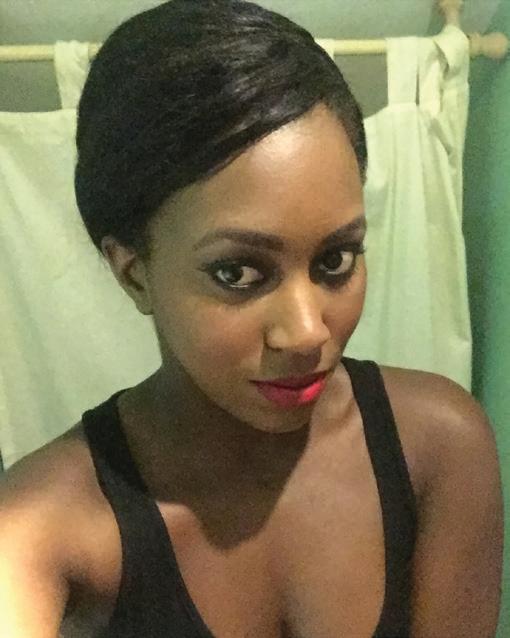
29 minute read
The Rise of the Data Translator Louise Maynard-Atem interviews
THE RISE OF THE DATA TRANSLATOR
Louise Maynard-Atem
6. Validating the model results against real life 7. Developing the story and recommendations based on insights 8. Driving ongoing adoption within the business
They are ultimately responsible for the success of data science and analytics projects.
To deliver on this objective, they must be highly skilled in the softer and non-technical areas like communication, domain expertise, interviewing/questioning, facilitation and project/stakeholder management. There are also the less teachable skills such as entrepreneurial mindset, creativity and adaptability.
They also require working understanding of what quantitative models exist, their applicability and what the output really means.
In this fourth and final part of the Data Series, I wanted to look to the future and try and get a view on what the data landscape is going to look like over the course of this new decade. For that, I posed some questions to my former colleague, Ben Ludford at Efficio Digital, who describes himself as a Data Translator, to understand what this new buzz phrase might mean for the world of data and data science.
WHAT EXACTLY IS A DATA TRANSLATOR? AND WHAT ARE THE KEY SKILLS REQUIRED TO BE ONE? A data translator is someone who can bridge the gap in expertise between technical teams, made up of data scientists, data engineers and software developers, and business stakeholders.
Their primary objective is to unlock the value of investment in technical resource and teams, by maximising the impact of their projects over the entire project lifecycle. This could involve (but isn’t necessarily limited to) the following steps:
1. Engaging the business functions to identify and prioritise projects in which data science could be beneficial 2. Defining the detailed business question/problem 3. Identifying the data that could be used within the project 4. Stakeholder management 5. Ensuring the model output will be understood and useable by users
IF DATA TRANSLATORS HAVE BOTH THE BUSINESS AND TECHNICAL UNDERSTANDING, HOW ARE THEY DIFFERENT TO DATA SCIENTISTS? A typical explanation of what a dat a scientist would say is something like ‘they are an interdisciplinary individual with expertise in statistics, computer science and an understanding of the applicatio ns and domain they are wor king in’ (see Figure 1).
Under that kind of definition, I would say that there are two big differences between them.
1. A translator does not need deep expertise in programming or modelling (but it certainly wouldn’t hurt) 2. Data scientists are often not defined as needing the translator soft skills
Translators do not do the hardcore model development and data handling that data scientists most enjoy. Some would argue that data scientists also have these soft skills. I do not disagree, but I would say that the activities associated with these soft skills are usually in the areas that are not the core focus for data scientists.
Therefore, the two can complement each other nicely in that a translator can take away the work that data scientists do not revel in, to allow them to focus on the things they really enjoy.
To summarise it, I would describe a translator as a soft skills specialist for data science.
FIGURE 1 THE INTERDISCIPLINARY NATURE OF THE MODERN-DAY DATA SCIENTIST
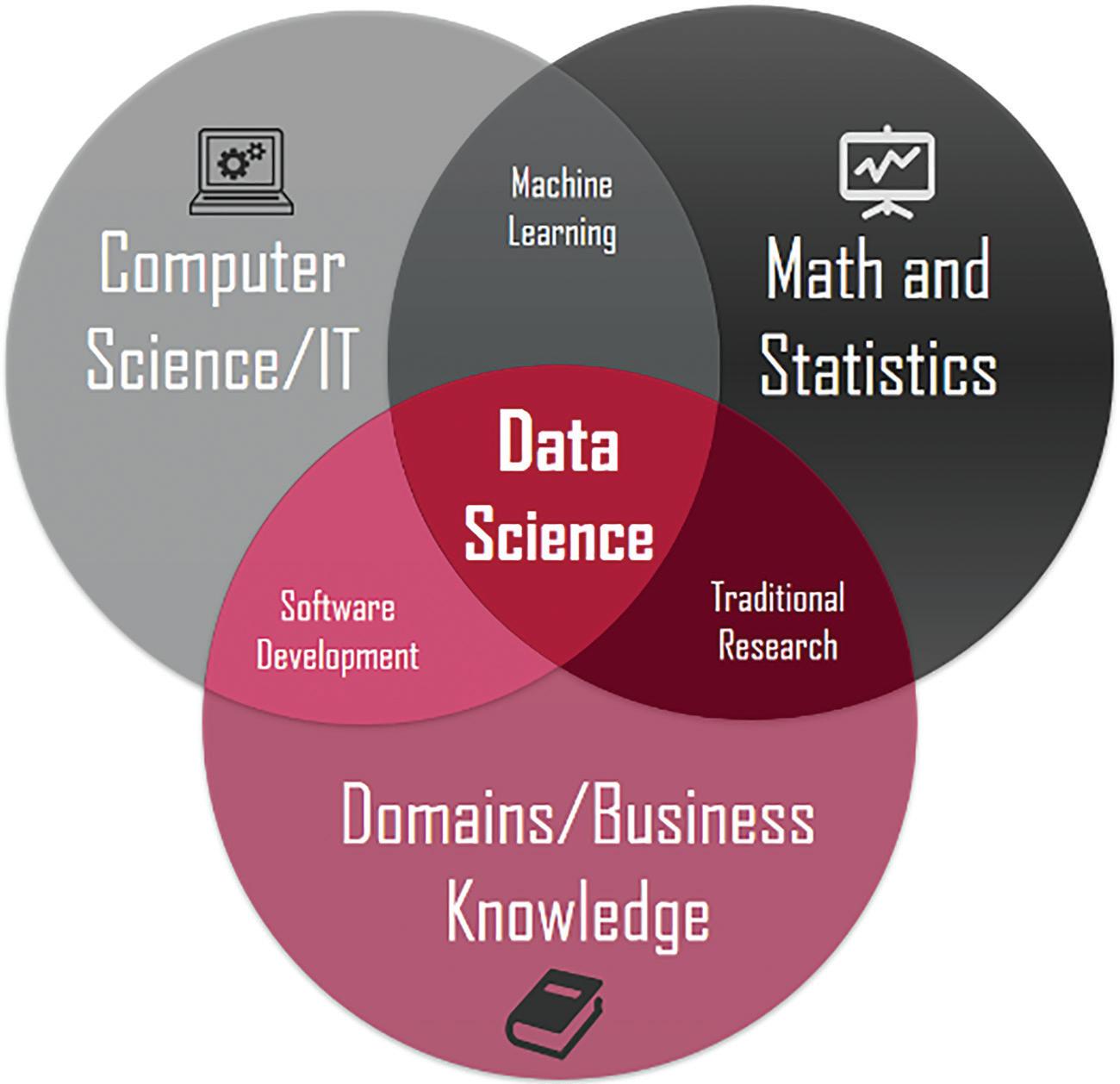
WE’VE ALL HEARD THE STATS ABOUT HOW MANY DATA SCIENCE PROJECTS FAIL. HOW CAN THE DATA TRANSLATOR ROLE IMPACT AND IMPROVE THOSE STATS? When I hear those statistics, it always makes me question what the definition of failure actually is. Data science projects, by their very nature, will be applying cutting edge analytical techniques to untapped and, most likely, previously unexplored data. This could result in the finding that the data does not contain sufficient predictive qualities to address the business question or need. This is not necessarily a failure; it has helped you learn and probably throws up secondary questions.
