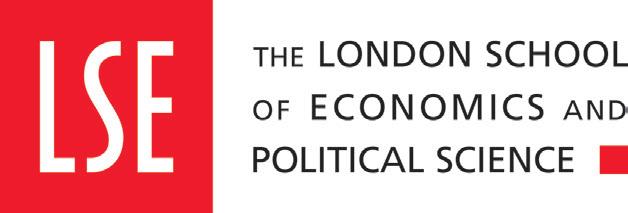
19 minute read
Universities making an impact
EACH YEAR, STUDENTS ON MSC PROGRAMMES in analytical subjects at several UK universities spend their last few months undertaking a project, often for an organisation. These projects can make a significant impact. This issue features a report of a project recently carried out at one of our universities: London School of Economics. If you are interested in availing yourself of such an opportunity, please contact the Operational Research Society at email@theorsociety.com
CUSTOMER FEEDBACK TEXT ANALYSIS AND ANALYTICS
(Kaiyi Chen, LSE, MSc Operational Research and Analytics)
BT is the UK’s largest provider of fixed-line, broadband and mobile services, and also provides subscription television and IT services. As a consumer-facing organisation, providing excellent customer experience is a key strategic goal and numerical measures of customer loyalty are used for feedback. This is complemented by analysis to create a clearer picture and to help focus service improvements where they’ll have the most benefit.
Kaiyi’s project explored the potential for using advanced text mining techniques and associated analytics to generate recommendations to the business based on a large pilot set of anonymised customer survey data. The core was the application of methods taught on the course but it was clear from the start that she would have to research additional tools and would need considerable consultancy and interpersonal skills to work across teams to deliver a meaningful outcome against tight deadlines.
Kaiyi was selected by BT from the shortlist forwarded by LSE in part
because of her exceptional academic record but also because of the strong ‘systems focussed’ thinking she demonstrated. She came to the LSE with a first class Materials Science degree from Oxford University and experience of leading a solar cell technology research team as well as consultancy with Accenture. Many students on the course have a mathematical background but some of the projects with most impact have actually been delivered by numerate engineers like Kaiyi with a broader background, and the LSE is looking for ways to encourage more engineers to join the course.
