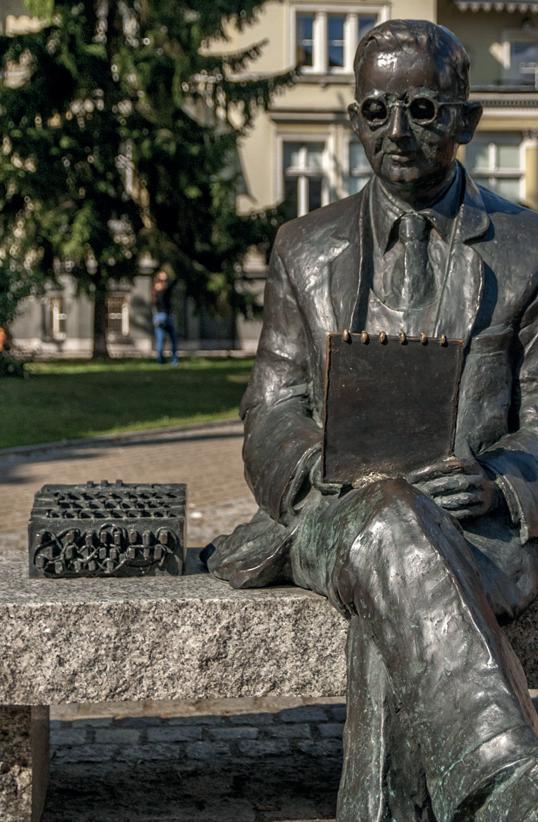
18 minute read
Letters
Upfront News
LETTER OF THE MONTH
Correcting the balance
This letter is prompted by David Forfar’s article, ‘Unsung heroes’, published in the July edition of The Actuary. David’s assertion that the achievements of the Polish cryptographers are not well known in the UK is certainly correct!
On 9 August 2018 The Times published an article (by David Sanderson) entitled ‘“Turing cult” has obscured role of Polish codebreakers’. This article pointed out that a nephew of Alan Turing was of the opinion that the ‘cult of Alan Turing’ had been taken to absurd extremes – to the extent that it overshadowed Bletchley Park’s debt to the Polish cryptographers. In an attempt to correct the balance, Sir Dermot Turing’s book X, Y & Z: The Real Story of How Enigma Was Broken was published in the following month.
In my presidential address to the Faculty in October 1992 I alluded to the Polish cryptographers. I did so to support my view that it is foolish to criticise a piece of research solely on the grounds that it has no obvious immediate application. The group theory studied by Rejewski and his colleagues as part of their university mathematics courses in the 1920s (at the University of Poznań) provided the key to their historic breakthrough. Today we must still be grateful that, more than 90 years ago, those young Poles paid such close attention to their fi rst lectures in abstract algebra.
Whenever I meet young Poles I always remind them of this country’s indebtedness to their compatriots.
PROF JOHN MCCUTCHEON
21 JUNE 2020
More comments are posted online about news stories published on www.theactuary.com IMAGE: IKONIMAGES STORMY FORECAST www.theactua ry.com www.theactuary.co m APRIL 2020 | THE ACTUARY | In-depth Modelling genesis and frequency. My focus is the statistical analysis of the number of major hurricanes in the North Atlantic since 1950, although bear in mind the number of hurricanes is only one of many factors of hurricane activity affecting insurance losses. Expected hurricane number and variance I used publicly available data from the HURDAT database of North Atlantic hurricanes, maintained by the National Oceanic and Atmospheric Administration to analyse the annual number of North Atlantic hurricanes that reached category 3 or higher (>180 km/hour) at some point before they dissipated. Kossin et al. ( Journal of Climate , June 2010) categorise hurricanes in four groups according to climatological characteristics. Figure 2 displays analysis for two groups containing some of the most dangerous major hurricanes. Group A hurricanes start near Africa and gather energy as they travel across the Atlantic; about one in five make landfall on the US east coast, around Georgia and the Carolinas. Group B hurricanes have straighter paths and are thus stronger, with approximately one in two hitting Florida or Gulf of Mexico states. Figure 2 shows the trend against the actual historical number and average number of major hurricanes since 1950. For both groups we see an increasing trend and higher M emories in insurance are often short. After Hurricane Andrew in 1992 caused problems for London Market insurers, there were not many hurricane losses until 2004, when four major hurricanes seriously impaired the profitability of several insurers. The following year, three major hurricanes – including Katrina – hit the US, leading to talk of increased hurricane activity and clustering. The debate then faded until, in 2017, a number of strong hurricanes caused much damage to the US and Caribbean. Climate change has put the Atlantic Basin in the spotlight, with a renewed interest in clustering. Figure 1 shows loss volatility between years. I produced this figure using the Weinkle et al. ( Nature Sustainability , FIG URE 1: Normalised losses from hurricanes h ave been increasing since 1990, often due t o several major hurricanes occ urring in the same year 2018) dataset: recalculated (normalised) historical hurricane losses adjusted for inflation and changes in exposure, such as the increase in the property stock and wealth. We observe both high year-to-year volatility and an increasing trend in annual damage costs. If one agrees with the normalisation of losses in Weinkle, then the observed increase reveals a recent increase in risk. Popular insurance wisdom often claims that annual hurricane losses come from one large hurricane rather than a high frequency of hurricanes in the same season. Figure 1 dispels this myth. There are years with high losses from a single catastrophic hurricane, such as 1992 and 2012 (Hurricanes Andrew and Sandy respectively), but there are also years in which high losses came from a number of major hurricanes, as in 2004, 2005 and 2017. Atmospheric science, in particular hurricane modelling, has progressed meaningfully during the past 40 years. Compelling accounts of this evolution can be found in the books Divine Wind by Professor Kerry Emanuel and Storm Surge by Professor Adam Sobel. Atmospheric models are good at predicting hurricane paths and their potential maximum intensity, but significantly less so at predicting