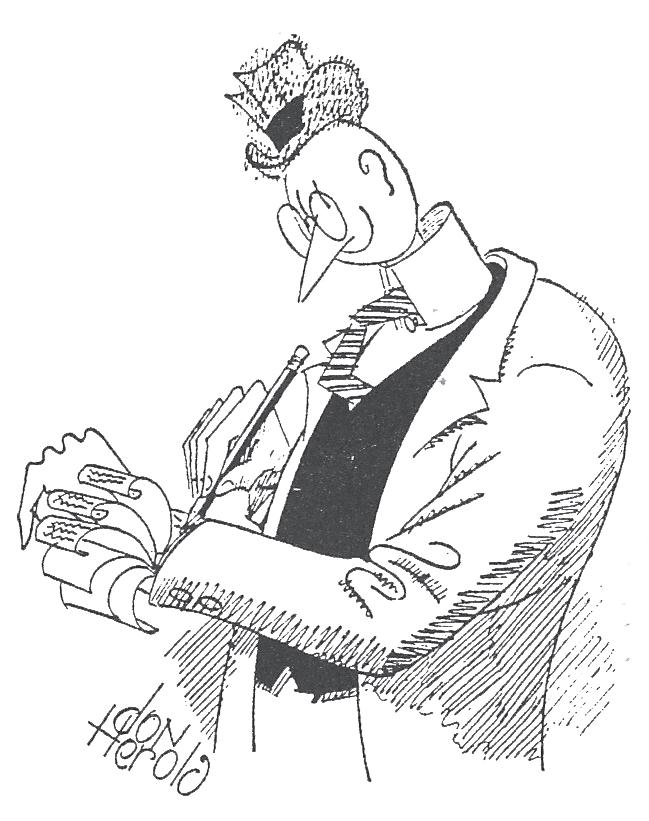
13 minute read
Libraries, AI and Training Collections
By Lorcan Dempsey (Professor of Practice and Distinguished Practitioner in Residence, The Information School, University of Washington)
One reason we are sensitive about genAI (generative AI) is because knowledge and language are central to our sense of ourselves and to the institutions which are important to us. Accordingly, application of genAI raises major questions across the cultural, scholarly and civic contexts that are important to libraries. In this context, I like Alison Gopnik’s characterization of genAI as a cultural technology. Cultural technologies, in her terms, provide ways of communicating information between groups of people. Examples, she suggests, are “writing, print, libraries, internet search engines or even language itself.” She goes on to argue that asking whether an LLM is intelligent or knows about the world is “like asking whether the University of California’s library is intelligent or whether a Google search ‘knows’ the answer to your questions.” She reminds us that previous cultural technologies also caused concerns, referencing Socrates’ thoughts about the effect of writing on our ability to remember and as a potential spreader of misinformation. And she also notes that past cultural technologies “required new norms, rules, laws and institutions to make sure that the good outweighs the ill, from shaming liars and honoring truth-tellers to inventing fact-checkers, librarians, libel laws and privacy regulations.”
GenAI is in very early stages. It is a technology which operates on representations of human knowledge, on web pages, documents, images and other knowledge resources. It transforms such inputs into a statistical model which can be used to generate new representations in response to inputs. As such, it is a cultural technology which can not only be used to discover, summarise or otherwise operate with existing knowledge resources, but can be used to generate new resources. Such generation is imitative but is potentially significant in various ways.
Many expect it to become a broad-based cultural technology as an integral part of the systems and services which structure workflow, knowledge consumption and exchange, and commerce. If this is so, then we can expect to see evolution in several directions. We will see new “norms, rules, laws and institutions” which guide use. These are influenceable, and I argue elsewhere that library organizations should be pooling their influence and attention for strongest impact.
It also means that there will be increasing focus on the resources, the representations of knowledge, from which the statistical models that power the LLMs are created. The first generation of genAI, and the foundational LLMs that power the services from the major players, are based on large training collections opportunistically assembled from broad web scrapes, large content aggregations, and readily available knowledge resources (Common Crawl, Wikipedia, Project Gutenberg, …). In a delightfully presented discussion , Knowing Machines outlines how such LLMs are far from being a raw record of the available cultural record. The record is distorted by algorithmic manipulation at scale (what Knowing Machines calls “curation by statistics”), commercial and SEO interests in how web pages and images are presented, and other artifacts of their construction, selection and processing.
