passes between the current position and the destination. First, find the nearest grid point from Wp in the neighbour area of the line segment. In other words, in the Figure 7, Wq1 is the nearest point in the neighbouring area of the line segment between Wp and Wp1 for the first case, and Wq2 for the second case. ������ = min ��∈��(����,������)|���� ��| (21) ��(����,������) is the neighbouring area and |���� ��| is the distance between Wp and O, �� =1,2. Second, calculate the new feasible candidate from the infeasible one. ������ =����+(������ ����) |���� ������| ���� |���� ������| (22) where ds is the safety distance. In other words, the distance between Wqi and ������
�� =�� ��+�� (18) when O is on the line, then ���� =��∙����+�� (19) However, O may not be on the line but rather on the neighbouring areas. Thus, ���� ≈��∙����+�� The size of the neighbour is the grid scale s. ���� =|���� (��∙����+��)|≤�� (20) 4.2.2. Modify the infeasible candidate
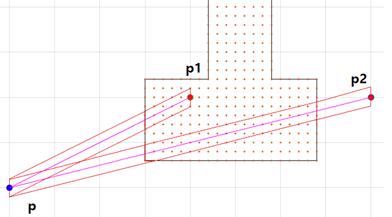
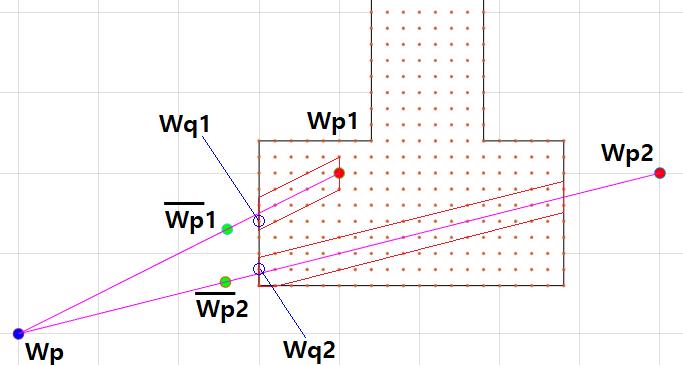
ISSN: 2252 8938 Int J Artif Intell, Vol. 11, No. 3, September 2022: 1184 1196 1192
. In the Figure 8, the corresponding gap vector is Vg = [0 0 1 1 1 1 1 1 1 0 0 0].
The robot follows a path generated by PSO BA algorithm between the starting point and a goal point until it detects an obstacle. The starting point is denoted by (SP) and the goal point is denoted by (GP). In this case, the robot uses ODA procedure to detect and avoiding the obstacle. The obstacle avoiding (OA) based on wall following procedure.
Figure 8. Obstacle detection
�� = min ��∈����������������|���� ��| (23) where O is a grid point of the obstacle. If �� ≤����, then the obstacle is detected, where ���� is the sensing radius. For the grid points inside the SR, calculate the angle of the line segment between Wp and the considered grid point O. �� =��������(���� ������ ���� ������) (24) Subsequently, which sensor point O is in the SR can be determined using angle ��.Thus, the sensing vector can be calculated. The sensing vector consists of bits, which is the sensor number. That is, each bit corresponds to each sensor. When the i th sensor detects the grid point, the i th bit is 1, and otherwise 0. ����(��)= {1, ������������������������������ℎ�������������������� 0, ��������
5. OBSTACLE DETECTION AND AVOIDANCE (ODA)
In the Figure 8, the sensing vector is Vs = [1 1 0 0 0 0 0 0 0 0 1 1]. In other words, the obstacle is sensed by sensors 1, 2, 11 and 12. Next, the gap vector, which indicates the direction the robot can pass through, is calculated from the sensing vector as ����(��)=������(����(��)��������(��+1))
5.1. Obstacle detection
When planning the path of a mobile robot, the first and most important aspect is to detect obstacles and avoid them. Sensors enable the robot to detect obstacles, and so it is necessary that the robot uses this sense to determine which path to follow. By deploying sensors around a robot, obstacles can be detected. All of them have the same Sensor Range (SR) and Angle. The SR represents the maximum distance that the mobile robot can measure, and the angle of the sensor is calculated by (360°/no of sensors). As obstacles are detected using sensor vectors (VS), which are binary vectors containing information about obstacles, their dimensions depend on the number of sensors (in this case, 12 sensors are used), therefore, the sensor vectors are represented by 12 bits indexed by ��∈ {1,2, … ,12}. The value of VS is [s(1), s(2), ..., a(12)]. The value of s(i) is either 1 which indicates that an obstacle is inside the sensing range, or 0 which indicates that the angle range is free as shown in Figure 8.