hurricane 2 2 3 4 ry.com ww.theactua ry. www theactua r.com heactu y heactuary.co m www.theactuary.cowww.theactuary.c ency. My focus is alysis of the nu mber n es in the North 50, although bear be r of hurricanes i s y factors of hurric ane g insurance losses . urri cane number e a vailable data fro m databa se of North an es, maintained al O ceanic and Admin istration to nua l number of North can es that reached hig her (>180 km/hour ) t bef ore they dissipat ed. l. ( Journal of Climat e ( , atego rise hurricanes ps ac cording to al ch aracteristics. plays analysi s for two aining some of the most major h urricanes. Group es star t near Africa and rgy as they travel acros s c; abo ut one in five mak e the U S east coast, aro und nd the C arolinas. Group B s have s traighter paths a nd tronger , with approximat ely o hittin g Florida or Gulf of ates. 2 shows the trend against l histor ical number and number of major hurricanes 50. For b oth groups we see an ng trend and higher KEY: Actual number of hurricanes Tr e nd (smoothe d spline) Average over the per iod K EY: Actual number of hur ricanes Trend (smoothed spline) Average over t h e period Florida and Gulf of Mexico gr oup numbers of major hurricanes, actual and modelled Year 180 160 140 120 100 80 60 40 20 0 Dimitris Papachristou examines whether North Atlantic hurricane clustering is a new reality FIGURE 2: Mean and variance of number of major hurricanes higher during the p a st 30 years. The number of major hurricanes in t h e Atlan t ic Basin has been increasing, and become more volatile. This is a different dataset f rom Figure 1 (whic h foc u sed on costliest hurricanes) since here we consider hurricane s wit h a wind speed of more than 180km/h Annual cost (billions US$) 195 0 1952 1954 1956 1958 196 0 1962 1964 1966 1968 1970 1972 1974 1 976 1978 1980 1982 1984 1986 198 8 19 9 0 1992 1994 1996 1998 2000 2002 2004 2006 2008 20 1 0 2012 2014 201 6 Number o f hurricanes Year 6 5 4 3 2 1 0 1950 1953 1956 1959 1962 1965 1968 1971 19 74 1977 1980 1983 1986 1989 1992 1995 1998 2001 2004 2007 201 0 2013 2016 Year 6 5 4 3 2 1 0 195 0 1953 1956 1959 1962 1965 1968 1971 1974 1977 1980 1983 1986 1989 1992 1995 1998 2001 2004 2 007 2010 2013 2016 Carolinas group number of major hurricanes, actual and modelled Number of hurricanes KEY: Annual loss Ave rage over the period Trend (smoothed spline) n Number of hurricanes if n>1 online about news st on www.the esis and frequ gen e the statistical ath na of major hurricano Atlantic since 19 5 in mind the numb only one of many activity affecting Expected hur I used publicly a the HURDAT d Atlantic hurrica by the Nationa Atmospheric A analyse the ann Atlantic hurric category 3 or h at some point Kossin et al June 2010) ca in four group climatologic Figure 2 disp groups conta dangerous m A hurricane gather ener the Atlantic landfall on Georgia an hurricanes are thus st one in two Mexico sta Figure 2 the actual average n since 195 increasin t he kle, eals often e losse s ane rath er urricane s e 1 dispels s with hi gh strophic and 2012 nd Sandy re are also osses came ajor hurrica nes, d 2017. ien ticular ce, in par i gresse ng, has pro d ri ng the pas t 40 ng ts of this accounts be the book found in t s r Ker y Professor K ry Storm Surg e by dam So Atmospher ic bel. A good a icting t pred paths a heir potenti a nd th l m intensity but significa ntl , b y predic urricane ting h istou North IMAGE: IKONIMAGES www.theactuary.com www.th eactuary.com MAY 2020 | THE ACTUARY | 25 In-depth Modelling L ife expectancy (LE) and its changes are crucial to the pensions and insurance industry, soc iety and government. Longevity-trend projections are used to manage longevity risk in pricing and reserving for insurance and annuity products, as well as for costi n g public and private pensions. Changes in mortality projections directly affect annuities costs, especially in the decreasing interest rates environm ent. LE is also a consideration for individu als planning their fin ancial goals and retirement strategies. P rev i ously, a vast majority of people would buy a lifetime annuity with their p ension pot. However, the introduction of pension freedoms in 2015 resulted in the emergence of a variety of flexible retirement options, from drawdown to fixed-term annuities. This has necessitated dynamic decision-making both by individuals and companies. Many financial tasks, from underwriting to retirement planning, need to account for a large number of important and time-varying determinants of health and longevity, such as socio-economic factors (income or residence), health status (new conditions and/or prescriptions) and lifestyle factors (smoking, obesity, alcohol usage), as well as their interactions. In addition, they must take into account the medical advances and public health interventions that are aimed at increasing the health of populations. Our research, funded by the Actuarial Research Centre, concentrates on dynamic statistical modelling of population-based individual-level data, collected over the long term and recorded in electronic health records (EHRs). Dynamic statistical analysis allows us to make more