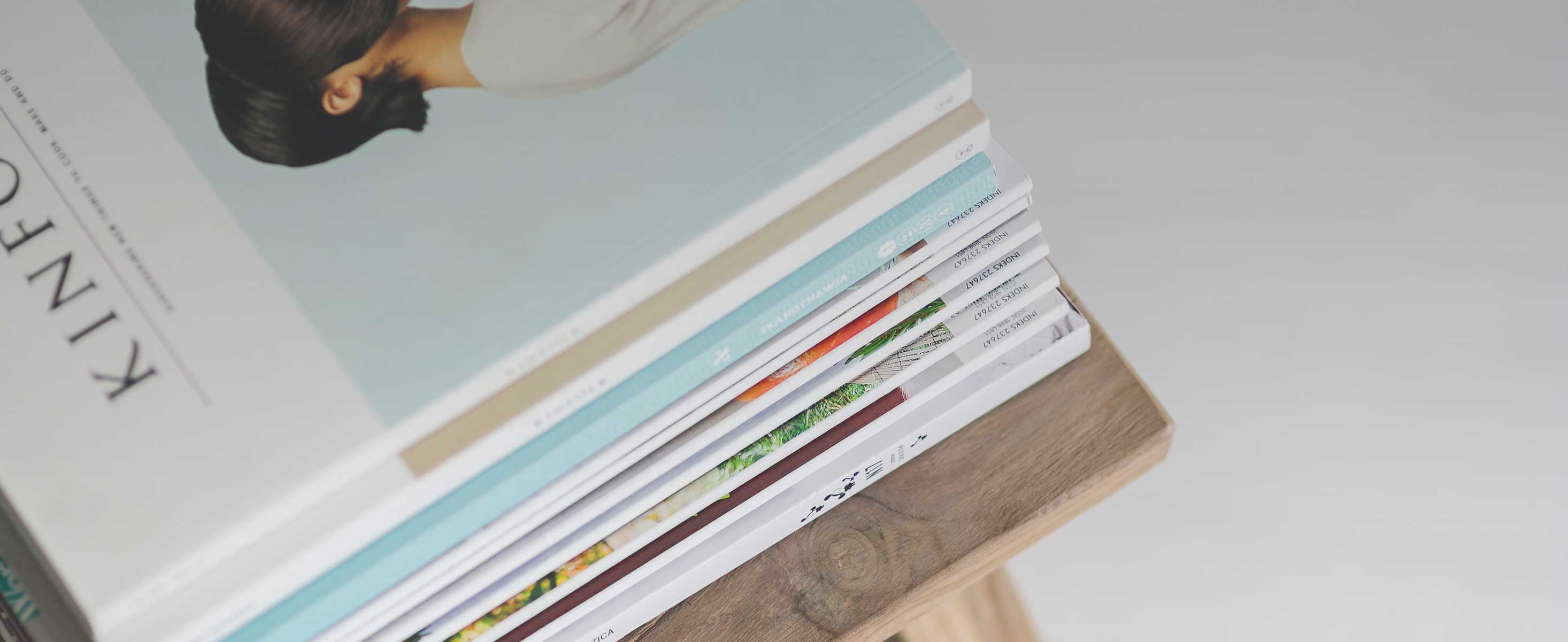
8 minute read
Life: Decoding disease
Features Life
In April 2003, the fi rst human genome was sequenced – in other words, the 3bn-long sequence of the four chemical groups constituting our DNA code were recorded in the form of one letter for each group, covering 100,000 pages of A4. Since then, we have rapidly accelerated our understanding of how the approximately 3m small genetic diff erences between each one of us aff ects who we are, infl uencing our height, hair colour, intelligence, susceptibility to disease, how long we live and so on.