The distance between the current position Wp and the obstacle is (23).
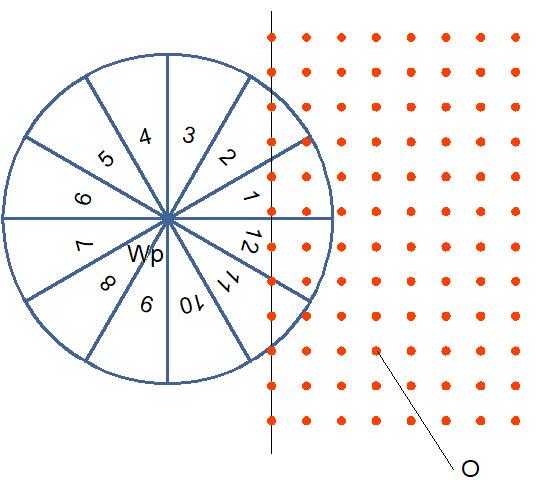
5.2.
Figure 9. Wall following algorithm Figure 10. Room boundary
In order to complete the path to reach the goal avoidance procedure is performed once the robot detects an obstacle. Sensors enable the robot to detect obstacles, and so it is necessary that the robot uses this sense to determine which path to follow. The avoidance procedure is performed using the wall following algorithm. The mechanism of this algorithm explains as following: Move to left or right direction along the ‘wall’ of the obstacle. If the obstacle is detected, the robot moves along the obstacle in the left or right direction. Figure 9 shows the left and right directions for following the wall. Detect the wall of the room and change direction While moving, the robot can detect the boundary of the room using the following conditions: Where Wpx and Wpy are the coordinates of the current robot position. Figure 10 shows the room boundary. The range of the room is xmin, xmax in the x axis and ymin, ymax in the y axis. Once the room wall is detected, the robot must change direction. This procedure details in Figure 11.
When no obstacle is detected, the robot can move towards the target. Figure 12 show moving the robot towards the target. An exception can occur, as shown in Figure 13. In this case, no obstacle is detected in the direction towards the target but the robot cannot actually pass through the path. This case can be avoided by memorizing the robot path during wall following. In the Figure 9, the orange points are those where the robot
Obstacle avoidance
Int J Artif Intell ISSN: 2252 8938 Multi objective optimization path planning with moving target (Baraa M. Abed) 1193
Figure 11. Changing movement direction Moving towards the target
|������ ��������|≤���� |������ ��������|≤���� |������ ��������|≤���� |������ ��������|≤����
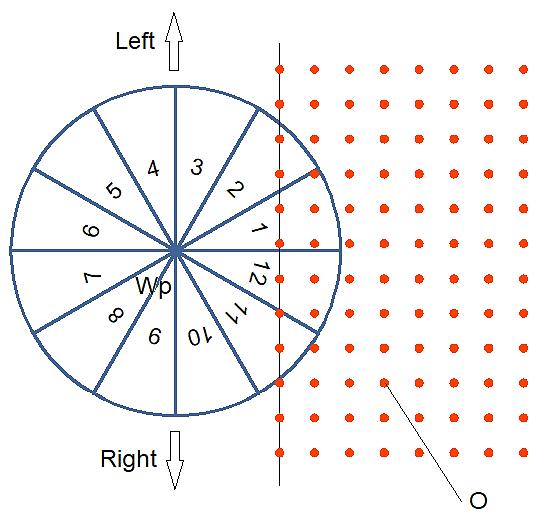
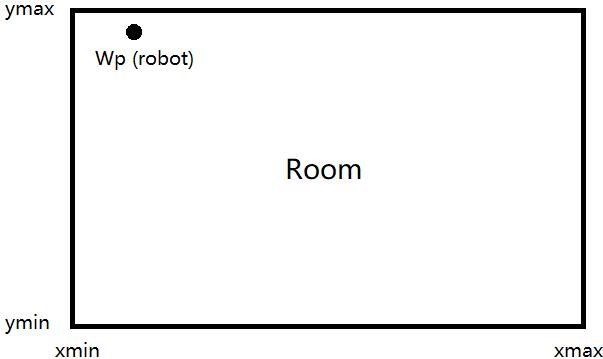
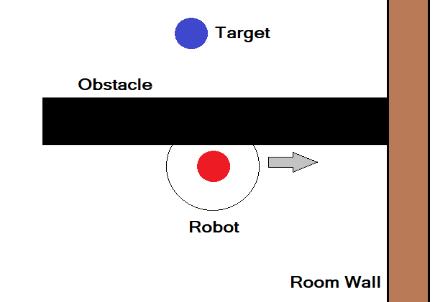
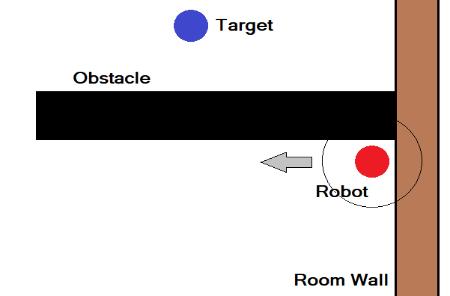
Figure 14. Robot moving directly to the target
This study proposed a path planning algorithm for mobile robots using a hybrid PSO BA optimization algorithm. PSO BA is integrated with the LS algorithm and ODA algorithm. LS is used to covert all infeasible points into feasible points, while the ODA algorithm is used to detect and avoid obstacles. The performance of the algorithm is tested in dynamic environments with moving targets in different scenarios. Using simulation results, the proposed algorithm demonstrates its effectiveness in avoiding both static and dynamic obstacles.