accurate predictions, based on real-time data, dynamic risk 24 | THE ACTUARY | MAY 2020 FI G URE 1: Hazard rat io of all-cause mortality associated with statin prescription compared to no prescription, for two birth cohorts of 1930-3 5 and 1936-40, from the landmark analysis with 30-year window. Results were adjusted for cardiac risk at three levels, sex, birth cohort, Townsend d e priva tion quintile, chronic kidney disease, diabetes, treated hypertensi o n, hypercholesterolemia, aspirin, BMI, al c ohol use, smoking status a nd g enera l practice. stratification and more personali s ed service. T raditional statistical methods use the baseline data for long-term outcome predictions (often beyond the study’s range). For instance, a person’s characteristics at retiremen t age are used to infer their hypothetical longevity. Dynamic analysis allo ws for changing personal circumstances and age-dependent changing risks, and incorporates them into more precise modelling. The age-varying survival benefits or harms of various predictors o btained from dynamic statistical modelling of big health data can be used to ob tain finely differ entiated projections of life expectancy for numerous subpopulations. We provide a case study on statins. Cox regression and landmark analysis Survival analysis, or time-to-event analysis, investigates the effects of various risk factors on mortality. The type of reg ression model typically used in survival a nalysis in medicine is the Cox pr oportional hazards regression. The Cox model fact orises the hazard or force of mortality Ɋ i ( x ) for a subject i with risk factors Z i at age x into an age-varying baseline hazard Ɋ 0 ( x ) and a constant subject-specific risk score. The risk score is a log-linear regression term which includes relevant risk factors, so that Ɋ i ( x , Ⱦ , Z i ) = Ɋ 0 ( x ) e Z i Ⱦ Taking a ratio of the hazard functions for two subjects, the hazard ratio Ɋ ( x , Ⱦ , Z ) = Ɋ i ( x , Ⱦ , Z i ) Ɋ j ( x , Ⱦ , Z j ) = e Ⱦ ( Z i - Z j ) is constant over time and does not depend on the baseline hazard. This proportional hazards assumption, if true, allows to easily estimate hazard ratios for various combinations of risk factors, regardless of the parametric form of the baseline hazards. A FINE SCALE The ARC is the IFoA’s global network of actuarial rese archers. The ARC delivers industry-relevant, cutting edge research prog r ammes that address significant challenges i n act ua rial science by working collabora t ively with academics, practiti oners, industry and other actuarial bodies. For more info visit actuaries.org.uk/arc KEY: Unadjusted landmark model Adjusted model i n patients born 1930-35 Adjusted mod e l in patients born 1936-40 Elena Kulinskaya , Ilyas Bakbergenuly and Lisanne Gitsels revisit longevity modelling 1.5 1 .4 1.3 1.2 1.1 1.0 0.9 0.8 0.7 0.6 0.5 60 65 70 75 80 85 Hazard ratio Age Atlantic i c a hur r n e clusteri ng is angis a r new Dimitris hr c Pa pa i examines r h e wh e t N hur r ic e L its changes are crucial to the pensions and insurance industry, soc iety and government. Longevity-trend projections are u sed to manage longevity risk in pricing and reserving for d annuity products, as ting public and private nges in mortality ectly affect annuities in the decreasing ironment. ideration for g their fin ancial t strategies. jority of people nnuity with wever, the n freedoms in rgence of a ent options, erm tated oth by nnin g, ber of vity, s pres wind quint al c oh S a va 1 .4 1.3 1.2 1.1 1.0 0.9 0.8 0.7 0.6 0.5 60 NE SCALE IMAGE: SHUTTERSTOCK www.theactuary.com www.theactuary.com JUNE 2020 | THE ACTUARY | 25 In-depth Modelling A s advanced modelling methodologies become widely available to actuaries, the way models are used within financial services is increasingly constrained by legal developments and regulatory scrutiny. Two examples in the UK are the Financial Conduct Authority’s review of general insurance pricing practices and the Information Commissioner’s Office consultation on an AI auditing framework. A more longstanding and familiar regulation is the EU gender discrimination directive, which requires that pricing models do not discriminate by gender. The risks of inadvertent discrimination with respect to protected characteristics seem to be higher in complex models than in simple ones, as complex models may exploit intricate patterns in data to derive proxies for, say, gender. In addition to the legal and regulatory risks, ethical concerns could arise if models were found to be using unacceptable proxies. Defining discrimination in such an intuitive way may appear straightforward, but without a FIGURE 1 Portfolio propo rtions – distribution of gender acros s age classes and population aver age prices’. We can see that, as age increases, claims costs for females and males diverge – in particular, claims costs for females become progressively higher. The question is: how should insurance be priced? A common method for avoiding discrimination is simply ignoring gender. Then, the insurance rate for a policyholder of any gender at age 50 is nothing but the average cost of the corresponding age class. We call a price