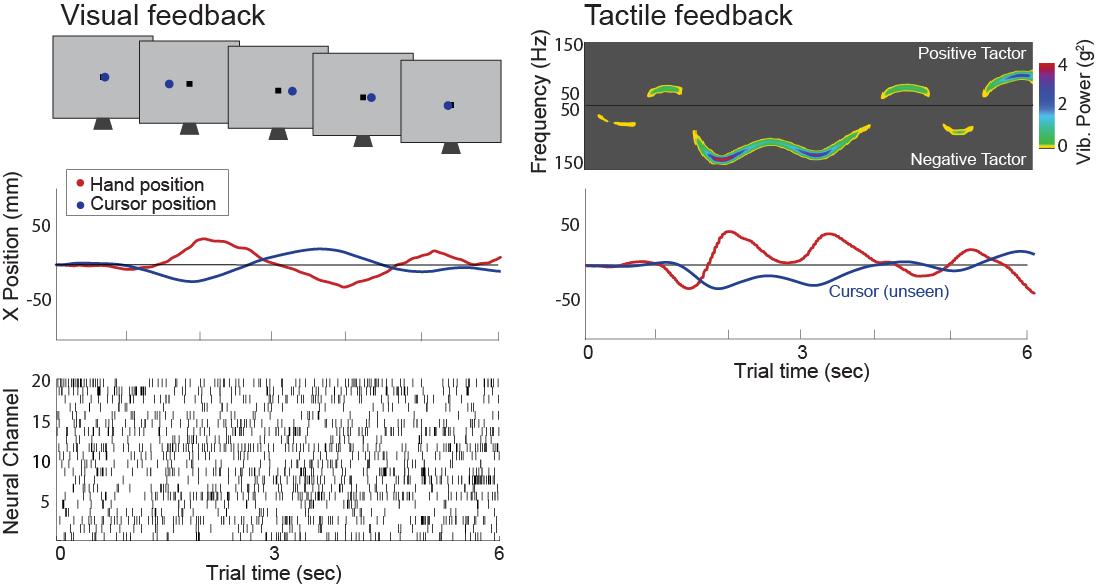
4 minute read
Aaron Batista, PhD
that 302 Benedum Hall | 3700 O’Hara Street | Pittsburgh, PA 15261requires continuous sensory-driven motor interaction with a simplified
The P: 412-383-5394task can be scaled in the future to embody more complex interactions. aaron.batista@pitt.edupredictions about neural responses in sensory and motor areas of the concert to https://smile.pitt.eduintegrate sensory information for the control of ongoing movements. e combine multielectrode neurophysiology with quantitative analysis of organized to inform a computational model of sensory-motor interaction our research is the Critical Stability Task (CST), wherein subjects must (vision and/or tactile) in order to make corrective actions to maintain a computer display for several seconds. Corrective actions to move the cursor hand movements, or via BCI control. The CST was originally formulated [31] dynamics governed by the differential equation,
Sensory-Motor Integration Laboratory and Engineering
Dr. Aaron Batista’s research group examines the neural control of visually-guided action. We seek to understand basic principles that underlie the function of the cerebral cortex, and to use those discoveries to improve the function of clinical brain-computer interface (BCI) systems as a treatment for paralysis. Here we describe two of the research endeavors taking place within the laboratory.
Neural constraints on learning
Why are some new skills learned relatively quickly, while others take far longer? What changes in the brain when we learn, so that pre-existing abilities are retained even as we learn new skills? We are examining the neural underpinnings of learning using a novel paradigm, brain-computer interface control, which allows us to study the neural basis of learning more directly than is possible with arm movements. We can directly request of our animals that they generate specific patterns of neural activity across a population of 100 or so neurons. We can then observe whether the animals are capable of generating the patterns we requested. Mathematical tools drawn from machine learning enable us to predict which new neural activity patterns (and, corresponding skills) are relatively easy to learn (a day or so), and which will be more difficult (a week or two), just by examining the pre-existing patterns of neural activity prior to learning. This work is pursued along with colleagues Byron Yu and Steve Chase of Carnegie Mellon University. It is currently supported by an NIH R01 grant from the National Institute of Child Health and Human Development.
