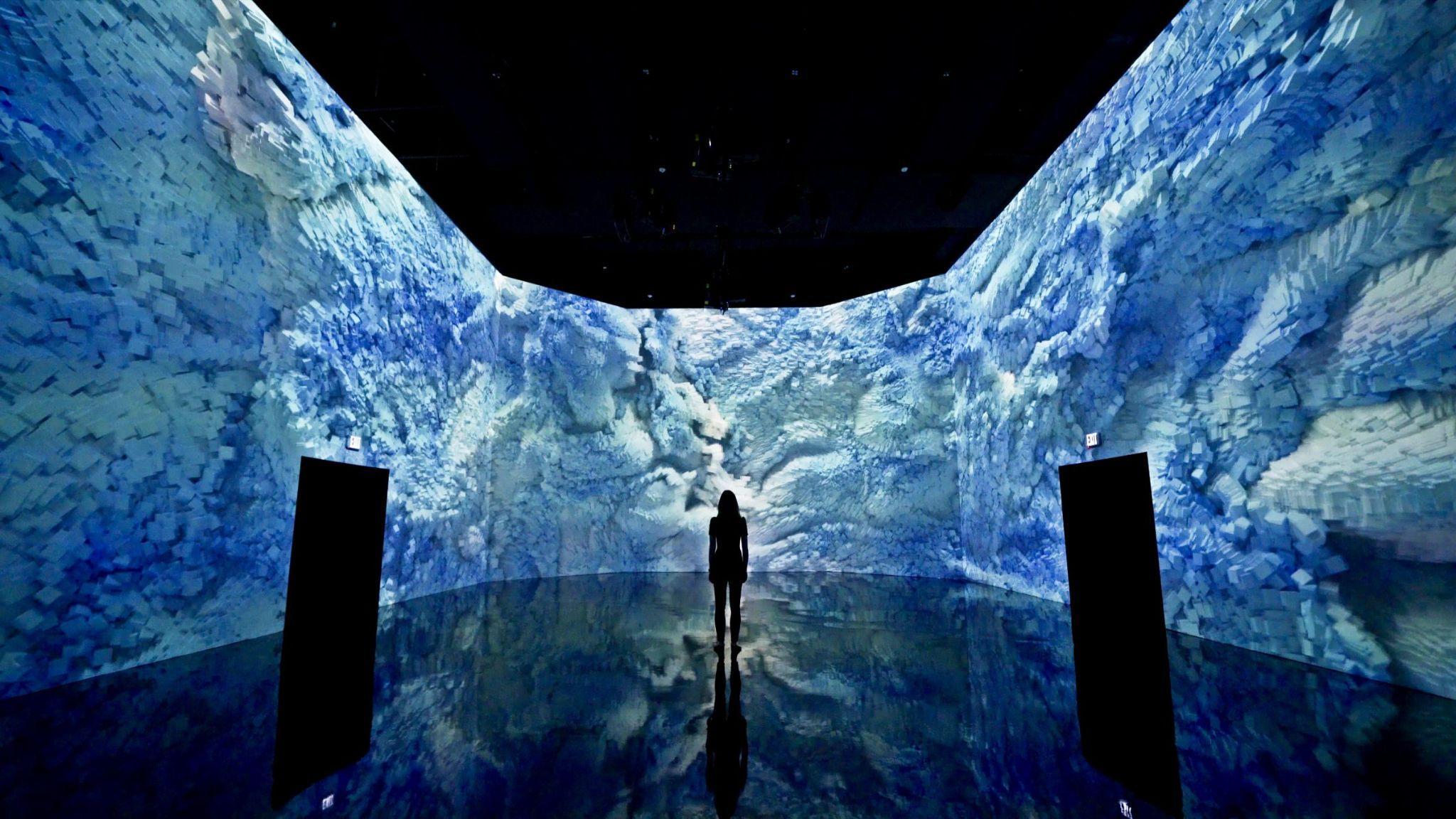
6 minute read
Applications
Art - Rafik Anadol
Rafik Anadol creates multi-sensory art installations by using data sets as fodder and processing them into a machine, specifically machine learning algorithms, to sort and filter the data to be visualised. The data is visualised in mesmerising fluid shapes14 . These “data sculptures” are embedded into architecture to make the building “process memories, dream and hallucinate” . The following is called Bosphorous. The description provided by Rafik Anadol is as follows “A data sculpture that explores the rhythms of Marmara Sea in Turkey to visualise the poetic experience of the organic movement. Inspired by high-frequency radar collections, taken in 30-minute intervals for 30 days, Bosphorus transforms long sea surface activity into a kinetic piece of art.