The purple point represents the target point for Robot 1, which is (20, 60). The second robot (Robot2) represented by red point at the location (160, 5). The first robot (Robot1) attempts to reach its target, while the second robot (Robot2) reaches the meeting point with the first robot (Robot1). Several dynamic obstacles with different shapes are moving linearly in this environment, and six static obstacles have different forms and dimensions. The colour of dynamic obstacles is red while the colour of static obstacles is black.
7. CONCLUSION
The algorithm executed with MATLAB R2020a programming language, the following results are obtained as a sample of difference scenario of experiences. MATLAB code is run on a computer with a 2.80 GHz Core i7 processor and 16 GB of RAM. Figure 15(a) (f) shows the simulation results shown in the appendix. A blue point represents the first mobile robot (Robot1) at the starting point SPR1 = (120, 120).
As shown in Figures 15(b) to (e) the first robot moving towards its fixed target, while the second robot attempting to move towards the first robot (moving target) while all dynamic obstacles also move in a linear manner. Figure 15 (f) shows the 88th (final) iteration, where the second robot reaches the target without collision with any obstacles in the environment.
Therefore, when passing the points in the direction towards the target, the robot continues to follow the obstacle wall. Otherwise, the robot moves directly towards the target as shown in Figure 14.
Figure 12. Moving towards the target Figure 13. Exception case
6. SIMULATION RESULTS
ISSN: 2252 8938 Int J Artif Intell, Vol. 11, No. 3, September 2022: 1184 1196 1194passed.
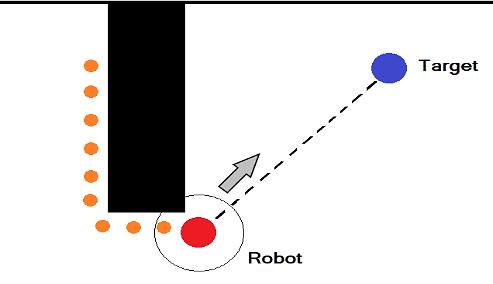
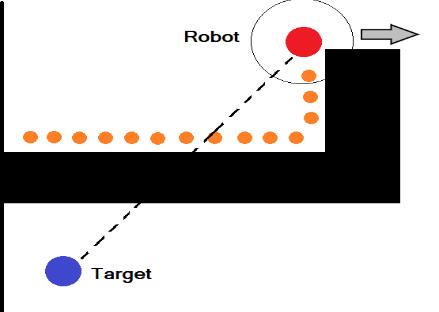
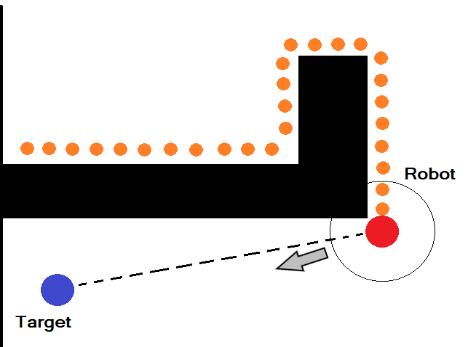
Figure 15. Path planning history: (a) first iteration, (b) Iteration no. (51), (c) Iteration no. (56), (d) Iteration no. (72), (e) Iteration no. (82), (f) Iteration no. (88)
Int J Artif Intell ISSN: 2252 8938 Multi objective optimization path planning with moving target (Baraa M. Abed) 1195 8. APPENDIX
[8] W. Wang, M. Cao, S. Ma, C. Ren, X. Zhu, and H. Lu, “Multi robot odor source search based on Cuckoo search algorithm in ventilated indoor environment,” Proc. World Congr. Intell. Control Autom., vol. 2016 Septe, pp. 1496 1501, 2016,
(a) (b) (c) (d) (e) (f)
[6] M. A. Hossain and I. Ferdous, “Autonomous robot path planning in dynamic environment using a new optimization technique inspired by bacterial foraging technique,” Rob. Auton. Syst., vol. 64, pp. 137 141, 2015, doi: 10.1016/j.robot.2014.07.002.
