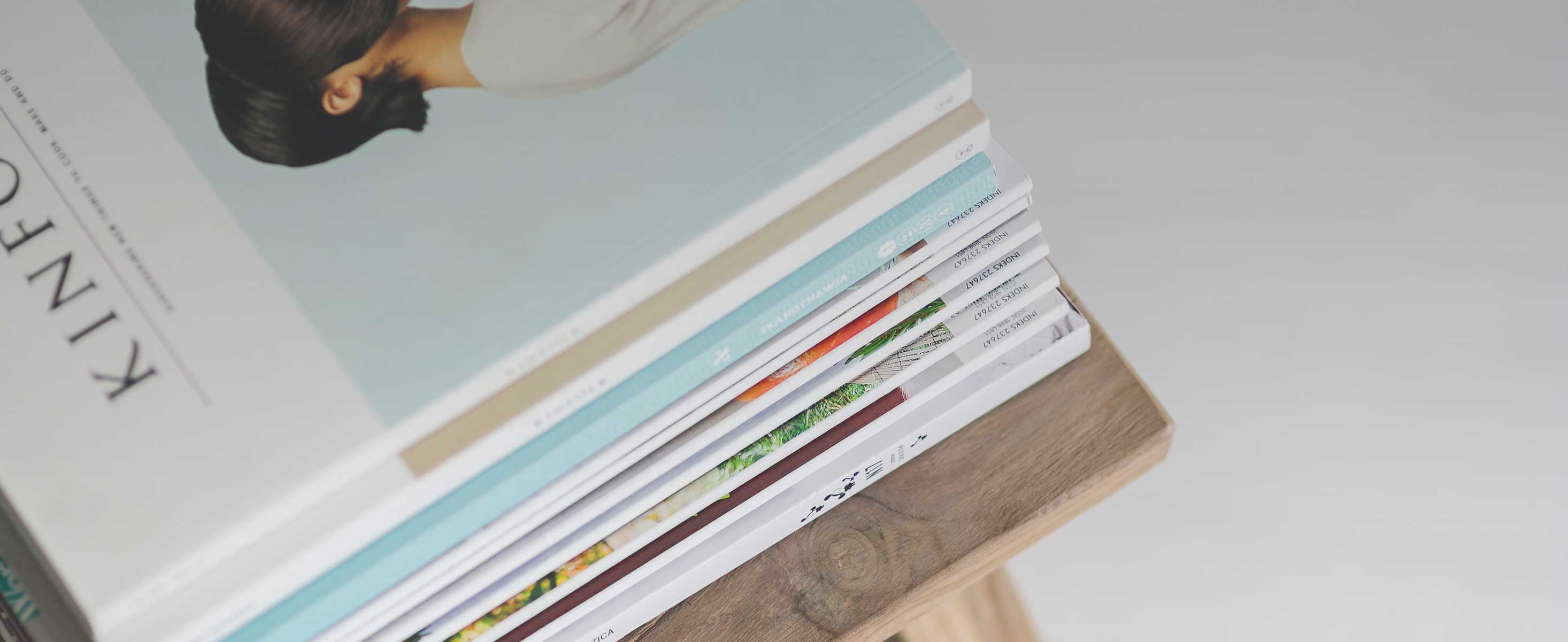
5 minute read
DATA NORMALIZATION
from Pt 1/2: It's What Makes a House a Homo: Queer and Trans Residential Geographies of Toronto
by samcasola
DATA COLLECTION
Data was first collected in order to analyze Toronto’s LGBTQ2S housing landscape within Professor Victor Perez-Amado’s research project, Aging Together: LGBTQ2S Housing. For the purpose of the research project and this research, Data was collected from the topic Offering (a home) as each of these posts provides information about an available residential space within the City, following a template *(see appendix) that outlines details pertaining to the space. Required details include location & type of accommodations, rent cost, accessibility and queer safety, in addition to demographic information, the age, gender, sexuality and ethnicity of individuals that will be sharing the dwelling or are currently living in the dwelling (Homes for Queers Toronto, n.a.). Although some individuals may be leaving the space, the post provides information about LGBTQ2S+ individuals who have recently lived at the identified location.
Advertisement
Information from 115 Facebook posts, each corresponding with one available location of residence, was gathered. The posts were published over the span of nearly two years, between June 2020 and April 2022. It is important to note that the collected data does not comprise all posts made within the identified time frame as individuals are encouraged to remove their posts after housing has been filled. Given that one residence may be shared with existing tenants and any provided information about the tenant moving out was gathered for analysis, the data includes a total number of 213 individuals.