Jonathan Malpass, a Research Manager in BT’s Applied Research department, originated and supervised the project for BT. Jon said: “When we signed up to the LSE programme we knew that success is never guaranteed, but Kaiyi exceeded our expectations on a challenging project and delivered results that were genuinely useful to the business. She demonstrated both her technical and ‘soft’ skills on this for BT and her Distinction for this part of her masters degree was fully deserved.”
Kaiyi agreed that it had been something of a leap into the unknown, but she was well supported by the BT team. “The project allowed me to apply the research and analytical skills I accumulated during my studies and previous work experience, but it also helped me develop a wide range of valuable consultancy skills that have already proved very useful.”
The aim of the projects on the MSc programme is to develop and test the consultancy and project management skills taught on the course as well as the students’ technical ability. This is demanding on the students and on the LSE’s supervision team, who have to coach and mentor them through the project. However, the LSE believes the result is a more rounded and more employable graduate who has demonstrated more than technical competence. Kaiyi is now working in London with British engineering consultancy Atkins as a transport consultant.
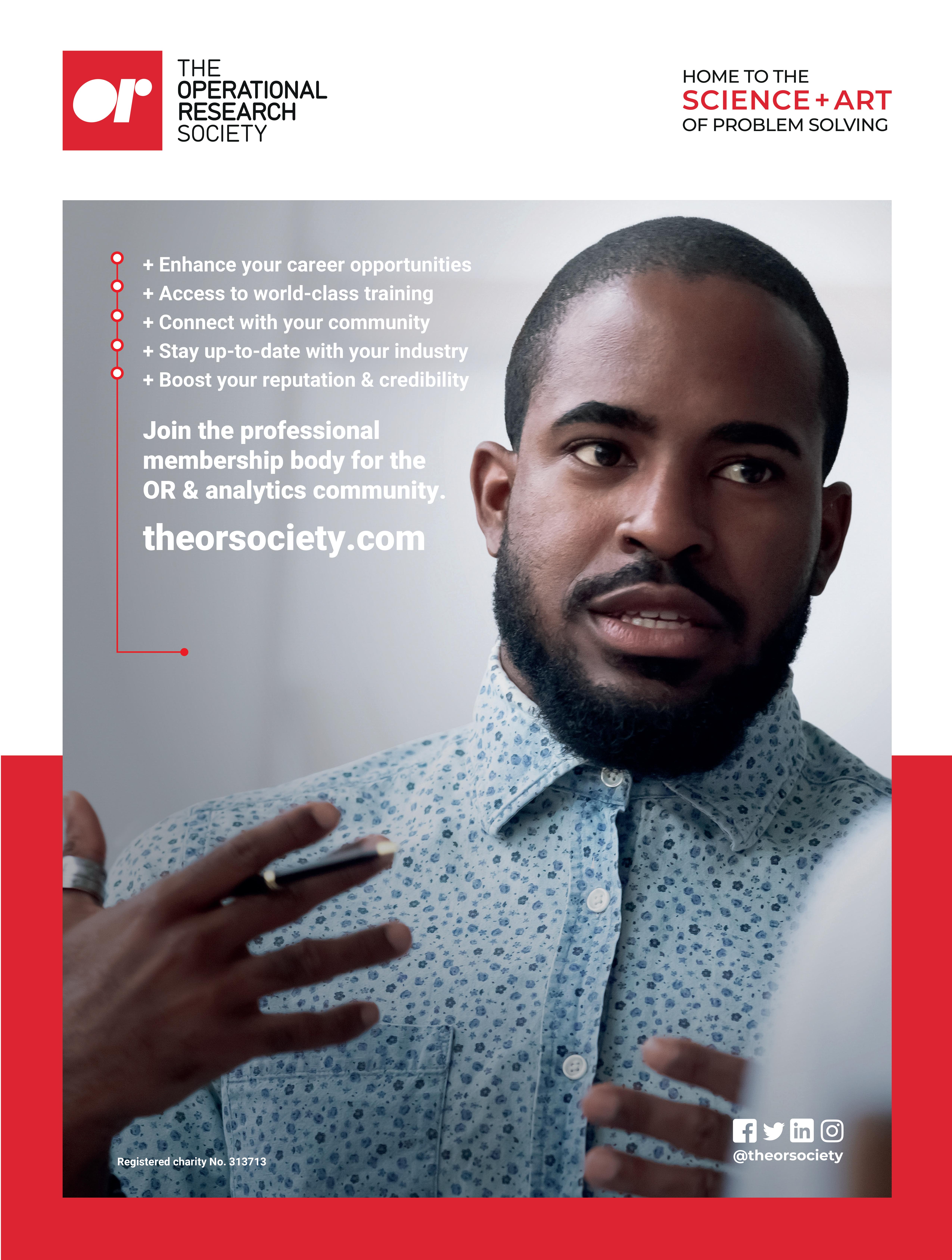
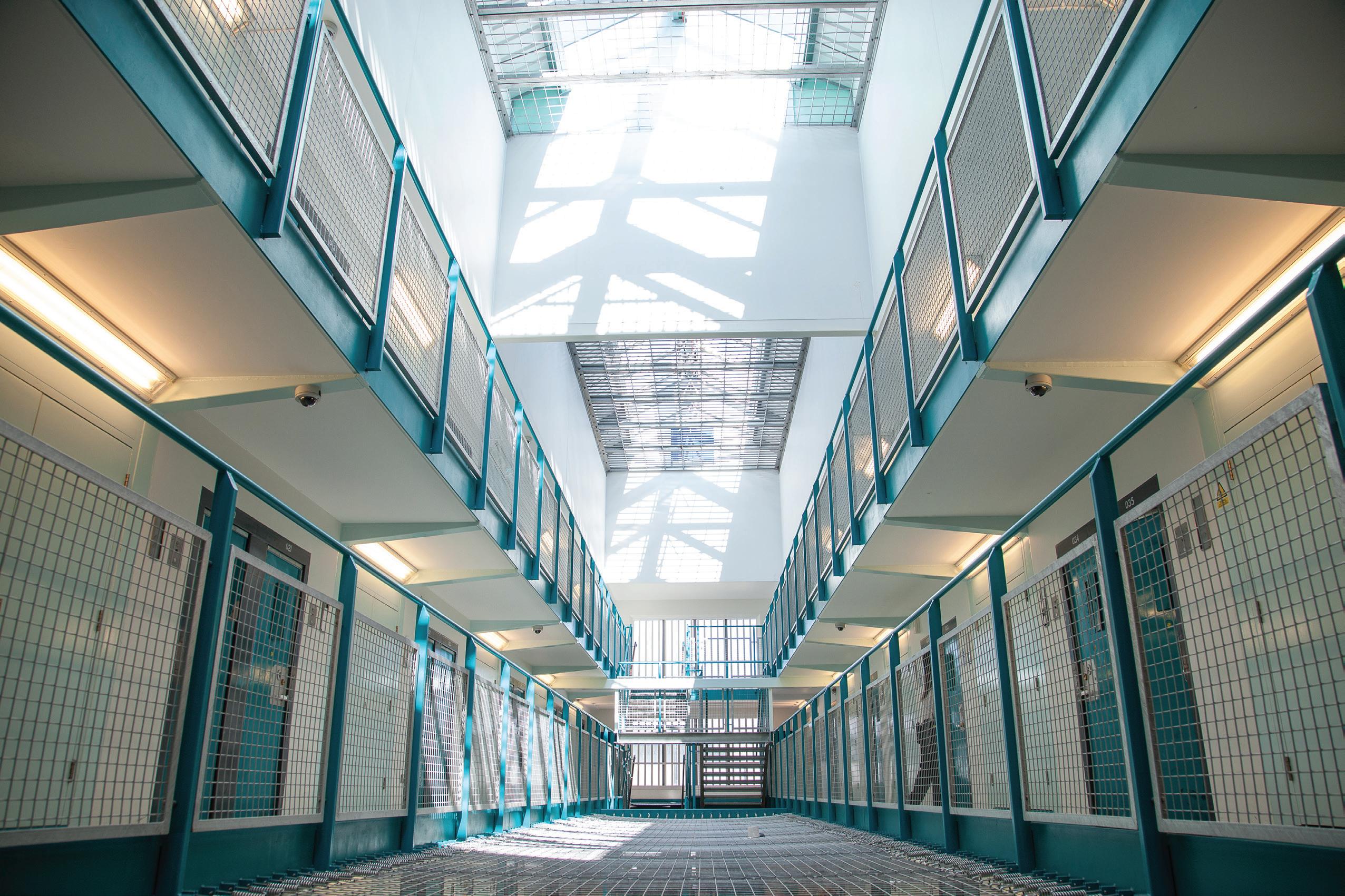
HARNESSING OPERATIONAL DATA TO MAKE PRISONS SAFER
MARTINE WAUBEN, PHIL MACDENT, ADAM BOOKER, AND BEN MARSHALL
STORIES ABOUT THE SUFFERING caused by violence, self-harm, and deaths in custody can make for difficult reading. At any one time, there are roughly 83,000 people in prison custody. However, annually we see more than 34,000 assaults, 57,000 self-harm incidents, and just over 300 deaths in custody. These figures are taken from https://www.gov.uk/government/ collections/safety-in-custody-statistics. The Ministry of Justice are responsible for safeguarding everybody in the justice system. Keeping people living and working in prisons safe is the top priority for the prison service, and we are exploring all possible approaches to reduce these levels.
The Ministry of Justice’s Data Science Hub was set a challenge: how can Operational Research (O.R.) help reduce the rising levels of violence in our prisons?
We needed to harness operational data, deliver top-notch O.R., and manage its delivery. It was vital that our insights would be embedded inside the prison service. It is all well and good having fabulous figures and shiny sums, but if operational researchers can’t embed their work inside business delivery, it has no impact, no benefit, and no longevity. Our job was not just to be accurate, it was to be useful and novel in our delivery to stand the test of time.
