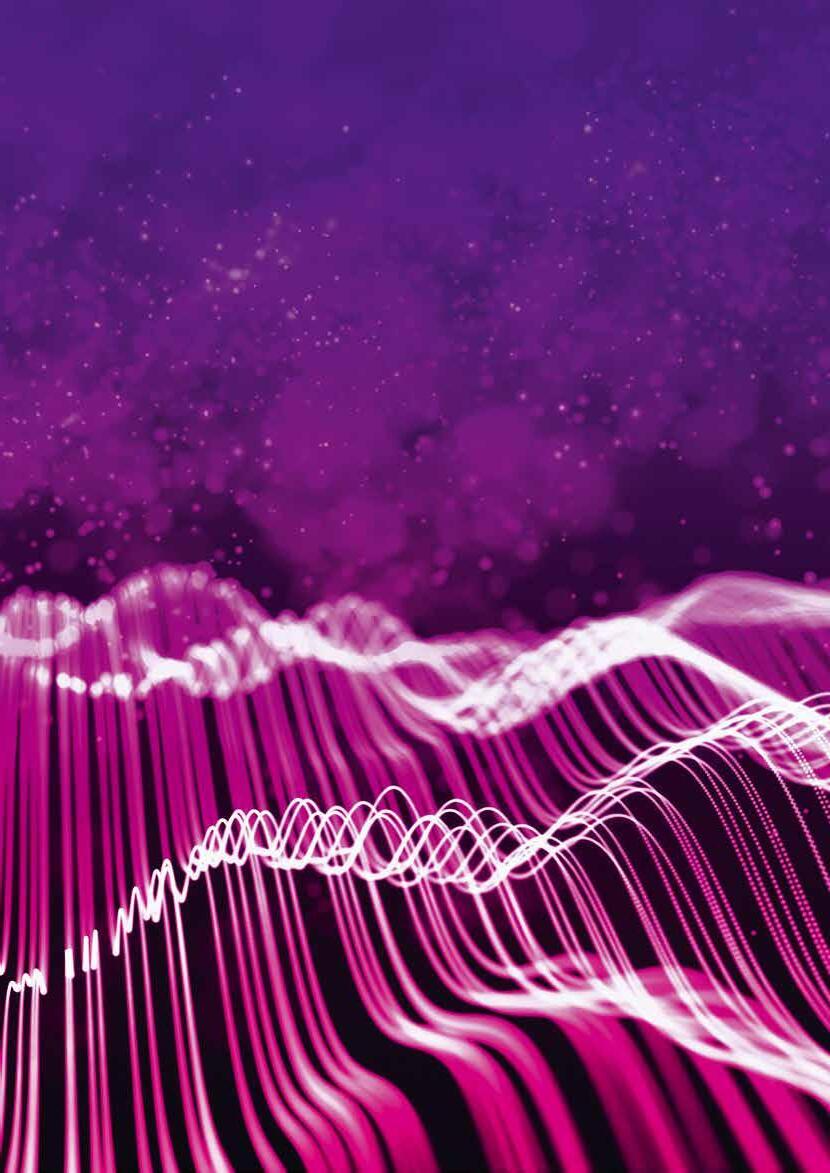
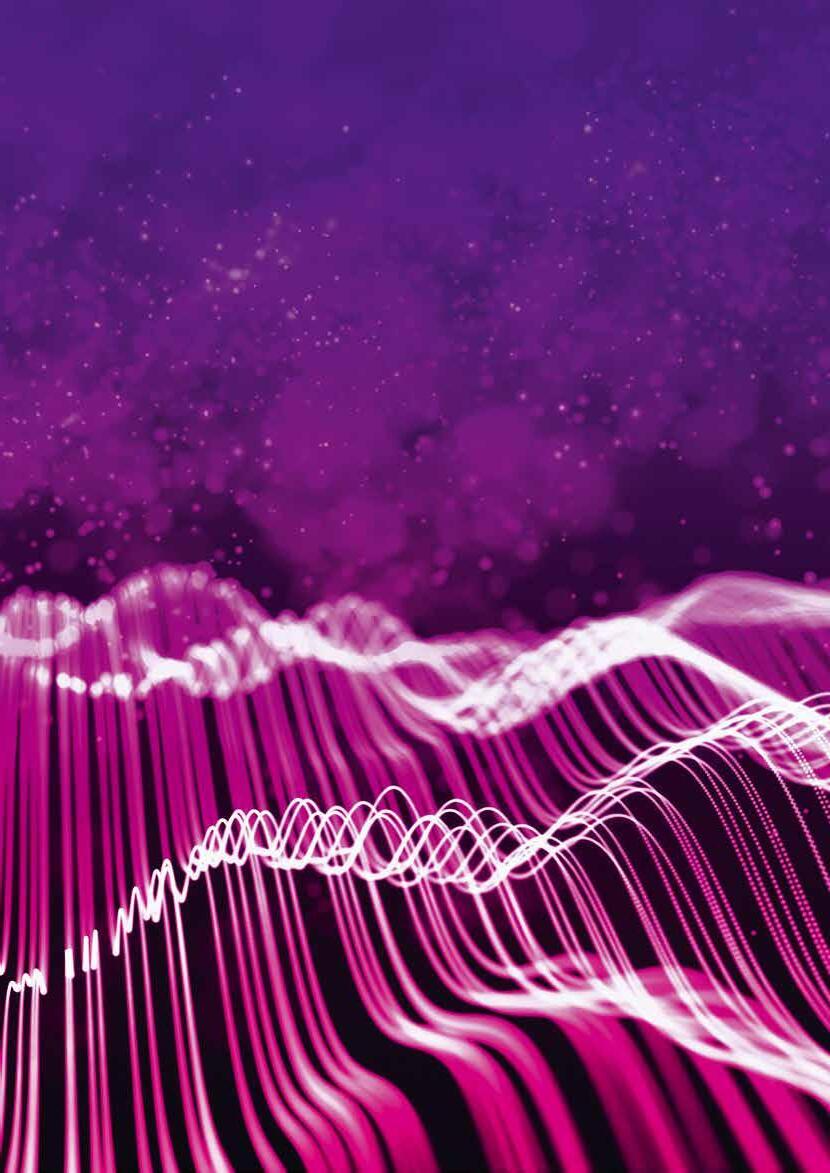
Kommunikation Mensch zu Mensch
Rund 300 Spezialistinnen und Spezialisten arbeiten bei Stämpfli an ihrem Kommunikationserfolg.
Ganzheitlich, fokussiert und umsetzungsstark.
Was bringt die digitale Transformation, und was wird von uns erwartet? Die medizinischen Labore sind Vorreiter und Beispiel für andere Dienstleister im Gesundheitswesen. Aber wir dürfen nicht stehen bleiben.
Die medizinischen Labore sind wie alle anderen Dienstleister im Gesundheitswesen dazu aufgerufen, die Digitalisierung voranzutreiben. Ich habe jedoch den Eindruck, dass eine grosse Unsicherheit besteht, was das eigentlich bedeutet. Man könnte sich sogar fragen, ob wir das wirklich brauchen. Sind ITProjekte nicht sehr teuer, erfordern viel Personal und funktionieren nicht richtig? In dieser Ausgabe der «pipette» diskutieren wir die Gründe, die eine digitale Transformation notwendig machen und welche Probleme des Gesundheitswesens wir mit neuen Anwendungen überwinden können. Wir zeigen, in welchen Aspekten digitale Anwendungen den Menschen überlegen sind. Und wir stellen neue Instrumente vor, die einen Vorgeschmack auf zukünftige Anwendungen bieten. Zudem diskutieren wir Hindernisse der digitalen Transformation. Besonders empfehlen möchte ich Ihnen das Interview mit Professor Antoine Geissbuhler, in dem wir gerade diese grundsätzlichen Fragen besprechen. ●
Qu’apporte la transformation numérique et qu’attendon de nous? Les laboratoires médicaux sont des précurseurs et des exemples pour d’autres prestataires de services dans le secteur de la santé. Mais nous ne devons pas nous arrêter là.
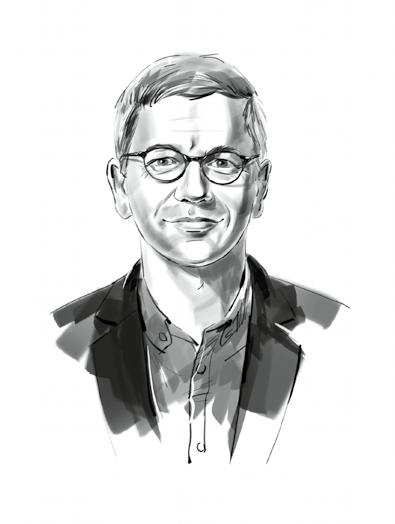
Les laboratoires médicaux, comme tous les autres prestataires de services dans le domaine de la santé, sont appelés à faire progresser la numérisation. J’ai toutefois l’impression qu’il existe une grande incertitude quant à ce que cela signifie réellement. On peut même se demander si nous en avons vraiment besoin. Les projets informatiques ne sontils pas très coûteux, ne nécessitentils pas beaucoup de personnel et ne fonctionnentils pas correctement? Dans ce numéro de pipette, nous discutons des raisons qui rendent une transformation numérique nécessaire et des problèmes du secteur de la santé que nous pouvons surmonter grâce à de nouvelles applications. Nous montrons les aspects sur lesquels les applications numériques sont supérieures aux humains. Et nous présentons de nouveaux instruments qui donnent un avantgoût des applications futures. Nous discutons également des obstacles à la transformation numérique. Je vous recommande tout particulièrement l’interview du professeur Antoine Geissbuhler, dans laquelle nous abordons justement ces questions fondamentales. ●
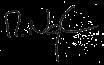
Generative AI for the laboratory professional
Themen
Laboralltag
«Decision support tools» als Instrument zur Weiterentwicklung der Labormedizin

S. 4
Nachrichten
Voraussetzung für die Weiterverwendung von Labordaten
S. 12
Hintergrund
Digital Health: Die Transformation unseres Gesundheitswesens muss einen Gang
zulegen!
