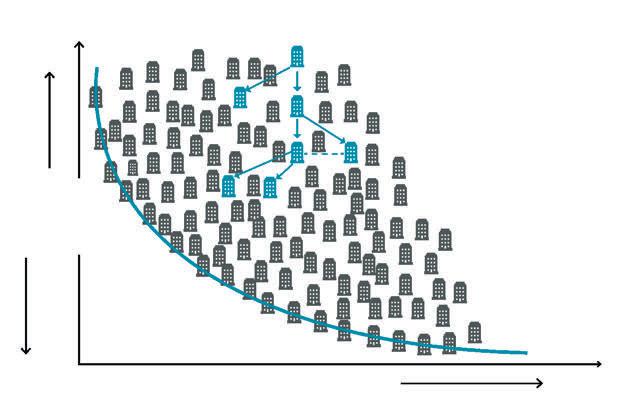
5 minute read
simulation
How AI is impacting building simulations
Dr Parag Rastogi, lead building physicist at proptech firm arbnco, explores how the power of Artificial Intelligence (AI) and machine learning can be harnessed to optimise energy efficiency in buildings
Artificial Intelligence and machine learning are increasing in prominence in the building, architecture and construction sectors. One area that is ideally suited to take advantage of these powerful new technologies is building energy simulation. There are various reasons why building energy modelling is a vital resource. Whether it is to demonstrate compliance with legislation such as the Minimum Energy Efficiency Standards (MEES) through EPCs, increase energy efficiency, or investigate poor performance, the ability to simulate what-if scenarios is crucial to informed decision-making.
The current applications of AI on the market now do not replace human judgement. Rather, where AI comes into play is the ability to run millions of calculations in seconds to ensure feasible options are not disregarded for lack of time or resources for analysis.
Removing laborious manual calculations frees up ener-
Applying machine learning to retrofit exploration
My interest in applying machine learning to building simulation stems back to my extended research stay at the RIKEN Institute for Advanced Intelligence Project in Tokyo, Japan, where I collaborated with Dr Mohammad Emtiyaz Khan, a world-leading researcher in data science and machine learning.
In August 2017, I was given the opportunity to build upon our experiments thanks to funding from The Data Lab, an innovation centre focused on helping Scottish industry collaborate with public sector and universities to promote the use of data science and artificial intelligence.
The nine-month research project enabled building energy simulation company arbnco to work with the Department of Architecture at the University of Strathclyde to understand how best to introduce AI in building simulations.
The project focused on the arbn consult platform software which provides the ability to quickly and accurately assess the Energy Performance Certificate (EPC) rating of a commercial property, producing bespoke and fully-costed retrofit packages for delivering improvements in energy performance.
The software, based on the industry-standard Simplified Building Energy Model (SBEM), tracks regulated energy loads, fixed building services such as heating, cooling, lighting, controls, ventilation, hot water, fuel and refrigerants.
Using this energy model, the platform delivers an analysis of commercial real estate improvements to determine retrofit strategies for mitigating risk and improving financial performance of commercial real estate.
Project outcomes Our team of researchers combined expertise in building science, machine learning, automation in construction, software, and building G
EPC rating Energy use [kWh/m 2 ]
A+
simulation. Over the course of the project we explored ways that machine learning can be used in tandem with building simulation software to make quicker, more informed decisions.
The research project enabled arbnco to upgrade its existing retrofit-exploration software arbn consult. The Cost of renovation
arbn consult software offers users the possibility of a Multi Objective Optimisation, taking into account both EPC ratings and costs to retrofit commercial properties. Users can input constraints such as cost or payback period to determine the optimum retrofit strategy. arbnco’s product offering Designs explored manually Pareto front
All possible designs
is currently based on UK compliance software. Going forward, it intends to apply this research to dynamic energy modelling software such as Energy Plus, developed by the US Department of Energy, and ESP-r, developed by the University of Strathclyde, to increase global usage.

gy assessors to use their time to greater Conscious & unconscious bias Every facilities manager and energy asseseffect, creating more value for the end cli- Given the hundreds of variations and sor has different knowledge and experient. It would be impossible to build a per- thousands of combinations to consider in ence, and with that comes blind-spots, fect energy model of every individual any given building, energy managers use which this process could help reduce. commercial property, and every renova- their experience and knowledge to make tion option. Collecting and analysing recommendations on retrofit strategies. So, is AI really coming for our jobs? data at scale is an expensive and slow When doing so they are making judge- AI isn’t perfect, it certainly doesn’t replace undertaking, even if the data exists. ments based on unconscious and con- judgement or engineering education; often Ultimately, therefore, AI enables scalability. The promise of AI is that it solves the bulk of ‘‘ Ultimately, AI and machine learning are new the first piece of advice given to engineers exploring AI is that if you put garbage in, then you will the problem - processing mathematical tools that must be adapted for get garbage out. Data 80% of the options can purpose. It isn’t a case of simply introducing them quality and quantity are allow the user to focus in on the 20% - ultimately into the analysis and expecting a miracle both crucial. Where its strength lies increasing efficiency and using their expertise to greatest effect. AI will be an important scious bias - for example, making the ’’ is in identifying patterns faster, with potentially better accuracy. Ultimately, AI and lever to unlock the potential of building assumption that a particular retrofit machine learning are new mathematical physics to improve design, engineering, strategy will succeed because it had a pos- tools that must be adapted for purpose. It and architecture. Digitising the shelves itive impact in a previous similar project. isn’t a case of simply introducing them into and shelves of documents about build- By running a very large number and the analysis and expecting a miracle. In any ings will mean that every element of a combination of options and strategies, AI case, the smartness and appropriateness of building becomes more searchable and and machine learning can help remove the recommendations is irrelevant if they accessible, making problems easier to that bias, suggesting options the assessors are not acted upon in the physical world! detect, locate and solve. themselves may not have thought of. ■ arbnco.com
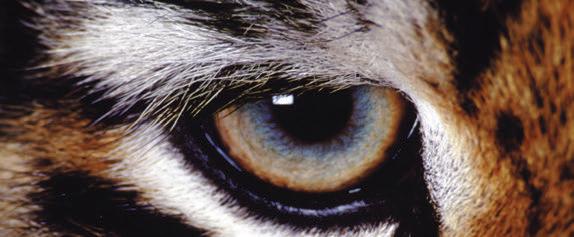
