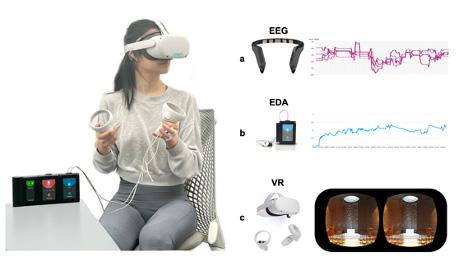
4 minute read
Analyzing Affective Responses to Virtual Spaces Using Physiological Sensors and Verbal Descriptions
Han Tu
SMArchS Computation and EECS
Advisor: Randall Davis & Takehiko Nagakura
Reader: Sarah Williams
During the past two years, the COVID-19 pandemic introduced the pulse oximeter as a new must-have household product for survival in many cities. This small, non-invasive device clips onto the finger and measures the oxygen saturation level of blood, and often, heart rate. Likewise, recent developments in healthcare technology have brought similarly affordable wearable sensors for monitoring the state of our body, including electroencephalogram (EEG) and electrodermal activity (EDA). This paper discusses whether, and if so, how these wearable sensors are useful for design research, especially within the context of AI applications, in terms of analyzing and generating human-centered spatial designs.
Design science using AI for architecture has made advances in various generative algorithms and optimization techniques over the past decade. Numerous architectural studies predating the age of computers, as well as emerging psychological research, take advantage of contemporary sensors. However, it is challenging to provide a data-driven evaluation of humans’ senses in generated spaces, as well as their feelings when occupying them.
How do people actually feel in a particular architectural environment, and what features of its design cause these feelings? Such a question is important and timely because architects select a design that appeals to the human senses, regardless of whether the feelings are generated synthetically. Eminent architects have always imagined and chosen wisely, unlike machines, which still cannot. This paper surveys relevant research practices with wearable sensors in cognitive psychology and affecting computing about architecture and urban design. It demonstrates a pipeline that uses selected measurement devices with a virtual reality (VR) headset to identify patterns among physiological metrics and spatial design through machine learning. First, we created VR environments for four experiments where all the participants follow the same route of spatial experiences. The VR environments includes rendered abstract spatial design of geometrics, combinations of these abstract geometrics to simulate real-world spatial sequences, and 3D scanned real world spaces. These different environments are designed to explore step by step the effects of space shape, spatial sequence, spatial material and spatial sound on subjects’ physiological data. Second, we recorded the physiological data, including EEG, EDA, and heart rate, and interviewed the participants to obtain their responses and feelings to the VR environments (Figure 1). Third, we visualized the physiological and spatial data to align the physiological pattern to the participants’ visual target of the spaces in VR environments. Finally, we proposed a potential application by which a machine could not only generate but also evaluate the generated design spaces as humans do.
Image (opposite): created and drawn by Author, credit to Takehiko Nagakura, Guzden Varinlioglu, and Han Tu.
PLACEIFY:
A data-driven framework for evaluation-by-analogy in early-stage urban analysis and design
Rohit Priyadarshi Sanatani
SMArchS Computation and SM EECS
Advisor: Takehiko Nagakura
Readers: Randall Davis & Sarah Williams
Within the field of urban design and planning, the explicit parameterization of many aspects of urban environments becomes a challenge. Specifically, the parameterization of the intangible experiential and affective qualities of such environments becomes a daunting task, and the quantitative evaluation of such qualities becomes extremely difficult. Owing to these challenges, representation through reference has long remained an essential component in urban design processes. Through case studies of existing environments, designers often attempt to represent, convey and evaluate specific qualities of their own designed outcomes. However, there exist very few frameworks for systematic and data-driven referencing in contemporary urban design workflows.