THREE CHALLENGES We used problem structuring methods to find opportunities for analytical approaches to tackle this challenge. We found that front-line staff were not in a position to best use data for three reasons.
UNSUNG HEROES Our first obstacle was that operational data took too long to get from the database to the reports used on the front line. In some cases, the process took weeks. We would have to wait for static extracts from our legacy systems, make copies for different team members, manually clean them all, and somehow discern which spreadsheet was the true final version. No doubt this lengthy, error-prone, human process is familiar to many working in large organisations with legacy systems. To ensure that staff could use the information to make operational changes, we needed to deliver insights from this data before it became outdated.
Our data engineering team has created a central database that automatically refreshes every night. Each morning, this provides us with all incidents from 2010 to yesterday. At the end of the pipeline sit opensource tools for analysis, pointed at millions of rows of data about individuals, sentences, and incidents. These tables may be massive, but we are able to explore the data, test hypotheses, and visualise our
1. The data wasn’t timely enough; 2. Systematic approaches to identifying and understanding the individuals driving violence in custody were limited at best; 3. Data wasn’t delivering the right insights.
As a result, data was not used optimally in decision-making and O.R. wasn’t embedded in front-line processes. Therefore, we set out to not only deliver top-notch analysis, but also resolve some of these organisational problems that would prevent our insights from being embedded and used. results in a matter of seconds using tools like JupyterLab and RStudio. This improves our analysis and its reproducibility.
Engineering teams like this are the unsung heroes of high-impact data science. We have used technologies like AWS, Docker, Spark and Parquet. Our analytical platform is fully coded in the open. It is of such high quality that other government departments are now using our codebase to learn lessons for their own development.
This is a step change for government O.R. and underlies a lot of the impact we were able to deliver.