calculated in this way an ‘unawareness price’, shown by the black line in Figure 2 . It is striking that the unawareness price is very close to the best-estimate price for women at lower ages, and then drops to nearly the best-estimate price for men at higher ages. This is due to the much higher prevalence of women within the lower age classes (90%), as we saw in Figure 1 . The unawareness price uses age as a proxy for gender – to be precise, the calculation of unawareness prices implicitly relies on the conditional probability of gender, given age. In summary, ignoring gender in price calculation did not remove its impact on prices. This is indirect discrimination. What should the price be for, say, a policyholder aged 50? We know that gender must somehow be allowed for in the calculation, since ignoring it leads to indirect discrimination. Furthermore, prices should lie somewhere between the extremes given by the grey and dark yellow lines in Figure 2 ; in particular, the price at age 50 should be a weighted average of the corresponding F IGURE 2 Different types of insurance prices rigorous definition of discrimination, it becomes difficult to guarantee that pricing models are free of it. How can we make sure that illegal or unwanted discriminatory factors are not influencing the results of a model? Our recent research paper ‘Discrimination-Free Insurance Pricing’ ( bit.ly/2KLG5CK ) proposes an approach to ensuring that the results of actuarial models are not influenced by protected characteristics. This proposed discrimination-free pricing method is a simple add-on to existing pricing methodologies and does not require major changes to insurers’ predictive models. It can remove discriminatory effects from all categories of pricing techniques currently in use, from generalised linear models (GLMs) to gradient boosting machines and deep neural networks. Method We start by taking it as given that protected characteristics such as gender are not used within pricing models as rating factors – meaning direct discrimination is avoided. What do we mean, then, when we say that a price may still be discriminatory? We illustrate our ideas with a simple stylised example; a full mathematical definition of discrimination in pricing can be found in our paper. We consider the case of a simple pricing model for a health insurance portfolio. The two relevant covariates are the policyholder’s gender and age class. The portfolio population is split 50/50 between women and men – shown in Figure 1 , together with the split across ages. In this example, 90% of policyholders in the younger age classes are female, with the reverse happening for older age classes. The expected claims costs by age class and gender are shown by the grey and dark yellow lines in Figure 2 ; we can view these as ‘best-estimate Age Age 100 % 90% 80% 70% 60% 50% 40% 30% 20% 10% 0% 120 115 110 105 100 95 90 85 80 Percentages of females in portfolio Insurance price KEY: P(female | age) Population average KEY: Best-estima te (fema les) Best-es timate (males) Unawareness price Discrimination-free prices 30 30 50 50 70 70 90 90 0 0 If we want to avoid discrimination in insurance pricing, we need to be able to take protected characteristics into account, say Mathias Lindholm , Ronald Richman , Andreas Tsanakas and Mario Wüthrich THE PROXY PROBLEM Have your say
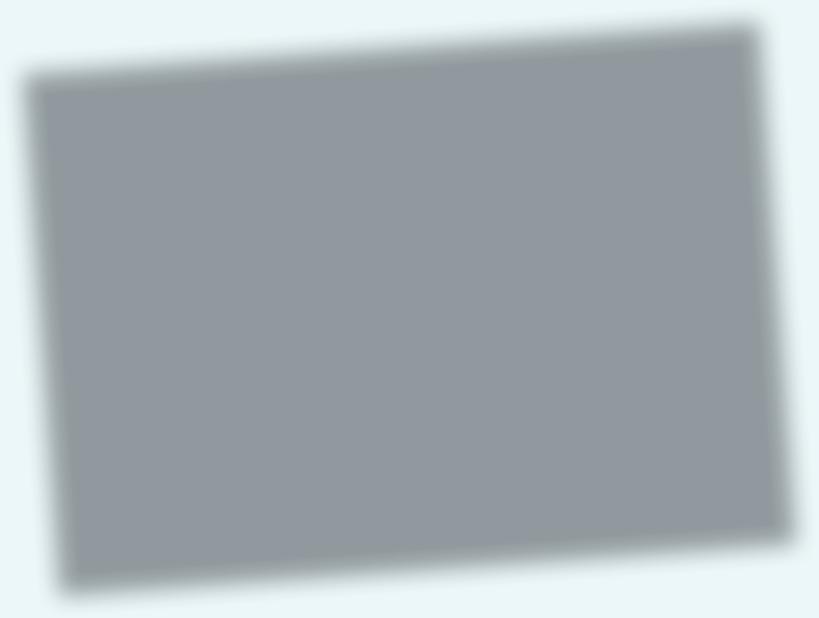
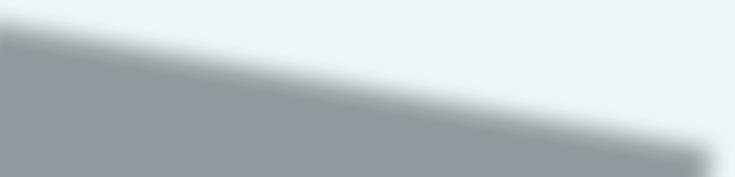
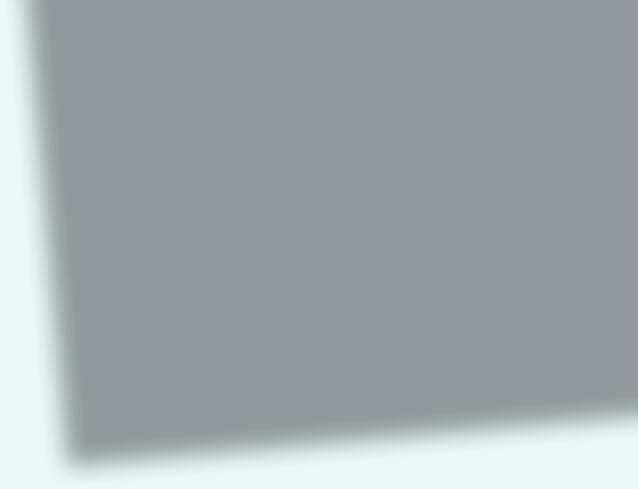
Technical excellence
I have noticed the in-depth actuarial modelling section in The Actuary each month and I wanted to write and say I have appreciated these articles. I like the level of technical detail (including formulae!) and that they give enough background for a practitioner to understand many of the issues.
There have been a lot of impressive moves by actuaries in the last decade to improve our (often lacking) softer skills, but in an increasingly competitive fi eld we also need to maintain our technical excellence. Other professions such as quants, data scientists and statisticians cover overlapping areas and these professions have highly technical backgrounds which we need to keep up to speed with. Regular in-depth technical modelling articles from The Actuary play a small part in helping us do this.
Thank you!
JAMES SHARPE FIA
21 MAY 2020
Taboo busting
What a courageous Immediate Past President we have. Many readers of the June edition of The Actuary have been amazed by the candour of John Taylor’s article ‘Behind the curtain’, in which John refl ects on his mental health experiences.
I’m a past President of the IFoA. It is no secret, but probably not widely known, that my husband, a much loved and widely admired doctor (GP), died by suicide.
We don’t go around with Post-it Notes on our foreheads: “I’ve a history of depression”; “My husband died by suicide”, etc.
Mental health and suicide are still taboo. What I know is that many of us are directly aff ected by mental wellbeing. I so admire John for his incredibly candid article about his experience.
Thank you, John. You will have helped many other people by being so open and are helping to shatter the taboo.