Building upon advances in urban-informatics and computer vision, this body of research aims to demonstrate a data-driven design referencing and benchmarking framework that will allow urban designers and analysts to systematically reference existing urban environments based upon similarities with their designs along specific high-level urban parameters. A data collection pipeline is created for the extraction of selected visual, morphological, configurational and demographic features from user-generated photographs, street-view imagery, Point of Interest (POI) data, Geographic Information System (GIS) data, and census data. For design referencing and benchmarking, the high-level features of the designed/test environment are extracted and nearest-neighbor algorithms are used to identify relevant samples in the database, based on similarities along specific sets of urban attributes as chosen by the designer. This allows for referencebased scenario building through the modification of specific urban parameters based on imagined environments, and also through direct database queries along such attributes. The research culminates in a web-based tool for location-based design referencing and evaluation. Such a framework has the potential to serve as a valuable decision support system, by providing relevant data at each iteration of the imagination-modification-evaluation cycle.
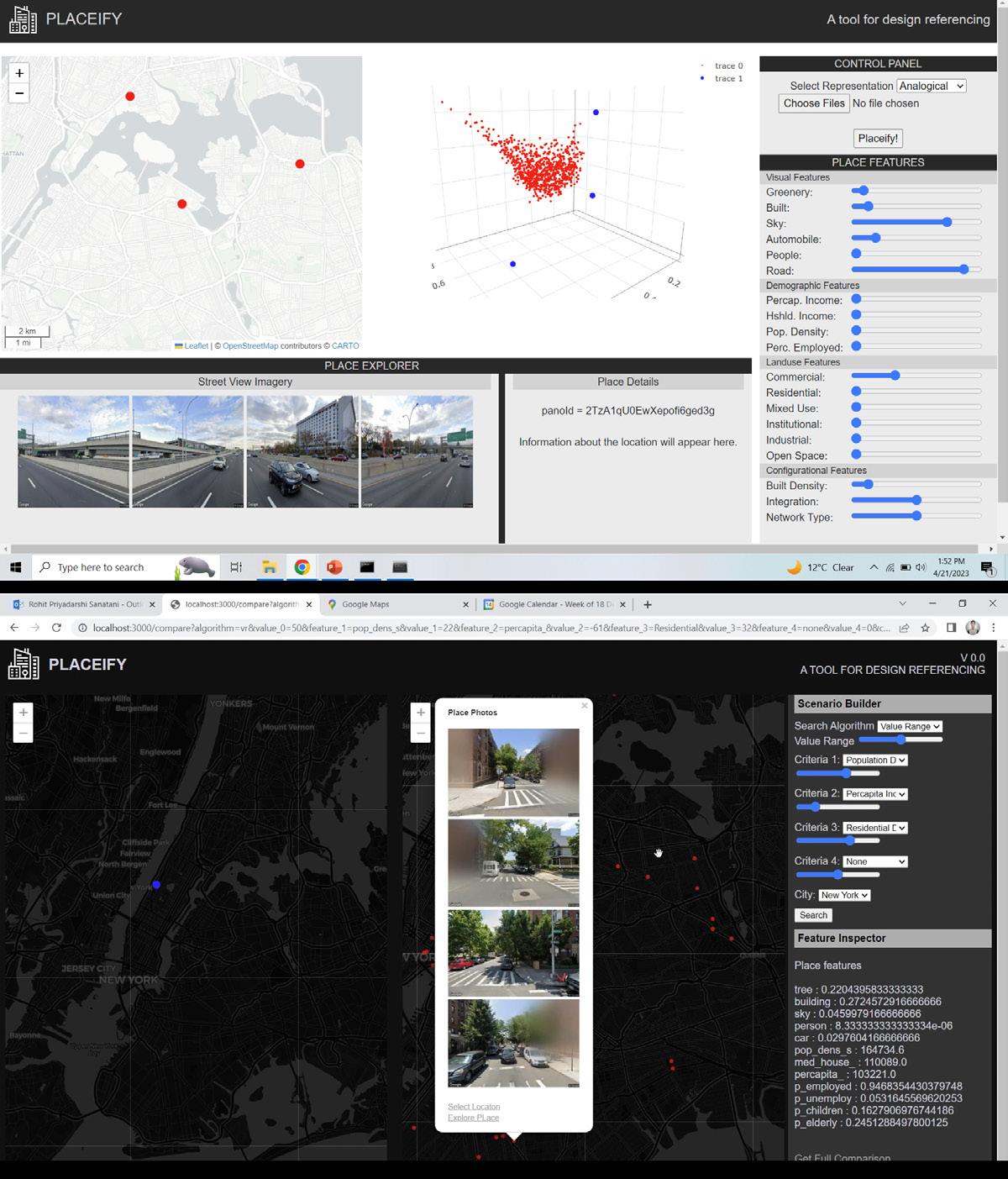
Early Stage Embodied and Operational Analysis for Guiding Sustainable Architectural Design Decisions
Yiwei Lyu
SMArchS Building Technology

Advisors: Randolph E. Kirchain & Leslie K. Norford
Reader: Caitlin T. Mueller