VIOLENCE VARIABILITY The second obstacle we tackled was the fact that systematic approaches to identifying and understanding the individuals driving violence in custody were limited. We set out to provide staff with individual-level information on prison violence. This boils down to a textbook O.R. question: for any given person in custody, how violent should we expect them to be?
This process resulted in the Violence in Prison Estimator (ViPer). It is a
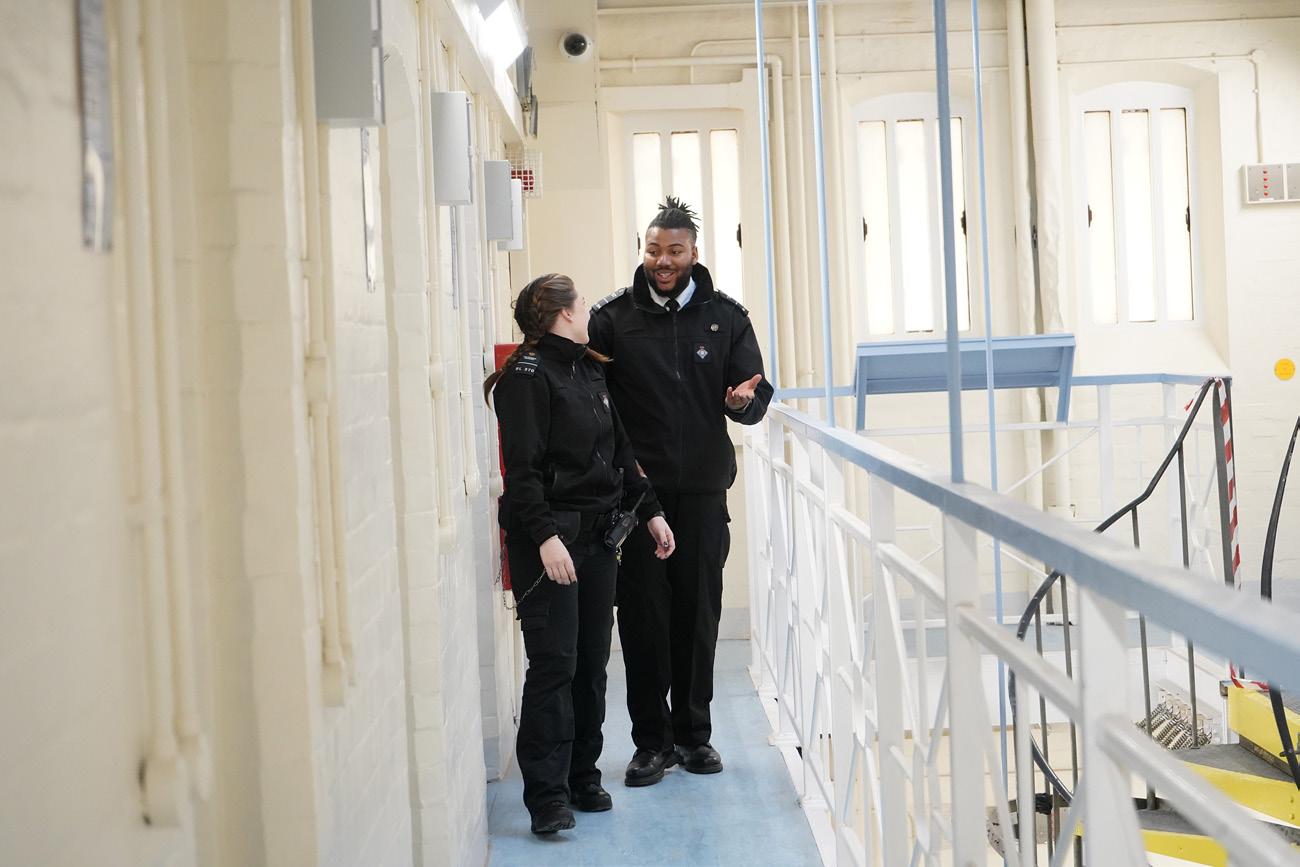
linear mixed effects model to estimate how violent an individual may be during their time in custody, based on that person’s behaviour during previous time in custody. We adjust for the background level of violence in each prison and the historic upward trend each year by including them as random population effects in the model.
Crucially, the model also includes a random effect that allows the model to vary its outputs based on an individual’s unique ID. This makes its estimates tailored to each individual. The model may seem deceptively simple, but this individual random effect makes the model hugely computationally intensive!
ViPer does not produce a prediction, but a description of a person’s current rate of violence. This is one piece of information staff can use to inform their approach to offender management.
