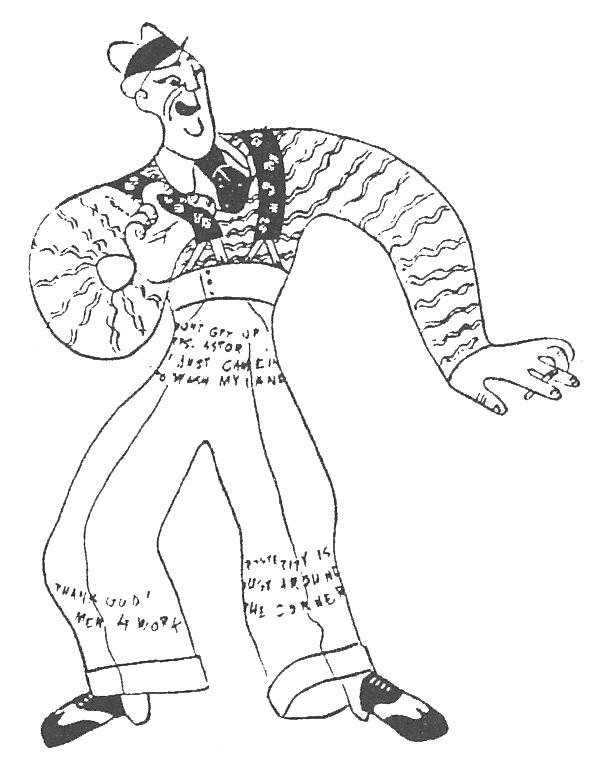
12 minute read
Embracing Serendipitous Innovation in Libraries
By Shawn Averkamp (Director, R&D Services, AVP)
If you’ve experimented with AI for specific tasks, like writing your journal article or helping you do your taxes, you’ve likely been underwhelmed by the initial results. However, approaching a chatbot for a conversation without a specific objective, armed only with curiosity and a playful attitude, might have inspired solutions for problems you didn’t even know you had or sparked ideas for approaching a problem space differently. This concept of serendipitous innovation unbounded by objectives isn’t new and didn’t arise with the advent of generative AI. We have all likely heard the stories of accidental discovery of inventions like the microwave or penicillin. Yet, the seemingly boundless array of applications of AI technology, particularly generative AI, promises a significantly greater potential for unexpected discoveries.
Despite the seemingly unpredictable and uncontrollable nature of serendipity, the topic has received a surge of recent attention in the field of management studies. In a literature review of serendipity in an organizational context, economics professor Christian Busch defines serendipity as a “surprising discovery that results from unplanned moments in which our decisions and actions lead to valuable outcomes.”1 Serendipity requires individual agency , an element of surprise , and a recognition of a discovery’s value. Through his synthesis of the literature, Busch posits that organizations can cultivate serendipity by creating an environment that encourages experimentation and collaboration and by communicating the organization’s problem area at a broad enough level of specificity that allows individuals to associate value to their discoveries.
In libraries, where technological innovation is often restricted to departments with access to grants and IT resources, AI’s potential for rapid ideation, coupled with the increasing accessibility of these tools to the consumer market, could be a gamechanger for materializing serendipitous discoveries across organizations. As libraries face the threat of drastic transformation through AI’s upending of search, discovery, and publishing, empowering more diverse groups of individuals to collaborate and experiment could accelerate the discovery of novel ideas for reimagining library services, data, and technology that will help users traverse this new information landscape. For highly siloed, objective-driven organizations, however, the shift needed to broaden the innovation space may be challenging to make. So how might libraries cultivate an environment where serendipitous discoveries are not only encouraged, but nourished and matured into library operations?
From my participation on the team building Indiana University’s Audiovisual Metadata Platform (AMP), I can share an example that illuminates how an openness to serendipitous discovery helped us reframe a problem space. The original hope of the AMP project was to extract metadata from digitized and previously undescribed audiovisual materials, but in testing AI tools for this purpose, we often found them falling short of our expectations. We hoped they would provide descriptive information useful for cataloging materials, but their poor accuracy and consistency made it challenging to confidently integrate them into the platform. However, through this process of experimentation and testing, we serendipitously discovered other valuable functions they could serve. For example, In exploring human-readable outputs of video shot detection algorithms — which identify the start and end times of every shot in a video — we experimented with a contact sheet format that displays a thumbnail image from each shot, arranged in order on a grid. Although this tool did not seem to offer direct benefits for video cataloging, we found, through discussions with collection managers on the team, that the contact sheets could be extremely helpful to them for other tasks. This was especially true in environments where making video accessible to staff early in the processing pipeline is technically challenging. With the availability of machine-generated contact sheets, archivists processing newly digitized materials could quickly get a sense of what is contained on unmarked media or assess how much of a tape or reel was used for recording, without needing to scrub through the entire video. Copyright specialists could efficiently scan the contact sheets to identify any potential intellectual property or performance rights needing deeper review. Researchers, too, could benefit from visually skimming frames from video content that copyright restrictions prevented them from viewing remotely, helping them decide if a trip to the reading room was worth their time.