From an actuarial point of view, though, surprisingly little has changed. In 1997, the
UK government and Association of British
Insurers agreed the Concordat and
Moratorium on Genetics and Insurance, which has successfully managed patients’ concerns around their interests, as well as insurers’ fears around information asymmetry. Nonetheless, since the UK
Biobank released genetic and health details for 500,000 participants in 2015, genetic understanding has accelerated – and change for actuaries is coming. Falling costs and increasingly connected knowledge are helping to form a virtuous circle, and many new applications of this knowledge beckon.
Genetic pinpointing
The progress on costs has been remarkable: the fi rst human genome took 15 years to complete and cost US$2.7bn, whereas we can now perform this feat in a day for around US$1,000. This means researchers can now conduct very large studies – for example, recent lifespan research at the University of Edinburgh used the DNA of more than half a million subjects. These subjects were generally still alive, so we did not know their lifespans. However, we overcame this hurdle using information about their parents. We tested associations of inferred parental DNA with the parents’ 1m often complete lifespans. We detected a myriad of eff ects caused by DNA changes, spread across the entire length of the genome. Similar to discoveries for other common diseases, the impact of these relatively frequent gene changes were individually small – perhaps 0.25 years of expected lifespan.
This is not to deny that modest changes in DNA cannot have large eff ects, but that is the exception, not the rule. Nonetheless, initial clinical and actuarial focus were the exceptions – conditions where a single change in one gene had a big, often diseasedefi ning eff ect, such as in Huntington’s disease, cystic fi brosis and some forms of breast cancer. Genetic pinpointing was possible precisely because a specifi c change had a large and clear eff ect. In these cases, the principal mitigant to information asymmetry was the continuing ability of the insurer to use family history, bolstered by clinical practice that restricted genetic testing to those with family history of disease. In any case, these conditions are relatively rare, and in the great majority of
Features Life
cases, early-onset – manifesting before the age at which insurance would normally be taken out. A customer who had had a genetic test for a (rare) disease was often already showing clinical symptoms, or had family members with such symptoms. The underwriter could use these factors, even if a genetic test was not disclosed. Importantly, clinician-patient interests – carrying out predictive testing and informing interventions – did not need to clash with insurers’ interests, not least as public opinion would likely back the former.
Multifactorial traits
While the human genome project has continued to increase the scope and depth of our understanding of these rare genetically determined diseases, the biggest change has been in our understanding of more common diseases. The role of genes here is much more complicated. Environmental eff ects such as diet, lifestyle and social economic status combine with the small eff ects of many diff erent genes to aff ect an individual’s risk of diseases such as most forms of cancer or cardiovascular disease. These diseases are thus known as multi-factorial. This is an important but poorly understood insight; inherited DNA plays a (possibly small) role in many traits – some unsurprising, such as height or cholesterol levels, but also aff ecting traits such as education level, propensity to smoke, or even propensity to take risks such as speeding.
The fi rst tool in the geneticist’s box for multifactorial traits is ‘heritability’, which measures the degree to which a trait is genetically determined, on a scale of 0-100%. Such measures are diffi cult to make, but estimates are made by looking at how much a trait runs in families – and
Peter Joshi asks: what are the actuarial implications of the Human Genome Project?