Available spatial data and respective qualitative data was collected in a spreadsheet organized into three categories that correspond with the research questions; Neighbourhood explores geographies of residence, Housing includes data related to dwelling type and living accommodations and Identity highlights demographics and relationships through living accommodations. Each category was sub-divided as follows:
● Entry Number
● Date Listed
● NEIGHBOURHOOD
Location (either identified as the closest intersection or address)
Neighbourhood
Explanation (of the location for rent or the neighbourhood) ● HOUSING
Building Type
Floor (identifying the floor on which the unit for rent is located)
Number of Bedrooms
Accomodations: (type of space for rent such as apartment unit, condo unit, entire house)
Shared? (Y/N, identifying whether the space is shared with other individuals)
Number of People (If shared, identifies the number of tenants desired + the number individuals currently living in the space)
Rent (lists the monthly rent to be paid by the new tenant )
IDENTITY & KINSHIP
Relation (identifies if individuals are noted as partners, siblings, etc.)
Gender
○ Pronouns
○ Sexuality
Age
Ethnicity/Culture/Race
Within the category Identity & Kinship, all data was input verbatim as individuals listed within the Facebook post. An supplemental section entitled ‘Themes’ was utilized to track additional information noted in the post which provided insight on the morals, values and kinship structures that exist and are desired in a new tenant. As data was collected, it was coded for thematic analysis within the spreadsheet. Themes were analyzed after completing the data collection and prior to mapping the remaining data. Recurring themes will be considered when analyzing the maps produced.
DATA NORMALIZATION
In order to produce maps that accurately represented the collected information, the data was normalized. The organization methods used aimed to account for multiple individuals living within one dwelling while ensuring that dwellings were not included more than once on a map. The data was first divided by individual whereas each individual included in the data was represented in their own row. Due to cohabitation, the organization caused duplications of data within the Neighbourhood and Housing categories. Each individual/row of data was then given an ID in order to separate the information into two spreadsheets for mapping. ID’s were provided for each row using a number and a letter, each number represented a different dwelling while the letter represented the individuals who lived in the dwelling.
Data was then separated into two spreadsheets titled Dwelling and Demographics. The Dwelling spreadsheet included information from the categories Neighbourhood and Housing, which was used to map dwelling type, accomodations and location data. Within these categories, all rows including the letter ‘A’ remained while the others were removed. Given that all other letters represented more than one individual living in the same dwelling, removing all rows but those with ‘A’ ensured that dwellings, with the associated location building information, were not included more than once within the map. No information was included from the Identity & Kinship category.
The Demographics spreadsheet included information from the categories Neighbourhood and Identity & Kinship, which was used to map demographic data in relation to dwelling location. This spreadsheet included all rows within these categories to account for multiple individuals living within one dwelling. No information was included from the Housing category.
Information was then formatted for uniformity within each of the categories in order to be geocoded within ArcGIS Pro. Where information was unavailable, the data was formatted as ‘unspecified’ if needed to be visible in GIS and ‘NA’ if it was not necessary to include in analysis. Further, the ‘Themes’ section within the Identity & Kinship category was used for thematic analysis prior to mapping, therefore it was not needed for mapping and was not included in the data to be geocoded. Sections normalized and included for geocoding are indicated below: NEIGHBOURHOOD
Section not included for mapping is ‘Explanation’. This section was used to identify the location and building type of the available space.
○ Location: Intersection wording was altered to list all intersections as ‘street name and suffix abbreviation & street name and suffix abbreviation”. Building address remained unchanged.
Neighbourhood: Neighbourhoods were included for posts which listed them. Those that were not mentioned in posts were left as n/a and were generated through geocoding.
City: The city for all posts was listed as ‘Toronto, ON’ and was included for the purpose of geocoding.
HOUSING
One section was not included in the mapping; ‘Floor’. This section aided in identifying the accommodations/ types of space for rent.
○ Building Type: Building types were categorized as house, mixed-use, mid-rise, apartment and condo based on information from the ‘Explanation’ and ‘Floors’ sections
○ Number of Bedrooms: Numbers identified the number of bedrooms within the unit for rent. This section did not need to be normalized.
Accomodations: Types of space for rent were categorized as apartment, house, and condo unit. This section identifies the specific space within the building type that is available to inhabit.
Shared: Y or N was used to indicate whether the space for inhabitation was to be shared with other individuals. This section did not need to be normalized.
○ Number of People: The collected number of desired tenants and number of individuals currently living in the space were added together. Numbers indicate the number of people sharing the accommodations.