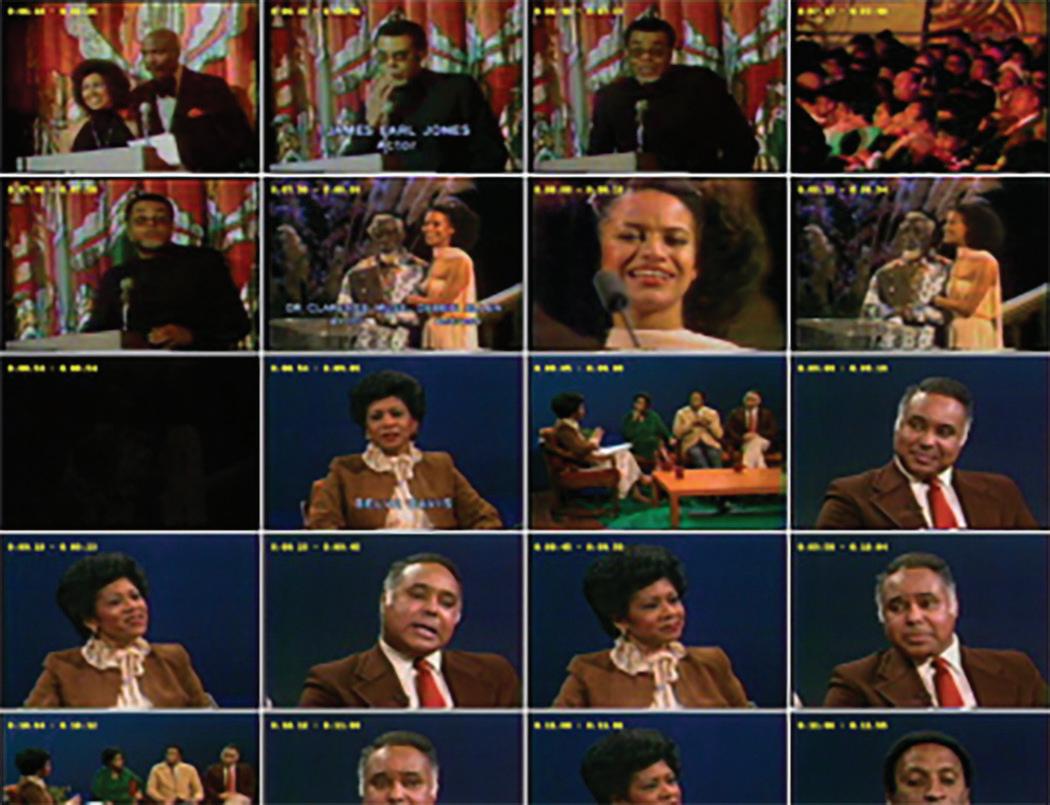
Soon, the team found ways to make other AI tools, which had performed poorly at their originally intended tasks, more useful by displaying their outputs in the contact sheet format. Video OCR, which transcribes text like signs or title screens from videos, was often inaccurate, and its JSON outputs were difficult to structure meaningfully. However, it excelled at identifying which frames in the video contained text, which could then be displayed as thumbnails on a contact sheet for humans to manually transcribe. Facial recognition, fraught with flaws and potential for bias, could still be used to find frames with faces for human review on a contact sheet. The potential of the entire AMP platform, initially envisioned as a metadata creation pipeline that would input undescribed media and output basic catalog records with minimal human mediation and feedback, expanded to become a workbench for the full processing and description lifecycle, its primary strength not in replacing human labor and expertise but in supporting and enhancing it. This unexpected discovery and recognition of the value of contact sheet functionality for solving other problems contributed to our greater goal of improving the efficiency of audiovisual cataloging, just not in the ways we had anticipated.
Recent experiments supported and hosted by the Library of Congress can show us how AI experiments without narrow purposes in mind can provide tools to spark new discoveries. At the Table with: Mary Church Terrell, an experiment led by interns Ide Amari Thompson and Madeline Toombs, offers an interface for the random discovery of creative prompts extracted through natural language processing from the Library’s collection of writings by civil rights activist and suffragist Mary Church Terrell. While imagined as a method to support creatives in the generative process of writing, this alternative entryway into library collections sets a stage for limitless surprise and potentiality. Starting from prompts removed from their context, like “Let them not perish of heart hunger for want of the crumb of comfort which it is in our power to bestow,” and “Remove Mary’s adenoid,” users can then dive back into the source material to learn more. While the interface may prove difficult in supporting a traditional research approach, this AI-curated randomizer provides an experience ripe for serendipitous discovery of new research or creative methodologies.
Organizations often resist solutions in search of problems, aiming instead to prove their value by strategizing with goals and objectives and measuring success with actions and key performance indicators. This approach identifies many problems in need of solutions, with employees proving their worth by aligning their work with these goals, leaving little room for exploration beyond their assigned problem space. In our consulting work, my team is usually brought into organizations to address specific problems. Even the human-centered design process we use in our work focuses on defining the right problem to solve and then ideating possible solutions to it. So how might we awaken and harness the latent potential for serendipitous innovation in AI while still achieving our goals, meeting our users’ needs, and satisfying our stakeholders?
