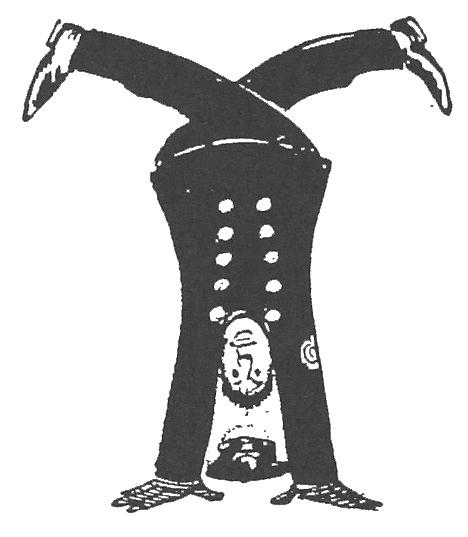
16 minute read
Some Next Generation Legal Issues for Training Generative Technologies
By Brandon Butler (Executive Director, ReCreate Coalition)
Introduction
Generative technologies1 like large language models and diffusion models can generate startlingly advanced textual and visual creations based on relatively minimal human prompting. Versions of these technologies have been around for years, but the high-profile release in the last year or two of some especially powerful consumer-facing tools — ChatGPT and Stable Diffusion, in particular — created a stir in the popular imagination. They also prompted a storm of litigation as copyright holders sought to block or license the use of in-copyright materials to “train” these technologies as well as any allegedly infringing works they may create. For their part, technology companies have already collected and exploited massive amounts of data from the open internet without asking permission, and their thirst for data has them looking at whether and how they can find more training data.2
Current litigation will likely provide useful answers to the two threshold questions that every “what’s up with AI and copyright?” explainer must inevitably cover:
1) Are the creators of LLM and diffusion models within their fair use rights (more specifically within the line of fair use case law that permits computer processing of in-copyright works for “transformative” purposes) when they use in-copyright works as “inputs” to train these models?
2) Under what circumstances do the “outputs” of these models infringe on the copyrights of existing works?
The most likely answers are “Generally, yes,” and “The same circumstances as when a human author’s work is infringing, i.e., when the output is substantially similar to an in-copyright work.” Judges are human, of course, and juries even more so; they can zig when you expect them to zag. But my interest here is in what happens next. Assuming we get those most likely answers or something close enough that this technology continues to develop without major copyright constraints, what follows is a brief tour of what we might call the next generation of legal questions and challenges for training generative technologies. Fundamentally, these are questions about whether and how creators and controllers of data (understood broadly to mean any kind of information that could be used in training, not just, and not typically, numerical data) can block, monetize, or otherwise shape the use of that data in training generative models. After exploring some of the strategies they might try under current law, I’ll discuss briefly whether any of this is good policy.
After Copyright – Publishers’ Legal Tools for Controlling, Blocking, and Monetizing Generative Technology Training
Creators and (more often) aggregators and monetizers of content that could be used in the development of generative technologies have a variety of tools at their disposal to control access and reuse. Some of these tools are self-help, but most have an element of legal enforceability, creating at least a risk of liability for those who ignore, circumvent, or defy them. Generative technology developers are likely to run into each of these in their search for data to refine and improve their tools.
Scraping publicly accessible websites is a popular way to gather training data, but a tangle of potentially overlapping legal provisions awaits the would-be scraper. Recent litigation seems to have taken the federal anti-hacking statute (the Computer Fraud and Abuse Act) mostly off the table; this is welcome news, but its import has been wildly exaggerated. As one expert has quipped, “If you’re looking for legal issues, web scraping is a hornet’s nest on top of a beehive on top of a wasp’s nest resting on an anthill with scorpions.”3 Breach of contract, trespass to chattels (the equivalent of trespassing on private land, but for other property like servers), and any number of state law tort claims (civil claims that can arise when someone’s wrongful action causes harm to another, including to their reputation, privacy, or livelihood) are all still live possibilities. If “training” generative tools is held to be fair use, technology developers may argue that federal copyright law preempts these other legal claims, but the success of that argument will depend on the facts in a specific case.