Appraisal of knowledge resources will become progressively more important as the focus on mitigating bias, on quality of response, and on richness of content and context increases.
There is a double dynamic here, which is of central importance to those who have historically curated the scholarly and cultural record, including libraries. Existing providers of LLMs will want to use high quality knowledge resources to create more useful training data, to improve their models and the services based on them. We can see this at play in the discussions between AI companies and major providers of knowledge resources (e.g the New York Times or Reddit). Some of these discussions are being pursued in commercial negotiation, some are being pursued in the courts as questions over the boundaries of authorized use of content are litigated. The New York Times recently reported some of the ways in which the big providers are trying to extend the reach and raise the quality of materials in their training collections. OpenAI, for example, converted YouTube audio to text, and added the transcripts to the training collection of one of its models. Apparently, the NYT reports, Meta considered acquiring Simon & Schuster as a source of high-quality text.
Second, providers of knowledge resources will want to benefit from this latest cultural technology themselves, preferably in controlled ways. This may mean licensing data to the large players, creating LLMs themselves (if they have the scale), or participating in emerging cultural, scholarly or other missionaligned initiatives. Of course, there will be multiple possible service configurations. For example, several providers in our space are experimenting with retrieval augmented generation, combining searches in their own resources with the processing capacity of LLMs. Another example is use of the custom GPTs that OpenAI recently made available, where a provider can load their own data to work alongside GPT. The Dimensions GPT from Digital Science is an indicative example. Digital Science emphasizes trust through the “combination of scientific evidence and generative AI.”
I was tempted to call the representations that are used to train large language models “training libraries” here, to highlight that they are selected and can be curated to a greater or lesser degree. It seems to me that the use of the phrase “training data” does not signal the discretion in what is collected. It is more technocratic, somehow signaling that it is neutral, routine and under control. However, “collection” might be better, as it does not have the connotation of library, but does acknowledge the selective activity of collecting.
I recently discussed several initiatives to create specialist LLMs based on curated training collections of scholarly and cultural materials. The National Library of Sweden, for example, has created training collections based on Swedish cultural materials. The Paul Allen Institute has developed a training collection, Dolma, based on various freely available collections of material to support its scholarly large language model, OLMo This includes data sets commonly used by others, but it also includes data from a large collection of open access articles (the Paul Allen Institute produces Semantic Scholar). I also noted how Bloomberg, Forbes and others were leveraging their archives.
A particularly interesting case is provided by the BBC, whose historical archives represent a deep cultural and social resource of potentially major interest. They are cautiously discussing the addition of this resource to the training collections used by the large technology firms developing foundation models. However, they are also looking at creating their own large language models for internal use. This dual approach may become more common, as organizations consider how best to participate in this emerging cultural technology.
Here, I want to focus briefly on two potential training collections related to library interests. The first involves knowledge resources created or controlled by the library; the second relates to the scholarly literature, where the library is a partner and a consumer. My perspective is prospective.
Libraries, archives and related organizations have invested major intellectual effort in several areas over many years. In each case, there is significant contextual or relationship data which could be amenable to this latest cultural technology processing. Here are three examples. First is LibGuides. The aggregated LibGuides collection contains many resources contextualized by subject, course and other attributes. I will be interested to see whether Springshare, who provide LibGuides, works with the contributing libraries to see what might be possible. Is this a deep enough resource to be of interest? I am not sure, but it would be interesting to know what services based on it look like, whether this is in conjunction with GPT (or another LLM) or not.
A second is around bibliographic infrastructure and associated resources. OCLC has invested in a linked data infrastructure around WorldCat and associated vocabularies. HathiTrust curates a large collection of full texts, which are described by WorldCat. What would a library built from WorldCat and HathiTrust be like? How useful would it be? What about WorldCat, HathiTrust and Jstor? This is unlikely to happen without some major external stimulus, and it is unclear what the organizational context of output would be, but it seems like it is worth a discussion? An interesting question in this context is whether Google books is already mobilized within Gemini, the Google LLM?
The third area is maybe the most intriguing as it is the least replicable elsewhere. In aggregate, libraries and archives have a massive collection of finding aids, rich contextual, hierarchical data. There are some extant aggregations of finding aids and related data in Calames, in France, for example, or in the Archives Hub and ArchiveGrid in the UK and US respectively. There is also a range of regional and other aggregations, such as Archives West, for example. This data is not always highly structured. And of course, it is very far from complete, at the institutional level and at the aggregate level. But thinking about a large aggregation of finding aids and related data as a training collection extends the ongoing discussion about discovery. For example, a recent major collaborative study, NAFAN (Building a National Finding Aid Network), explored the feasibility of a national US approach to archival discovery, understanding the gaps in coverage and other issues.