Advertisement
Figure 11. Image showing the installation “Bosphorous”15
14
15 TED. (2020, August 19). Art in the age of machine intelligence | Refik Anadol [Video]. YouTube.
Art in the age of machine intelligence | Refik Anadol Anadol, R. (2019, August 18). Infinite Space. Refik Anadol. Infinite Space
Neri Oxman is pioneering a field that promotes design opportunities that were once considered impossible. Her work operates at the intersection of technology and biology16 . The design process moves away from the assembly of different parts and implements a process where the objects are computationally “grown” , additively manufactured and biologically augmented to create heterogeneous and multifunctional constructs.
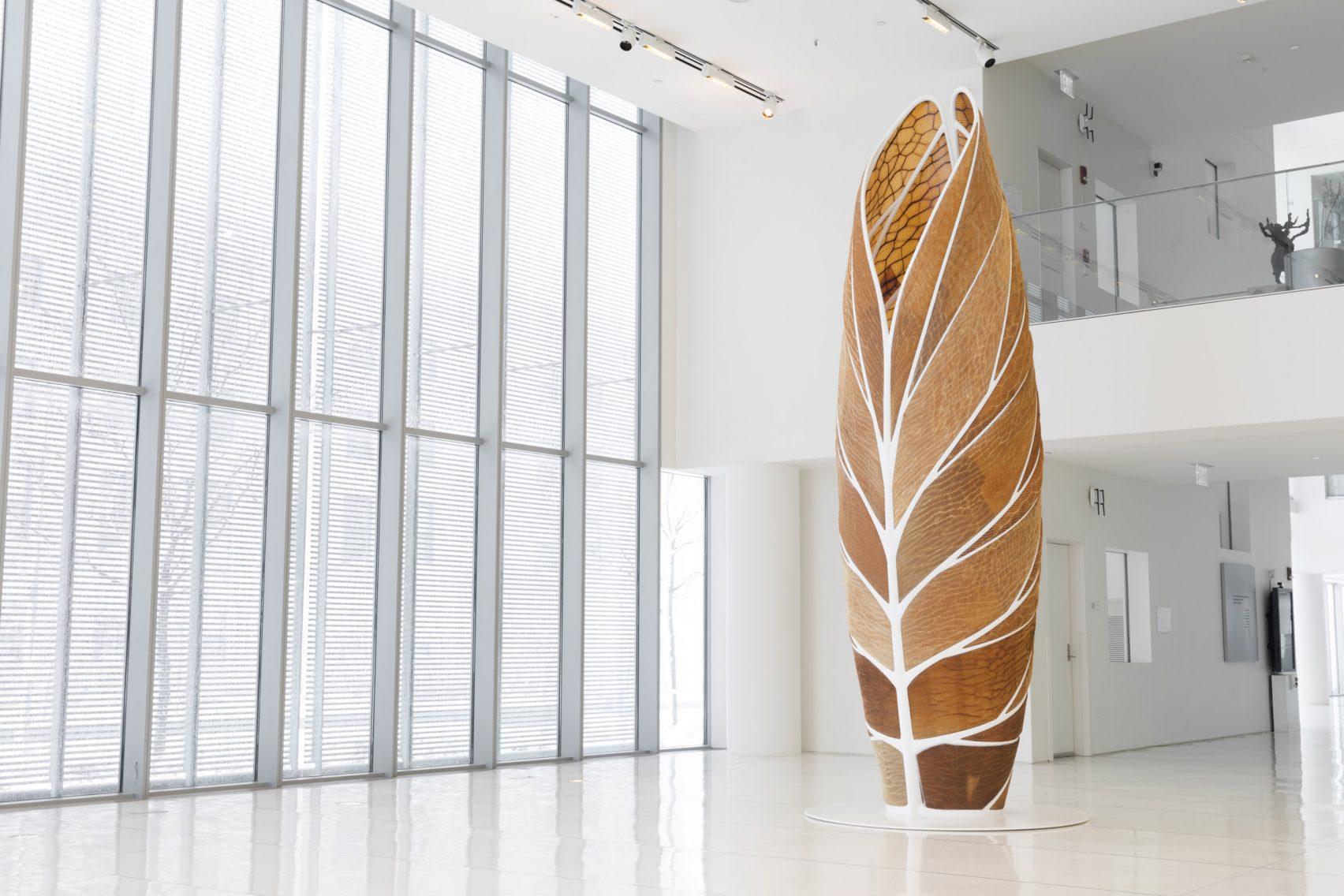
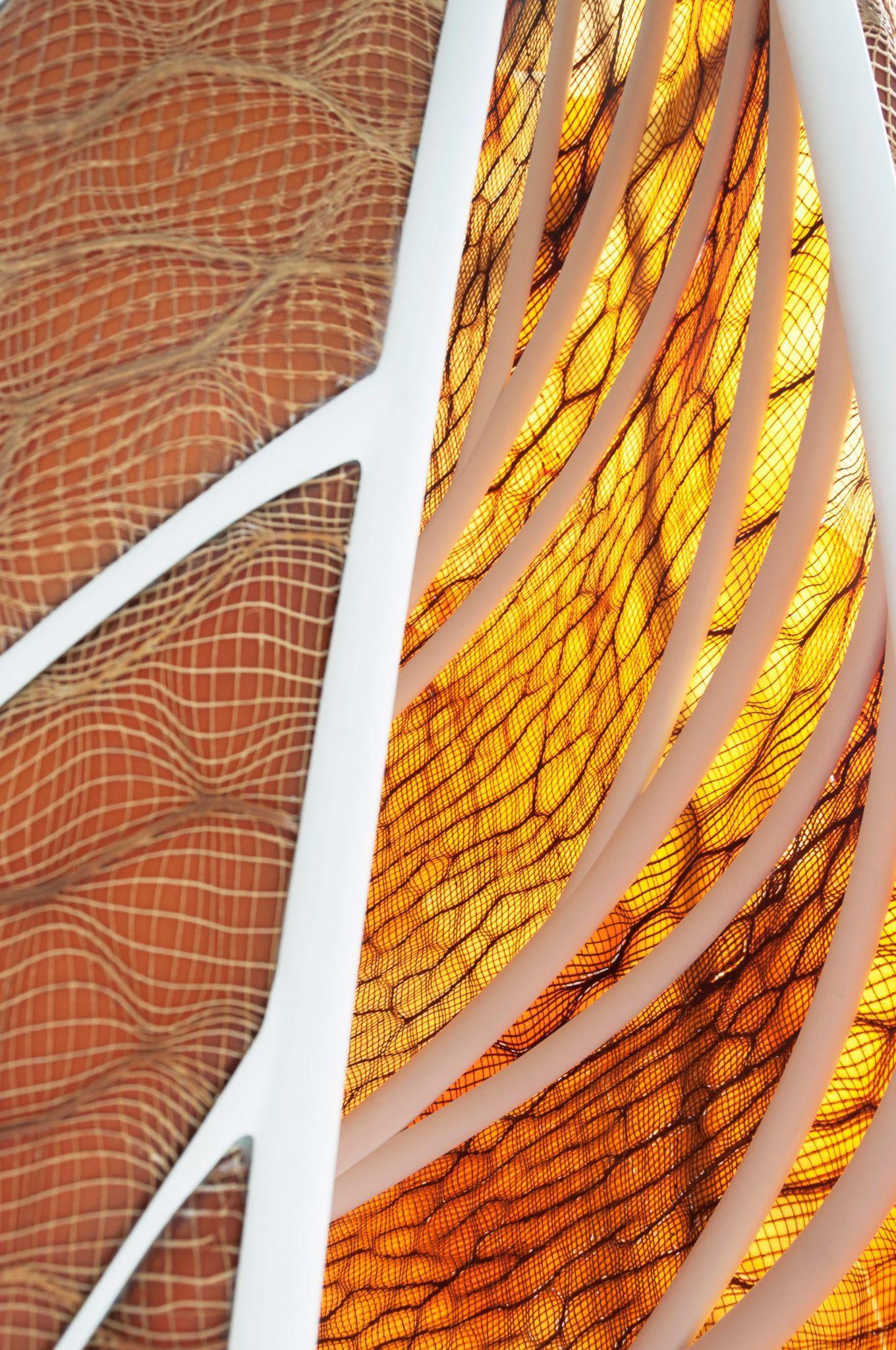
Figure 12. Images showing the installation “Aguahoja I.
Aguahoja I. is an installation digitally designed and robotically fabricated using Chitin. Chitin is a material that is found at a molecular level in trees, insect exoskeletons and bones. The structure examines how materials, one would usually consider waste, can inform design. According to the Mediated Matter Group, Aguahoja I is “Derived from organic matter, printed by a robot, and shaped by water, this work points towards a future where the grown, and the made unite”17
16
17 Neri Oxman: Material Ecology. (n.d.). Retrieved July 12, 2021, from Neri Oxman: Material Ecology Project Overview ‹ Aguahoja –. (2019). MIT Media Lab. Overview ‹ Aguahoja — MIT Media Lab
Architecture
Autodesk Office, Toronto18
The Autodesk office in Toronto is the first example of the application of the Generative Design optimization engine for Project Dynamo. The design team took under consideration qualitative aspects such as workstyle preference, adjacency preferences to create quantitative parameters which helped generate options in the layout.