Beyond the open web lies the vast sea of content kept behind a paywall, typically subject to express agreement (i.e., the user has to affirmatively acknowledge, truthfully or not, that they have read, understood, and agreed) with terms of use as a condition of access. Commercial STEM publishers, increasingly many news outlets, and commercial streaming platforms will all fit into this category. Terms of use on open websites may be subject to challenge on the grounds that users are not on notice of their terms, do not give consent, or both; terms imposed on paywalled content are not likely to be vulnerable to such challenges. Universities have already drawn attention to major publishers like Lexis adding or highlighting “no AI” language in their licenses and user agreements.4 Whether such provisions can override copyright limitations and exceptions like fair use is an unsettled question in the law, and one with increasingly broad and pressing implications for libraries and other research institutions.5 The impact of contracts on the otherwise lawful development and use of generative technologies can be added to the list of concerns.
Two other forms of rightsholder “self-help” got a boost in the late 1990s from the Digital Millennium Copyright Act: so-called “technological protection measures” (TPMs) and “copyright management information” (CMI). TPMs are like digital locks placed on copies of works to control access and use; examples include encryption and the use of authentication servers. CMI includes watermarks and digital fingerprints that identify the copyright owner of a work. TPMs and CMI may enable rightsholders to prevent or track use of their materials and removing these measures (which can be technically simple and may even happen inadvertently) can trigger legal liability. A special triennial rulemaking hosted by the U.S. Copyright Office can grant exemptions that let certain users break TPMs for lawful purposes,6 and the Office has granted exemptions related to text and data mining,7 but there is not currently an exemption that would clearly permit circumvention of TPMs for the purpose of training generative technologies. And since a rulemaking cycle is currently underway, no such rule will exist before October 2027, at the earliest.
Some technology developers may not break locks or breach user agreements themselves, but they may use training data released by individuals or groups who did. Indeed, plaintiffs in some of the lawsuits regarding this technology allege that some major generative tools have been trained partially on data derived from so-called “shadow libraries” of in-copyright books made available online without the copyright holder’s permission — entities like Bibliotik, Library Genesis, or Z-Library. The “books3” dataset is the primary example of training data drawn from these sites, and journalists report that several major models have been trained with that data.8 This could be an issue for technology developers if the courts decide to consider the origin of the data as part of their fair use analysis, counting arguably ‘ill-gotten’ data as a sign of “bad faith.” The Supreme Court wrote in Harper & Row v. The Nation, Inc. that “fair use presumes good faith and fair dealing,” and penalized The Nation for relying on a “purloined manuscript” as the source of excerpts it published from a forthcoming book.9 Scholars and courts (including the Supreme Court itself in a later case10) have cast doubt on the validity of the “good faith” factor, but it lives on.11 If courts decide to give weight to the factor, the use of contracts and technical security measures could become an effective bar to fair use, even against users who do not breach contracts or circumvent digital locks.
Policy Implications: the End of Copyright?
Commentators sometimes suggest that digital technologies like these could spell “the end of copyright” if they can operate free of copyright constraints due to fair use. The previous section raises the specter of an opposite outcome: that the use of paywalls, passwords, contracts, and common law torts could spell the end of the centuries-long balance copyright strikes between the protection of expression on one hand and the free and fair circulation and reuse of facts, ideas, and information on the other.
This is not the first time that unfettered copying has made data publishers nervous. Decades before the Authors Guild v. Google case (in which the Second Circuit held that fair use permits copying millions of in-copyright books to create a search tool, among other things), the Supreme Court faced the question of whether and how to apply copyright to the wholesale copying of large collections of factual information. The publishers of factual databases argued for years that because of the effort involved in gathering, organizing, and publishing factual data (things like sports statistics, market performance data, and even address information), they should be entitled to copyright protection in the resulting databases. Under this theory, the first entity to gather and publish data could block others from extracting and sharing information from them without payment or permission. According to this theory, also known as the “industrious collection” or “sweat of the brow” doctrine, protection is appropriate to prevent “free riding” and ensure adequate incentives for the creation of new databases. Until 1991, U.S. courts were divided on this theory, but the Supreme Court settled the issue by rejecting “sweat of the brow” in Feist v. Rural Telephone Co., an opinion about warring publishers of telephone directories.