To be very clear, I am not suggesting the AI-assisted creation of finding aids here; my interest is in training collections and LLMs. I am thinking about amplifying the existing discussion about how their existence is effectively disclosed and how the intellectual investment in description is mobilized. How to make them more valuable. Of course doing something like this would be a major undertaking, not least because of the major collective action problem it poses. While many people might like to see this, it is difficult to individually advance it. The conjunction of an existing archival discovery question with the possibility of AI amplification, however, does rise to the level of a community grand challenge, which poses three initial questions. The first is where to secure the funding to get something like this off the ground. The second is one of agency, both in any startup phase but also from an operations and business perspective going forward. The third is securing post start-up funding. As a community, we are not good at sustaining large scale infrastructure as a community asset. While one could imagine some income from licensing a resource like this to the foundational LLM providers, it is unlikely to sustain an operation over time. Again, I think that a discussion about this might be advanced by several key national organizations. While individual libraries will have materials harvested by the LLM providers, it would also make sense to think about how one would advance a community discussion about infrastructure and organized disclosure. The NAFAN partners may be a basis for some discussion, but the potential interest goes beyond this to other organizations and funders.
My second example is less speculative; indeed components of it are already in place. In recent years, we have seen the emergence of three organizations which I have called “scholarly communication service providers.” These are Elsevier, Digital Science (a part of Holtzbrinck Publishing Group, also the majority owner of Springer Nature), and Clarivate. These organizations share several characteristics. They have the scale and expertise to do work in this space. They curate major reservoirs of scientific literature, representing a significant part of the scientific record. However, their interests are not just in the published record, they are interested in a full characterization of the scientific enterprise. To that end, they also curate a variety of other entities (people, for example, institutions, funding, methods, and so on) and their relationships. Each has made a significant investment in building out a research graph, manifested in various products. They have also built a range of workflow services, supporting researcher behaviors and the creation and management of data about these research entities. They provide research and university analytics services, leveraging their data assets to provide intelligence to universities and departments about their own research performance and how it compares with others. Each has also been making announcements about AI adoption and partnership.
There are other players here, notably the Paul Allen Institute, which I have already mentioned, which has the expertise, connections and pockets necessary to make an impact.
However, the three organizations above are especially interesting in our space given the range of their involvements and the variety of ways in which the scholarly community, including libraries, already relies on their services. Clearly, we are seeing some incremental changes as AI gets incorporated in discovery and related services. This will continue. We are likely to see summarization, literature reviews, recommended reading or relevant areas of study. It will be interesting to see what sort of analytics services emerge. And beyond that there is clearly scope for new services.
The scale, data and reach that these three organizations have mean that they will be able to create important new services, based on rich training collections of bibliographic, profile, and other data. This raises some questions for libraries and universities. One is the extent to which they are willing to have the data from their workflow solutions (e.g., research information management systems, research data management systems, library systems) flow into the training collections. A second is around trust in black boxed outputs, not only in discovery contexts but in decision-making contexts. That said, these companies share a compelling interest in developing approaches which inspire confidence in outputs. However, it also raises the prospect of interacting with scientific knowledge in different ways, facilitated by new cultural technologies. This potentially shifts the open access debate in important ways. The focus of the open access discussion has been on the article, the journal and the package. If an important element of access to scholarly knowledge is through new genAI-enabled services, then who has access to them and under what conditions becomes a central question. The open access discussion shifts to the LLM or the AI-enabled configuration.
GenAI appears to be an important cultural technology. Emerging training collections are core to its operation. The large foundation models will continue to grow, alongside more specialist resources which might be used in various configurations. Transparency in the construction of training collections has emerged as an important consideration. Understanding and explaining the construction of training collections will be an important part of what libraries might do, especially where they are licensing services based upon them or seeing data from their institutions go into them. However, it would be good to be more actively involved in this important cultural technology. This poses a collective action question, which makes it critical for those who act in the collective library interest to develop frameworks in which libraries can work together.
Endnotes
1. Sometimes by bending or possibly breaking rules as discussed in this NYT article
2. https://www.accessdata.fda.gov/drugsatfdadocs/label/2024/217686s000lbl.pdf
3. Such as TEI XML or JATS XML.