[7] G. Wang, L. Guo, H. Duan, L. Liu, and H. Wang, “A bat algorithm with mutation for UCAV path planning,” Sci. World J., vol. 2012, 2012, doi: 10.1100/2012/418946.
REFERENCES
[4] S. Hosseininejad and C. Dadkhah, “Mobile robot path planning in dynamic environment based on cuckoo optimization algorithm,” Int. J. Adv. Robot. Syst., vol. 16, no. 2, pp. 1 13, 2019, doi: 10.1177/1729881419839575.
[1] W. Jasim and D. Gu, “H∞ path tracking control for quadrotors based on quaternion representation,” Lect. Notes Comput. Sci. (including Subser. Lect. Notes Artif. Intell. Lect. Notes Bioinformatics), vol. 8717 LNAI, pp. 72 84, 2014, doi: 10.1007/978 3 319 10401 0_7. [2] L. B. Amar and W. M. Jasim, “Hybrid metaheuristic approach for robot path planning in dynamic environment,” Bull. Electr. Eng. Informatics, vol. 10, no. 4, pp. 2152 2162, 2021, doi: 10.11591/EEI.V10I4.2836. [3] H. Cheon and B. K. Kim, “Online Bidirectional Trajectory Planning for Mobile Robots in State Time Space,” IEEE Trans. Ind. Electron., vol. 66, no. 6, pp. 4555 4565, 2019, doi: 10.1109/TIE.2018.2866039.
[5] T. T. Mac, C. Copot, D. T. Tran, and R. De Keyser, “A hierarchical global path planning approach for mobile robots based on multi objective particle swarm optimization,” Appl. Soft Comput. J., vol. 59, pp. 68 76, 2017, doi: 10.1016/j.asoc.2017.05.012.
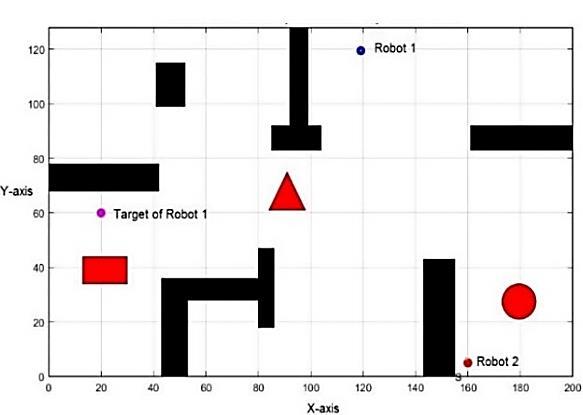
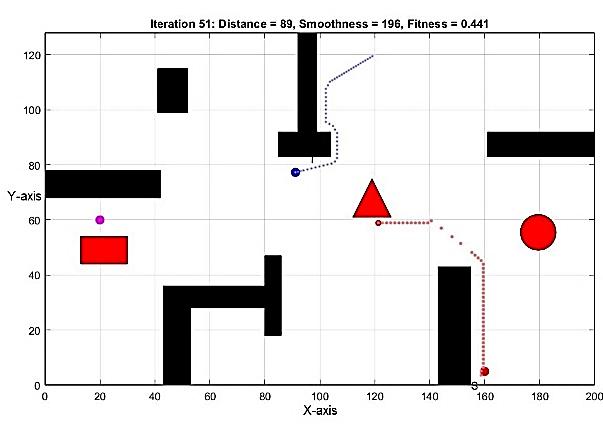
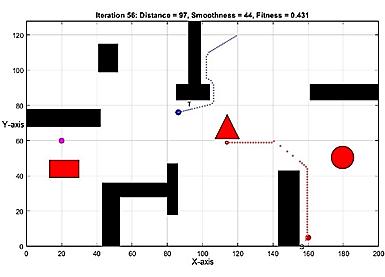
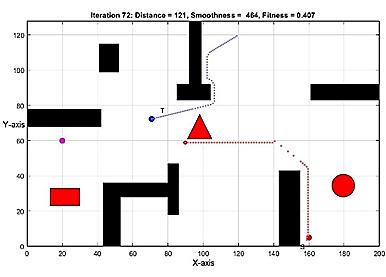
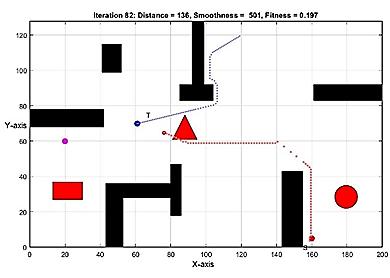
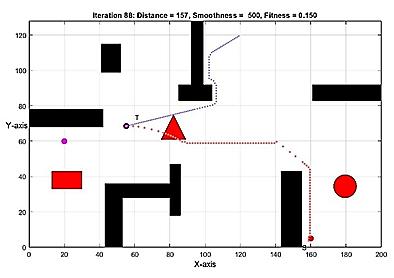
[24] F. H. Ajeil, I. K. Ibraheem, M. A. Sahib, and A. J. Humaidi, “Multi objective path planning of an autonomous mobile robot using hybrid PSO MFB optimization algorithm,” Appl. Soft Comput. J., vol. 89, pp. 1 27, 2020, doi: 10.1016/j.asoc.2020.106076.
ISSN: 2252 8938 Int J Artif Intell, Vol. 11, No. 3, September 2022: 1184 1196 1196 doi: 10.1109/WCICA.2016.7578817.
[14] T. K. Dao, T. S. Pan, and J. S. Pan, “A multi objective optimal mobile robot path planning based on whale optimization algorithm,” Int. Conf. Signal Process. Proceedings, ICSP, vol. 0, pp. 337 342, 2016, doi: 10.1109/ICSP.2016.7877851.