FIONA MORRISON
23 JUNE 2020
INSIDE
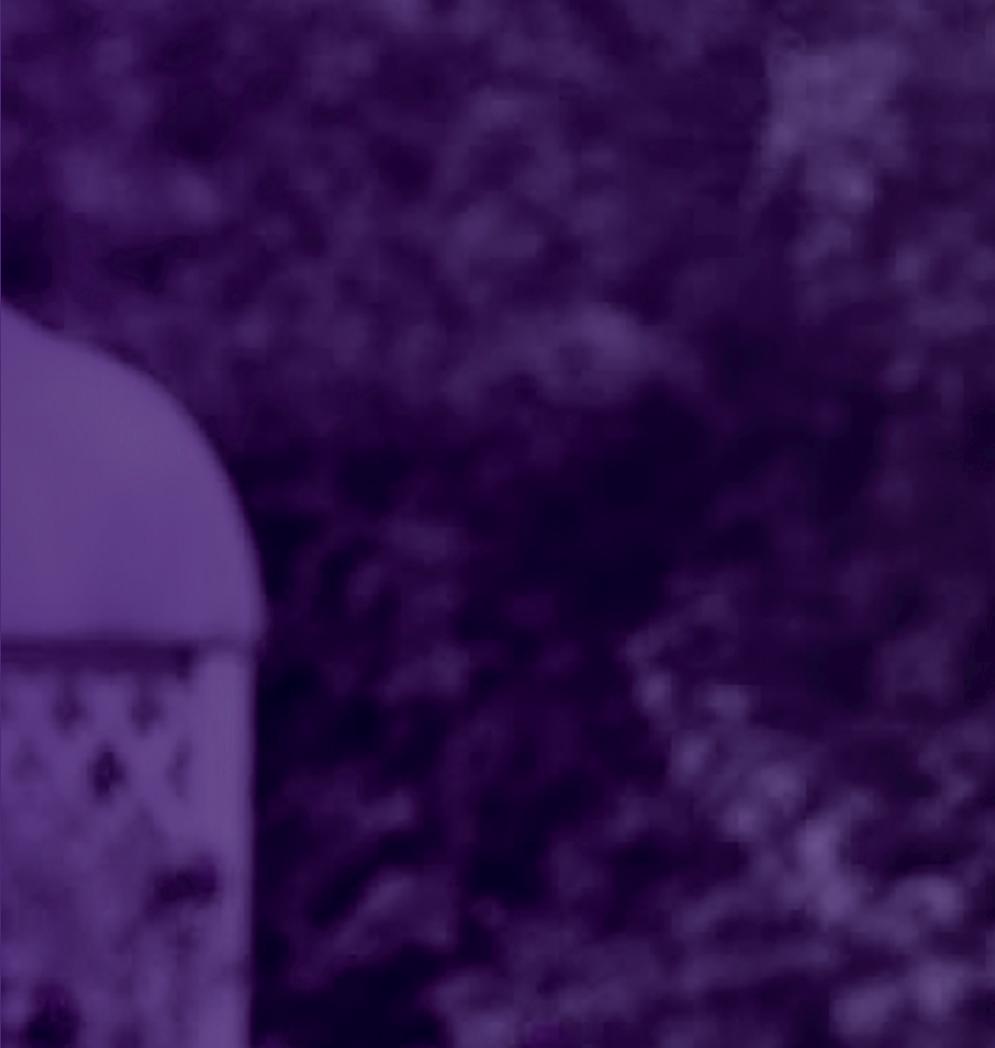
Thinking beyond the box
The former Bank of England governor on quantifying risk
Decoding disease
How could genetic research impact actuaries? Peter Joshi explains
Lonely hearts
Olivier Menoukeu Pamen, Corina Constantinescu and Kira Henshaw discuss coupled life dependence
Smoke signals
What is the risk profi le of e-cigarette users? Niel Daniels investigates
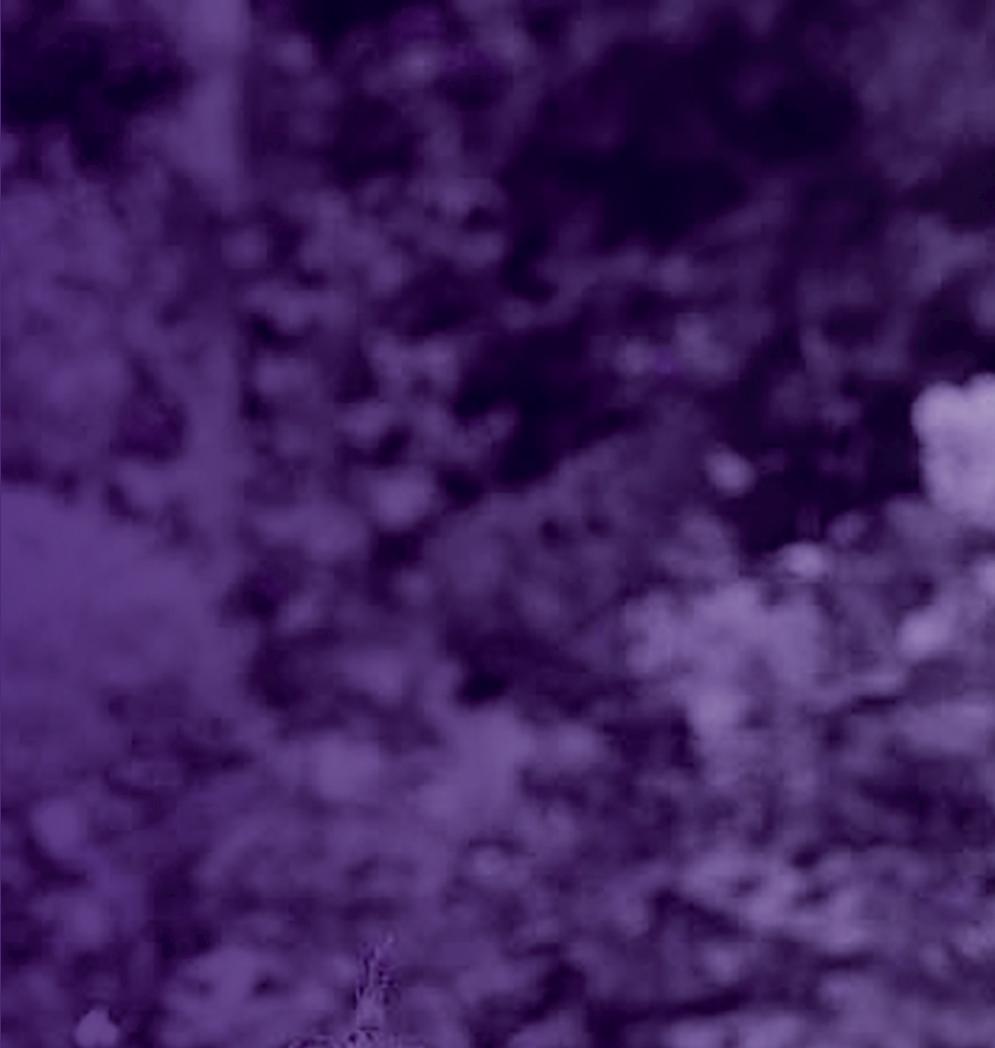
The deep end
How neural networks could be used in mortality forecasting
Protecting generation rent
Siobhan Lough and Richard Purcell on protection policies among renters
New insights
Technology is advancing DFID’s work, say Tim Harris and Jacob Wilcock
Infl ated worth
Jonathan Camfi eld refl ects on the implications of the RPI reforms
Time to settle up?
