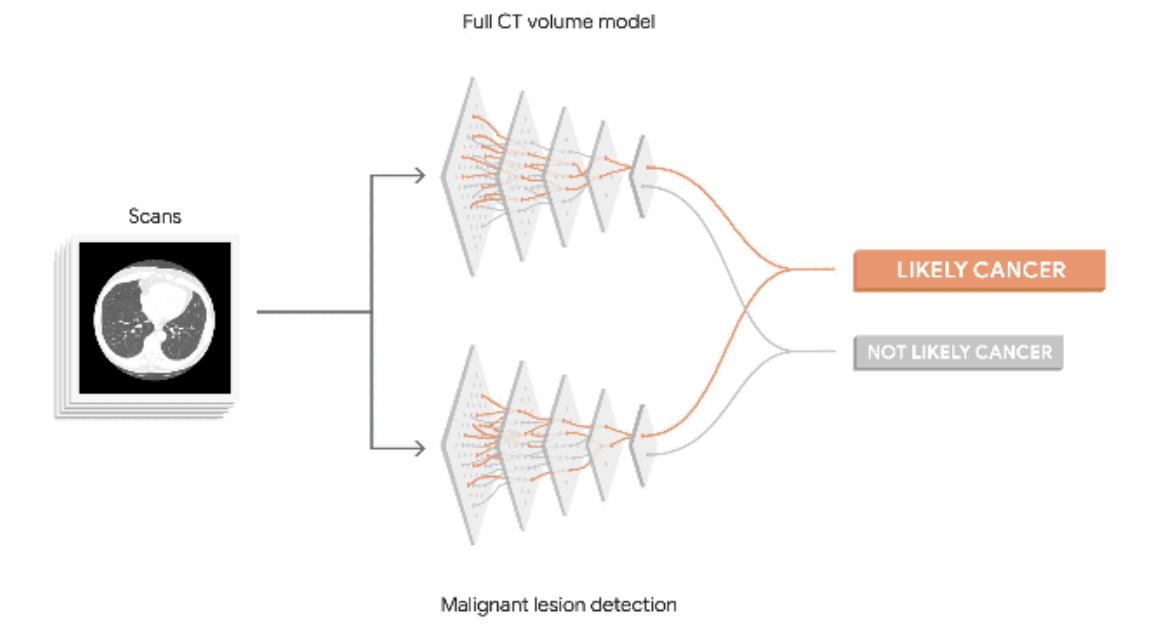
6 minute read
GOOGLE'S DEEP DIVE INTO HEALTHCARE USING AI
By Chloe Palumbo Esther Beck
Alan Turing’s 1950 paper, Computer Machinery and Intelligence, introduced a simple yet novel question: Can machines think? His answer came in the form of the Turing Test, which sought to determine whether a computer could reliably pass as a human when prompted with a series of questions. Turing’s work set the precedent for artificial intelligence by bringing the question of whether machines can exhibit human intelligence to the fore.
Advertisement
But what exactly is artificial intelligence? For many, the term “artificial intelligence” conjures up images of machines taking over the world in some sci-fi apocalypse. Despite all the buzz about artificial intelligence in the past decade, most people still only have a vague understanding of what exactly artificial intelligence is and the role it plays in modern society. At the most rudimentary level, artificial intelligence is the act of replicating human intelligence in computer systems. Whether it be through speech-recognition or decision making, artificial intelligence has made profound leaps in industries as wide-ranging as automobiles and healthcare.
One such advance came in 2008 after Google launched their Google Health project, marking the commencement of their venture into the healthcare industry. Originally designed to allow Google users to centralize their health records, the Google Health project has shifted its focus in recent years to artificial intelligence research.
One predominant focus of the Google Health project is Acute Kidney Injury (AKI) prevention. At a staggering 13.3 million cases worldwide per year, AKIs pose a significant global health threat by causing kidney failure in as less as two days due to the accumulation of waste products in the blood. If diagnosed early enough, experts believe that up to 30% of AKIs are treatable, but early detection systems have plenty of room for improvement. The DeepMind team at Google Health seized this opportunity to initiate a research project that employs artificial intelligence in order to predict AKIs as quickly as 48 hours before they strike. To do this, they’re testing a neural network that processes electronic health records at certain time steps and outputs the probability of AKI development within the next 48 hours. Any probability above a certain threshold indicates a positive result and will notify clinicians as soon as possible. Additionally, the model provides a measure of uncertainty with each prediction that allows clinicians to distinguish between more and less ambiguous cases. The artificial intelligence technology is so accurate, in fact, that it correctly detected AKI in 90% of patients with conditions that became so severe they required dialysis.
In addition to acute kidney injuries, Google Health’s artificial intelligence technology has ventured
into oncology, tackling lung cancer diagnosis using advances in 3D volumetric modeling. Rather than having radiologists sift through hundreds of 2D images per single CT scan, which is both time-consuming and often prone to errors, Google Health’s artificial intelligence technology uses a patient’s current CT scan as well as any previous scans to generate an overall malignancy prediction and to identify subtle malignant tissues. So far, the results have been very promising, with artificial intelligence technology performing at the same proficiency, if not better, as U.S. board-certified radiologists. In fact, an additional 5% of cancer cases were detected using artificial intelligence technology.