Despite its emphasis on solving the right problem, human centered design (HCD), or design thinking, can lay the groundwork for fostering a culture of serendipitous innovation. HCD is a framework for people-focused experimentation and problem-solving, built on four principles: centering on people, solving the right problem, thinking in systems, and developing iteratively. Throughout its six-stage process — empathize, define, ideate, prototype, test, and implement — practitioners alternate between divergent thinking to explore possibilities and convergent thinking to narrow focus and direction. While applying the HCD process from start to finish on specific problems can be an effective method for generating solutions, exploring elements of the framework at the organizational level can help to set the stage for organization-wide AI experimentation.

In the Empathize phase, we seek to understand the humans affected by a problem who will benefit from our solutions — their experiences, needs, desires, pains and challenges. If we consider the organization’s mission to be one broad problem area, we can use methods from the Empathize phase to better understand and communicate the breadth of needs of our users, communities, and staff (which could potentially be served by AI, amongst other solutions). If your organization has not yet developed a complex understanding of your users, their needs, and how you can serve them, now is a good time to start. Techniques used in the Empathize phase, like interviews, focus groups, shadowing, and persona development, can help to elicit more information not only about the goals your users are trying to achieve but also the challenges they face. It will also be important to understand your users’ constraints and vulnerabilities as you encourage broader solutioning across the organization, particularly when experimenting with AI, so you can continue to provide a safe space and an environment of trust and accountability. Consider building on your foundational user research through organization-wide workshops or focus groups to identify how your users and their communities might be vulnerable to AI harms, such as bias, discrimination, and privacy or intellectual property violations, and which specific risks pose the greatest threat to them. Resources such as the IBM Risk Atlas and the Fraunhofer Institute’s AI Assessment Catalog, which detail many types of possible AI risks, can be valuable for sparking these discussions. Future risk assessments of AI technologies you decide to adopt will depend highly upon the specific uses you intend for them. Ensuring broad diversity among your users and staff in this process will reveal more needs and risks and help you prepare to mitigate any unintended consequences of AI.
In the Define phase, our goal is to understand and articulate the core problem that needs solving before we begin ideating possible solutions. This phase is crucial for defining the right problem — one that is narrow enough to allow for actionable solutions but broad enough to accommodate a wide range of creative possibilities. At an organizational scale, this problem might be encapsulated in the mission statement or a strategic plan. Techniques like abstraction laddering can help to achieve the right level of granularity. Frame the mission statement as a problem statement (perhaps starting it with “we need to” or “how might we”) and then ask “how?” to narrow the scope of the problem. Conversely, if starting with a narrower objective, asking “why?” can broaden the scope. For example, asking “why?” to the original AMP problem statement of “how might we extract metadata from audiovisual materials?” could return the answer “to process and catalog audiovisual materials more efficiently.” This new problem statement, “how might we process and catalog audiovisual materials more efficiently” opens up the problem space to invite many more potential solutions. Expanding and narrowing this problem space for your organization until it includes a wide range of problem statements that reflect your users’ needs can pave the way for serendipitous solutions.
Other techniques and principles from HCD can also encourage the generation of unexpected solutions with AI: Emphasizing iteration and ideation, and allowing staff ample access, time, and space to experiment with new tools without the pressure to solve an immediate problem or produce immediate results. Inviting diverse perspectives can foster more creative solutions, offering staff opportunities to share their findings or collaborate with individuals outside their functional areas in AI experimentation, such as through group prompt writing. Creating sandboxes, tools, and safe environments for staff to use or manipulate data, better understanding of potential risks, and testing new ideas can facilitate innovation without the risk of exposing public users to premature or risky outcomes.
With a solid foundation in understanding user needs, AI risks, and an organizational problem space, organizations may also consider defining an AI values statement, similar to the one crafted by the Smithsonian. Such a statement can guide responsible AI development and use and to open a conversation with the communities they serve. By documenting and effectively communicating these values along with the potential benefits and risks of AI within a clearly but openly defined problem space, libraries can provide staff with guideposts to align their discoveries. As these solutions find their respective problems, they can be integrated into new, more structured cycles of the HCD process using the empathize and define phases to better understand the use case and ideation, prototyping, and testing to explore potential implementation methods and evaluating the solutions’ success and value against an established risk framework.
As libraries begin to navigate the evolving landscape of artificial intelligence, building the capacity and culture for serendipitous innovation offers a unique opportunity to not only revolutionize the way libraries support their users but to test organizational models outside of siloed, objective-driven methods that limit cross-functional collaboration. By embracing HCD methodologies and fostering a culture of curiosity, openminded experimentation, and embracing the unforeseen, libraries can lead the way in utilizing AI not just as a tool for optimizing current practices but as a catalyst for discovering new ways to connect with and serve their communities.
Endnotes
1. Busch, C. (2024), Towards a Theory of Serendipity: A Systematic Review and Conceptualization. J. Manage. Stud., 61: 1110-1151. https://doi. org/10.1111/joms.12890