Prepare for liability regimes, advise Quintin Rayer and Karsten Haustein
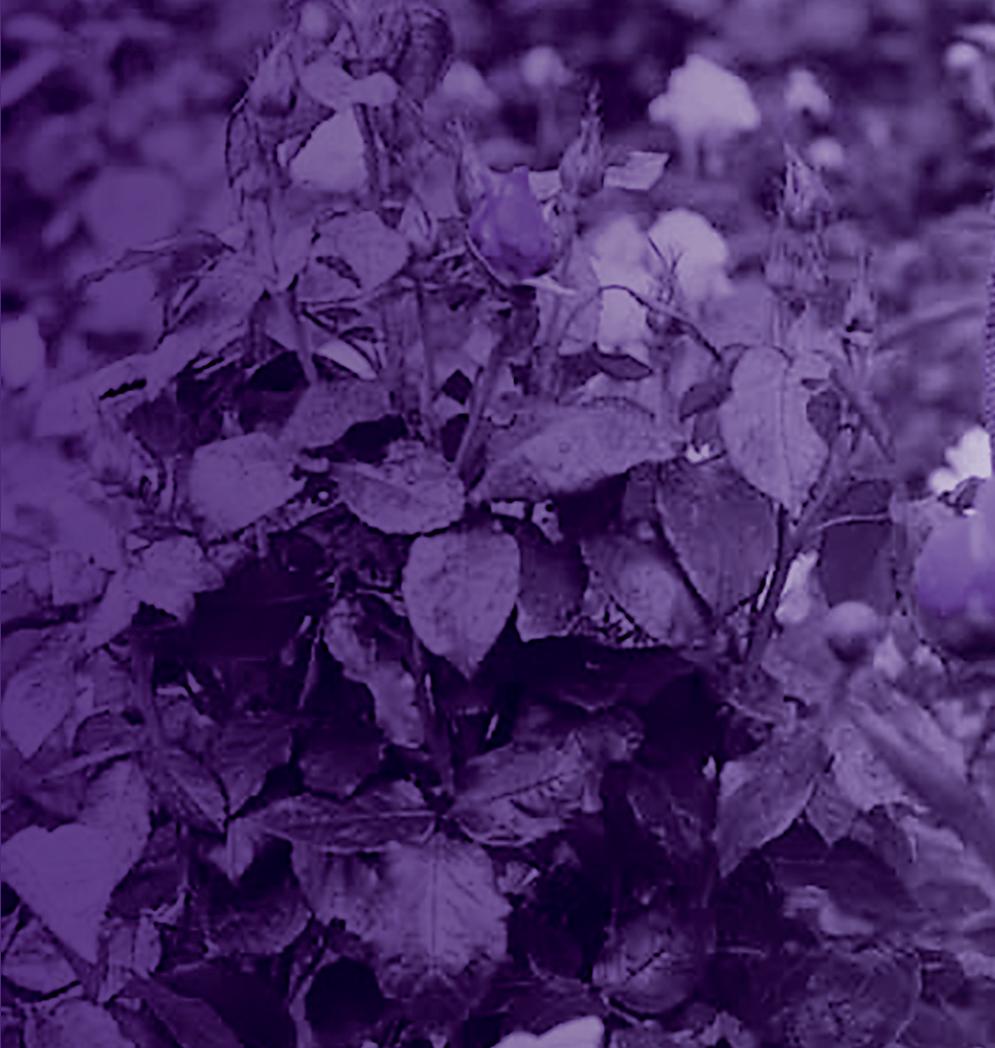