S. 18
Porträt
Thomas Sauter, Professor für Telemedizin und ehealth
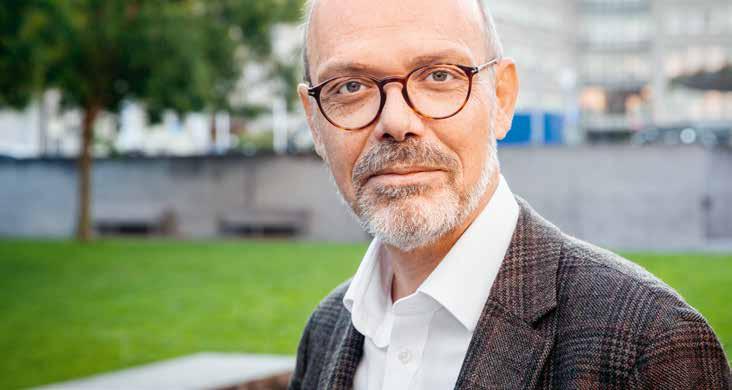
S. 20
Inspiration
Hören und lesen
Generative AI such as ChatGPT will quickly become adopted in a wide range of clinical contexts
S. 7
S. 22
Epilog
Vorschau und Feedback
S. 24
S.14
Portrait
Thomas Sauter est professeur de télémédecine et de cybersanté à l’Hôpital de l’Île de Berne.
P. 21
Möchten Sie frühere Printausgaben der «pipette» bestellen? Wenden Sie sich an: pipette@sulm.ch
Au quotidien
«Decision support tools» comme instrument de développement de la médecine de laboratoire
P. 5
Dossier
Generative AI for the laboratory professional
P. 7
Actualités
Conditions préalables à l’utilisation secondaire des données de laboratoire
P. 13
Interview
Why does healthcare digitalization matter?
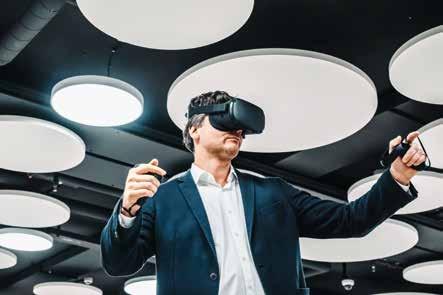
P. 14
Informations de fond
La santé numérique: la transformation de notre système de santé doit passer à la vitesse supérieure!
P. 19
Inspiration
Écouter et lire
P. 23
Épilogue
Aperçu et commentaires
P. 24
Die «pipette» berichtet über relevante Themen der Schweizer Labormedizin.Alle Ausgaben auf sulm.ch/de/pipette/ ausgaben
«Il est important qu’en tant que professionnels de la santé, nous participions à l’élaboration des développements et qu’ils ne soient pas simplement imposés par l’industrie ou la technique.»Insel Gruppe / Pascal Triponez
«Decision support tools» als Instrument zur Weiterentwicklung der Labormedizin
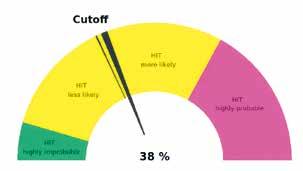
Die Labormedizin muss mitgestalten, anstatt nur zu reagieren, um auch in Zukunft als wichtige Disziplin zu bestehen. Eine Möglichkeit sind sogenannte «decision support tools». Hiermit können verschiedene Laborwerte und weitere diagnostisch wichtige Informationen (klinisch; Bildgebung) integriert werden, häufig mithilfe von MachinelearningAlgorithmen. Idealerweise kann die klinische Fragestellung des Arztes direkt beantwortet werden. Das setzt
jedoch minutiös geplante klinische Studien voraus. Ein Beispiel ist der kürzlich entwickelte und validierte Algorithmus zur Diagnose der Heparininduzierten Thrombozytopenie (HIT).
sulm.ch/23/l7 sulm.ch/23/l8
Schnittstelle gut – alles gut?
Oder könnte Digitalisierung basierend auf «Data Literacy» mehr?
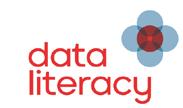
Digitalisierung ist unerlässlich, und wir entkommen den mit ihr verknüpften positiven wie negativen Entwicklungen nicht. Umso dringender sollten wir uns die Frage stellen, ob wir Digitalisierung als Chance wahrnehmen und im Sinne eines Wandels zu einer gemeinsamen DataLiteracyKultur nicht innovativer und interprofessioneller angehen sollten. Wäre es nicht
hilfreich, kritische und weniger kritische Normwertüberschreitungen differenzierter zu signalisieren und entsprechend abgestufte Warnsysteme einzubauen? Könnten wir personalisierte Zielwerte einbauen, irrelevante, marginale Normwertüberschreitungen als solche auch für Patienten verständlich visualisieren?
So könnten wir uns weg von SchwarzweissDiskussionen hin zu einer interaktiven Diskussionskultur rund um Labordaten, ihre Wertigkeit und Kontextualisierung bewegen (www.dataliteracy.ch).
Beitrag Dr. med. Henning Nilius, Universitätsinstitut für Klinische Chemie, Inselspital, Bern Beitrag Dr. med. Monique Lehky Hagen, Präsidentin Walliser Ärztegesellschaft, VSÄGSchnittstelle:
Digitalisierung als Anstoss zu einem fundamentalen
Wandel unseres Umgangs mit Daten.»
Contribution Dr med. Henning Nilius, Institut universitaire de chimie clinique, Hôpital de l’Île, Berne
La médecine de laboratoire doit participer à la conception et non pas seulement réagir, si elle veut rester une discipline importante à l’avenir. Les «outils d’aide à la décision» sont une possibilité. Ils permettent d’intégrer différentes valeurs de laboratoire et d’autres informations importantes pour le diagnostic (clinique; imagerie), souvent à l’aide d’algorithmes «machine learning». Dans l’idéal, il est possible de répondre directement à la question clinique du médecin. Cela suppose toutefois des études cliniques minutieusement planifiées. Un exemple est l’algorithme récemment développé et validé pour le diagnostic de la thrombocytopénie induite par l’héparine (TIH):
sulm.ch/23/l9
sulm.ch/23/l10
Contribution Dr med. Monique Lehky Hagen, présidente de la Société Médicale du Valais, SMVS La digitalisation est indispensable et nous n’échappons pas aux évolutions positives et négatives y relatives. Il est d’autant plus urgent de se demander si nous ne devrions pas percevoir la digitalisation comme une chance pour l’aborder de manière plus innovante et interprofessionnelle afin d’initier un changement vers une culture commune de «data literacy». Ne seraitil pas utile de signaler de manière plus différenciée les dépassements critiques et moins critiques des valeurs normatives et d’intégrer des systèmes d’alerte gradués en conséquence? Pourrionsnous intégrer des valeurs cibles personnalisées, visualiser les dépassements de normes marginaux et non pertinents en tant que tels, de manière compréhensible pour les patients? Nous pourrions ainsi nous éloigner des discussions «en noir et blanc» et nous diriger vers une culture de discussion plus interactive autour de la valeur et de la contextualisation des données de laboratoire (www.dataliteracy.ch).
Une bonne interface tout baigne? Ou la numérisation basée sur la «data literacy» pourraitelle faire plus?
«Decision support tools» comme instrument de développement de la médecine de laboratoire
«Ich träume davon, dass Digitalisierung mehr sein könnte als eine
«Je rêve que la digitalisation puisse être plus qu’une interface – la digitalisation comme impulsion vers un changement fondamental de notre approche des données»
QUALAB und Praxislabor
Beitrag Dr. Martin Risch, Präsident QUALAB
Zwei Fragen erreichen mich seit einigen Monaten regelmässig aus den Praxislaboren: Warum muss ich mich mit einer zusätzlichen GLNNummer registrieren, und was ist die QUALAB eigentlich? Die kurze
Begründung: QUALAB ist die «Schweizerische Kommission für Qualitätsentwicklung im medizinischen Labor». Im gesetzlichen Auftrag handelt diese die Qualitäts
entwicklung im medizinischen Labor aus, weshalb auch die Praxislabore verpflichtet sind. Vertragspartner sind FAMH, FMH, H+, pharmaSuisse, santésuisse und MTK. Zur Qualitätskontrolle gehören die interne und die externe Qualitätskontrolle, und registriert werden Laboratorien, die mit einer eindeutigen GLNNummer identifiziert sind. Die ausführliche
Begründung findet sich hier: sulm.ch/23/l11
QUALAB et laboratoire
de cabinet médical
Contribution Dr Martin Risch, président de la QUALAB
Depuis quelques mois, une question me parvient régulièrement des laboratoires de cabinet médical: pourquoi doisje m’enregistrer avec un numéro GLN supplémentaire et qu’estce que la QUALAB en réalité? La réponse est brève: la QUALAB est l’«Association suisse pour le développement de la qualité dans les laboratoires médicaux». Dans le cadre de son mandat légal, elle négocie le développement de la qualité dans les laboratoires médicaux, à laquelle les laboratoires de cabinet médical sont également tenus. Les partenaires contractuels sont la FAMH, la FMH, H+, pharmaSuisse, santésuisse et MTK. Le contrôle de qualité interne et externe fait partie du contrôle de qualité et les laboratoires identifiés par un numéro GLN unique sont enregistrés. La réponse détaillée se trouve ici: sulm.ch/23/l12
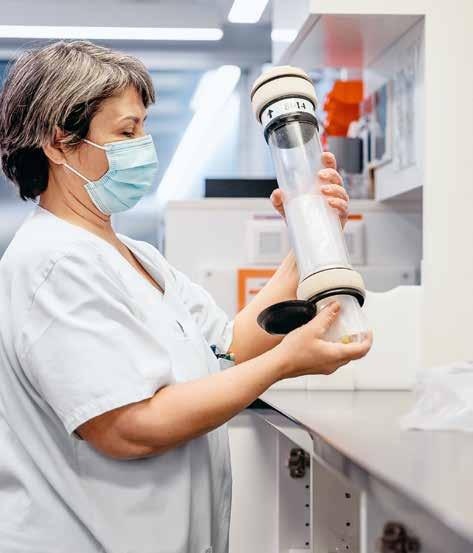
Generative AI for the laboratory professional
Janna Hastings, PhD, Professor of Medical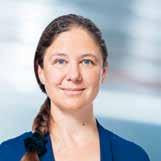
ChatGPT, developed by the company OpenAI, is an example of a language model, an AI system able to generate text in response to an input prompt. Since its release in autumn 2022, it has shown remarkable success for medical challenges that were previously unsolved by AI applications. For example, it has succeeded in achieving a passing grade in written medical exams, and it has been found to respond appropriately and empathically to patient questions from an online forum. Of course, the performance of the system on
these tasks is not perfect, and each of the studies has limitations, but it would be fair to say that the technology has achieved a breakthrough performance relative to what was possible before. Even more significantly, this performance was achieved despite the fact that the system was not specifically trained with medical knowledge. Rather, it was trained domainindependently to understand and be able to respond to text – whatever topic the text was about. The very same system has also achieved a breakthrough performance in challenges within a wide range of other domains, including examples as diverse and far from medicine as law and computer programming.
