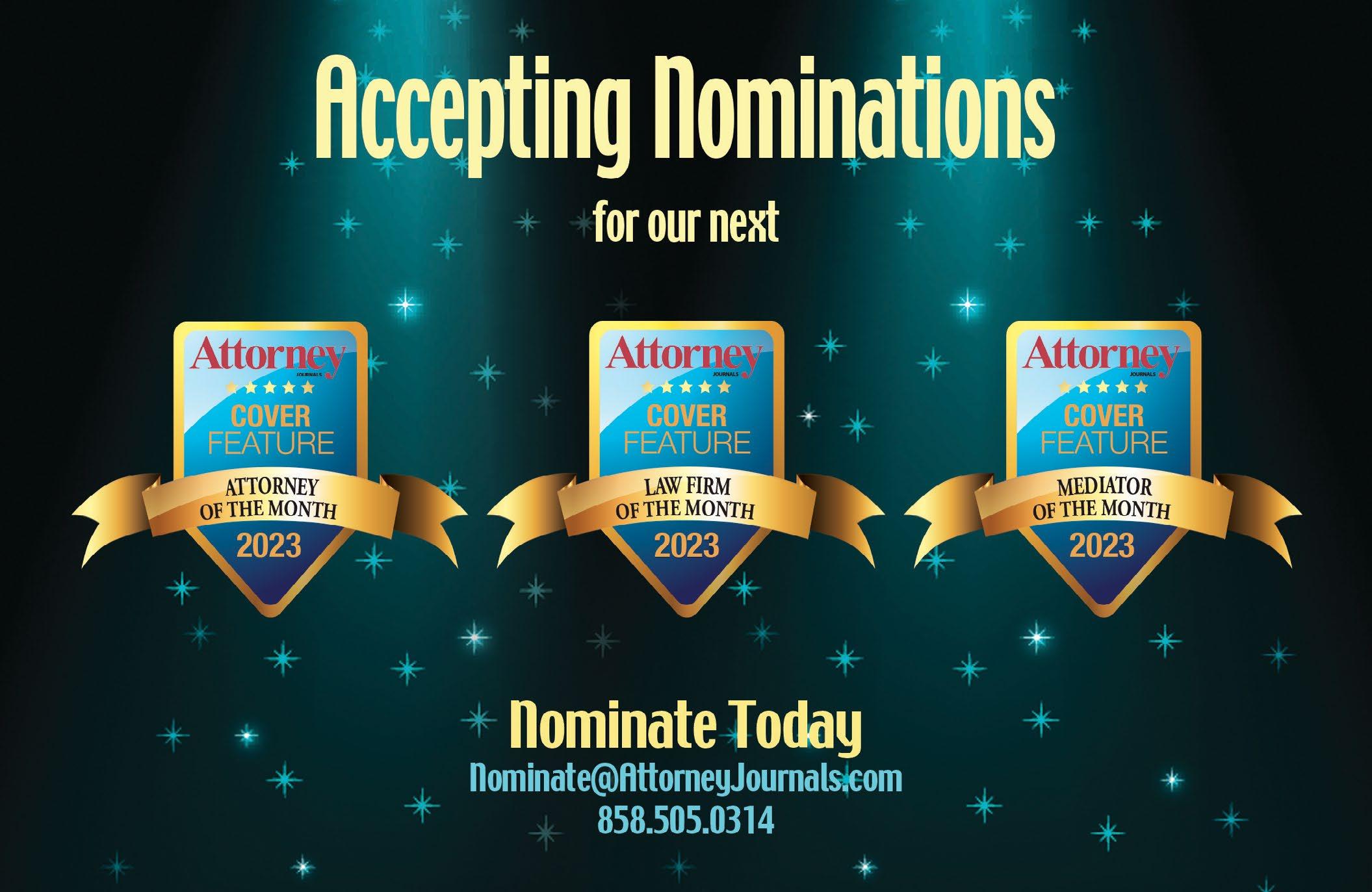
1 minute read
6 Common Data Quality Management Issues and How to
Solve Them
by Chris Fritsch
3. Incomplete or Missing Data
Incomplete or missing data can hinder your ability to gain valuable insights and make informed decisions. It is crucial to establish data capture protocols that ensure all required fields are consistently populated. Automated data validation checks can help identify missing data during entry, while periodic data audits can address incomplete records. Additionally, incentivizing employees to maintain data completeness and conducting regular data hygiene practices will contribute to better data quality.
4. Lack of Data Governance
Without proper data governance, organizations may face data quality nightmares due to inconsistent standards, lack of accountability and poor data management practices. Establishing a data governance framework that includes clear ownership, defined roles and responsibilities and standardized data policies is crucial. Regular data governance reviews, data quality assessments and ongoing training and communication about data governance practices will ensure a strong foundation for data quality management.
5. Data Integration Challenges
When data is scattered across multiple systems or departments, data integration becomes a significant challenge. Disparate data sources, incompatible formats, and inadequate integration processes can lead to data inconsistencies and inaccuracies. Implementing a robust data integration strategy, utilizing appropriate integration tools, and establishing data transformation rules can help streamline data integration processes. Regular data reconciliation and validation checks will ensure data integrity is maintained throughout the integration process.
6. Lack of Data Quality Monitoring
Data quality is not a one-time task but an ongoing process. Without regular monitoring and measurement, it is difficult to identify and rectify data quality issues. Implement data quality monitoring mechanisms, such as automated alerts, reports, and dashboards, to proactively identify anomalies or deviations from established quality standards. Conduct periodic data quality assessments and establish data quality metrics to measure and track progress over time.
Effective data management is the key to avoiding data quality issues that can hinder your business success.
By implementing the strategies outlined in this article— addressing inconsistent data entry, managing duplicate records, ensuring data completeness, establishing data governance, tackling data integration challenges, and implementing data quality monitoring—you can maintain high-quality data that drives accurate analysis, informed decision-making, and operational excellence.
Keep in mind, prioritizing data quality is an investment that pays off in improved efficiency, better client experiences and a competitive edge in today’s data-centric business landscape. n
As a CRM Success and Business Development Technology Consultant, Chris Fritsch works together with leading professional services firms across the country to help them select and implement the right Client Relationship Management and eMarketing solutions to support their marketing and business development efforts and maximize value and return on investment. Chris frequently writes and speaks on legal marketing, technology and business development topics including CRM, email marketing, data quality and competitive intelligence. She is also the author of a leading CRM blog at CRMSuccess.net.