Buildings account for a significant portion of global energy consumption and greenhouse gas emissions. Simulating building performance in the early design stage allows architects and engineers to adjust design decisions to reduce embodied carbon and energy consumption. Life-cycle assessment (LCA) is one of the most comprehensive methodologies to evaluate the environmental impact of architectural production and operation. This thesis aims to address the challenges involved in applying LCA to architectural design in the early design stage. After identifying the gaps in existing research and tools, this project continues to develop a novel workflow in Grasshopper that calculates greenhouse gas (GHG) emissions and costs from both embodied and operational phases. The workflow addresses the early-stage uncertainty through random inputs with a Monte Carlo approach and implements surrogate models to accelerate the process for each iteration. My contribution to the workflow includes improving its robustness and accuracy by redesigning the simulation model to generate more accurate training data and transitioning to a new machine-learning algorithm. With the enhanced workflow and upgraded surrogate models, the embodied and operational GHG emissions of buildings were analyzed to find the common features of low-carbon concrete structures: higher number of floors, lower primary and secondary span lengths, lower primary and secondary span ratios, and low concrete strengths for slabs, beams, and foundations. On the other hand, increasing the volume-tofacade ratios, decreasing the window-to-wall ratios, and incorporating concrete enclosures can help to lower operational emissions in the climate of Los Angeles. The parallel case study shows that although concrete has lower operational emissions in Los Angeles, the offset is not sufficient to match the difference in the embodied carbon of wood construction. Steel, among the three major construction materials, has the highest life cycle emissions with the limitations and assumptions of this research.
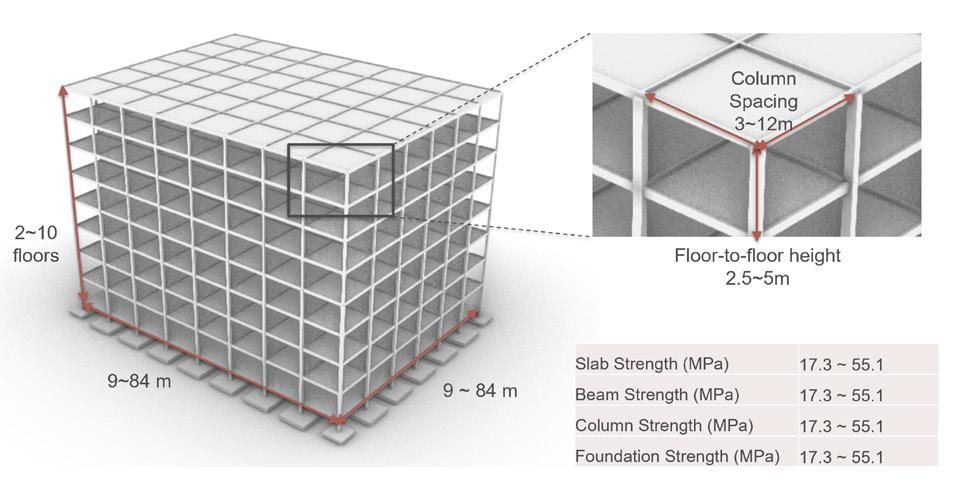