After explaining that as a Constitutional matter, copyright only protects an author’s original expression, and not any facts or ideas that may be conveyed in that expression, Justice O’Connor explains the underlying policy for this treatment:
It may seem unfair that much of the fruit of the compiler’s labor may be used by others without compensation. As Justice Brennan has correctly observed, however, this is not “some unforeseen byproduct of a statutory scheme.” It is, rather, “the essence of copyright,” and a constitutional requirement. The primary objective of copyright is not to reward the labor of authors, but “[t]o promote the Progress of Science and useful Arts.” To this end, copyright assures authors the right to their original expression, but encourages others to build freely upon the ideas and information conveyed by a work. … This result is neither unfair nor unfortunate. It is the means by which copyright advances the progress of science and art.12
Justice Brandeis gave equally eloquent expression to this general principle in his dissent in Int’l News Service v. Associated Press: “The general rule of law is that the noblest of human productions — knowledge, truths ascertained, conceptions, and ideas — became, after voluntary communication to others, free as the air to common use.”13
This principle — that copyright protects the author against unfair circulation of her expression, not of the facts and ideas it conveys — explains why copyright cannot (and should not) protect rights holders from some kinds of competition by generative technologies. For example, a generative tool whose training included books about Franklin D. Roosevelt might be able to answer a factual question like “When was Franklin D. Roosevelt stricken with polio?” No doubt its ability to do so would be the result of the inclusion of this fact in some of the works in its training data, and for some users of the tool this answer will obviate the need to buy any of the works represented in the data. But, as Judge Leval explained in his opinion in the Google Books case, when a book search result (a “snippet” of a book’s text) reveals the answer to a factual question like this: what the searcher derived from the snippet was a historical fact. [The] [a]uthor[’s] … copyright does not extend to the facts communicated by his book. It protects only the author’s manner of expression.… [I]t would be a rare case in which the searcher’s interest in the protected aspect of the author’s work would be satisfied by what is available from snippet view.14
So if the user of a generative tool is satisfied by its reproduction of facts and information derived from a protected work, that’s not a substitution effect that copyright protection has ever or should ever prevent. A new biography of a major political or cultural figure may include significant, newlydiscovered, difficult-to-unearth facts that the author hopes will entice readers to buy the book. Nevertheless, the day it comes out, any diligent Wikipedia editor is free to transpose all those juicy facts over to the subject’s Wikipedia entry, making all those hard-won facts easily and freely available to all. Tabloids, social media personalities, and even the New York Times all benefit from this freedom to circulate, discuss, and build on facts and information revealed in others’ in-copyright works. Generative tools are only the latest mechanism to take advantage of the law’s recognition that facts and ideas should spread as freely as Thomas Jefferson’s famous candle flame.15
At the same time, generative tools will have to play by the same copyright rules as everyone else when they answer these factual questions — they cannot offer up answers that are substantially similar to the expressive elements of their training data. So, to the extent that the value of an author’s work derives from her writing, rather than her diligent collection of facts, that value is still protected by copyright.
The courts will likely rule that development of generative technologies is generally protected by fair use, in part because they provide the public with increased access to unprotected elements of in-copyright works — facts and ideas that have always been “free as air to common use.” If so, the protectionist legal tricks described above would be, if effective, nothing less than circumventions of the Constitutional design of copyright.
As this article went to press, the federal court in the Northern District of California issued an opinion that gives reason for hope that copyright’s balance may survive the war over generative tech. In X Corp. v. Bright Data Ltd., 3:23-cv-0369816 (N.D. Cal. May 9, 2024), Judge Alsup rejected attempts by the social network formerly known as Twitter to block scraping of public data using several of the non-copyright theories canvassed above — breach of contract, trespass to chattels, and state competition law. The court found that none of these claims could block Bright Data from scraping public data from X’s website. Most importantly, the court found that federal copyright law preempts X’s attempt to “yank into its private domain and hold for sale information open to all.” The court also relied heavily on its finding that X could not show any cognizable harm from Bright’s scraping. In essence, the court ruled that if the only interest being vindicated by a legal claim is the interest in controlling the collection and reuse of information, that interest should be settled by copyright law. Here’s hoping other courts take note.
Endnotes
1. I use the term “generative technologies” as a bit of a provocation here in an attempt (in vain, too late, but still!) to avoid repeating terms like “artificial intelligence” and “machine learning” that can obscure as much as they reveal about these tools. See generally Emily Tucker, Artifice and Intelligence, Center on Privacy & Technology at Georgetown Law (Mar. 8, 2022), https://medium.com/ center-on-privacy-technology/artifice-and-intelligence%C2%B9-f00da128d3cd (last visited Jun 16, 2023)(characterizing “artificial intelligence” as “a phrase that now functions in the vernacular primarily to obfuscate, alienate, and glamorize.”).