[11] G. Li and W. Chou, “Path planning for mobile robot using self adaptive learning particle swarm optimization,” vol. 61, no. May, pp. 1 18, 2018, doi: 10.1007/s11432 016 9115 2.
BIOGRAPHIES OF AUTHORS Baraa M. Abed Abed is a Ph.D. Student in the field of Robotics and Machine learning. He has received B.Sc. and M.Sc. degrees in Computer Science from University of Anbar. His research interests are autonomous systems, robotics, machine learning, classification and optimization technique. He has published research papers at national and international journals, conference proceedings. He can be contacted at email: burasoft@gmail.com. Wesam M. Jasim Jasim received the B.Sc. and M.Sc. degrees in control automation engineering from University of Technology, Baghdad, Iraq, and Ph.D. degree in computing and Electronics from University of Essex, Essex, UK. Currently, he is an Assistent Professor with the College of Computer Science and Information Technology, University of Anbar. His current research interests include robotics, multiagent systems, cooperative control, Robust control, linear and nonlinear control, Deep learning. He has published research papers at national and international journals, conference proceedings as well as chapters of book. He can be contacted at email: co.wesam.jasim@uoanbar.edu.iq
[21] S. Mirjalili, S. Saremi, and S. Mohammad, “Multi objective grey wolf optimizer : A novel algorithm for multi criterion optimization,” vol. 47, pp. 2015 2017, 2016.
[15] A. Hidalgo Paniagua, M. A. Vega Rodríguez, J. Ferruz, and N. Pavón, “MOSFLA MRPP: Multi Objective Shuffled Frog Leaping Algorithm applied to Mobile Robot Path Planning,” Eng. Appl. Artif. Intell., vol. 44, pp. 123 136, 2015, doi: 10.1016/j.engappai.2015.05.011.
[17] S. Salmanpour, H. Monfared, and H. Omranpour, “Solving robot path planning problem by using a new elitist multi objective IWD algorithm based on coefficient of variation,” Soft Comput., vol. 21, no. 11, pp. 3063 3079, 2017, doi: 10.1007/s00500 015 1991 z. [18] Y. Wang, T. Wei, and X. Qu, “Study of multi objective fuzzy optimization for path planning,” Chinese J. Aeronaut., vol. 25, no. 1, pp. 51 56, 2012, doi: 10.1016/S1000 9361(11)60361 0.