Back to nature
John Carstensen on nature-based climate solutions

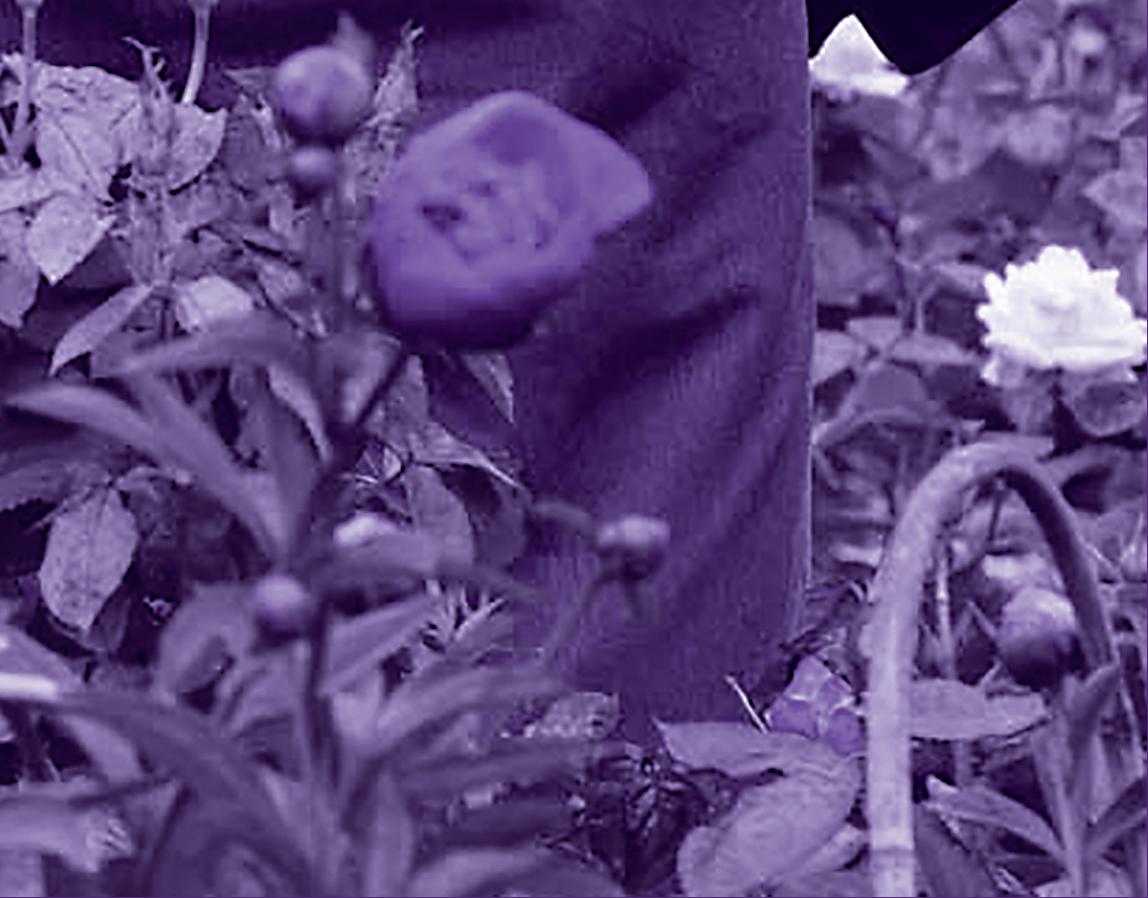