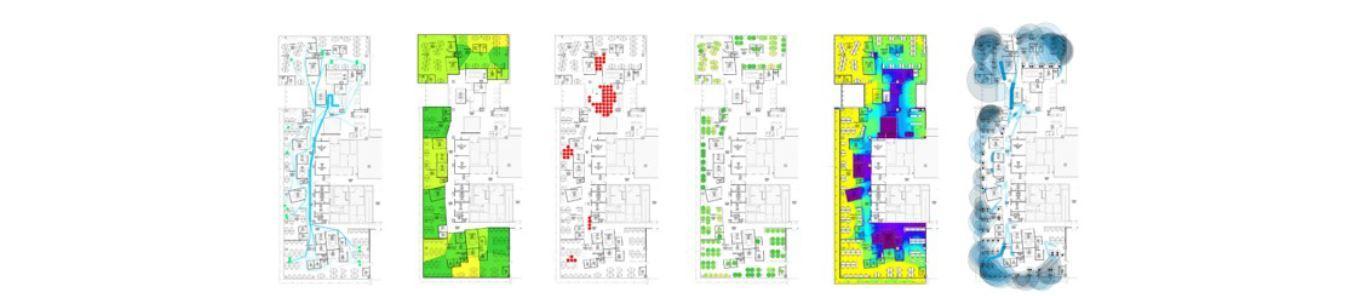
Figure 13. Figures showing analysis of the plans of the office based on the design goals; work style preferences, buzz, productivity, daylight, views to outside
The design has six different metrics to evaluate each design ● Workstyle ● Distraction ● Adjacency ● Interconnectivity ● Daylight ● Views to Outside
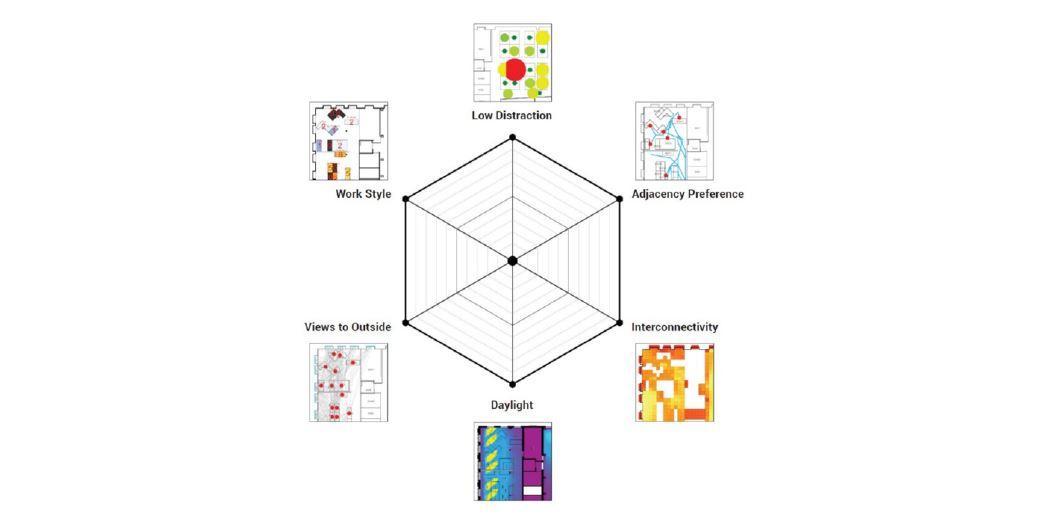
18 Hands-on with Project Rediscover: Generatively Designing the Autodesk Toronto Office. (2020, October 16). Autodesk University. Hands-on with Project Rediscover: Generatively Designing the Autodesk Toronto Office
Redesigning school, Simon Joel, Maine19
Simon Joel conducted an experimental research project called ‘Evolving Floor Plans’ . The goal is to optimize the architectural layout without considering its convention and practicality of it. The design methodology is to give the rooms specifications and the expected flow of people to a genetic algorithm to optimise walking time, traffic density.