This is one example of how a translator could help. In a brand-new area, the first objective for a data science project should not be to enable the business to predict an event using a dataset or system. It should be to answer the question, does this dataset contains the right information to attempt to predict an event. The next question will then be whether the event can be predicted. Answering each question will result in other questions forming to answer. And answering a question with no is not, in my opinion, a failure.
That being said, projects do fail. Reasons vary, so to summarise, here are 10 (https://caserta.com/data-blog/ reasons-why-data-projects-fail/):
1. Insufficient, incorrect or conflicting data 2. Failure to understand the real business problem 3. Misapplication of the model 4. Solving a problem no-one cares about 5. Poor communication of results 6. Change is not handled well 7. Unrealistic expectations from stakeholders 8. Poor project management 9. Excessive focus on the model over the problem 10. Lack of empathy
Now aside from number 3, none of these issues are down to a lack of core data science capability. But they are down to a lack of focus on everything else that can make a project a success in the real world. It is the soft skills I have mentioned that are behind this.
So, I absolutely believe that having translators on data science teams will ultimately only have a positive impact but
changing the way projects are delivered and managing closely those areas and interactions that are not invested in enough by existing teams.
Translators plug the gap that exists between data science teams and the rest of the business. They enable data scientists to do their best work, manage the expectations of wider stakeholders and drive better outcomes for the business overall.
WHAT DOES A PERFECT DATA SCIENCE TEAM LOOK LIKE IN YOUR MIND’S EYE? I don’t think there’s a perfect team, as it completely depends on the environment in which they operate. But in any given context, there are two things that will help describe what the optimal data science team looks like.
Firstly, they have a clear definition of what their capability needs to be and what will be filled from other parts of the organisation. For example, if an organisation has a Chief Data Officer who has a team dedicated to unlocking access to data, and has documentation about data sources then you do not need the replicate this capability.
Secondly, the team should attempt to achieve the full spectrum of skills missing elsewhere. The team needs to have the full set of capabilities. However, this is can be made up of a few subsets of different skillsets. topic on everyone’s lips at the moment: ethics. Data science is so open to ethical issues from people not truly thinking through the implications of the models they build and how the output might be used in unintended ways. This needs to be handled properly for every model or the field as a whole risks irreparable reputational damage.
As an opportunity, I think that, while specialist skills will continue to be hard to find, there will be an overall increase in data literacy within the workforce. This closes the communication gap between data science and stakeholders and will only have a positive effect on outcomes.
Data Science is not going anywhere anytime soon. It will continue to grow. Those who work within data science, or are customers of it, need to understand the risks and make sure that what they’re delivering is fit for purpose.
So there we have it, the future (or at least one version of it) is translation. I’m really keen to get your thoughts on this, and I’m sure Ben would too, so please feel free to reach out to either of us directly on LinkedIn.
WHAT ARE THE BIGGEST OPPORTUNITIES AND THREATS THAT YOU THINK THE INDUSTRY WILL FACE OVER THE NEXT DECADE? As a threat, it is lack of skills that always comes to mind. However, that is partly driven by a high expectation on the range of capabilities that those in data should have. For me, I think that as the industry grows, there will be more specialisation in roles and that will help to draw in more people from the edges of the data space, to help meet demand for data science.
Related to this threat is the proliferation of “data scienceas-a-service” type tools. These tools will likely be the biggest contributor in meeting the demand for data science skills. But the thing that concerns me is that I can see a future where anyone can create, build and deploy a model where the underlying assumptions have not been considered and the input data not really tested. And that brings us to the
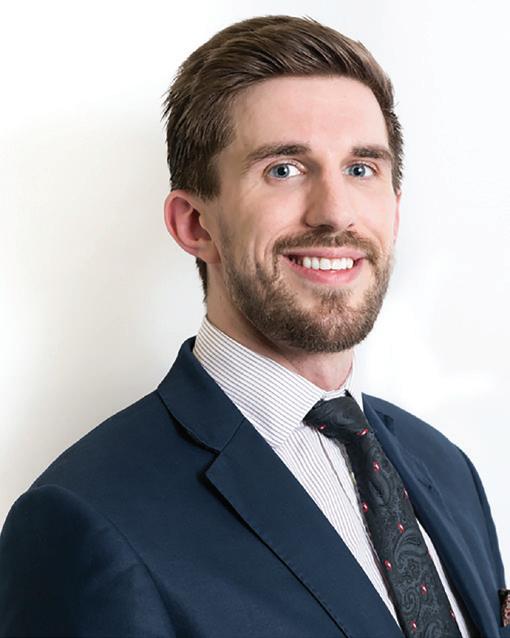
Ben Ludford is a Manager at Efficio Digital, a fast-growing specialist consultancy. He focusses on aligning new products and services in the data space with clients’ needs. His background is Operational Research having studied it as an MSc at Lancaster University Management School and then working in the field for several years.
Louise Maynard-Atem is an innovation specialist in the Data Exchange team at Experian. She is the co-founder of the Corporate Innovation Forum and is an advocate for STEM activities, volunteering with the STEMettes and The Access Project.