Multisensory Integration in Action
Our actions are shaped by our perceptual experience. Consider the fine adjustments a violinist makes to play the correct pitch. Sensory experience is often multimodal: DEPARTMENT OF BIOENGINEERING we see, hear, and feel the thing with which we are interacting, and our movements are adjusted on-the-fly to achieve our objectives. We seek to understand how
!" ! !" the brain uses sensory information to guide action, we train animals to perform challenging balancing tasks in a virtual environment. We record from motor and sensory areas, in the hope of discovering how the areas communicate to send detailed sensory information to sculpt the activity of motor neurons. This work is pursued along with my Pitt Bioengineering colleague Pat Loughlin. It is supported by an NIH R01 grant from the National Institute of Child Health and Human Development.
= ! ! ! +! ! , ! > 0, ! ≥ 0 (1) of the cursor (with x>0 representing the cursor to the right of center), ! ! generated by the subject, and t=0 indicates the start of the trial. The of the parameter, !. Because ! is positive, the cursor position will diverge compensating actions (control commands) are issued by the subject [89]. As ! faster, and the CST is more challenging. The maximum ! (which has units of control the cursor is related to the bandwidth of the stabilized closed-loop instability value” (CIV) since, beyond that value, the closed-loop system CIV, the more effective is the subject’s sensorimotor control. the investigation of motor control that requires sensory feedback, consider required to keep the cursor from drifting, i.e., what is !(!) such that the cursor condition (!"(!) !" = 0) into Eq. (1) shows that the subject must generate a equal and opposite to the current cursor position: ! ! = −!(!). Thus, must know the state of the cursor. A subject who could issue this perfect 100% accurate) would be able to keep the cursor completely still. command is not possible because of sensorimotor noise and nervous system will continuously move, and maintaining control – keeping it from rapidly ongoing sensory-guided motor action (Fig. 1). With adequate sensory command signal that keeps the cursor centered about an equilibrium point subject’s CIV. A subject’s CIV captures important aspects of their speed and accuracy with which he or she can react to sensory input. The effective the CIV provides an measure of several type of our sensory control), the movements or Fig. 1. Two CST trials. 1st row: and the (schematic of) visual [left] and and less motor faster (spectrogram of) vibrotactile [right] feedback. 2nd row: cursor and hand position traces. Bottom left: raster of multichannel neural activity from M1. modality or establish the CIV for each animal under each of the configurations of
Professor
RESEARCH LETTER
a
Units
Neural activity
Time
BCI mapping Neural activity: 85–91D
b
Unit 1 Unit 2 Unit 3
1 2
3
Excitatory Inhibitory Unit 1 FR
c Outside-manifold perturbation control space Intuitive
d
Unit 1 FR Unit 2 FR Unit 3 FR Cursor velocity
Within-manifold perturbation control space control space perturbation control space control space Unit 1 FR
Unit 2 FR Figure1 | Usingabrain–computerinterfacetostudylearning.a movedtheBCIcursor(bluecircle)toacquiretargets(greencircle)by modulatingtheirneuralactivity.TheBCImappingconsistedoffirstmapping thepopulationneuralactivitytotheintrinsicmanifoldusingfactoranalysis, thenfromtheintrinsicmanifoldtocursorkinematicsusingaKalmanfilter. Thistwo-stepprocedureallowedustoperformoutside-manifoldperturbations (bluearrows)andwithin-manifoldperturbations(redarrows).D,dimensions. b,Asimplified,conceptualillustrationusingthreeelectrodes.Thefiringrate (FR)observedoneachelectrodeinabriefepochdefineapoint(greendots) intheneuralspace.Theintrinsicmanifold(yellowplane)characterizesthe prominentpatternsofco-modulation.Neuralactivitymapsontothecontrol space(blackline)tospecifycursorvelocity. c,Controlspacesforanintuitive mapping(blackarrow),within-manifoldperturbation(redarrow)andoutsidemanifoldperturbation(bluearrow). 9d,Neuralactivity(greendot)elicits differentcursorvelocities(opencirclesandinset)underdifferentmappings. Arrowcoloursasin c.