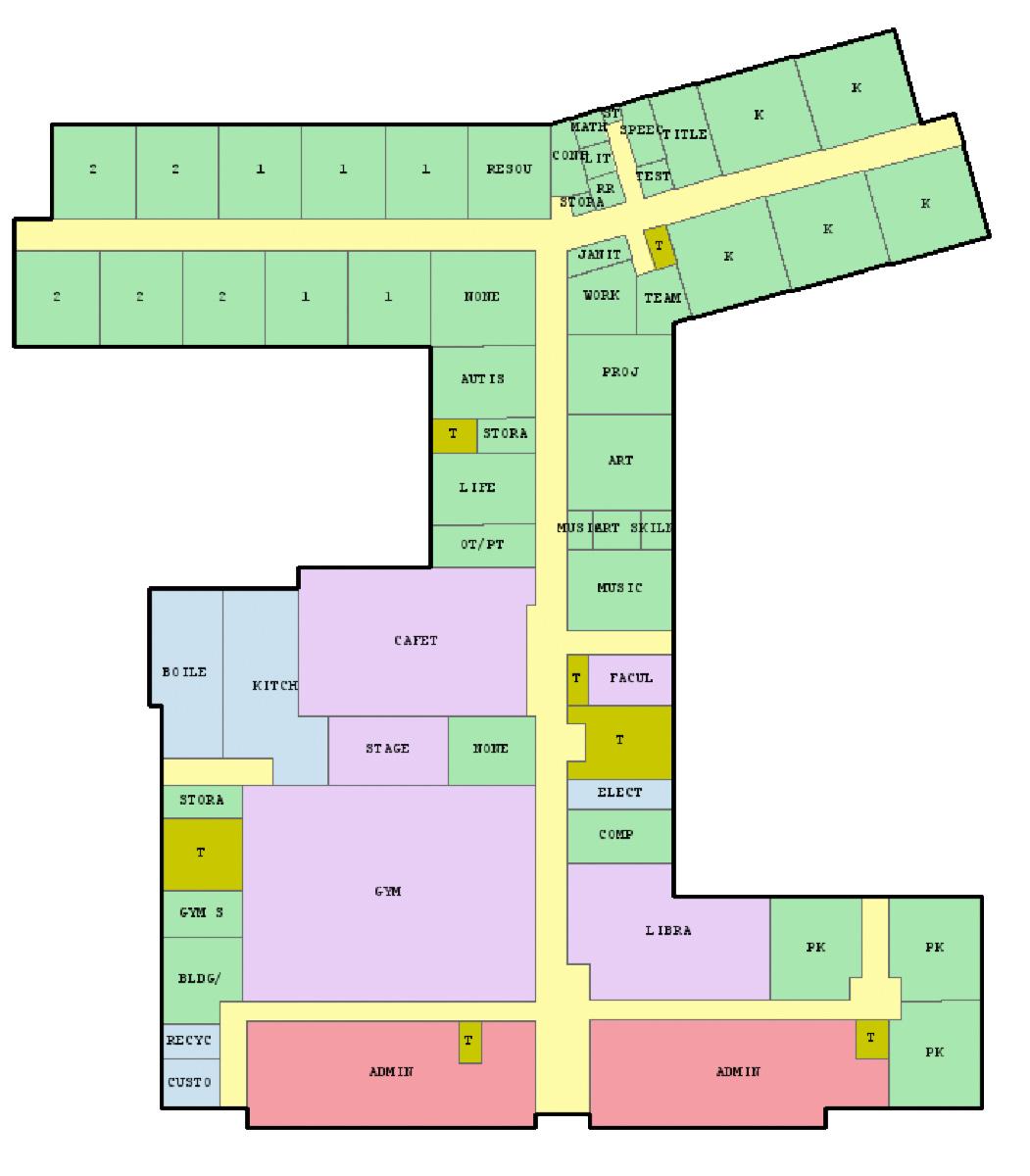
Figure 14. Plan showing the existing layout of the school
The results were biological in appearance with varying widths of hallways and they were not conventional.