A VISION FOR DATA SCIENCE AT BRITISH AIRWAYS
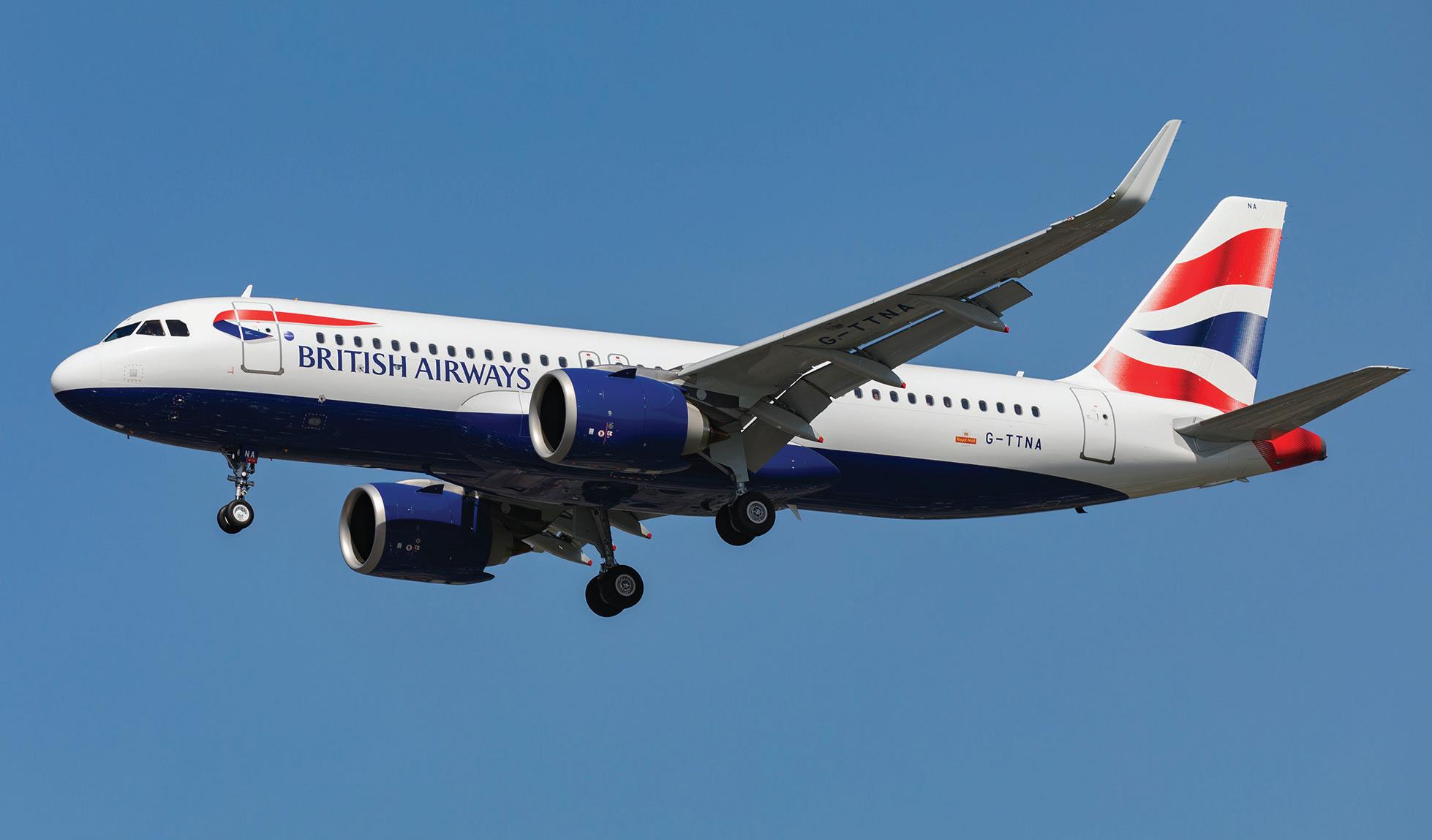
STEFAN JACKSON AND JOHN TOZER
BRITISH AIRWAYS HAS A HUGE AND COMPLEX OPERATION, centred at Heathrow, connecting hundreds of destinations worldwide. Although capacity is constrained at our main hub, we are gradually expanding our network to keep up with demand. We are proud of our punctuality, the main driver of customer satisfaction.
In 2019 we celebrated British Airways’ centenary – 100 years of connecting Britain with the world and the world with Britain – and collected the CAPA award for Airline of the Year, as well as two prestigious Superbrand titles as the top British brand for consumers and business.
But none of this allows us to stand still as a business. The way our customers choose and consume their air travel is changing. They can no longer be stereotyped as ‘business up front, leisure down the back’, as they mix and match premium and economy travel for many different reasons. In this market we must provide quality, choice and value for everyone.
Customers expect more when things go wrong – a quick resolution and compensation.
British Airways is very aware of its environmental responsibilities, having recently committed to a target of Net Zero greenhouse gas emissions by 2050. This multi-faceted problem requires a multi-faceted response that will mean changes to our aircraft fleet, and making more and more choices where sustainability is the overriding consideration. From the start of 2020 we are offsetting all our domestic flights, and customers travelling further afield can choose to offset their individual emissions with our carbon calculator. We are also investing in the development of sustainable aviation fuel with our colleagues at Velocys and Shell, and are the first airline in Europe to build, with our partners, a waste-to-fuels plant.
Our ability to respond to change depends entirely on the decisions made by our colleagues and management in the air, on the ground and at Head Office.
DATA SCIENCE AND ANALYTICS IN BRITISH AIRWAYS In the Central Analytics department, we like to say that the company’s success is equal to the sum of its decisions. For the 70 years since the original Operational Research Team was formed in 1949, we have delivered data and analysis based on that equation; and our department has gone from strength to strength. The current Central Analytics department comprises around 80 data scientists, analysts and performance specialists, whose remit is to drive smarter decisions across British Airways.
The analytics teams see it as their responsibility to adapt new techniques and technologies to the airline’s benefit. Two years ago, we set up our Data Science and Innovation team to pioneer new techniques and Artificial Intelligence. We believe AI will transform the airline industry, and data is key to AI. The team is a core group of data scientists and data engineers who drive innovations, proactively looking for new ways to help our business. We have a mandate and funding to innovate, and we invest in our people and in the tools we use. Part of our work is pure innovation or exploration of data and methods, but an equally important part is finding ways to deploy the new methods within British Airways’ many long-established processes and systems.
We have looked at the opportunities for Data Science in each part of British Airways. We have thought about the key business challenges, but also looked at other companies that successfully apply Data Science to their business process and customer proposition. From the research we have created several themes and prioritised them. At the same time, we have reviewed our analytics architecture of IT and techniques, and identified where we can improve it. We summarise the vision as “Bringing contemporary and edge analytics into the business”.
Bringing a new approach means we are defining the requirements for infrastructure, tools, and working practices that will more easily manage data, that will get models in to testand-learn frameworks, and that will deploy the finished products into operational processes long term. There are some key components to this. First, an appropriate cloud infrastructure to process data at scale, and aid deployment. Second, a DevOps way of working: this is a set of practices that combines software development (Dev) and IT operations (Ops) to shorten the product development life cycle while delivering features, fixes, and updates frequently in close alignment with business objectives. And third, the technology building blocks that will make analysis and analytics reporting slicker. To deliver all this we work in close partnership with British Airways’ Data team, which manages our databases and data tools.
Data Science and AI are bringing, or may soon bring, improvements to many parts of our business. In the following paragraphs we look at some of the areas that we are working in and investigating.