The innovation did not come out of nowhere – AI has a long history –, and the past years have seen a series of key ingredients developed that have come together in the explosion of capabilities we see today (Figure 1). Early medical AI “expert systems”, which are now sometimes referred to affectionately as “good oldfashioned AI”, relied on logical reasoning together with explicit factual knowledge being captured in a form that a computer could understand. However, capturing such knowledge could be slow and many areas of clinical uncertainty could not be well described in rigid logical languages. Machine learning, in which complex models such as neural networks are trained from data to be able to make predictions on unseen data, could handle uncertainty better, and enabled AI systems to be developed that learned from examples rather than having to have explicit rules being provided.
A major breakthrough occurred with the development of algorithms that improved the training process on “deep”
expert systems, knowledge standards, rules and dynamic logic-based inference
expert systems, knowledge standards, rules and dynamic logic-based inference
growing applications of data-driven supervised machine learning in imaging, pathology, discovery, diagnosis and prognosis
growing applications of data-driven supervised machine learning in imaging, pathology, discovery, diagnosis and prognosis
deep learning with arti cial neural networks, big data, growing computer resources
deep learning with arti cial neural networks, big data, growing computer resources
really big data, transfer learning, large-scale general-purpose generative pre-trained models for language, images and more
really big data, transfer learning, large-scale general-purpose generative pre-trained models for language, images and more
ContributionKnowledge
Decision
Until recently, artificial intelligence (AI) systems were only able to perform narrowly defined tasks to automate small steps in clinical workflows. However, this is set to change with generative AI, the new technology that underlies blockbuster tools such as ChatGPT. But what is new about this type of AI, and what impact is it likely to have in the laboratory?Figure 1: Milestones in the development of artificial intelligence technologies for medical applications
neural networks (which have multiple “layers” between input and output). These networks were significantly larger and more complex than previous models had been and were thus able to significantly outperform previous approaches on a number of problems, provided sufficient training data could be found with which to train them. A further key innovation was the development of techniques to train these large models on unlabelled data, simply by hiding parts of the input and getting the model to predict back the missing bits. This expanded the capability to amass sufficient training data for larger and larger models to be trained, alongside improvements in computing capability on highperformance machines. The volumes of text needed to train modern largescale language models such as ChatGPT are truly enormous. Hundreds of gigabytes of text data have been scraped off the internet for this purpose – including the texts of books, encyclopaedias, social media posts, discussion group conversations, blogposts, and vast numbers of individual websites.
The final piece in the puzzle that led to the breakthrough of ChatGPT was the addition of a dedicated training step specifically towards performance in a dialogue setting. Dialogue capabilities were trained by applying a new type of training to further refine the model after the generalpurpose language training was complete: reinforcement learning from human feedback. To make this possible, many human workers were employed to create examples of good dialogue responses as training data for the model, and to give dynamic feedback on the model’s performance. Equipped with the basic capability of processing written text, and then further trained for performance in a dialogue
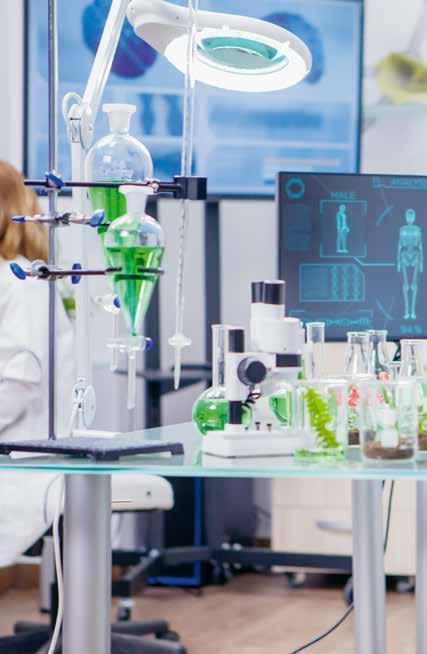
«Generative AI will quickly become adopted in a wide range of clinical contexts. Therefore, it is important to know the limitations and potential risks.»
setting, the model was then released to the public to obtain additional feedback from which it could learn further. The result is a new wave of AI tools capable of responding to wellformed text instructions, by producing wellformed text in response, where the response is conditioned on a substantial part of the general knowledge available on the internet, including medical knowledge. Moreover, textbased models such as ChatGPT are not the only type of generative AI models, even if they are currently the most wellknown. There are generative models for image based data as well, and many other data types of clinical relevance, such as proteins and drugs. In addition, there are “multimodal” models that are trained on combinations of data types, such as text and images, where the description of the images in text contains important and relevant information about the images. This type of multimodal model has the capability
of generating text in response to images (i.e. automating the description of images) and also for generating images in response to text.
But what impact is this kind of generative AI technology likely to have on laboratory medicine? We might start with a little experiment and ask ChatGPT itself to tell us the answer (see text box).
As is a typical behaviour for the system when answering contentheavy questions, it lists several possibilities. These are: answering questions, research assistance, test interpretation, educational support, protocols and procedures, quality assurance, and training and education. To answer questions and assist research are relatively unproblematic applications of the technology. The possibility of providing educational materials and assisting in explaining and summarising content is potentially exciting, as it could
What ChatGPT can do for laboratory medicine, in its own words
Janna Hastings: What can you do for laboratory medicine? ChatGPT: As an AI language model, I can provide assistance and support in various ways within the field of laboratory medicine. Here are a few examples:
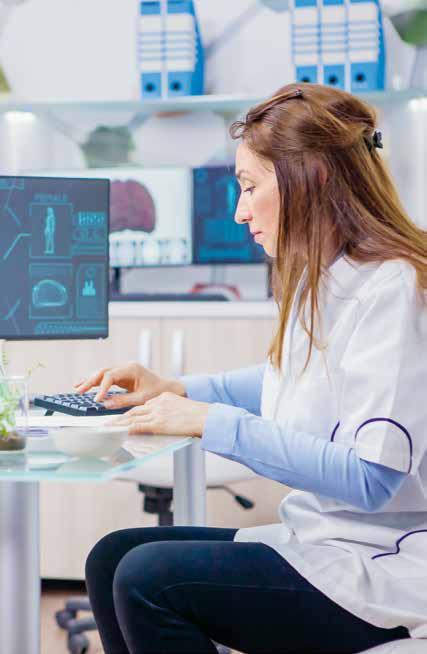
1. Answering Questions: I can help answer questions related to laboratory medicine, including laboratory techniques, equipment, test interpretation, and methodologies.
2. Research Assistance: I can aid in literature searches, summarizing scientific articles, and providing up todate information on laboratory medicine topics.
3. Test Interpretation: I can help explain laboratory test results and their implications, including reference ranges, normal values, and potential clinical significance.
4. Educational Support: I can assist in explaining complex concepts in laboratory medicine, providing explanations, definitions, and examples to enhance understanding.
5. Protocols and Procedures: I can help with the development or clarification of laboratory protocols and procedures, ensuring accuracy and adherence to best practices.
6. Quality Assurance: I can offer guidance on quality control and quality assurance processes within the laboratory, including troubleshooting and error prevention.
7. Training and Education: I can assist in developing educational materials, presentations, or training modules related to laboratory medicine topics.
It’s important to note that while I can provide information and support, I cannot replace the expertise and judgment of a qualified healthcare professional or laboratory specialist. Always consult with trained professionals for accurate diagnosis, treatment decisions, and laboratory results interpretation.
be a route by which difficult or challenging material could be simplified and summarised on several different levels for digestion by different learners or as steps along a learning trajectory. However, for the remaining points – test interpretation, protocols and procedures, and quality assurance –it is not immediately clear how a generalpurpose model could be directly applied, as these processes would depend on knowledge about an individual patient’s situation being combined with contextual and laboratoryspecific factors that would not typically be available to a language system such as ChatGPT. A custom implementation would be required to apply the relevant language technology as an embedded capability within a specific hospital and laboratory system before it could begin to be helpful in these ways.