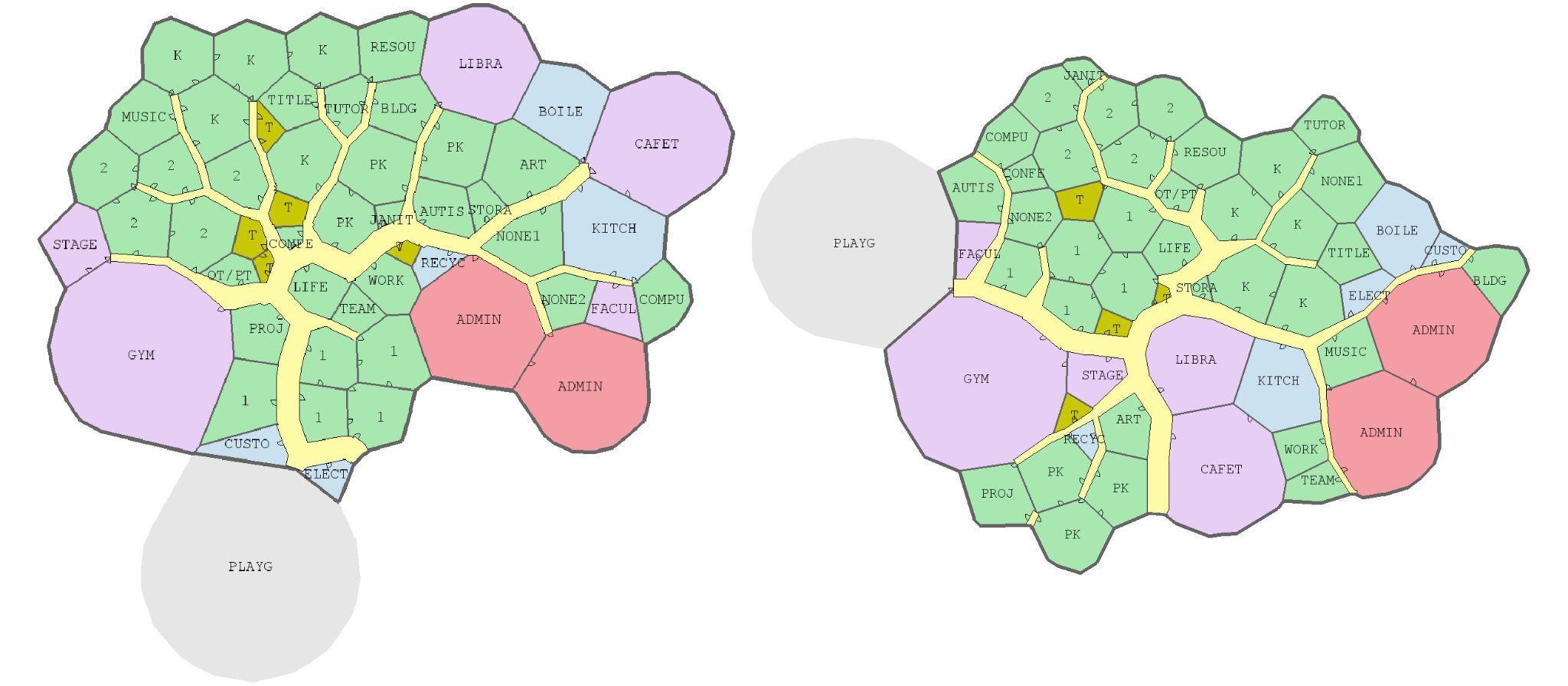
Figure 15. Plans that were generated by the genetic algorithm
19 Evolving Floorplans. (n.d.). Simon Joel. Retrieved July 12, 2021, from Joel Simon
Figure 16. Image showing the design process of the genetic algorithm
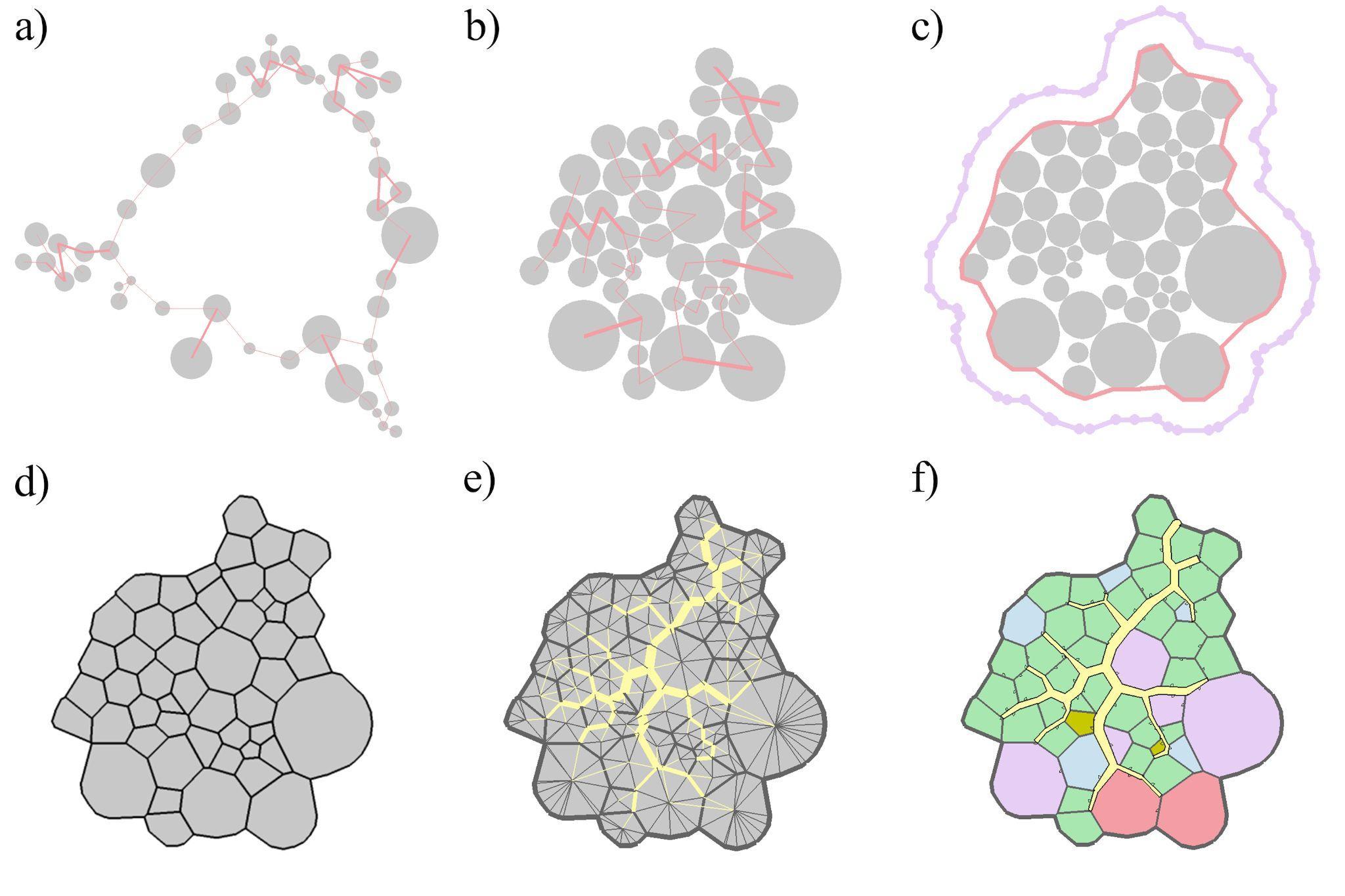
The complete design process of the school is as below.
a) The initial physics simulation using a spectral layout as input. b) The final result of the physics simulation. c) The concave hull of points (red) has been inflated to produce boundary Voronoi seeds (purple circles). d) The Voronoi tessellation creates geometry mesh. e) The floor plan with interior edges added and results of the hallway algorithm drawn in yellow. f) The final floor plan phenotype. Hallways are merged into a final geometry and interior edges used for door placement.
Inferences
Reasoning with the conventional method
When Vertical housing is compared around the world, it can be noticed that there are significant similarities.
Figure 17. Image showing the Urbanscape of different cities
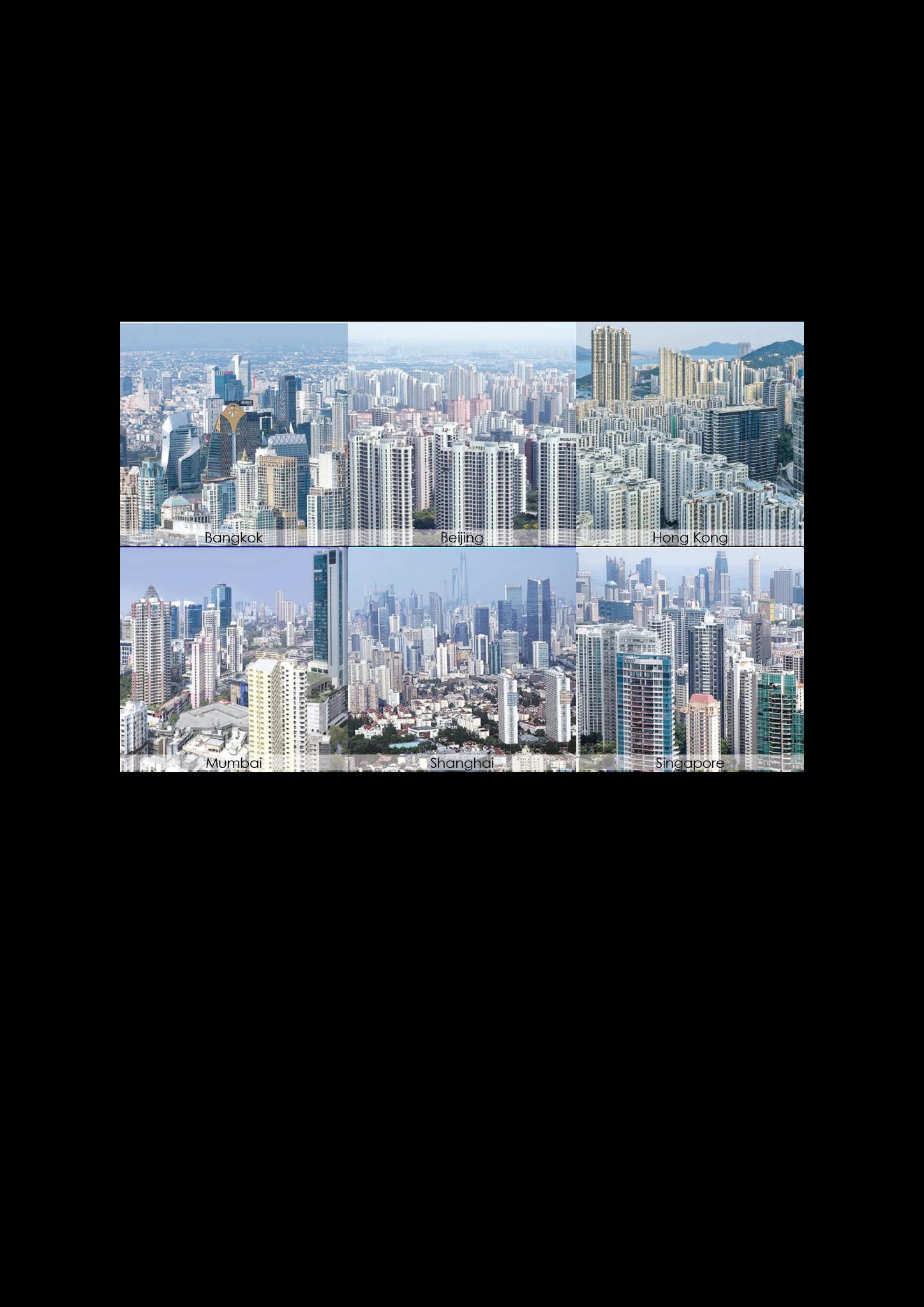
On reasoning about why such a similar phenomenon is occurring everywhere, several reasons answer these questions 1. Globalization: There’s a preconception about development and how “modern” buildings appear. 2. Mass production: There’s mass production and consumption of materials like concrete, steel, glass. 