Planning the operation A successful airline operation depends on successful planning. Understanding and forecasting changes to plan is a critical analytics role that supports making the right day-to-day decisions. The decisions in turn lead to actions that minimise disruption and inconvenience to customers, aircraft and crew, and ensure we meet our commercial goals. We want to apply more sophisticated methods to input into decisions, complementing or upgrading the existing models. And before we can exploit the latest techniques we need to test and review
AN ELECTRIC MOTOTOK TUG PUSHES BACK AN AIRCRAFT AT HEATHROW T5
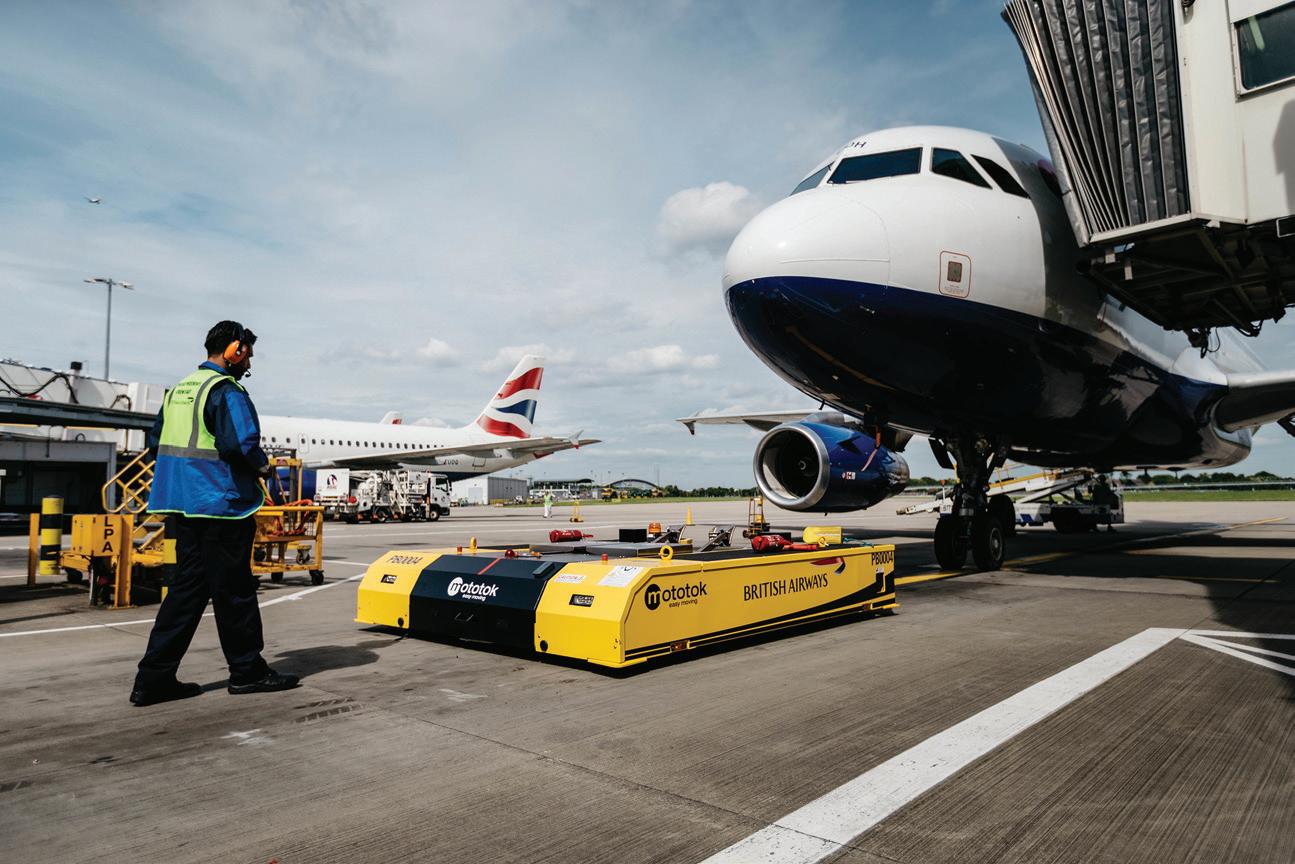
In making decisions on our future aircraft fleet, Data Science, forecasting and optimisation techniques could bring underlying improvements to the existing models, for example, improvements to the prediction of the demand for individual flights and total market demand.
The company is also committed to reducing its carbon footprint through the development of new, greener aviation fuels, and through introducing more electric vehicles in airport operations. A recent Data Science project looked at optimising the charging regime of electric tug batteries using telematics data.
their performance. As well as Data Science methods, we are investing in new computer simulation software to experiment with operational and planning decisions. The aim is always to improve the service we offer our customers and ensure their flights depart on time.
Analytics and forecasting have been fundamental to improving levels of customer satisfaction for our Buy on Board catering. We have designed and improved machine learning algorithms to adjust the loading of fresh food, and integrated these into the automated supply chain systems. We have also introduced anomaly detection, to identify and rectify potential loading errors. We have processes to constantly monitor customer satisfaction and regularly review our product range, including the use of text analytics to analyse customer feedback.
With tens of thousands of flying and ground colleagues, we can encounter complex and dynamic people scheduling issues. There is a need to design and implement shift patterns (rotas) that efficiently match rostered hours to the workload or business demand subject to operational, contractual and social constraints. One particular problem is to decide how many flying crew to have on standby. The requirement changes dependent on a variety of issues – time of year, sickness rates, number of flights, and others. Here we have opportunities to test and learn and deploy analytics, especially forecasting and optimisation techniques.
Flying sustainably British Airways is committed to achieving Net Zero greenhouse gas emissions by 2050, with a number of milestones needed to be reached over the next thirty years. A large percentage of the target will be met by replacing older aircraft with newer, more fuelefficient ones.
Staying safe Safety is always the airline’s top priority and British Airways has a programme of scheduled maintenance for every aircraft, with a 24-hour highly skilled Engineering team to handle any issues. In addition to this programme, the Data Science team has introduced predictive maintenance techniques to help determine the condition of in-service equipment and provide smarter data to predict the optimum window for minor maintenance to be performed. It is known as condition-based maintenance, carried out on the basis of very high-quality estimations of the lifespan of a piece of machinery. This approach to part of our maintenance programme promises increased efficiency over routine or time-based preventive maintenance, because tasks are performed when they are warranted. One approach we have taken is to use readings from airframe sensors, to identify anomalous patterns in the data, either during the flight, or average readings from flight to flight.
In the wider business, risk management is the identification,
evaluation, and prioritisation of risks followed by the right application of resources to minimise, monitor, and control the probability or impact of adverse events. The opportunities for Data Science and AI include using new data to identify risks (Internet of Things, telematics data) and improving the intake and storage of existing data in our data warehouses and data lakes. recommend destinations to customers based on their travel and browsing histories.