The technology has other potential applications not envisioned by ChatGPT in this example response. Naturally, clinicians will be interested in how such technologies can make clinical work easier. One promising possibility is that the technology can be applied to reduce the burden of administrative documentation in electronic health records. Because of the improved languageprocessing performance of this technology, it can be anticipated that language interfaces for capturing and recording information will improve to support the production of clinical documentation at an accelerated rate with less manual data entry effort. Exchange of information between practitioners may become more natural. And the technology has great potential to support clinicians in keeping on top of the evergrowing medical evidence base.
With all these potential benefits, it is likely that generative AI will quickly become adopted in a wide range of different clinical contexts. Therefore, it is important to also know the limitations and potential risks. Most importantly, it is important to be aware that language models do not possess actual knowledge, in the sense that they do not “know” the difference between what is true and what is not. They can be wrong, and they can make up plausiblesounding “facts”. It is
important to check the output of the tool carefully, and to be mindful of the context in which the output is intended to be used. Language models are also biased, as they are trained on biased data. One important bias is the bias towards the English language. Even though such models can perform translations and operate in languages other than English, their performance is potentially impacted negatively by the language medium. Other stereotypes and biases that are reflected in their training data may also be represented in their outputs, such as sexism and racism. For all these reasons, the technology should be employed with vigilance, and only in contexts that enable suitable verification.
However, the biggest impact of these new technologies is likely to be more subtle than its direct application in clinical workflows: an acceleration of the rollout of AI applications for an extremely wide range of clinical applications. This is because the data bottleneck that limited the development of highperformance AI systems in medicine until now will be overcome by the new technology in several ways. Firstly, generalpurpose models can serve as foundations such that relatively fewer examples are needed for additionally training such models for specific clinical applications. In addition, the technology will be able to support and accelerate the pre processing of data and data exchange through supporting extraction from the electronic health record and pretreatment (cleaning, standardising, filling in missing data). Finally, the system will be able to augment and supplement existing data sets with expanded synthetic examples. With the removal of these traditional bottlenecks, more clinical data will become unlocked for the benefit of downstream AI systems. And while the development of largescale models such as ChatGPT have initially been driven by large commercial organisations, the power of the technology is not restricted to commercial providers. Some models with similar capabilities are being developed opensource, which enables private and democratic use, and accelerates further development. ●
«While in past medicine has been a relatively slow adopter of AI technology, generative AI just might be the key that unlocks the floodgates.»
Auf Surveillance kommt es an
PCR-Schnelltests für eine optimale Infektionskontrolle
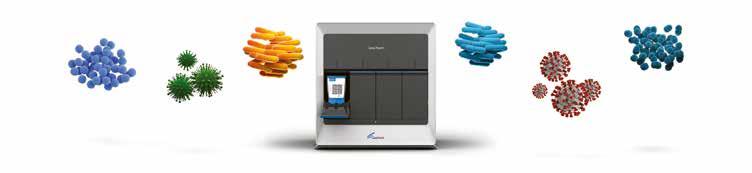
Für Patient/innen
• Schnellere Entisolierung der negativen Patient/innen
• Reduzierung unnötiger Antibiotikaprophylaxe
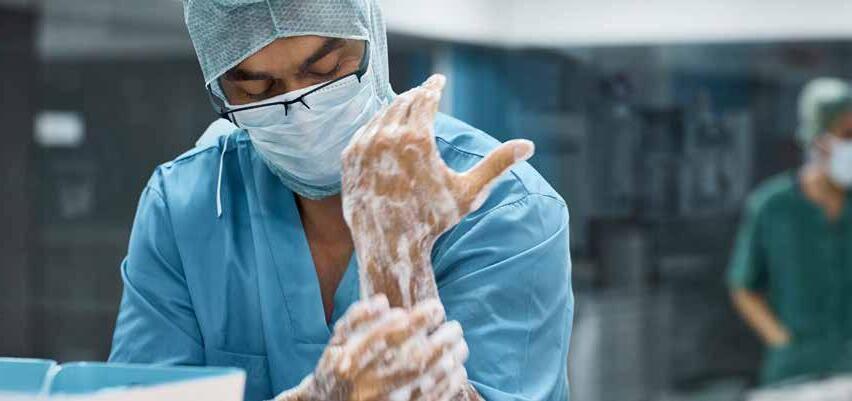
• Aufenthalt in einem Krankenhaus, das frei von nosokomialen Infektionen ist
Für Klinikpersonal
• Schnelle Ergebnisse können das Patientenmanagement verbessern
• Isolierungen nur bei Bedarf
• Angemessenerer Einsatz von Antibiotika
Für Ihr Krankenhaus
• Reduzierung von Transmissionen und Ausbrüchen
• Besseres Patientenmanagement
• Kostenreduzierung und bessere Budgetkontrolle (Isolation, Aufenthaltsdauer, weniger Antibiotika)
Eine einfache Plattform. 31+ verfügbare Tests
Besuchen Sie uns auf www.cepheid.com/DE und erfahren Sie mehr über die CE-IVD-Tests von Cepheid, die auf dem GeneXpert system laufen.
www.Cepheid.com/de

Laufzeit hängt vom jeweiligen Test ab. Genaue Angaben zur Laufzeit entnehmen Sie bitte der jeweiligen Packungsbeilage.
Abbruch des Assays (Early Assay Termination, EAT) nur bei positiven Ergebnissen bei In‑ uenza oder nur bei RSV. Ausgabe von negativen Ergebnissen und Ergebnissen von Kombinationstests in 30 Minuten.
Abbruch des Assays (EAT, Early Assay Termination) bei SARS CoV 2. Negative Ergebnisse nach ca. 45 Minuten.
In-vitro Diagnostikum. Möglicherweise nicht in allen Ländern erhältlich.
Artificial Intelligence ist derzeit – insbesondere nach dem Aufkommen von «Large Generative Models» (z.B. ChatGPT) – in aller Munde und macht auch vor der Labormedizin nicht halt. In einer so stark digitalisierten Disziplin wie der unseren bieten sich vielfältigste Anwendungsmöglichkeiten, aber wie bei jeder neuen Technologie auch unzählige Herausforderungen: Während sich bisher der Fokus stark auf Prozessund analytische Qualität richtete, kommt den FAIRPrinzipien (findable, accessible, interoperable, reusable) für die Zweitnutzung eine neue Bedeutung und der Labormedizin ein neues Handlungsfeld zu.
sulm.ch/23/n17
sulm.ch/23/n18
LOINC Codes können angefordert werden
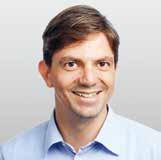
Die Kodierung von Laborwerten mit der Hilfe von LOINC Codes wird als wichtige Voraussetzung für Digitalisierungsbemühungen in der Labormedizin angesehen. Ein Problem entsteht, wenn es den entsprechenden LOINCBegriff noch nicht gibt. Nun ist es jedoch möglich, neue Codes anzufordern. Dafür hat die Organisation einfache Eingabemasken entwickelt: sulm.ch/23/n19
Mehrere Studien zur Laborbranche Schweiz unterwegs
Die Kosten des Gesundheitswesens sind in der politischen Diskussion ein heisses Thema, und die Labortarife stehen aktuell im Fokus. Mehrere Initiativen einschliesslich der KostenbremseVolksinitiative sind dazu unterwegs. Diese Aktivitäten könnte die medizinischen Labore unter Druck setzen und sogar zu einer Unterversorgung führen, zum Beispiel im Falle einer erneuten Pandemie. Um sachlich fundierte Argumente für die politische Diskussion zu erarbeiten, sind drei grössere Studien unterwegs, die von der FAMH, der SULM, dem SVDI und anderen Organisationen in Auftrag gegeben worden sind. Diese sollen den Wert und die Situation der Laborbranche in der Schweiz erarbeiten. Die «pipette» wird in den kommenden Ausgaben die Ergebnisse präsentieren.
«Die Weiterverwendung von Labordaten im Rahmen von AIAnwendungen ist mit vielerlei Herausforderungen verbunden»Beitrag Prof. Dr. med. Alexander Leichtle, Gruppe Computational Medicine, Universitätsinstitut für Klinische Chemie, Inselspital Bern
Die Vertragsfreiheit bei Laboranalysen ist vom Tisch
Der Nationalrat kippte in der Sommersession die Einführung der Vertragsfreiheit bei Laboratorien. Dem Originaltext des indirekten Gegenvorschlags zur Kostenbremse Initiative folgend, sollten Laboranalysen nur noch dann vergütet werden, wenn die Krankenkasse des Patienten einen Vertrag mit dem Laboratorium geschlossen hat. Das hätte erhebliche Unsicherheiten und einen grossen Verwaltungsaufwand zur Folge gehabt. Der indirekte Gegenvorschlag ist eine Massnahme des Bundesrates zur Volksinitiative der MittePartei: sulm.ch/23/n20
Contribution Alexander Leichtle, groupe Computational Medicine, Institut universitaire de chimie clinique, Hôpital de l’Île, Berne Conditions
préalables à l’utilisation secondaire des données de laboratoire
L’intelligence artificielle fait actuellement l’objet de toutes les attentions – notamment après l’apparition des «large generative models» (p. ex. ChatGPT) – et même la médecine de laboratoire n’y échappe pas. Dans une discipline aussi fortement digitalisée que la nôtre, les possibilités d’application les plus variées s’offrent à nous, mais comme pour toute nouvelle technologie, les obstacles à surmonter sont innombrables.