To make such a tool acceptable to prison staff, we needed to balance ease of use and transparency. This modelling approach is fully transparent, and allows us to separate out populationwide trends from individual effects. This standardisation is important to ensure ViPer doesn’t change when individuals are transferred to a different prison, which would be difficult to explain to staff.
We also needed to communicate uncertainty. ViPer needs some information about individual custodial history to be accurate. If a person is completely new to custody, it will be unreliable. We used the model’s standard error to approximate this uncertainty, and communicated that through a one- to three-star rating system.
As a result, we have an easyto-use tool that can inform population management. This has had a transformative effect on the conversation around violence in custody, and allows staff at all levels to have common terminology to describe individuals across the estate.
DELIVERY REVOLUTION The final obstacle was that prisons are a low-tech environment. The IT in the prison service is, by necessity, very locked down and secure. However, as a side-effect the systems were not suited to delivering our insights to the front line.
We achieved a break-through with the Safety Diagnostic Tool (SDT). The SDT integrates R Shiny and bespoke HTML/CSS/Javascript/D3.js with existing IT infrastructure.
The basic premise is that simply using the data properly, applying innovative analytics to it, and a strong focus on user needs, combine to a very powerful tool. The SDT covers the number of assaults that have happened, and what sort they were. It covers how many people are self-harming, and how often. It lists the most recent incidents, so staff who weren’t on shift yesterday can catch up. And it lists the individuals most in need of management, as evidenced by ViPer.
Besides individual-level information, it also includes dashboards that show trends over time: what are the most common reasons for assault? What selfharm methods are on the rise? When and where are incidents happening?
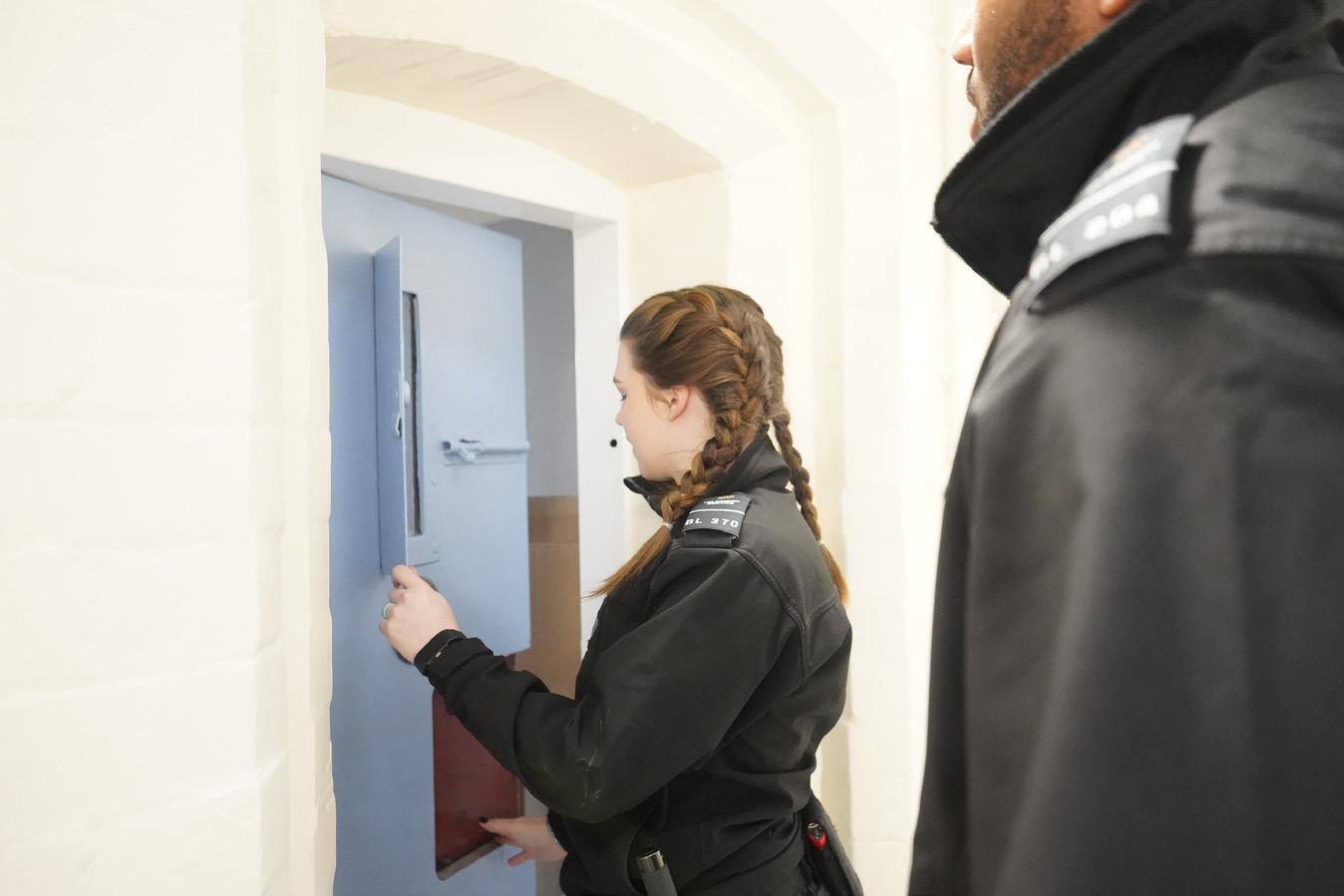
All this can inform regime changes: are scuffles breaking out when queueing for food? Maybe unlock fewer wings
at a time. Are people using medication to self-harm? Maybe officers should keep a closer eye on whether everyone is taking their own drugs. This is a revolution in how data is used to make these crucial decisions, potentially saving lives.