Offering the right product at the right price British Airways has a corporate programme to bring a step change in the way we price add-ons to the basic flight product, such as extra baggage or a selected seat. One part of the programme is introducing new mechanisms and systems to price these ancillary products appropriately for our customers. As we better understand our customers’ needs, we are building models to allow seats to be dynamically priced based on the features of the booking and flight. We are also looking to make an ambitious step change in the capability and structure of our flight revenue management system. We aim to develop new models and capability that exploit Machine Learning within any new system developed.
We want to understand and relate to our customers as individuals. One important element of Customer Relationship Management and oneto-one personalisation is creating and using new customer models. These models often make use of external data sources or deploy data science techniques. More specifically, we need to employ new methods like machine learning to refine established models. Two examples are: the algorithm that estimates whether a booking is for business or leisure travel; and the recommender engines that
Listening to customers and colleagues (and talking back) We regularly ask our customers and colleagues for feedback – in a highly competitive environment we recognise this is what makes us better. We are setting up strategies to process, analyse and propose actions based on the raw verbatim feedback we receive from respondents. We use text analytics to automatically generate insights from crew feedback, and to process online customer feedback, for example filtering out personal comments about individuals. We have methods for analysing social media, and for finding themes in feedback from different customer surveys. Another application is tracking
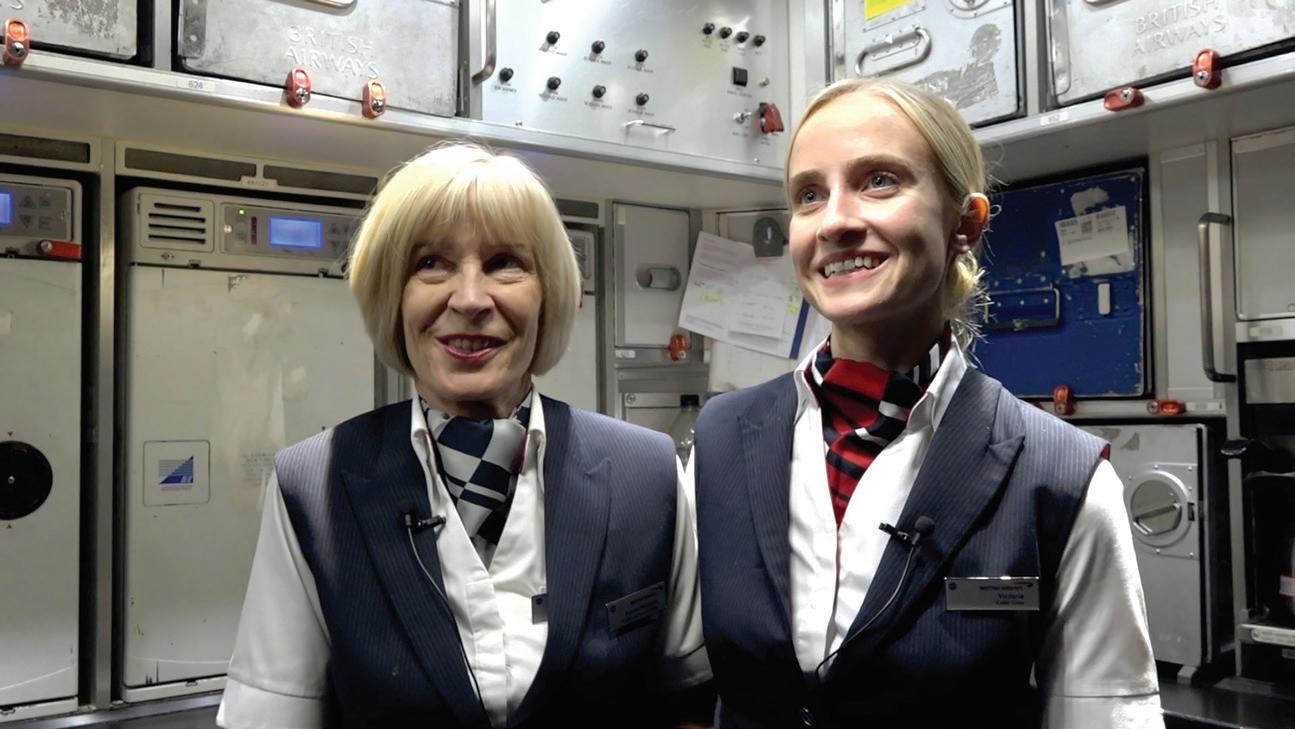
BRITISH AIRWAYS CABIN CREW
colleague sentiment, as a way of monitoring satisfaction. We intend to shift the work away from ad-hoc project work, to automated self-service reporting.
Chatbots, or the ‘conversational virtual assistant platform’, enables customers, businesses and colleagues to self-serve with information and simple transactions, linking in to back-end systems. We are helping define Business to Business, Business to Customer and Business to Employee use cases. The more advanced third-party virtual assistant uses natural language processing and machine learning, so there is an opportunity to collaborate with the vendor in its development. We also recognise that these tools generate new data in large quantities – presenting both opportunities for analysts and challenges for data engineers.
A VISION FOR DATA SCIENCE We said earlier that we view British Airways’ success as the sum of its decisions. When we look at new data analytical methods and new technologies, our focus is to identify where we can most effectively employ
them to improve decisions in an organisation with many established practices and constraints, but one that strives to evolve in a changing business environment. This means that sometimes we need to explore, speculate, innovate, and even fail; at other times we need to adapt our methods to existing systems and processes. The Data Science and Innovation team is open to both approaches. The team’s make-up deliberately combines newly-recruited postgraduates with a grounding in the latest techniques, and established experts with great business, systems and data knowledge.
We believe that a rich and flexible learning programme is key to the success of the department. We offer a range of learning opportunities, from short, in-house knowledge sharing sessions, external training and attendance at conferences, to accreditation, MSc apprenticeship courses, and mentoring.
Data Science and Innovation is a small team with a big remit in a large organisation, and we depend on our relationships across the business – fellow analysts, IT and data professionals, and colleagues across head office and out into the business – to deliver. But we are also developing ways to deploy our products faster and with less dependency on functions such as IT to implement them. We are exploring DevOps (an industry standard methodology for Data Science projects), big data infrastructure, APIs (web interfaces for data) and software containerisation to this end.