[19] Z. Zhu, J. Xiao, J. Q. Li, F. Wang, and Q. Zhang, “Global path planning of wheeled robots using multi objective memetic algorithms,” Integr. Comput. Aided. Eng., vol. 22, no. 4, pp. 387 404, 2015, doi: 10.3233/ICA 150498.
[10] P. K. Das, S. K. Pradhan, S. N. Patro, and B. K. Balabantaray, “Artificial immune system based path planning of mobile robot,” Stud. Comput. Intell., vol. 395, pp. 195 207,2012, doi: 10.1007/978 3 642 25507 6_17.
[25] F. Gul, W. Rahiman, S. S. N. Alhady, A. Ali, I. Mir, and A. Jalil, “Meta heuristic approach for solving multi objective path planning for autonomous guided robot using PSO GWO optimization algorithm with evolutionary programming,” J. Ambient Intell. Humaniz. Comput., no. 0123456789, 2020, doi: 10.1007/s12652 020 02514 w.
[27] R. Eberhart and J. Kennedy, “New optimizer using particle swarm theory,” Proc. Int. Symp. Micro Mach. Hum. Sci., pp. 39 43, 1995, doi: 10.1109/mhs.1995.494215. [28] A. Koubaa, H. Bennaceur, I. Chaari, S. Trigui, and A. Ammar, Robot Path Planning and Cooperation, vol. XXII, no. 2. 2018. [29] X. S. Yang and A. H. Gandomi, “Bat algorithm: A novel approach for global engineering optimization,” Eng. Comput. (Swansea, Wales), vol. 29, no. 5, pp. 464 483, 2012, doi: 10.1108/02644401211235834.
[30] S. Yilmaz, E. U. Kucuksille, and Y. Cengiz, “Modified bat algorithm,” Elektron. ir Elektrotechnika, vol. 20, no. 2, pp. 71 78, 2014, doi: 10.5755/j01.eee.20.2.4762.
[22] J. H. Zhang, Y. Zhang, and Y. Zhou, “Path planning of mobile robot based on hybrid multi objective bare bones particle swarm optimization with differential evolution,” IEEE Access, vol. 6, pp. 44542 44555, 2018, doi: 10.1109/ACCESS.2018.2864188.
[9] H. S. Dewang, P. K. Mohanty, and S. Kundu, “A Robust Path Planning for Mobile Robot Using Smart Particle Swarm Optimization,” Procedia Comput. Sci., vol. 133, pp. 290 297, 2018, doi: 10.1016/j.procs.2018.07.036.
[13] F. G. Guimari, “Multi Objective Mobile Robot Path Planning Based on,” no. Iccke, pp. 7 12, 2016.
[12] J. Hu and Q. Zhu, “Multi objective mobile robot path planning based on improved genetic algorithm,” 2010 Int. Conf. Intell. Comput. Technol. Autom. ICICTA 2010, vol. 2,pp. 752 756, 2010, doi: 10.1109/ICICTA.2010.300.
[16] A. Hidalgo Paniagua, M. A. Vega Rodríguez, J. Ferruz, and N. Pavón, “Solving the multi objective path planning problem in mobile robotics with a firefly based approach,” Soft Comput., vol. 21, no. 4, pp. 949 964, 2017, doi: 10.1007/s00500 015 1825 z.
[26] B. K. Oleiwi, H. Roth, and B. I. Kazem, “Modified Genetic Algorithm based on A* Algorithm of Multi Objective Optimization for Path Planning,” J. Autom. Control Eng., vol. 2, no. 4, pp. 357 362, 2014, doi: 10.12720/joace.2.4.357 362.
[20] H. Hliwa and B. Atieh, “Multi Objective Path Planning in Static Environment using Region of Sight,” Proc. 2nd 2020 Int. Youth Conf. Radio Electron. Electr. Power Eng. REEPE 2020, pp. 1 5, 2020, doi: 10.1109/REEPE49198.2020.9059199.
[23] S. Geetha, G. M. Chitra, and V. Jayalakshmi, “Multi objective mobile robot path planning based on hybrid algorithm,” ICECT 2011 2011 3rd Int. Conf. Electron. Comput. Technol., vol. 6, pp. 251 255, 2011, doi: 10.1109/ICECTECH.2011.5942092.
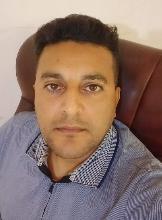



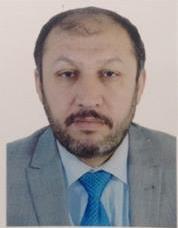