DATA-DRIVEN DECISIONS The SDT has been a massive success. We have thousands of registered users and get more than 200 unique daily log-ins across 120 prisons. The SDT is now part of most safety officers’ standard daily routine and they have come to rely on it for crucial tasks like preparing assessments.
Feedback has reflected how integral the tool is. It is now so embedded that it will continue to be used for many years. Front-line staff have said that “this will be the ‘go to’ tool in regard to incident management and planning of prisoners involved in most incidents”, and “I think it’s a superb tool. Now that I have it I would not want to let it go”.
The benefits are tangible. We have seen teams use ViPer to better spread the risk of violence across prisons and wings, so they can better manage it. SDT data is used in assessing prisoners for security and cell-sharing risk. It allows for more bespoke interventions for individuals because, most crucially, it saves staff huge amounts of time previously spent behind desktops in offices collating all this data.
Staff now also recognise the value of data. One wonderful result has been that officers are better at recording information, which we can then use to improve our analysis. For example, after we rolled out, staff were much more likely to record the reasons for assaults. We can now use this to spot patterns and anomalies, which then get fed back to improve services. Thus, the benefits all focus on delivering better front line services.
THREE SOLUTIONS We tackled each of the three significant obstacles to using data on the front line:
1. We made data available by refreshing the data overnight and automating cleaning and pre-processing, thereby improving timeliness. 2. We used innovative, advanced
O.R. methods to provide a way to systematically identify and understand individuals driving violence in custody, and adapted them so they could be accepted as business-as-usual. 3. We revolutionised the delivery of these insights through an interactive user interface.
All steps were crucial for setting the scene to fundamentally change how data and O.R. are used by staff all the way down the management chain, and how it is delivered to those brave people trying to change peoples’ lives every day.
The SDT has created a lasting culture shift, with demonstrable benefits for the front line. Our analysis supports those making a difference in the lives of people in custody. By meeting staff where they are, we can embed insights into existing processes. We help them make more data-driven decisions to rehabilitate and support offenders and keep the public safe. Fundamentally, that is what we are here to do.
Martine Wauben is a Data Scientist and Government Statistician at the Ministry of Justice, focusing on self-harm in custody. She has a background in Psychology and Epidemiology, through a BA from the University of Oxford and an MSc from Utrecht University.
Phil Macdent is the Head of Prison Data Science at the Ministry of Justice, working on improving outcomes for offenders and reducing work pressures for staff through innovative data science solutions. He holds an MSc in Mathematics from the University of Warwick.
Adam Booker is the Head of Prison Data Engineering at the Ministry of Justice. He holds an MSc in Operational Research from the University of Southampton and has a background in data science.
Ben Marshall joined the Civil Service after studying philosophy and criminology at the University of Sheffield. He is currently pursuing a PhD in statistics. His research focuses on developing probability models for machine perception.
This work was awarded the OR Society’s President’s Medal in 2019.
This article is published under an Open Government Licence http:// www.nationalarchives.gov.uk/doc/ open-government-licence/version/3/
THINK LIKE A ….?
Geoff Royston
gives of engineering thought and action – reducing traffic congestion, introducing just-in-time production, or even the development of London’s Victorian sewer network. So maybe a better question is; are there ways in which engineers think differently from analysts? (apart of course from those who have an engineering background!) - and if so, are there lessons for improving the way analysts work with clients?