We have endorsement of our vision at the highest level of our business. Alex Cruz, British Airways’ Chairman
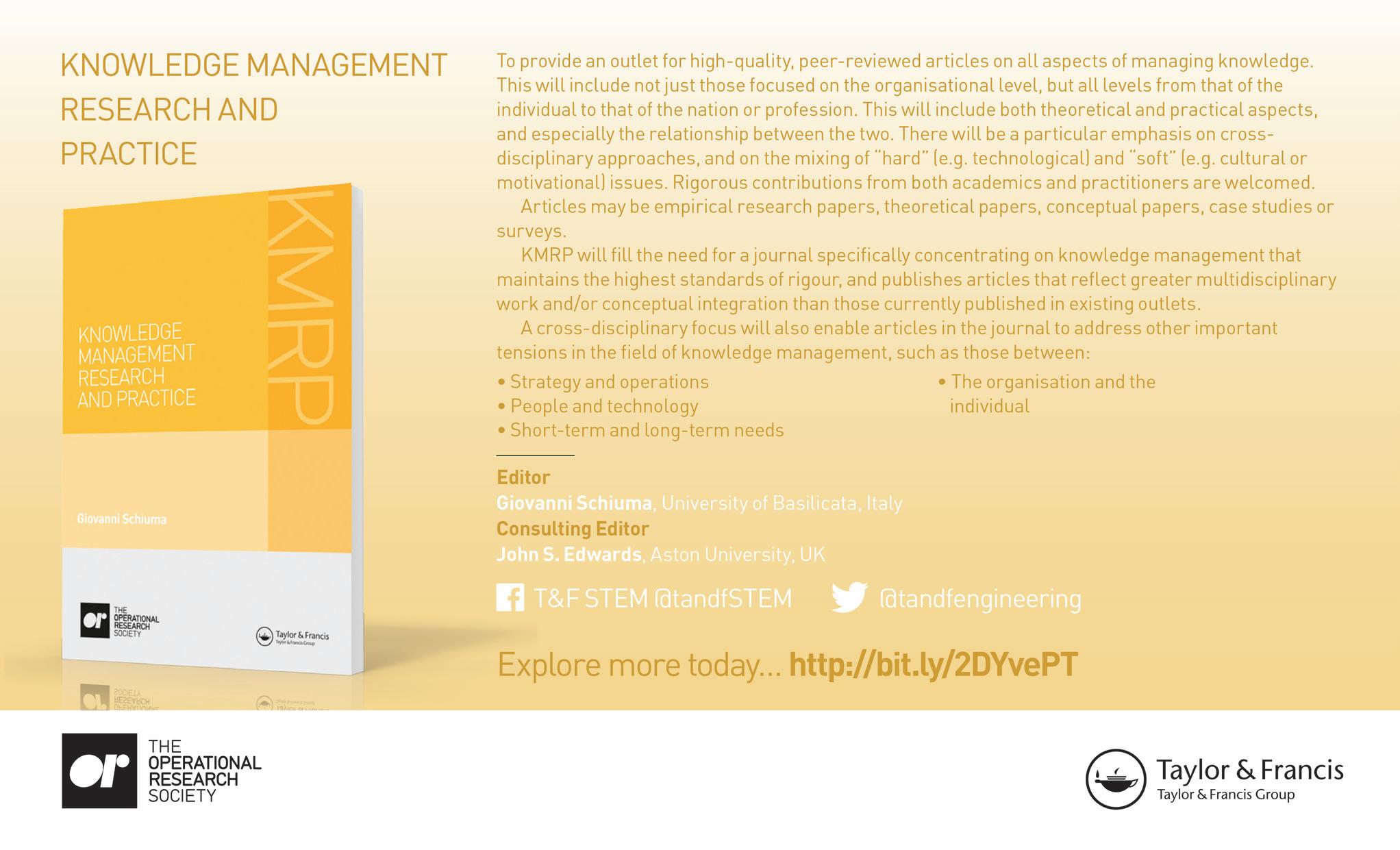
and CEO, talking to the Central Analytics teams recently, described the opportunities for Analytics and Data Science as ‘limitless’. With all this goodwill we still need to produce a constant flow of success stories, and we are adopting agile project methodologies and communications to keep our central place in the business.
Stefan Jackson is British Airways’ Data Science and Innovation Manager, with a background in Operational Research.
John Tozer is a Data Exploitation Specialist with a background in Operational Research and Data Management in British Airways.
This article was written in January 2020.
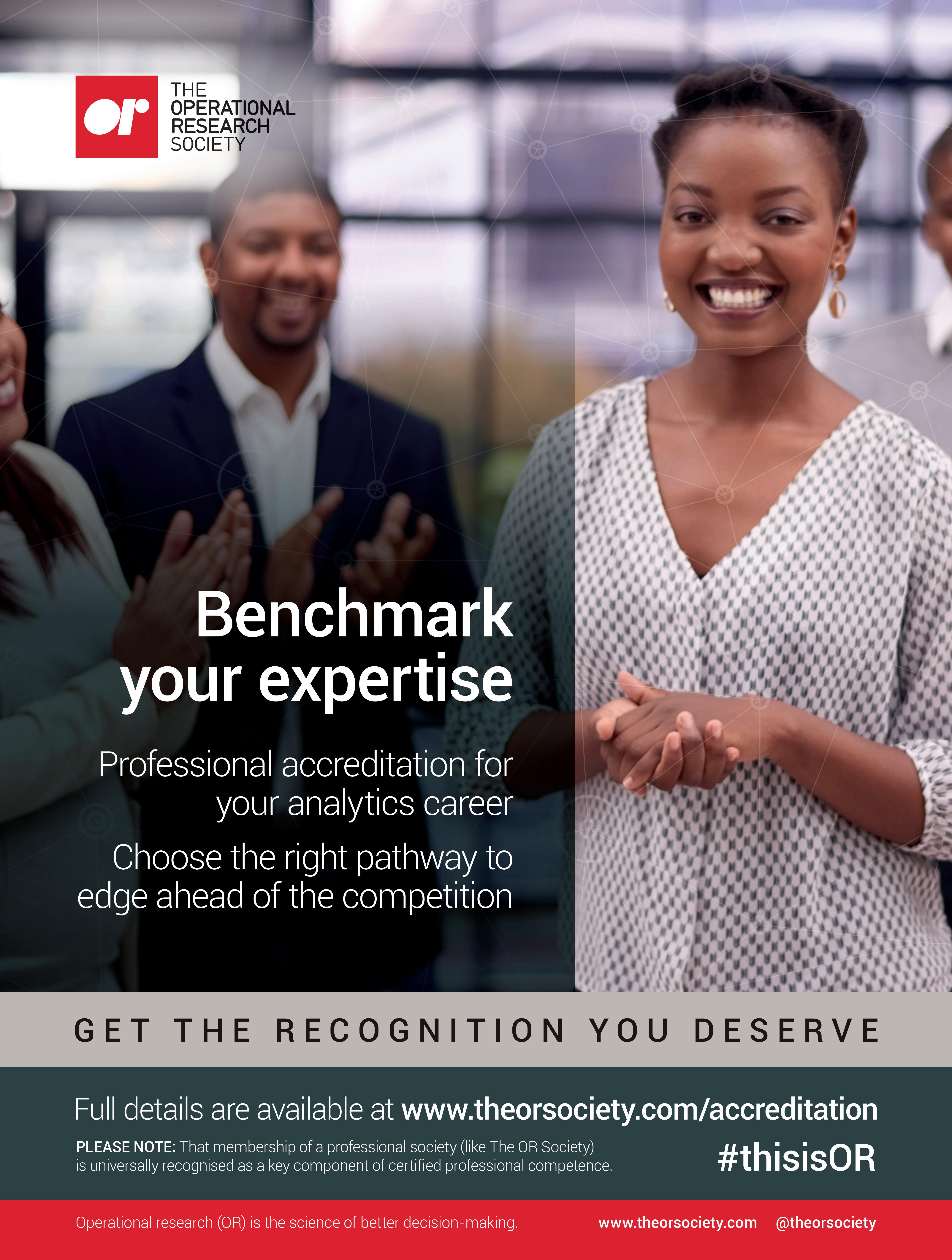
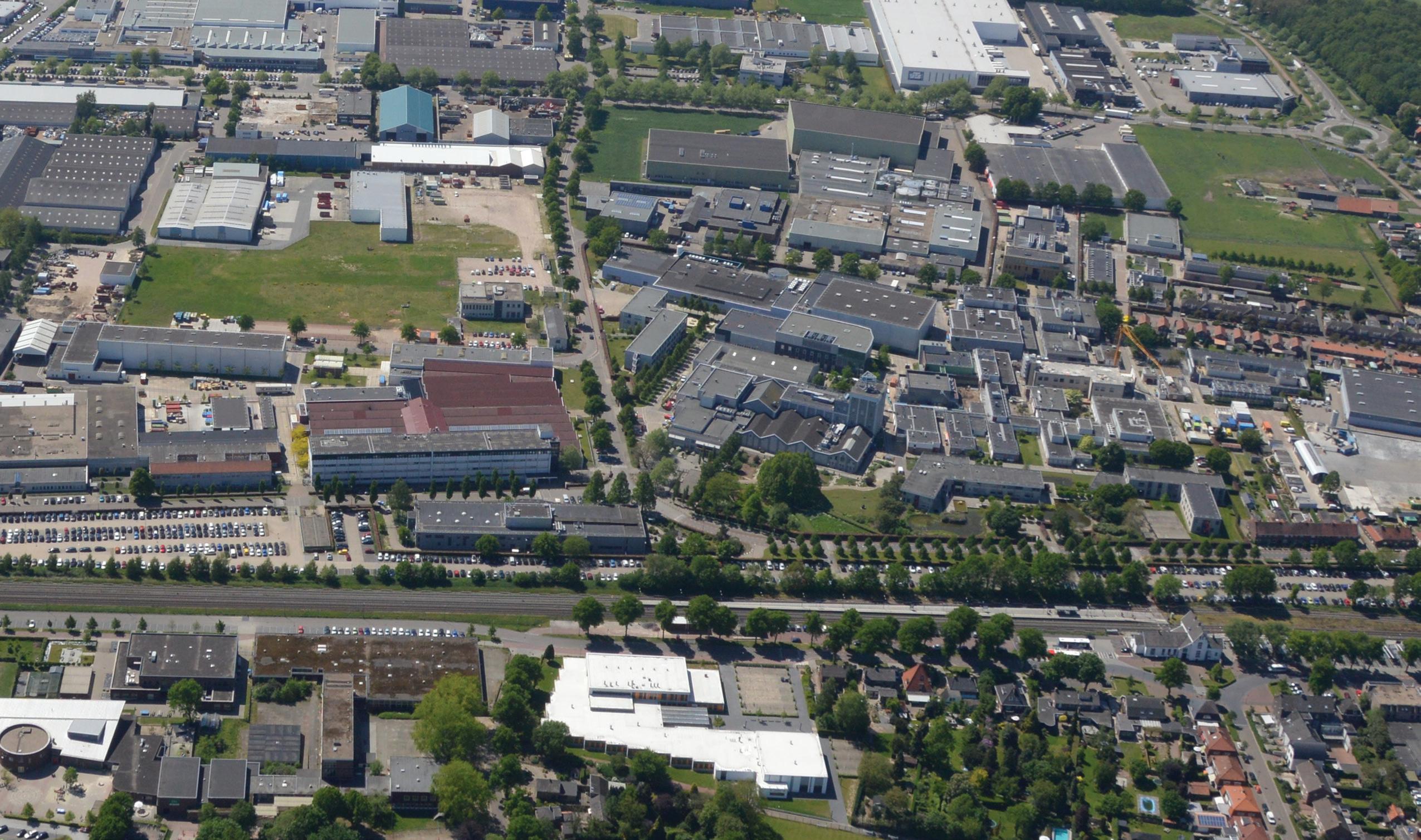
TUGCE MARTAGAN
MILLIONS OF PATIENTS HAVE BENEFITTED FROM NEXT GENERATION DRUGS to recover from cancer, autoimmune disorders, and many other diseases. These drugs are produced using biomanufacturing technologies. The biomanufacturing industry is growing rapidly and becoming one of the key drivers of personalised medicine and life sciences. As such, the global biomanufacturing market is projected to reach $388 billion in 2024.
Despite its success, biomanufacturing is a challenging business. It is cost intensive with high risks of failure. In addition, biomanufacturing methods use live systems (e.g., bacteria, virus, insect cells, etc.) during the production process. This enables highly complex and unique active ingredients to be generated compared with conventional
drugs. However, the use of live systems leads to unique production challenges related to predictability, stability, and batch-to-batch variability.