Alors que jusqu’à présent, la priorité était fortement donnée à la qualité des analyses et des procédures, les principes FAIR (findable, accessible, interoperable, reusable) revêtent une nouvelle importance pour l’utilisation secondaire et la médecine de laboratoire se trouve confrontée à un nouveau champ d’action. sulm.ch/23/n21 sulm.ch/23/n22
Les codes LOINC peuvent être demandés
La codification des valeurs de laboratoire à l’aide de codes LOINC est considérée comme une condition importante pour les efforts de numérisation en médecine de laboratoire. Un problème survient lorsque le terme LOINC correspondant n’existe pas encore. Il est toutefois désormais possible de demander de nouveaux codes. Pour ce faire, l’organisation a développé des masques de saisie simples: sulm.ch/23/n23
Plusieurs études sur le secteur des laboratoires en Suisse en cours
Les coûts du système de santé sont un sujet brûlant dans le débat politique et les tarifs des laboratoires sont actuellement au centre des préoccupations. Plusieurs initiatives, y compris l’initiative populaire «Frein aux coûts», sont en cours. Ces activités pourraient mettre les laboratoires médicaux sous pression et même entraîner une pénurie de soins, par exemple en cas de nouvelle pandémie. Afin d’élaborer
des arguments fondés pour le débat politique, trois grandes études sont en cours, commandées par la FAMH, l’USML, l’ASID et d’autres organisations. Elles ont pour but de déterminer la valeur et la situation du secteur des laboratoires en Suisse. La pipette présentera les résultats dans les prochains numéros.
d’actualité
Lors de la session d’été, le Conseil national a renversé l’introduction de la liberté de contracter pour les laboratoires. Suivant le texte original du contreprojet indirect à l’initiative sur le frein aux coûts, les analyses de laboratoire ne devaient plus être remboursées que si la caisse maladie du patient avait conclu un contrat avec le laboratoire. Cela aurait entraîné des incertitudes considérables et une charge administrative importante. Le contre projet indirect est une mesure prise par le Conseil fédéral en réponse à l’initiative populaire du parti centriste.
sulm.ch/23/n24
«La réutilisation des données de laboratoire dans le cadre d’applications IA est liée à de nombreux pièges»
La liberté de contracter pour les analyses de laboratoire n’est plus
Why does healthcare digitalization matter?
Prof. Dr. Antoine Geissbuhler, Vicerector of the University of Geneva, Director of the Division of eHealth and Telemedicine at Geneva University Hospitals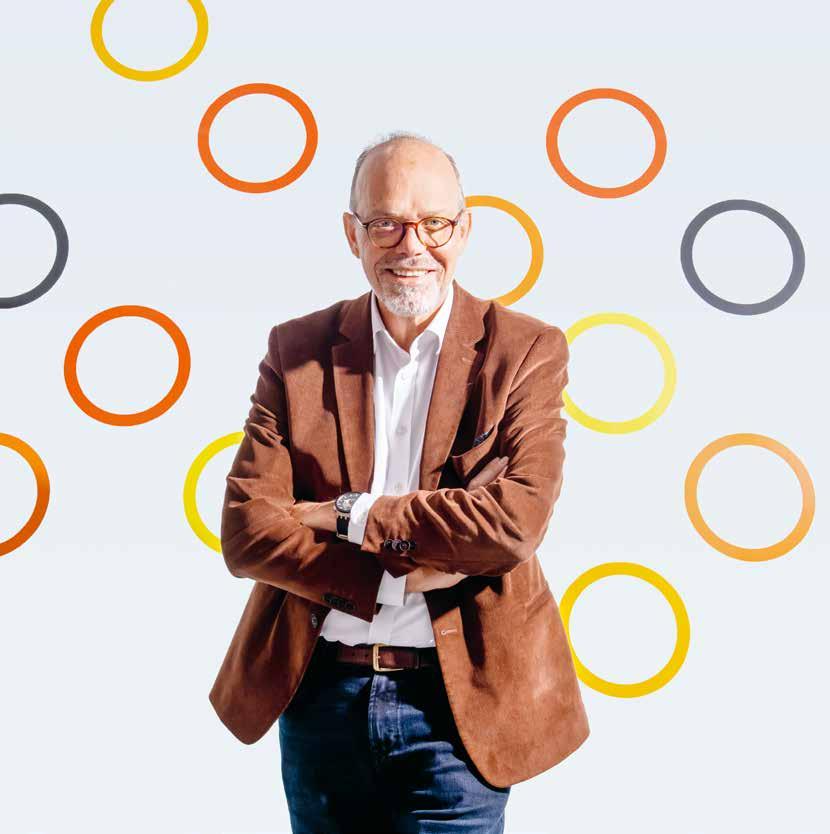
Interview Prof. Dr. Dr. Michael Nagler, Editor-in-chief «pipette»
Digitalization is seen as a key tool for addressing healthcare challenges. But is it worth the effort, and how big are the risks? We discuss these questions with Antoine Geissbuhler, Professor of Medical Informatics and Vicerector of the University of Geneva.
MN: Prof. Geissbuhler, you are the director of the division of eHealth and Telemedicine at Geneva University Hospitals, and you are the Pastpresident of the International Medical Informatics Association. Why is digitalization in healthcare important?
AG: Healthcare is about processing information. And some quality experts have coined a slogan that says, information is care. Having quality information and being able to transmit it to make it available when decisions need to be made improves healthcare quality. Medical errors are the third leading cause of death, at least in the U.S. and most likely throughout the developed world. So, it’s important. We have been trying for decades now to see if we can improve the quality and safety of healthcare by digitizing this information, by making it computerprocessable, and by sharing it in a way that is more efficient. And when we do so, we change the healthcare system systemically. I doubt that any hospital that has implemented a laboratory information system or hospital information system would go back to paper now. But there is another, more fundamental reason: the limitation of the human brain. We know that our capacity to manage a lot of information simultaneously is limited. In a busy setting of a university hospital, we probably reach that level quite soon. Besides, we have a limited
ability to store and to memorize information, especially with the explosion of medical knowledge. We know also that we can get tired at times. The fourth thing is that we have a pretty bad track record in dealing with probabilities. That’s why casinos are still successful. And all those things computers can do well.
MN: To reduce these sources of error, we began digitizing data transmission in the laboratory decades ago. With 30,000 laboratory tests per day, as in our laboratory, manual work would quickly result in an unbearably high error rate.
AG: In fact, it’s no wonder why labs have been at the forefront of healthcare digitization. There’s a culture of quality improvement, there is a well understood risk of transcription, much more so than when you deal with prescriptions of medicines. One of the key challenges remaining is the semantic interoperability, the ability to share information in such a way that lab results produced in one place can be compared and processed with lab results produced elsewhere. LOINC codes are probably the best and most widely accepted terminology. However, there are adoption issues in Switzerland, and that’s something that we would like to address. This will enable enhanced functionalities that are very significant for healthcare safety and quality. And I think at the time where we start deploying electronic health records, it’s somewhat of a shame that the only thing we can find in those electronic health records at the most are PDF files.
MN: However, one might argue that hospital and lab information systems are very expensive, require a lot of human resources to build them and yet do not function properly.
AG: This is a big challenge indeed. These tools are the nervous system of hospitals and labs. Nervous systems by definition are quite complex and expensive. I believe humans use 25% of total energy for their nervous system. But if you don’t have these systems, you’re stuck in very basic behaviors. I think we have to recognize that information systems are going to be an issue, are going to be expensive, are going to be complex. The tools are evolving, the methodologies are evolving, the professions are evolving. And you cannot
«It’s no wonder why labs are at the forefront of healthcare digitization. There’s a culture of quality improvement, and there is a well understood risk of data transfer.»
have it cheaply if you want to have those systems reliable, secure, connected, and able to evolve.
MN: At the Inselspital, we are currently building the EPIC system.
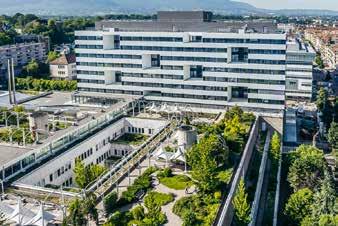
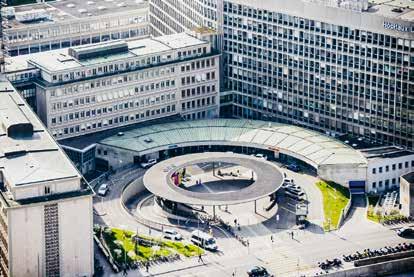
AG: I think it’s a brave choice in many ways, but it has pros and cons. When you buy something that is so much integrated, you get into a culture, you have to adapt the culture of your hospital to the system. Just like when you install SAP, you become an SAP enterprise.
And I think if you buy EPIC, you become an EPIC hospital. It helps in terms of standardization because you capitalize on a lot of experience. But it also changes the culture towards a much more, I would say, American view of what is healthcare. It is going to be a transformational opportunity for the hospital. But all those systems are working well enough that you can build on top new added value services for patients and professionals. I view those systems as infrastructure on top of which you
should be able to buy real added value tools. These tools are going to be scenario centered, user centered tools, mostly built on mobile devices, and mostly enabled by AI.
MN: What contribution can laboratory medicine make to improve healthcare in this context? I am not only thinking of new laboratory values with marginal additional benefit, but also faster processes, beside testing and decision support system that help in interpretation.
AG: That’s an interesting question. Obviously, producing the results in a reliable and digitized way is important. But going one step further and helping to answer the clinical question is very important. I think one of the questions is whether we should see this as a laboratory medicine issue or as part of a much more comprehensive diagnostic strategy that obviously involves the laboratory? My view and our view in Geneva is that we now have a diagnostic department that combines the different sources of diagnostic information, but also information technology and information sciences. The idea is that it is not just a service to clinicians but a partnership between clinicians and the providers of diagnostic information. But this is new territory, and I hope that we can find support for doing research in this area because that is really changing the perspective. Besides, I believe that it becomes even more important working on the lessismore approach. To make sure that we order what really makes sense rather than checking all the boxes on the order form.
