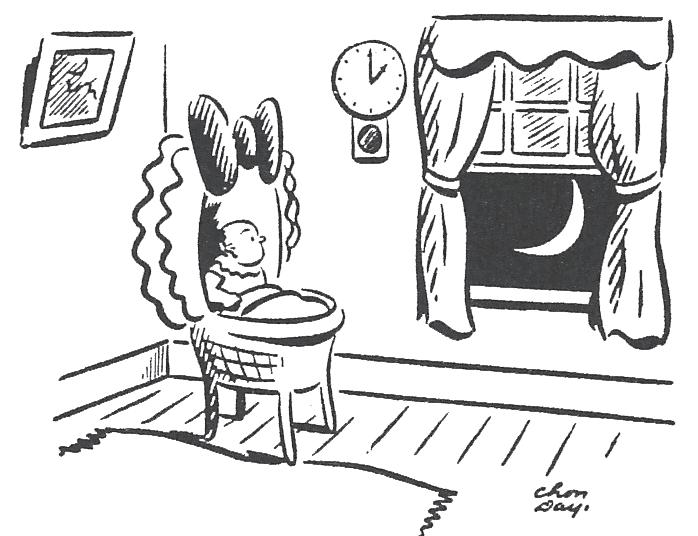
8 minute read
... but this is wondrous strange (Hamlet, Act 1, Scene 5, line 171)
By Vessela Ensberg (Associate Director, Data Architecture, University of California Davis Library) and Peter Brantley (Director, Online Strategy, University of California Davis Library)
Excitement and apprehension have been surrounding the explosion of accessible generative AI in the last few years. As we finished putting together this issue, the field of generative AI remains very dynamic. We tried to capture how the latest development affects libraries, and the vision, challenges and opportunities of how libraries can affect that development.
Before we dive into what is probably the most discussed technology today, it may be helpful to reflect on evolving perceptions around similar groundbreaking innovations as a guide to managing generative AI. When the library community encountered Google search and Wikipedia, some of the initial reactions may have included skepticism and caution. Today, Google Scholar is considered a good place to initiate a search, and libraries have set up Wikipedia editathons and hired Wikidata librarians. The initial assessments of the tool limitations were right at the time, but perhaps the potential was not sufficiently appreciated. With any new technology, it is helpful to assess what functionality is needed to move it from limiting to useful, and make a strong case for prioritizing that.
There are signs that we may be descending into the “Trough of Disillusionment” of the hype cycle1 for AI; professionals seem to have moved from being surprised by hallucinations to checking for them, and from praise to growing criticism. In this issue we want to acknowledge generative AI’s limitations and at the same time recognize its potential.
Some of the disillusionment may be due to misunderstanding of what can be expected of AI models. Many of our writers touch on the general workings of generative AI, but it’s worth summarizing the key concepts here. Generative AI is a complex neural network algorithm that predicts what element is most likely to belong in a sequence.2 For language models, the elements are words; for diffusion models, they are pixels. The statistics are derived from training datasets — collections of texts and/ or images–and the model outputs result in novel, often fascinating–texts and/or images. The bigger the model, the more data and computational power are required to train it and the higher the environmental and financial costs. The datasets and their inherent biases, discussed in more detail in Lucy Lu Wang’s article, are a consistent source of concern. Finally, the models are non-deterministic, which means that answers to the same question or prompt will vary to some degree, and there are parameters that can be modified by users to make them more or less so. Because of the probabilistic nature of the algorithms, they are prone to making statements that may not reflect reality and facts.3 The complexity of the models makes it difficult, if not impossible, to understand how they derived a particular output. It is as if we arrived at the answer “42” in the “Hitchhiker’s Guide to the Galaxy”; the question remains to be computed. Reducing hallucinations and understanding model outputs is in itself a research field. Generative AI is used in a number of disciplines from finance to medicine, and recently researchers in clinical imaging have made some progress in understanding breast cancer risk forecasts.4 Successful application of the methods in other models will be a significant advancement in understanding their outputs. Meanwhile, adding retrievalaugmented generation to the model is an increasingly popular approach to try to establish the provenance of an output. The approach provides an additional set of documents to the algorithm to enhance inferencing on the source material.5 However, the original training and the resulting parameters are still defining the model, so while hallucinations are reduced, they are not eliminated.6 This result is disappointing if the models are expected to perform perfectly, but, like Google search and Wikipedia, generative AI is a tool, and the way to deal with failings of a tool is to understand and attempt to fix them, rather than reject the tool altogether.
To use a different metaphor: cars are dangerous. If you have a teenager, or if your neighbor does, you probably had an uneasy feeling when they got behind the wheel. But you likely still own a car, and feel better that between 1886 and now cars have been equipped with seatbelts, and airbags, and engine control units. The authors in this issue flesh out the safety equipment for generative AI as they reflect on how libraries should work with the tool.