To date, several industries have benefited from Operational Research (O.R.) methodologies to improve operational efficiency, and reduce costs and lead times. However, the applications of O.R. methodologies to the biomanufacturing industry are still immature. One of the main reasons is that the competitive advantage in biomanufacturing used to be mainly driven by the scientific advances related to the underlying biological and chemical dynamics. However, with the growing market competition, the industry encounters an increasing need for a data-driven, O.R.-based approach to improve business practices.
A multi-disciplinary team of researchers from Eindhoven University of Technology (TU/e) and Merck Sharp & Dohme Animal Health (MSD AH) have been collaborating over three years to improve biomanufacturing efficiency. The team consists of Dr. Tugce Martagan, Prof. Dr. Ivo Adan, and Ph.D. candidate Yesim Koca from TU/e, and Oscar Repping, Bram van Ravenstein and Marc Baaijens from MSD AH. The collaboration resulted in a portfolio of optimisation models and decision support tools to reduce biomanufacturing costs and lead times. The project combines operations research and life sciences. This is one of the first examples showing how operational research can improve biomanufacturing practice.
Overall, the project used techniques such as machine learning and simulation-optimisation to predict and control biological systems. A variety of optimisation models and decision support tools were developed to translate the underlying biological dynamics into business metrics, such as lead times and costs. Although the project was conducted and implemented by MSD AH, its true impact extends to other biomanufacturing companies, including human health applications. This is mainly because the project addresses common industry challenges related to predictability, batch-tobatch variability and planning under uncertainty. In general, the project consists of three parts: reducing bioreactor changeovers, increasing fermentation yield, and creating better production plans.