Google Health artificial intelligence technology has also ventured into blindness detection caused by diabetic retinopathy. Diabetic retinopathy induces lesions towards the posterior of the retina and poses a risk to 415 million diabetics worldwide. Luckily, with early diagnosis and consistent screening, such occurrences can be prevented. What’s most widely used by ophthalmologists is optical coherence tomography (OCT), a detailed 3D image of the back of the eye, to diagnose eye diseases. However, OCT images require lengthy expert analysis for them to be accurate. The sheer quantity of scans alone in hospitals worldwide creates delays between the scan and treatment that can cost a patient their eyesight. Google Health’s artificial intelligence model was constructed in a stepwise fashion. Firstly, the model was equipped with anonymous pre-labeled retina images by over 50 ophthalmologists which were rated on a scale from 1 to 5, depending on the quantity and severity of diabetic retinopathy signs present. These pre-labeled images were then inputted into an image recognition algorithm so that the model could identify certain features indicative of diabetic retinopathy, including nerve tissue damage, swelling, and hemorrhaging. After the model was trained on a large enough data set, it could then be transferred to a device, termed the Automated Retinal Disease Assessment (ARDA) in which retina images are accepted as inputs and analyzed for signs of diabetic retinopathy. After detection, the program informs physicians which patients are in need of treatment and corroborates its decision with specific features that provide insight into how it came to its decision, as well providing the percent confidence in its recommendation. In recent trials, Google Health’s artificial intelligence technology could detect eye diseases in a matter of seconds.
Promising results in a lab is one thing, but whether that can translate into a clinical environment is another. One of the first instances of ARDA’s implementation in a clinical setting was in Thailand, where
Modeling framework for Google’s artificial intelligence lung cancer detection technology.
a total of 4.5 million diabetics were screened by only 200 retinal specialists. After its incorporation, nurses claimed that although it sped up the process significantly, ARDA would often return no result at all. Part of this phenomenon can be explained by the fact that during the training process only the highest quality scans were used in an attempt to guarantee higher accuracy. While that worked in early trials, when it came to ARDA’s application in a clinical setting, scans that were not up to its quality standards were rejected. In fact, a staggering 20% of scans were rejected due to low quality. If artificial intelligence technologies are ever to be successfully incorporated in real-world clinical settings, more rigorous processes need to be implemented to ensure that scans ranging in quality can be analyzed for successful diagnosis.
There is no doubt that artificial intelligence holds immense potential for changing the healthcare industry as we know it. Promising results serve as a testament to the incredible power of computer systems in detecting and diagnosing diseases, thereby reducing time delays and potentially making the difference between life and death. However, rigorous trials must be conducted before it can gain regulatory approval. Translating encouraging laboratory results to a clinical setting is a pertinent requirement before artificial intelligence can truly become a fixture of the healthcare industry.
“A Major Milestone for the Treatment of Eye Disease.” Deepmind. Accessed
November 13, 2020. https://deepmind.com/blog/article/moorfields-majormilestone. “Acute Kidney Injury.” International Society of Nephrology, August 25, 2020. https://www.theisn.org/commitment-to-kidney-health/focus-areas/acutekidney-injury/. “Applying Deep Learning to Metastatic Breast Cancer Detection.” Google AI
Blog, October 12, 2018. https://ai.googleblog.com/2018/10/applying-deeplearning-to-metastatic.html. “Diagnosing Diabetic Retinopathy with Machine Learning.” Google. Accessed
November 13, 2020. https://about.google/stories/seeingpotential/. “Google Health.” Wikipedia. Wikimedia Foundation, November 12, 2020. https://en.wikipedia.org/wiki/Google_Health. Heaven, Will Douglas. “Google's Medical AI Was Super Accurate in a
Lab. Real Life Was a Different Story.” MIT Technology Review. MIT
Technology Review, April 27, 2020. https://www.technologyreview. com/2020/04/27/1000658/google-medical-ai-accurate-lab-real-life-cliniccovid-diabetes-retina-disease/. Shravya Shetty, M.S. “A Promising Step Forward for Predicting Lung Cancer.”
Google. Google, May 20, 2019. https://blog.google/technology/health/ lung-cancer-prediction/. Shravya Shetty, M.S. “Using AI to Improve Breast Cancer Screening.” Google.
Google, January 1, 2020. https://blog.google/technology/health/improvingbreast-cancer-screening/. Tomašev, Nenad. “A Clinically Applicable Approach to Continuous Prediction of Future Acute Kidney Injury.” Nature News. Nature Publishing Group,
July 31, 2019. https://www.nature.com/articles/s41586-019-1390-1. Turing test. (2020, October 29). Retrieved November 13, 2020, from https:// en.wikipedia.org/wiki/Turing_test “Using AI to Give Doctors a 48-Hour Head Start on Life-Threatening Illness.”
Deepmind. Accessed November 13, 2020. https://deepmind.com/blog/ article/predicting-patient-deterioration. “What Is Artificial Intelligence? How Does AI Work?: Built In.” What is
Artificial Intelligence? How Does AI Work? | Built In. Accessed November 13, 2020. https://builtin.com/artificial-intelligence.