MN: I want to move to the topic of telemedicine, one of your particular areas of interest. Why is this associated with particular potential for improving patient care?
AG: The ability to move expertise without moving people is critical for making better decisions because sometimes you don’t have the ability to make physical contact. We have seen in the COVID crisis that there are many situations in which added value can be generated in this way. Also, it enables care professionals in complicated situations to bring in additional expertise. In Geneva, there is a typical use case that we often see: nurses detecting outofrange glucose levels at the home of patients. Should I call for a physician?
«The ability to move expertise without moving people is critical for making better decisions because sometimes you don’t have the ability to make physical contact.»
But that’s difficult to get. Should I send the patient to the ER? But that can be complex. Or can I get advice using telemedicine? In 80% of the cases, we can actually solve the case without having to move the patient. It’s better for the patient, it’s better for the care professional, and it’s better for the healthcare system. However, currently there is no reimbursement.
MN: Further, young people don’t want to wait five or six weeks to see a specialist…
AG: Indeed. And if you combine that with AI, then you get very interesting possibilities. If you call your dermatologist and say, I have a strange thing
on my hand, when can I see you? The answer is probably in three months. If you say, I’ve taken a picture and the AI tells me there’s 90% chance it is a melanoma, when can I see you? It’s unlikely that the answer is going to be in three months. These systems will change the way we organize care for the better. Prioritize what needs to be prioritized.
MN: My last question is on healthcare in low and middle income countries, an issue you are particularly interested in. Does digitalization have any particular advantages there?
AG: If digitalization is about moving information and expertise without mo
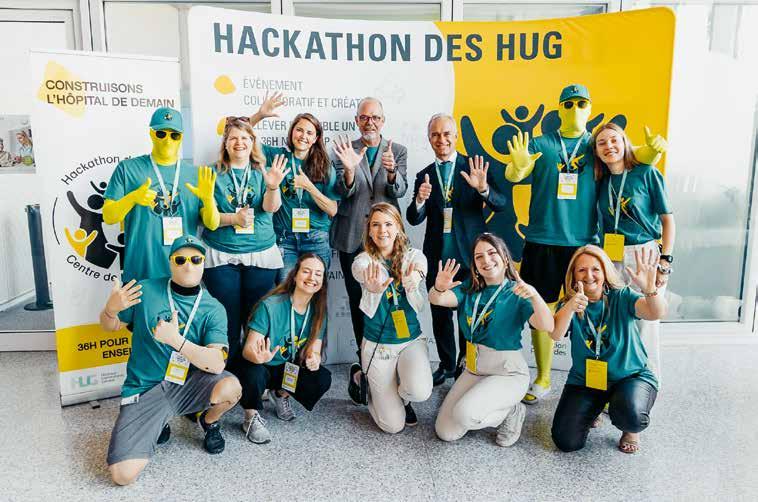
ving people, it makes a lot of sense. If you look at Mali, with a war in the north of the country, it can take several days just to get to a city where there is a specialist. That’s why we’ve started there. Twentyfive years ago, I wanted to implement telemedicine in Switzerland but nobody was interested – not even the patients. This development has started in Australia and Canada, where the distances are great. But now things have changed, also in Switzerland as we begin to be confronted with medical deserts.
MN: Professor Geissbuhler, thank you very much for the inspiring conversation. ●
«Healthcare is about processing information. It’s an informationintensive
Digital Health: Die Transformation unseres Gesundheitswesens muss einen Gang zulegen!
Ein clever digitalisiertes Gesundheitswesen ist das bessere System. In der Schweiz schreiten wir jedoch nur in kleinen Schritten voran. Warum ist das so? Das liegt nicht an unserem Können, jedoch etwas am Dürfen und sehr viel am Wollen.
Daten reibungslos zwischen zahlreichen Akteur:innen. Dabei kam es noch nie zu einem echten Supergau, zum Beispiel in der Hinsicht, dass Tausende Konten aus Versehen gelöscht wurden. Man muss nur mit den richtigen Anbietern zusammenarbeiten. ITErfolgsprojekte wie die CovidZertifizierung zeigen, dass dies auch im Gesundheitswesen möglich ist.
Dürfen: Zuckerbrot und Peitsche
Prof. Dr. Alfred Angerer, Management im Gesundheitswesen und Digital Health Lab, ZHAW
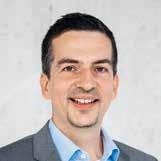
Platz 14 von 17. Dort ist die Schweiz im grossen DigitalHealthLändervergleich der Bertelsmann Stiftung (2018) gelandet. Und die seither gemachten Fortschritte sind nicht wirklich ermutigend. Kein Ruhmesblatt für ein Land, das ansonsten stolz davon erzählt, zum 12. Mal in Folge im UNInnovationsranking weltweit an Nummer eins zu stehen (WIPO, 2022). Warum sind wir einerseits Weltmeister in Sachen Innovationskraft und dann wiederum so rückschrittlich, sobald es um das Thema digitale Transformation des Gesundheitswesens geht? Mein Erklärungsansatz verläuft entlang der Dimensionen Können, Wollen und Dürfen.
Können: Die technischen Grundlagen sind vorhanden Die Technik ist nicht das Problem. Viele der Herausforderungen im Gesundheitswesen sind schon vor Jahren in der Industrie gelöst worden. Kann das Übermitteln von Patientendaten sicher erfolgen? Da muss man nur die Banken fragen. Sie übermitteln schon seit Jahren grosse Mengen an
Eins sollte klar sein: Digitalisierung kostet Zeit und Geld. Langfristig handelt es sich zwar um gut angelegtes Geld. Kurzfristig erhöht sie jedoch noch mehr den ökonomischen Druck, den viele Akteur:innen heute bereits verspüren. Für diese ist es entsprechend nicht attraktiv, in die Digitalisierung zu investieren. Ein Laborleiter oder eine Hausärztin beispielsweise haben kaum Anreize, ihre Organisationen zu digitalisieren, da praktisch nichts davon vergütet wird. Um das zu lösen, sind zwei Aktionen notwendig. Zum einen müssen Tarife angepasst werden, damit die einzelnen Leistungserbringer nicht allein für die Investitionen aufkommen müssen. Andererseits braucht es aber auch mehr Verpflichtungen, wie das Beispiel der fehlenden Interoperabilität zwischen den Hunderten von ITSystemen am Markt bisher zeigt. Hier ist ein konsequentes Eingreifen des Gesetzgebers vonnöten, der Standards und Richtlinien vorgeben sollte. Die berühmte «Zuckerbrot und Peitsche»Strategie scheint hier Erfolg versprechend zu sein.
Wollen: das Kernproblem
Das Thema Wollen ist unser Kernproblem in der Schweiz. Das können Sie empirisch testen, indem Sie auf der Strasse
Menschen fragen, wie schlimm sie es finden, dass wir seit 14 Jahren über das EPD diskutieren – und trotzdem gerade einmal 0,2% der Bevölkerung über eins verfügen (eHealth Suisse, 2023). Die Antwort wäre wahrscheinlich ein Schulterzucken. Denn wir haben es noch nicht geschafft, die breite Bevölkerung davon zu überzeugen, dass die digitale Gesundheitswelt aus Qualitäts und Effizienzgründen zu begrüssen ist. Und auch unsere Gesundheitsfachpersonen sind noch lange nicht alle überzeugt.
TREND HEALTH
Was tun? Kontinuierlich weiterarbeiten!
Die digitale Transformation ist ein Marathon und kein Sprint. Wir müssen diese systematisch vorantreiben –sprich Leuchttürme schaffen, den Nutzen klar messen, die Erfolge kommunizieren, auf die Gesetzgeber zugehen und klare Verbindlichkeiten fordern. Was wir nicht machen sollten, ist, einfach so lange zu warten, bis der Druck so hoch ist, dass wir handeln müssen. Denn als Feuerwehrübung mit dem Rücken zur Wand zu digitalisieren, war noch nie eine gute Idee. ●
Quellenverzeichnis
• Angerer, Alfred; Hollenstein, Eva; Russ, Christian (2021). Der Digital Health Report 21/22: die Zukunft des Schweizer Gesundheitswesens.
Winterthur: ZHAW.
https://doi.org/10.21256/zhaw2408.
• Bertelsmann Stiftung (2018). #SmartHealthSystems — Digitalisierungsstrategien im internationalen Vergleich.
https://www.bertelsmannstiftung.de/de/ unsere projekte/der digitale patient/projektthemen/smarthealthsystems.
• e Health Suisse (2023). Verbreitung des EPD in der Schweiz.
https://www.e healthsuisse.ch/ gemeinschaftenumsetzung/umsetzung/roadmap einfuehrung epd.html.
• World Intellectual Property Organization (WIPO) (2022). Global Innovation Index 2022: What is the future of innovation driven growth? Geneva. WIPO. DOI 10.34667/tind.46596 (225).
Santé numérique: la transformation de notre système de santé doit passer à la vitesse supérieure!