HOW TO REDUCE CHANGEOVER TIMES IN BIOMANUFACTURING? A typical biomanufacturing process can be broadly classified into two main steps, fermentation and purification. Fermentation is often carried out in bioreactors (e.g., highly controlled stainless steel vessels for a cell culture to grow). After fermentation, the batch proceeds with a series of purification operations (e.g., centrifugation, filtration, chromatography, etc.) to achieve good quality standards. The main focus of the first project is

FULL-SCALE PHARMACEUTICAL FERMENTER LINE USED IN BACTERIOLOGICAL ANTIGEN PRODUCTION
fermentation. During fermentation, the cell culture follows a specific growth pattern: The fermentation starts with the lag phase with no cell growth. The cells start to slowly grow in the acceleration phase, and continue with the exponential growth phase where the cell growth speed reaches its maximum. Then, the cell growth slows down through the deceleration and stationary phase, followed by the death phase. The cells produce the final product (e.g., proteins, antibodies) as they grow. Typically, the batch culture is harvested during the deceleration or stationary phase.
After the batch is harvested, the bioreactor needs to be cleaned and sterilised to be prepared for a new batch. These changeovers are costly and time consuming. For example, it might take one full work day to clean and sterilise a bioreactor. Therefore, there is a significant business case for reducing changeover times in biomanufacturing.
To reduce the bioreactor changeover times, MSD AH developed a new replenishment technique called bleed-feed. With this technique, some predefined fraction of the culture is extracted (to be sent downstream for further processing) and a special fresh medium is added to the remaining culture. The remaining cell culture acts as a new seed for the next bioreactor run. This means that the technique allows the changeover activities for the subsequent batch to be skipped. However, the technique can be performed during the exponential cell growth phase only. Otherwise, the technique does not work, and the culture needs to be harvested in full. In this setting, identifying the best bleed-feed time is challenging because the duration of each cell growth phase is stochastic. This means that we do not know the exact moment when the exponential growth phase stops. If the bleed-feed technique is carried out too early, then we might not achieve the maximum yield from that batch. In contrast, if it is conducted too late, then the batch needs to be harvested and the bioreactor needs to be set up for the next batch.
The proposed configuration has enabled an increase in the bioreactor yield by 50% while reducing the yield variability by 25%
To address this problem, a stochastic optimisation model was developed using the theory of Markov Decision Processes. The objective of the model is to identify the optimal bleed-feed time that maximizes the expected profit. The optimisation model captures the complex dynamics and uncertainties related to the underlying biological dynamics. Then, the model links the underlying biological dynamics with business metrics, such as cost and throughput. Prior to the optimisation model, the bleed-feed decisions were made based on domain knowledge. The optimisation model provided a data-driven, quantitative approach for decision-making, which improved process efficiency.
HOW TO INCREASE FERMENTATION YIELD? In this project, the main objective was to maximise the production yield obtained from fermentation. This was especially critical for the Boxmeer facility in the Netherlands, seen in the photograph at the start of the article, as one of their bioreactors consistently produced a lower amount of yield compared with others in the facility. Interestingly, the bioreactor with the lower output was new, using state-of-the-art technology. Further investigation showed that this new technology used a different bioreactor mixing mechanism, leading to a different type of airflow inside the bioreactor. This implied that some of the controllable input parameters needed to be adjusted for that specific bioprocess (specific parameters are not disclosed for confidentiality). However, there was no available information in the literature to help decision-makers define the best configuration for these controllable input parameters. This implied that several experiments needed to be conducted at industryscale to collect data and optimise the controllable input parameters. However, industry-scale experiments involved very high risks due to process uncertainties, limited resources, and high operating costs. Therefore, a smart decision-making mechanism was needed to identify the best parameter configuration through minimum number of industry-scale experiments. Although this problem was motivated by a case study in the Boxmeer facility, it addresses a common industry problem in the context of optimal learning: industryor laboratory-scale experiments need to be designed in a smart way to collect the required information in the least possible number of experiments. This is especially critical when the resources for conducting these experiments are limited because of budget restrictions or operational constraints.
To address this problem, predictive models were built, based on the theory of Bayesian design of experiments. These models used limited amount of industry data to predict how bioreactor yield would change as a function
of the critical process parameters. Then, a stochastic optimisation model that belongs to the class of the optimal stopping problem was designed to control these critical process parameters. As a result, the model suggested an optimum process configuration based on the results of eight industry-scale experiments. The proposed configuration has been implemented for more than one year at MSD AH and enabled an increase in the bioreactor yield by 50% while reducing the yield variability by 25%. This also helped to improve the environmental sustainability of these processes through higher production outcomes per bioreactor run.
HOW TO DEAL WITH PLANNING AND SCHEDULING? Biomanufacturing operations are performed by highly skilled scientists using specialized equipment. Capacity planning for these limited resources is critical for successful and timely completion of orders. Failing to satisfy delivery dates results in loss of credibility and reputation. In addition, biomanufacturers face unique challenges in operational scheduling. Each client order requires several tasks to be completed. The tasks and their durations differ between orders depending on the final product requirements and quality of the starting material. The use of live cells often introduces ‘no-wait’ constraints between steps. The engineered nature of these products adds uncertainty at each step and imposes simultaneous requirements on highly skilled labour resources and specialized equipment to guarantee the best outcome. Creating a good schedule that can quickly react to these dynamics is a challenge. Despite these challenges, there is a need for a specific rhythm in the production system.
To address these planning and scheduling challenges, we first developed a simulation model of the biomanufacturing operations. The simulation model was developed with the Arena software, and contained 8000 connections representing 48 different products with their unique routings on 25 pieces of equipment and more than 50 process steps. The simulation model was validated using two years of historical production data on lead times, utilisations, bottlenecks, inventory levels, and throughout. Then, simulation-optimization was used to generate a portfolio of flexible production schedules for each week (namely, rhythm wheels). The optimisation module used the Tabu search algorithm to maximise the throughout. The tool was designed to enable MSD AH to quickly react to changes in their production system by dynamically adjusting their production schedules. In addition, the tool was also used to evaluate and justify capacity expansion decisions.
INVENT. IMPACT. INSPIRE ‘The project greatly serves towards our motto: Invent. Impact. Inspire.’ says Bram van Ravenstein, Associate Director at MSD AH. More specifically, it created novel tools to complement life sciences with operational research. The developed tools have been in-use at MSD for almost two years. As a result, the production outcomes of certain batches from the Boxmeer facility increased by 97% without investments on additional resources, such as equipment and workforce. Recently, several follow-up projects were initiated nationally and internationally. For example, the Boxmeer facility is currently collaborating with several other facilities to help them use the O.R. tools. The upper management has recently encouraged new initiatives for knowledge transfer to the human health department. As more companies embrace the applications of O.R. methodologies, the impact will be significant for the society – cheaper and faster access to lifesaving treatments. As Oscar Repping, Executive Director at MSD AH states, ‘We [the industry] will benefit from O.R., as such, we will avoid investments, we will become more predictive, leading to cost reduction, leading to more capacity on our production lines, meaning that we can make this world a better place.’
Tugce Martagan is an Assistant Professor in the Department of Industrial Engineering at Eindhoven University of Technology. She received her Ph.D. in Industrial and System Engineering from the University of Wisconsin-Madison in 2015. Her research focusses on stochastic modelling and optimisation, especially in the context of the pharmaceutical industry and healthcare operations management.