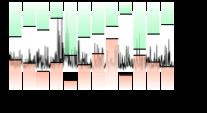
1 minute read
Daniel R. Jiang, PhD
1002 Benedum Hall | 3700 O’Hara Street | Pittsburgh, PA 15261 P: 412-624-1852
drjiang@pitt.edu http://www.pitt.edu/~drjiang Assistant Professor
Research Overview
Daniel Jiang’s research interests are in the area of sequential decision making in the presence of uncertainty, where he has a special interest in handling the theoretical and computational challenges introduced by risk. Previous research has focused on topics in energy, an application area that is not only timely and relevant considering today’s rapidly changing energy landscape, but has also proven itself to be a rich problem domain, due to inherent stochasticity, the often-present curse of dimensionality, and the importance of managing risk in a sequential way.
Research Projects
• Approximate dynamic programming algorithms that exploit structural properties of intractable sequential decision problems. • Optimal policies for energy arbitrage involving dynamic bidding strategies. • Data-driven methods for solving risk-averse sequential decision problems. • Spectrum of risk-averse policies for electric vehicle charging policies. • Monte Carlo tree search using information relaxation upper bounds applied to ride-sharing. • Naloxone procurement and distribution amidst the opioid crisis. • Policies for incentivizing voluntary demand response.
B
risk parameters allorder–up–topolicies
R⇤(B)
risk–averseoptimalpolicies

Biographical Sketch
Daniel Jiang joined the Department of Industrial Engineering in September 2016. He received his PhD in May 2016 and MA in May 2013 from Princeton University in the Department of Operations Research and Financial Engineering. He completed his Bachelor’s degrees in Computer Engineering and Mathematics from Purdue University in May 2011. The methodologies developed in his dissertation, titled “Risk-Neutral and Risk-Averse Approximate Dynamic Programming Methods,” are readily applicable to sequential optimization problems related to the electricity market, energy storage, integration of renewables, demand response, and electric vehicles.