Several of our authors remark on the value of curation and evaluation of content. Gary Price explores the challenges and opportunities for our community to develop “driver’s ed” for AI. His piece focuses on what it takes to achieve trustworthiness of output. His advice to information professionals is to focus on life-long learning and to embrace curation, advocacy and verification. Lucy Lu Wang makes a complementary case in her piece of “Generative AI for Scholarly Information Access,” emphasizing the connections libraries foster with their communities as crucial for equitable curation. Her article addresses concerns about biases introduced through training materials.
Similarly, Lorcan Dempsey urges libraries to actively engage with shaping the norms around AI use. He also invokes curation of collections as a major leverage point in directing and correcting the course of development of generative AI. After all, if John Wiley & Sons can receive $23MM for the use of its collection for AI model training,7 what possibilities might arise if libraries (and publishers) coordinated efforts? What if libraries defined clear criteria and expectations for models they would be willing to endorse? What is our responsibility in reducing perpetuation of bias? Treating AI as a tool means to accept a challenge and a responsibility: seeing AI’s potential is on us.
In his article “Some Next Generation Legal Issues for Training Generative Technologies,” Brandon Butler boldly goes into mapping the legal landscape around training AI on copyrighted works. His discussion of the pending copyright infringement lawsuits against generative technology companies brings into focus another concept of fundamental importance to libraries: fair use. Brandon outlines the future impact that the outcomes of such lawsuits can have on organizations beyond the original litigants.
Francesco Ramigni gives us another glimpse of what the future may hold through his vision of contextualized search. In his piece “Visualising Collection Records With The Use Of Generative AI,” Francesco describes the integration of content from different types of media to transform the catalog from a list of records to a collection of trailers. He walks us through some of his own experimentation and outlines the long road to achieving his vision.
Shawn Averkamp’s motivational recount of her experience in working with libraries on incorporating machine learning into library operations encourages “Embracing Serendipitous Innovation in Libraries.” Her recipe of approaching work with AI tools as an experimentation, with curiosity and open mind, and most-importantly, with human-centric design, may be the key to unlocking that potential.
When reading about the challenges in getting the right output from AI tools, it may be helpful to take Gary’s advice on looking at how other fields use AI. The machine-learning algorithm AlphaFold2, which predicted protein structure with close to 90% accuracy,8 enabled the field of structural biology to leap into the previously impossible. The Levinthal’s paradox of protein structure was that it would take longer than the age of the universe to arrive at it through brute-force sampling.9 Reflecting on this feat is a motivation to keep exploring new applications for AI. If we choose carefully the seemingly impossible transformations that AI tools enable, we may very well be looking at a new golden age for collections, resurrecting people and their stories.10 As we readied this editorial, the Association of Research Libraries and the Coalition for Networked information published a report on AI scenarios, one of which explores this resurrection as an aptly named application LAZARUS.11
There are many other topics and visions that belong in this issue. We wish we could have had a discussion on the ability of AI tools to synthesize ideas at scale or to see connections in disparate contexts. The effect those functionalities will have on the perception of creativity may be similar to the way photography both supported the development of realism as an artistic movement 12 and catalyzed the emergence of impressionism.13 One cannot but wonder what the equivalent of Monet’s “Sunrise” and Pissaro’s “Boulevarde Montmartre” will look like in the wake of generative AI. We plan to reread this issue, with some trepidation, in a year to see what has come to pass and what else we have missed.
Meanwhile, we invite you to immerse yourself in the insights our authors have to share today. They approach generative AI tools with eyes open to their deficits, but also with many ideas on the roles for librarians and libraries that they open up. They identify the need for visionaries and trustworthy curators who represent community interests, whose expertise spans subject knowledge and technology, who build training collections, who explain benefits and pitfalls of using artificial intelligence for a particular task, and who are sensitive to serendipity. We hope you find their insight useful, and even inspiring.
Endnotes
1. https://www.gartner.com/en/research/methodologies/ gartner-hype-cycle
2. https://medium.com/@reyhaneh.esmailbeigi/bert-gptand-bart-a-short-comparison-5d6a57175fca
3. https://link.springer.com/article/10.1007/s00405-02308337-7
4. https://pubs.rsna.org/doi/10.1148/radiol.232780, https:// pubs.rsna.org/doi/10.1148/radiol.240474
5. https://medium.com/enterprise-rag/an-introduction-torag-and-simple-complex-rag-9c3aa9bd017b
6. https://arxiv.org/html/2404.08189v1
7. https://finance.yahoo.com/news/q3-2024-john-wileysons-043941097.html
9. https://faculty.cc.gatech.edu/~turk/bio_sim/articles/ proteins_levinthal_1969.pdf , https://misciwriters. com/2017/03/14/computing-levinthals-paradox-proteinfolding-part-2/
10. Some interesting work came close to exploring that at the Fantastic Futures 2023 conference.
11. https://www.arl.org/wp-content/uploads/2024/05/ARLCNI-2035-AI-Scenarios-5May2024.pdf
12. https://www.theartstory.org/movement/realism/
13. https://kiamaartgallery.wordpress.com/2015/05/05/ impressionism-the-influence-of-photography/