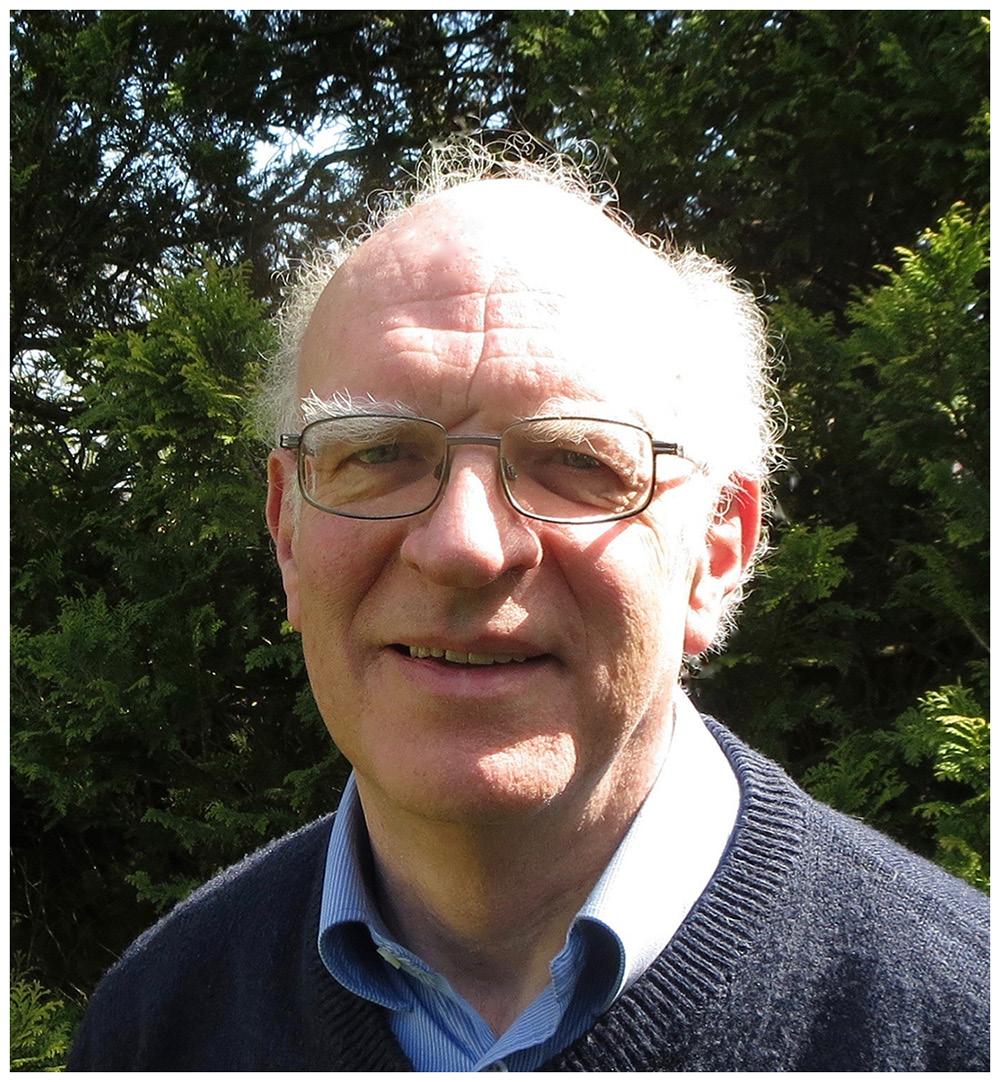
You can’t judge a book by its cover, nor sometimes even by its pages. If you saw a book with chapter headings such as “optimising” and “solutions under constraints,” you might well think you were looking at a textbook on operational research. If you then flipped through the pages and saw phrases like “modelling techniques” and “parameter variation”; you might feel your assumption was confirmed. But, for the publication to which I am referring here, you would be wrong.
The book is Think Like an Engineer by Guru Madhavan. As well as chapters with the headings mentioned, there are also chapters on “prototyping” and “learning from others,” which one might not be so likely to see in an O.R. textbook. Which raises the question, how far do - or should - analysts, think like engineers? How relevant is the engineering mindset to tackling managerial and policy issues? Anyway, how do engineers think?
HOW ENGINEERS THINK In his book, praised by readers such as Alvin Roth, economics Nobel prize winner, and Tim Harford, of BBC’s More or Less, Madhaven describes engineering thinking as having three key characteristics: ability to see structure (especially where there currently isn’t any); ability to deal with constraints (e.g. physical, financial or behavioural); and ability to make trade-offs between conflicting objectives such as performance and cost; finding the intersection of feasibility, desirability and affordability.
This begins to look a lot like an O.R. mindset, a view that is reinforced by some of the examples that Madhaven
THE BENEFITS OF FAILURE Madhaven’s book gives some hints about this. He explains that engineers are very interested in failure, or to be more exact, on the long journey of exploration and evolution to move from a primitive prototype to a polished end product (a journey which often is not recognised, as it is only the final product that most people see).