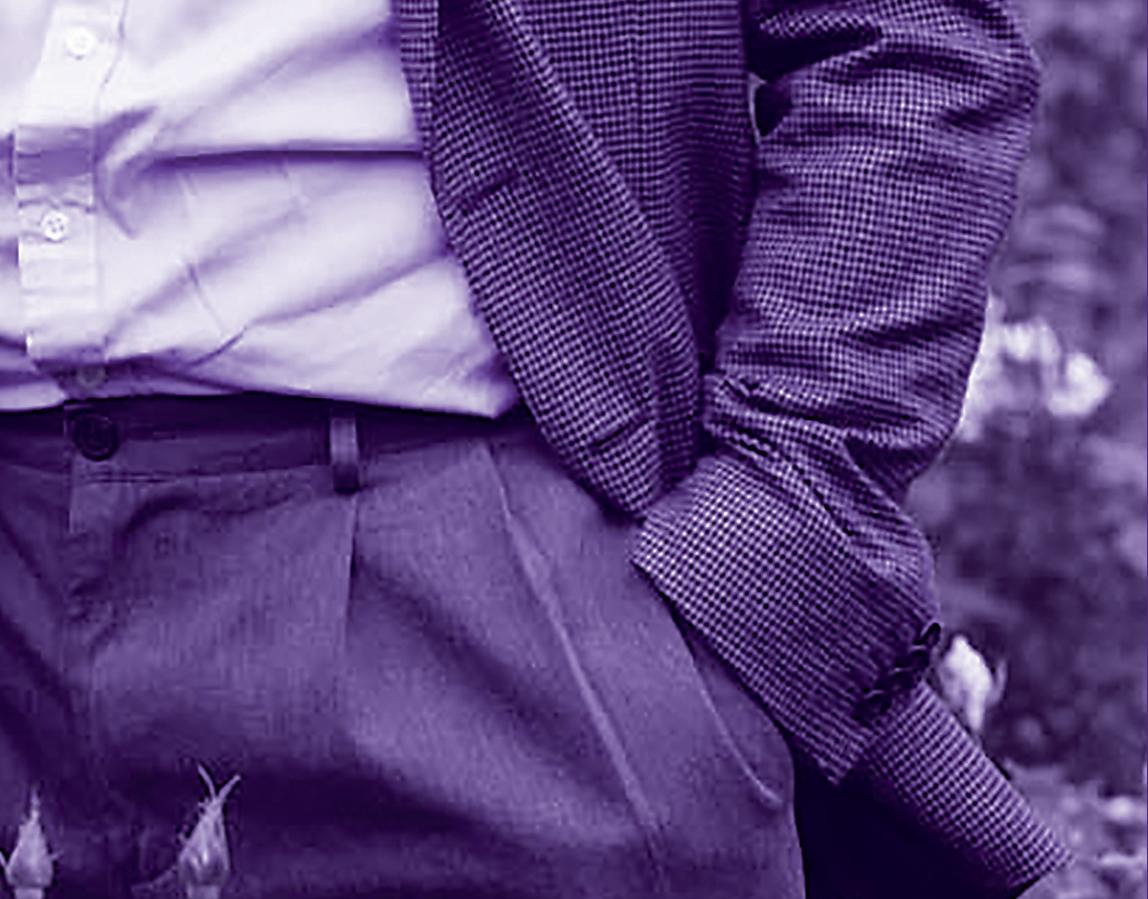
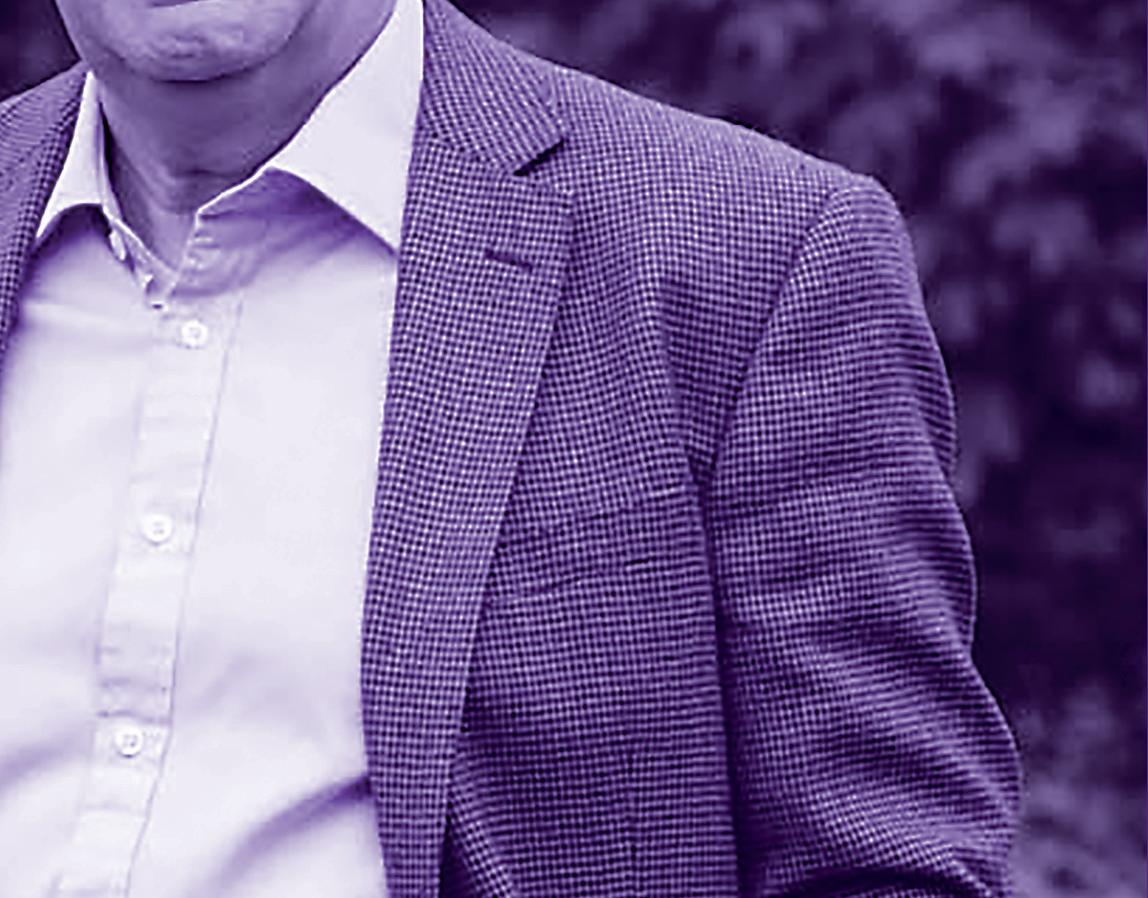
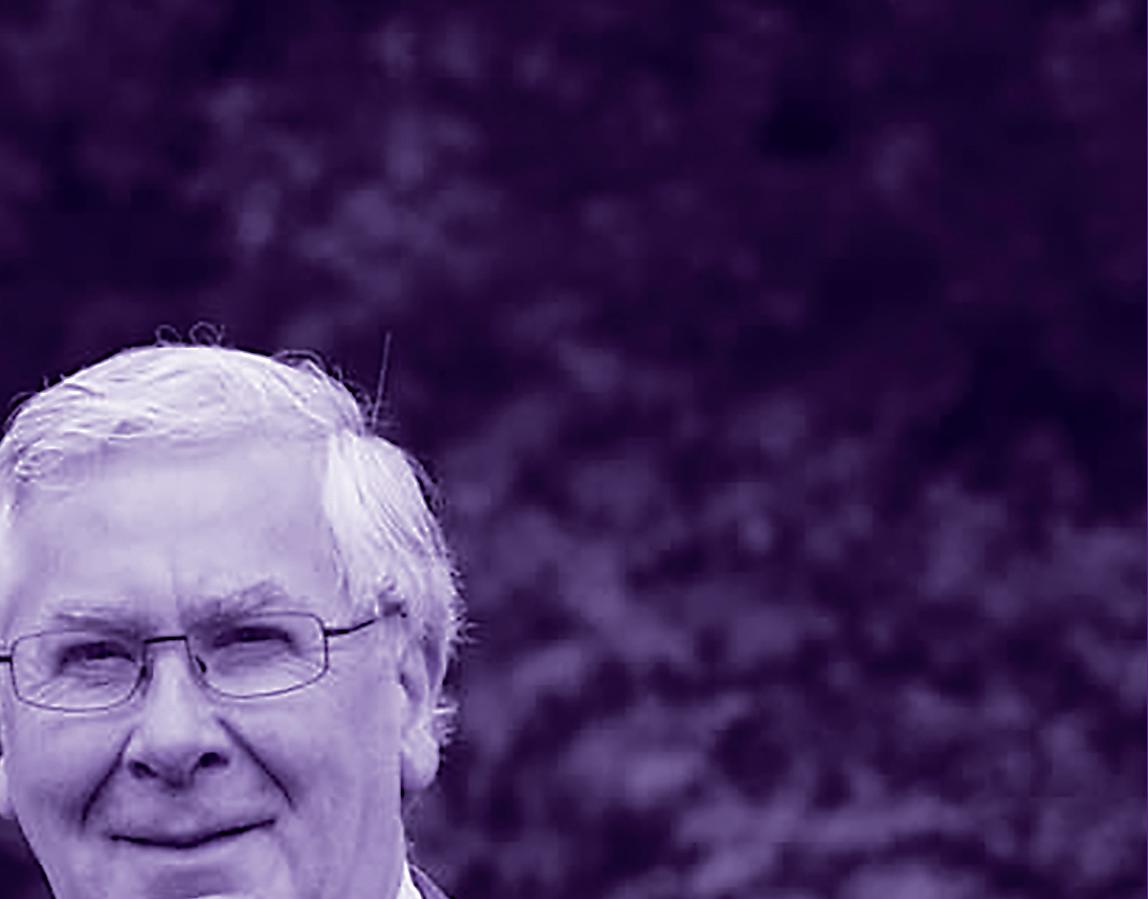