La numérisation du système de santé suisse ne progresse que très lentement par rapport à d’autres pays. Cet article énumère trois raisons à cela. 1. Ce n’est pas la technologie qui est en cause, les bases sont là. 2. Le cadre légal n’offre pas assez d’incitations (monétaires) pour les acteurs. Une véritable pression légale en faveur de la numérisation n’existe pas dans de nombreux endroits. 3. Il n’y a pas encore de pression sociale importante. Le grand public ne sait pas pourquoi un système de santé transformé par le numérique nous apporterait une meilleure qualité à des coûts moindres. C’est pourquoi l’appel est clair: nous devons créer davantage de phares numériques, communiquer largement les succès et modifier les lois de manière à ce que la numérisation devienne plus attrayante pour les fournisseurs de prestations, voire qu’elle ne soit plus une alternative. ●
Die Vielfalt von Digital Health: Das WIG Ordnungsmodell. Quelle: Angerer et al. (2021)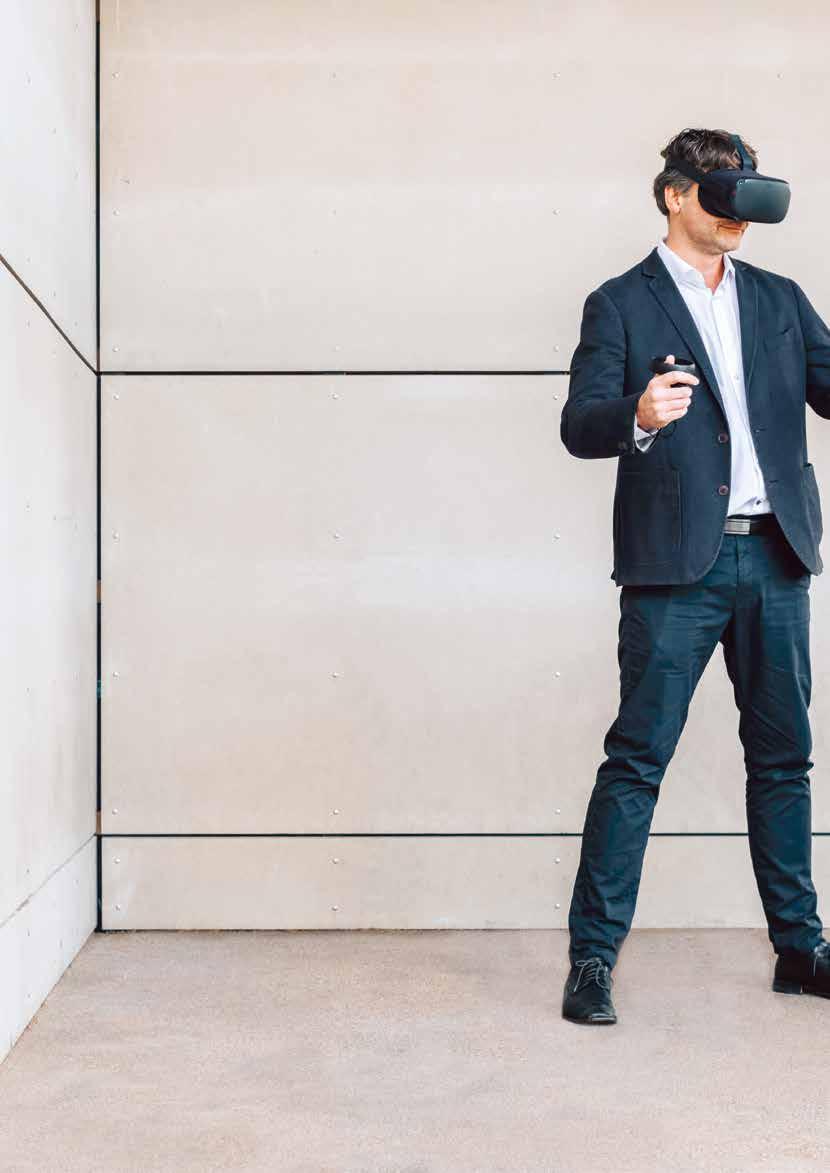
«Es ist wichtig, dass wir als Gesund heitsfachpersonen die Entwicklungen mit gestalten und sie nicht einfach von der Industrie oder der Technik aufgezwungen bekommen.»
Thomas Sauter ist Professor für Telemedizin und e-health am Inselspital Bern. Um die neuen Möglichkeiten sinnvoll nutzen zu können, braucht es spezialisierte Ärzt:innen, die a) die Bedürfnisse der Medizin mit den Technikpartnern austauschen, b) bei Gesundheitsfachpersonen das Bewusstsein für Möglichkeiten und Grenzen herstellen («digital literacy»), und c) die wissenschaftliche Evidenz zu den neuen Instrumenten generieren. Insbesondere die Telenotfallmedizin kann helfen, zukünftige Versorgungsdefizite durch Fachkräftemangel zu mildern. In innovativen Versorgungsmodellen könnten so beispielsweise Advanced Nurse Practitioners oder Rettungssanitäter medizinisch unterstützt werden. Wenn Eltern kleiner Kinder einen telemedizinischen Rat holen können, entlastet das nicht nur die vollen Notfallstationen, sondern auch die Nerven der Eltern. Unterstützt wird die Patientenversorgung zukünftig aber auch durch Smartphones, sogenannte «wearables» und sofort verfügbare Patientendaten.
Thomas Sauter est professeur de télémédecine et de cybersanté à l’Hôpital de l’Île de Berne. Pour pouvoir utiliser judicieusement les nouvelles possibilités, il faut des médecins spécialisés qui (a) échangent les besoins de la médecine avec les partenaires techniques, (b) sensibilisent les professionnels de la santé aux possibilités et aux limites («digital literacy») et (c) génèrent les preuves scientifiques concernant les nouveaux instruments. La télémédecine d’urgence, en particulier, peut contribuer à atténuer les futurs déficits de soins dus au manque de personnel qualifié. Dans des modèles de soins innovants, les infirmiers avancés ou les ambulanciers, par exemple, pourraient ainsi bénéficier d’un soutien médical. Si les parents de jeunes enfants peuvent demander un conseil par télémédecine, cela soulage non seulement les services d’urgence pleins à craquer, mais aussi les nerfs des parents. Mais à l’avenir, les soins aux patients seront également soutenus par les smartphones, les «wearables» et les données des patients immédiatement disponibles.
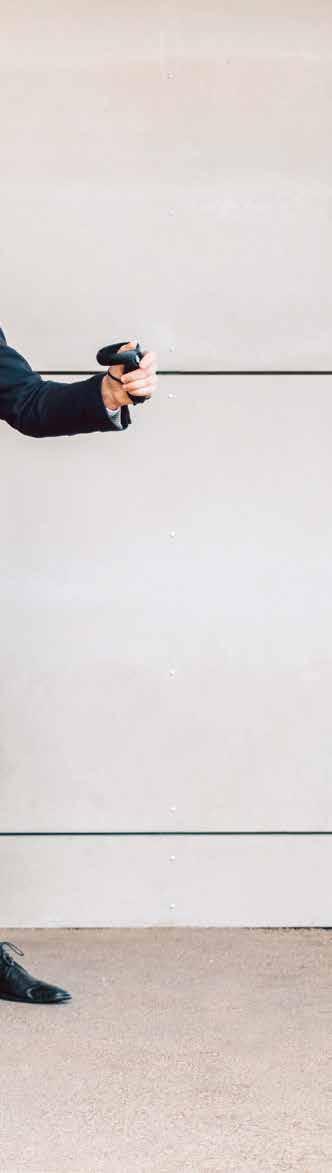
Hören und lesen
Podcast Theranos:
Betrug im Silicon Valley
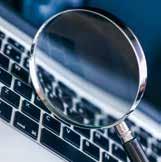
Und nochmals Theranos: Wer noch nicht in den wahrscheinlich spektakulärsten Betrug der Labormedizin eingestiegen ist, kann das in knapp zwei Stunden in PodcastForm tun. Spektrum der Wissenschaft hat den Fall auf Deutsch aufbereitet:
Zum Artikel: sulm.ch/23/i13
Der AI Gesund heitspodcast
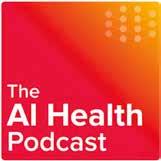
Wie wird AI die Gesundheitsversorgung, die Medizintechnik und die Medizin verändern? In Gesprächen mit Unternehmern, Investoren und Wissenschaftlern ergründet der folgende Podcast diese Fragestellung:
Zum Artikel: sulm.ch/23/i15
Click here for better chemistry
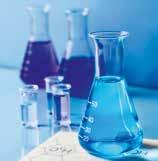
Click chemistry and biorthogonal reactions enable the formation of a specific product in a highly complex chemical environment. Thus, they allow a toolbox for biological studies and medical applications. The 2022 Nobel laureates Bertozzi, Meldal, and Sharpless explain how this is accomplished in an article in the New England Journal of Medicine.
Towards the article: sulm.ch/23/i14
Teilen Sie Entdeckungen mit uns!
Möchten Sie ein Buch oder einen Podcast in der «pipette» veröffentlichen? pipette@sulm.ch
Écouter et lire
Atellica® CI 1900
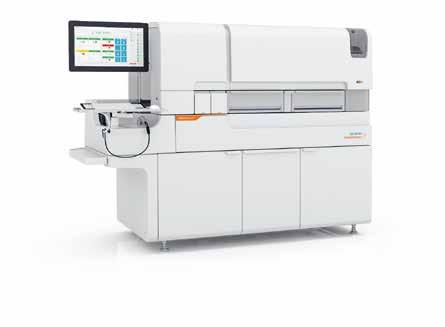
Mit einer Stellfläche von 1,9 m2 wurde der Atellica® CI 1900 Analyser speziell für mittlere und kleinere Laborumgebungen entwickelt und nutzt die gleiche Technologie und intelligente Software wie unser Flaggschiff Atellica® Solution.
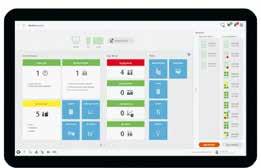
Podcast Theranos: la fraude dans la Silicon Valley
Et encore une fois Theranos: ceux qui ne sont pas encore entrés dans ce qui est probablement l’escroquerie la plus spectaculaire de la médecine de laboratoire peuvent le faire dans près de deux heures sous forme de podcast. Spektrum der Wissenschaft a traité l’affaire en allemand: sulm.ch/23/i16
Le podcast sur la santé d’IA
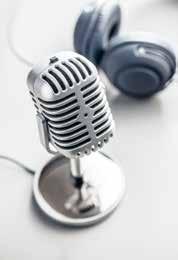
Comment l’IA vatelle changer les soins de santé, la technologie médicale et la médecine? Le podcast suivant explore cette question à travers des entretiens avec des entrepreneurs, des investisseurs et des scientifiques: sulm.ch/23/i17
Intuitive und einfache Benutzeroberfläche
Integration von häufig benötigten Laborfunktionen, um den Einarbeitungsaufwand zu minimieren und einen optimalen Arbeitsablauf zu gewährleisten.