In explaining the importance of failure and step-wise refinement in engineering Think Like an Engineer gives the example of the opening ceremony of the 2012 London Olympics, where part of the brief was for a scene depicting the industrial revolution; with factory chimneys, steam engines and so on, all to be delivered live and to full-scale and to immediately follow an act featuring the English countryside complete with meadows and grazing animals. How to achieve a seamless transition from pastoral to urban? How could large props like chimneys be erected and dismantled quickly, and be concealed before and after the scene? Use digitally projected images perhaps? Or painted chimneys on fabric which could be unfurled, and rolled up, rapidly? No, the client said that nothing less than real 3-D chimneys would do. The technical team experimented with numerous models, none of which proved satisfactory. Then they came up with the idea of inflatables. After much prototyping they produced an inflatable, full-height, realistic, chimney. At the opening ceremony no less than seven “brick” chimneys rose majestically thirty metres into the sky, they even had smoke coming from the top.
We could argue of course that O.R. and analytics professionals also engage in iterative design, for example by using simulation modelling to explore different possible solutions to a problem. But are analysts quite as devoted to spending time on searching for a wide variety of potential solutions, to testing and evaluating prototypes in the real world, to extracting every bit of learning from failure, as the engineer?
DECISIONS OR DESIGNS? For that matter, do O.R. and analytics treat the whole business of design as seriously as do engineers? Madhaven describes the core of the engineering mindset as being about “modular systems thinking - combining systems thinking with systems building” and notes that “if the core of science is discovery, then the essence of engineering is creation.” Design lies at the very heart of engineering – whereas, in the way it is typically presented, design appears to be somewhat peripheral to O.R. and analytics. O.R. analysts generally talk first about helping clients make better decisions, and may not even consciously consider their work as also being about design. Yet appearances can be deceptive, improving any system entails both analysis and synthesis, and so O.R. and analytics often involves building better systems for clients – undoubtedly a design task. It matters if analysts – or clients – do not recognise this; for instance analysts operating only “downstream,” assessing and helping clients decide between a set of given options, risk overlooking other, better, options that might have been identified if analysts had also been involved at the “upstream “conceptual design stage. (Two of the past giants of O.R. - Russ Ackoff, Herbert Simon – did stress the importance of design - as their respective books, Idealised Design and The Sciences of the Artificial, demonstrate - but their message seems not to have been sufficiently heard. Yet managers have been increasingly realising the relevance of design principles and thought – as evidenced for example by the book by Roger Martin, The Design of Business: Why design thinking is the next competitive advantage.)
HOW ENGINEERS MAY NOT THINK Engineers have to be good with objects and ideas but are maybe not always so good with people – as caricatured by Dilbert, the engineering nerd created by the cartoonist Scott Adams. The importance of engineers balancing rationality with empathy is stressed by Madhaven: “engineers should rise above the comforts of cold, mechanistic, isolated problem solving.” This involves gaining an insight into what clients want, making sure they are involved right from the start in a process of codesign and coproduction to ensure that complex technologies are approachable and accessible. That must go for analysts too.
LEARNING FROM OTHERS At its worst, a failure to listen to customers, to respect their existing knowledge and expertise, can lead to catastrophe.
Think Like an Engineer gives the example of the water temples of Bali. For millennia its rice terraces used an integrated system of organic farming and shared water management. A network of irrigation tunnels allowed monsoon rainwater to be shared between upstream and downstream farmers, synchronising harvests and ensuring both reduced pest attack and water conservation. This wisdom of centuries of experience was ignored when an attempt was made to increase rice production by introducing high yield varieties, pesticides and chemical fertilisers, hoping to emulate the green revolution that had transformed grain production in India. But here “miracle rice produced miracle pests”; millions of tons of rice were lost, soil erosion and collapse of the water system followed. Subsequent computer modelling confirmed the superiority of the traditional approach in the local physical and cultural environment.
THINKING, HARD, SOFT AND CREATIVE So, maybe the message for analysts from Think Like an Engineer is that the mindset of engineers is not so different. Both are concerned with structuring problems, with dealing with constraints and with clarifying tradeoffs. Both need to ensure that they pay attention not only to the “hard” technical facets of problems but also to the “soft” social and behavioural aspects. But engineers do seem to differ in placing creative design at their professional centre; analysts could usefully give more emphasis to their own creative thinking and systembuilding skills – and do more to develop and deploy these further.
Dr Geoff Royston is a former president of the OR Society and a former chair of the UK Government Operational Research Service. He was head of strategic analysis and operational research in the Department of Health for England, where for almost two decades he was the professional lead for a large group of health analysts.