2. See, e.g., Cade Metz, Cecilia Kang, Sheera Frenkel, Stuart A. Thompson and Nico Grant, How Tech Giants Cut Corners to Harvest Data for A.I., The New York Times, April 6, 2024, https://www.nytimes.com/2024/04/06/technology/tech-giants-harvest-dataartificial-intelligence.html.
3. Kieran McCarthy, Hello, You’ve Been Referred Here Because You’re Wrong About Web Scraping Laws (Guest Blog Post, Part 2 of 2), Technology & Marketing Law Blog (2022), https://blog.ericgoldman.org/archives/2022/12/hello-youve-been-referred-herebecause-youre-wrong-about-web-scraping-laws-guest-blog-post-part-2-of-2.htm (last visited Mar 13, 2023).
4. The Office of Scholarly Communication at the University of California, Berkeley, has provided important leadership in pushing back on this effort. See, e.g., Rachael Samberg, Tim Vollmer and Samantha Teremi, Fair use rights to conduct text and data mining and use artificial intelligence tools are essential for UC research and teaching, Mar. 12, 2024, https://osc.universityofcalifornia. edu/2024/03/fair-use-tdm-ai-restrictive-agreements/.
5. See generally Katherine Klosek, Copyright and Contracts: Issues and Strategies (2022), https://www.arl.org/wp-content/ uploads/2022/07/Copyright-and-Contracts-Paper.pdf
6. The Copyright Office hosts a website with information about these rules, including current rules, an explanation of the rulemaking process, and an archive of materials related to past rulemakings: http://copyright.gov/1201
7. The Authors Alliance led the effort to secure this exemption, and they provide helpful information about it. Authors Alliance, Update: Librarian Of Congress Grants 1201 Exemption To Enable Text Data Mining Research, Oct. 27, 2021, https://www. authorsalliance.org/2021/10/27/update-librarian-of-congress-grants-1201-exemption-to-enable-text-data-mining-research/.
8. Kyle Barr, Anti-Piracy Group Takes Massive AI Training Dataset “Books3” Offline, Gizmodo, Aug. 18, 2023, https://gizmodo.com/ anti-piracy-group-takes-ai-training-dataset-books3-off-1850743763.
9. Harper & Row, Publishers, Inc., et al. v. Nation Enterprises, et al., 471 U.S. 539 (1985).
10. See Campbell v. Acuff-Rose, 510 U.S. 569, 585 n. 18 (1994) (noting range of opinions on relevance of “good faith,” including its complete rejection by Judge Pierre N. Leval in the same law review article the Court relied upon heavily for its fair use analysis elsewhere in the Campbell opinion, but not endorsing any position).
11. See generally Frankel, Simon and Kellogg, Matt, Bad Faith and Fair Use (September 1, 2012). Journal of the Copyright Society of the USA, Vol. 60, p. 1, 2013, Available at SSRN: https://ssrn.com/abstract=2165468. I have written elsewhere about this intersection between “good faith” and training generative tools with “pirate data.” Brandon Butler, “Stolen Books,” Bad Faith, and Fair Use, Fair Use Week, https://sites.harvard.edu/fair-use-week/2024/02/26/fair-use-week-2024-day-two-with-guest-expert-brandonbutler/
12. Harper & Row, 499 U.S. at 349-50 (internal citations omitted).
13. International News Service v. Associated Press, 248 U.S. 215, 250 (1918).
14. Authors Guild v. Google, Inc., 804 F.3d 202, 224 (2d Cir. 2015).
15. Letter from Thomas Jefferson to Isaac McPherson (Aug. 13, 1813), in 13 THE WRITINGS OF THOMAS JEFFERSON 326, 333–35 (Andrew A. Lipscomb ed., 1903) (“He who receives an idea from me, receives instruction himself without lessening mine; as he who lights his taper at mine, receives light without darkening me.”) Deliciously, recent scholarship reveals that Jefferson’s candle simile is itself a bit of light that he took almost verbatim from Cicero’s De Officiis. See Jeremy N. Sheff, Jefferson’s Taper, 73 SMU L. Rev. 299 (2020).
16. https://blog.ericgoldman.org/archives/2024/05/elon-musks-gifts-to-web-scrapers-guest-blog-post.htm