3. By-Laws: By-laws are necessary to prevent chaos in an urban fabric, but they restrict the design because of the linear way of the solution. 4. Ease of design: The conventional method of design involves the multiplication of units across multiple floors. These do not consider user customisation and are easier to design. 5. Fear of innovation: The psychological fear of whether people will accept new methods of design pushes the urge to follow the rest. 6. Resources: Prebuilding a housing system enables the easy allocation of resources and time management
The main concerns that can be noticed with conventional design are as follows1. Monotonous buildings 2. Rigid shapes and volumes 3. Spatial quality (Lack of front yards, backyards, communal spaces) 4. Lack of individuality 5. Limited user customisation 6. Limited response to context & climate
The concerns that can be solved through Generative Design are1. User density can be achieved through space optimisation. 2. The cost is reduced through energy-saving through climate responsive design. 3. GD allows testing and prototyping new materials and structures. 4. Due to the smaller time frame of design with the help of GD, user customisation can be possible. 5. With the help of context and climate data, it is possible to design contextually and climate-responsive buildings
Parameters involved
The following is a list of parameters involved in the design methodology of GD.
1. Site parameters.
These are the parameters that determine the physical boundaries of the design. The input parameters are as follows a. Site geometry b. By-laws such as FAR, offsets, height constraints c. Site conditions (Topography, Ecology)
2. Context parameters.
These are the parameters that determine the nature of the response to its immediate context (Physical and Metaphysical) a. Sensorial (Visual, auditory) b. Materials & construction techniques c. Climate (Sun, wind and rain)
3. Program parameters.
These are the parameters that are set for the users and they determine the type of units that forms the housing scheme a. Typology of units b. Requirement and configuration of spaces c. Adjacency
4. Unit parameters.
These are the parameters that are set for the users and they determine the nature of spaces within the units a. Privacy b. Connectivity c. Visibility
Since the application of the Generative Design is still at its emergent stage, there are some drawbacks 1. It is very difficult to quantify certain qualitative aspects. 2. Since some qualitative aspects are subjective, the set parameters might not be valid in all cases 3. User customization comes with the challenge of adding a plethora of choices that can be difficult to fulfil. So care must be taken to limit the range of customisation 4. The algorithm is only productive with well-defined parameters.