– Einfachheit unabhängig vom Trainingsstand
Es sind keine besonderen Softwarekenntnisse erforderlich, um grundlegende Aufgaben zu bearbeiten. Benutzer:innen mit unterschiedlichen Vorkenntnissen erhalten die Sicherheit, dass sie Routineaufgaben zuverlässig ausführen können.
– An Ihr Labor anpassbare Navigation und Warnmeldungen Passen Sie die Navigation der Benutzeroberfläche an Ihr Labor an, und legen Sie fest, welche Benachrichtigungen ans Smartphone gesendet werden sollen
– Lab Evaluations

Reagenzlotvergleich, QC Parallelmessung oder auch TagfürTagWiederholbarkeit. Dies und vieles mehr ist fester Bestandteil der Atellica User Software und bietet standardisierte Unterstützung sowie Auswertung nach CLSIEP15 Richtlinien.
Weitere Informationen: siemens-healthineers.ch/atellica-ci1900
Vorschau Feedback
Qualität
Qualität ist etwas, das uns im medizinischen Labor und in der gesamten Laborbranche antreibt. Das ist nicht nur Anspruch an uns selbst, sondern es wird von den Behörden, der Politik, den Ärzten und den Patienten gefordert. Aber was bedeutet das eigentlich? Diesen Fragen geht die nächste Ausgabe der «pipette» nach.
Qualité
La qualité est quelque chose qui nous motive dans le laboratoire médical et dans l’ensemble du secteur des laboratoires. Ce n’est pas seulement une exigence pour nousmêmes, mais c’est aussi une exigence des autorités, de la politique, des médecins et des patients. Mais cela signifietil vraiment quelque chose? Le prochain numéro de la pipette se penchera sur ces questions.
Was wollen Sie in einer nächsten Ausgabe lesen?
Schreiben Sie uns: pipette@sulm.ch
Herausgeberin: SULM Schweizerische Union für Labormedizin, c/o Solothurner Spitäler, Labormedizin, Schöngrünstrasse 36A, 4500 Solothurn Tel. 032 627 31 21, philipp.walter@spital.so.ch, sulm.ch
Chefredaktion: Prof. Dr. Dr. Michael Nagler, c/o Inselspital, Zentrum für Labormedizin, 3010 Bern
Adressänderungen: pipette@sulm.ch
Wir laden Sie ein, die «pipette» mitzugestalten.
Teilen Sie uns mit, was sie vom Relaunch halten. Ausserdem interessiert uns, was Sie bewegt, was Sie in der Schweizer Labormedizin verändern und welche Themen Sie in den künftigen Magazinen publiziert haben möchten.
Ihr Feedback erreicht uns unter: pipette@sulm.ch
Haben Sie eine neue Adresse oder Fragen zu Ihrem Abonnement? Dann wenden Sie sich an: pipette@sulm.ch
Hier abonnieren Sie
die Printausgabe: sulm.ch/de/pipette/ueber-die-pipette
Redaktionsmitglieder: Dr. Roman Fried; Prof. Dr. Gilbert Greub; Prof. Dr. A. Leichtle; Dr. Dr. Elsbeth Probst Müller; Marianne Schenk; Dr. Véronique Viette; Dr. Corinne Widmer
Konzept, Design und Gesamtherstellung: Stämpfli Kommunikation Wölflistrasse 1 3001 Bern staempfli.com
Auflage: 7814 Exemplare
Redaktionsbegleitung:
Stämpfli Kommunikation, Bern
Monica Masciadri Tel. 031 300 63 85 monica.masciadri@staempfli.com
Mediavermarktung:
Stämpfli Kommunikation, Bern
Michèle Bachmann, Telefon +41 31 300 63 70 mediavermarktung@staempfli.com
SSCC/SGKC 2023 Annual Assembly
The SSC/SGKC 2023 annual meeting will be held from October 4 to 6 in Crans Montana (Valais), at "Le Régent" Congress center.
The theme of the meeting will be Hormones, Toxicants and the Environment - topical issues of great importance to the medical laboratory that will surely generate exciting debate.
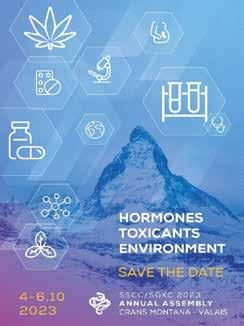
Visit the SSCC 2023 Congress website ww.sscc2023.ch to find the detailed programme and all the information you need to register and to submit abstracts .

In order to get the hotel category you want, we recommend that you book your accommodation in advance.
The members of the Congress Organizing Committee look forward to welcoming you to Crans Montana
Nouveau
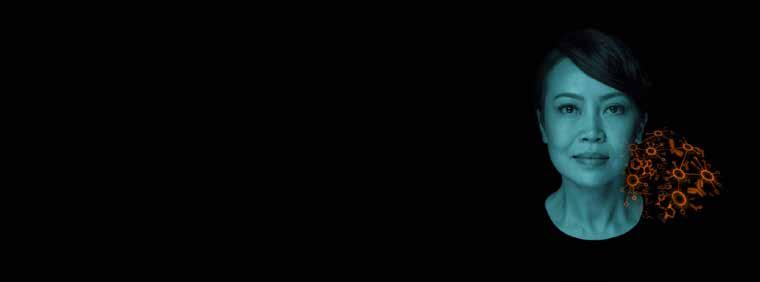
Atellica® CI 1900 Analyser
La solution clinico-chimique et immunodiagnostique spécialement prévue pour les laboratoires petits et moyens
• Faible encombrement – seulement 1,9m2
• Technologie, réactifs et consommables identiques à ceux d’Atellica® Solution
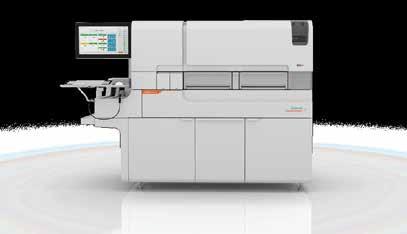
• Vaste menu de tests – plus de 200 tests pour les pathologies les plus variées
Venez nous rendre visite du 14 au 16 septembre. au SGKC, stand n° xx.
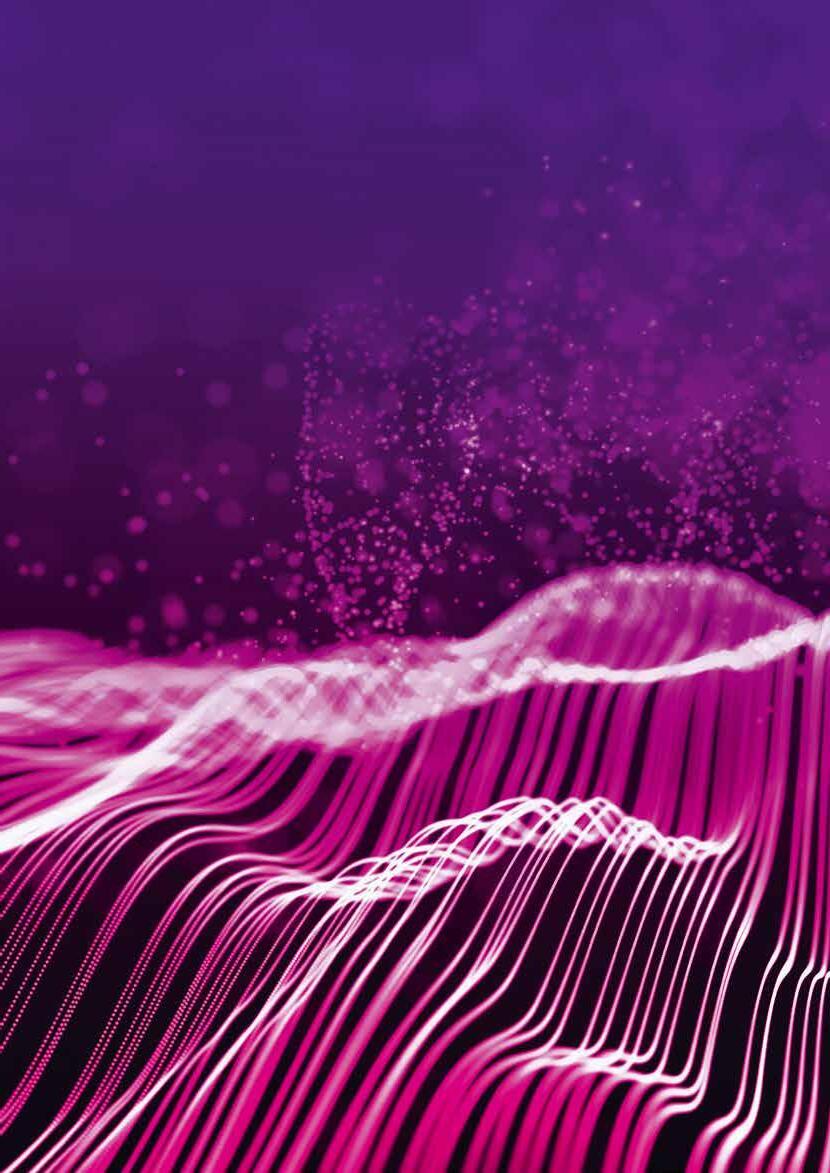