Features Life
APPLYING THE INFORMATION
The fi rst application of these DNA-based prediction techniques is likely to be in screening programmes. As the cost of DNA measurement falls, health systems may seek particularly by examining similarities and to measure the whole population, diagnosing rare conditions before they appear but diff erences between twins. As well as issues also developing personalised risk predictions and, it is speculated, varying the age at of sample size and mathematical which programmes such as breast cancer screening start, depending on risk. For complexity, the principal diffi culty in actuaries, this raises the question of information asymmetry again. The whole of a measuring heritability arises from population might have this data, and family history is likely to be a poor proxy. The big determining whether the phenomenon unknown is whether such information will aff ect customer behaviour. running in families is determined by DNA A second, currently poorly understood, question is to what extent normal or by something else – particularly family clinical and underwriting measures made at proposal already capture these environment. For example, a person’s fi rst genetic eff ects. Scores have so far have typically been developed using European language runs strongly in families and is a ancestry, and their misapplication to other ethnic groups would prove good example of a family environment controversial and pose practical challenges. These are potentially real and eff ect, rather than a DNA eff ect. increasing risks to insurer profi tability and reputation, but their magnitude is Nonetheless, geneticists have made not yet quantifi ed, and their mitigation may not be straightforward. In estimates for many traits, common diseases addition, insurer mitigants and opportunities need to be developed in a way and risk factors, such as height (around 70% that does not deter genetic screening or invade genetic privacy. genetic – Golan and Rosset, 2011; Vinkhuyzen et al., 2013), IQ (35-50% – Davies et al., 2011), susceptibility to heart The actuarial perspective genetics usefully predictive of disease disease (around 40% – McPherson and Of course, the modest genetic share is, in or lifespan? Tybjaerg-Hansen 2016) and lifespan many ways, good news, as environmental At an individual level, the short answer (around 10% – Ruby et al., 2018). interventions can more easily shift the is no. At present, even individual height
The lifespan fi gures might seem modest, dial at an individual or societal level. In cannot be strongly distinguished from but our intuition is not always reliable here: any case, genetic research off ers many the top and bottom deciles of the best if your father or mother lived 10 years longer potential benefi ts, such as our improved DNA height scores. However, the same than average, how much longer than average understanding of biological pathways, is true of a socioeconomic status-based can you expect to live? We can measure this which can inform eff ective interventions. prediction of lifespan. Nonetheless, reliably, and the answer is a fairly modest 0.8 Recent evidence has shown that group distinctions are drawn that are years – around 1.6 years if it was true for your drug trials for disease are much more meaningful to annuity underwriters based other parent, too. Some fairly sophisticated successful in candidates for whom there on socioeconomic status. It is still early modelling suggests that only around half of was pre-existing genetic evidence for the days, but using a score that we can calculate that eff ect is actually DNA, with the rest target. A focus of my research programme for anyone at birth or later, using DNA alone, being family environment (socioeconomic is identifying target genes to slow ageing we have found a 10% increase in mortality status etc). This gives us our estimate that and extend healthy life. However, from rates per standard deviation in the score. variation in lifespan within the UK is an actuarial point of view, genetic evidence This equates to around fi ve years of life perhaps only 10% genetic. has a diff erent interest – namely, is between the score’s top and bottom decile. Similar predictions are now possible for the F IGURE 1: Survival curves for highest and lowest deciles of lifespan genomic risk score. A DNA-based lifespan score risks of common diseases such as heart was made for each subject. Each subject was then binned into a score deciles. Participants’ DNA scores were then used disease, where whole genome predictions to predict parent lifespan (because of insuffi cient subject data: the subjects, but not their parents, are generally alive. Nonetheless, as genetic eff ects transmit 50% from parent to off spring, natural inferences are valid. Eff ects illustrated across the population are now as predictive [across decile 1(D1) to decile 10 (D10 )] are diluted by 50% because of this). In due course, eff ect sizes on subjects’ own as those for the rare single-gene forms of lives will be twice that illustrated. diseases (Khera et Scottish: Parents E&W: Parents al., 2018). The 100% 90% 33,196 deaths / 46,936 lives 100% 90% 39,346 deaths / 58,070 lives accuracy of such predictions is likely PETER JOSHI is an actuary and a 80% 80% to improve Chancellor’s Fellow Survival probability 70% 60% 50% 40% 30% 20% Women: PRS D10 PRS D1 Men: 70% 60% 50% 40% 30% 20% Women: PRS D10 PRS D1 Men: signifi cantly during the next few years as researchers move from studying perhaps a million subjects to tens of millions, and our at the University of Edinburgh, specialising in the genomic basis of human lifespan 10% PRS D10 PRS D1 10% PRS D10 PRS D1 machine learning 0% 0% 40 50 60 70 80 90 100 40 50 60 70 80 90 100 becomes more Age (years) Age (years) fi ne-grained as a (Source: Timmers et al., 2019) result.